- 1First Clinical Medical College, Guangzhou University of Chinese Medicine, Guangzhou, China
- 2Department of Gastroenterology, Baiyun Branch of the First Affiliated Hospital of Guangzhou University of Chinese Medicine, Guangzhou, China
- 3Department of Gastroenterology, The First Affiliated Hospital of Guangzhou University of Chinese Medicine, Guangzhou, China
Objectives: The purpose of this study was to investigate the role of 13 m5C-related regulators in colon adenocarcinoma (COAD) and determine their prognostic value.
Methods: Gene expression and clinicopathological data were obtained from The Cancer Genome Atlas (TCGA) datasets. The expression of m5C-related regulators was analyzed with clinicopathological characteristics and alterations within m5C-related regulators. Subsequently, different subtypes of patients with COAD were identified. Then, the prognostic value of m5C-related regulators in COAD was confirmed via univariate Cox regression and least absolute shrinkage and selection operator (LASSO) Cox regression analyses. The prognostic value of risk scores was evaluated using the Kaplan-Meier method, receiver operating characteristic (ROC) curve. The correlation between the two m5C-related regulators, risk score, and clinicopathological characteristics were explored. Additionally, Gene Set Enrichment Analysis (GSEA), Kyoto Encyclopedia of Genes and Genomes (KEGG) pathways, and Gene Ontology (GO) analysis were performed for biological functional analysis. Finally, the expression level of two m5C-related regulators in clinical samples and cell lines was detected by quantitative reverse transcription-polymerase chain reaction and through the Human Protein Atlas database.
Results: m5C-related regulators were found to be differentially expressed in COAD with different clinicopathological features. We observed a high alteration frequency in these genes, which were significantly correlated with their mRNA expression levels. Two clusters with different prognostic features were identified. Based on two independent prognostic m5C-related regulators (NSUN6 and ALYREF), a risk signature with good predictive significance was constructed. Univariate and multivariate Cox regression analyses suggested that the risk score was an independent prognostic factor. Furthermore, this risk signature could serve as a prognostic indicator for overall survival in subgroups of patients with different clinical characteristics. Biological processes and pathways associated with cancer, immune response, and RNA processing were identified.
Conclusion: We revealed the genetic signatures and prognostic values of m5C-related regulators in COAD. Together, this has improved our understanding of m5C RNA modification and provided novel insights to identify predictive biomarkers and develop molecular targeted therapy for COAD.
Introduction
Changes in gene expression are closely associated with the development of disease, and epigenetic processes are heritable changes in gene expression that do not alter the nucleotide sequence (Wu and Morris, 2001). Traditional epigenetic modifications, including chromatin remodeling, DNA methylation, and histone modifications, are involved in various biological processes related to the occurrence and progression of tumors, including gastrointestinal cancers (Dawson and Kouzarides, 2012; Darwiche, 2020; Grady et al., 2021). With considerable progress in zymology and high-throughput sequencing technology, epitranscriptomics has attracted significant attention recently (Angelova et al., 2018; Porcellini et al., 2018; Minervini et al., 2020; Song et al., 2020; Zhao et al., 2020; Schaefer, 2021). Research investigating the physiological and pathological functions of RNA modifications have identified multiple dynamic modifications of RNA, including N6-methyladenosine, 2-O-dimethyladenosine, 5-methylcytosine (m5C), 7-methylguanosine, N1-methyladenosine, and pseudouridylation (Roundtree et al., 2017; Shi et al., 2020). Increasing evidence suggests that RNA modifications play critical roles in tumorigenesis and the progression of different cancers (Barbieri and Kouzarides, 2020; Begik et al., 2020). m5C RNA modification is found in a variety of RNAs, including messenger RNAs, transfer RNAs, ribosomal RNAs. This modification introduces a methyl group in the fifth carbon atom of cytosine (Yu-Sheng Chen et al., 2021). Based on published data, m5C RNA modification plays a critical role in the translation, transport, and stability of mRNAs, and is also closely associated with the biogenesis and function of other RNA species (Xue et al., 2020; Hussain, 2021). As a dynamic and reversible process, m5C RNA modification is primarily regulated by “writers” (adenosine methyltransferases) and “erasers” (demethylases), and achieves different functions by interacting with “readers” (m5C-binding proteins). The “writers” include the NOL1/NOP2/Sun domain RNA methyltransferase family NSUN1-NSUN7 and DNMT2. m5C “erasers” include enzymes in the TET family (TET1, TET2, TET3) and ALKBH1. The “readers”, such as ALYREF and YBX1, recognize and bind to methylated RNAs to realize different functions (Nombela et al., 2021; Xie et al., 2020).
Globally, colorectal cancer (CRC) is the third most common cancer and the second most deadly neoplasm (Bray et al., 2018). Colon adenocarcinoma (COAD) is the most common pathological type of CRC, and despite considerable progress in diagnosis and therapeutic strategies for COAD, the prognosis of patients with COAD remains poor due to advanced stage and postsurgical recurrence (White et al., 2017; Miller et al., 2019). Therefore, the identification of novel biomarkers for early detection and effective therapeutic targets for treating patients with COAD is critical and urgent.
In this study, we analyzed a TCGA dataset for m5C-related regulators involved in COAD, the correlation between the expression levels of 13 m5C-related regulators and clinicopathological features, as well as potential independent prognostic m5C-related regulators and a risk signature to predict the prognosis of patients with COAD.
Material and Methods
Acquisition of Datasets
The RNA-seq transcriptome data (fragments per kilobase million, FPKM) from 437 samples (Mortazavi et al., 2008), copy number variant (CNV) data from 825 samples, single nucleotide variant (SNV) data from 399 samples, and clinical information from 385 patients with COAD in TCGA database (http://cancergenome.nih.gov/) were downloaded for our study. Patients with complete clinicopathological and survival information were included for further assessment (Table 1).
Selection of m5C-Related Regulators
Based on published data, 14 m5C-related regulators, including NOP2 (NSUN1), NSUN2, NSUN3, NSUN4, NSUN5, NSUN6, NSUN7, DNMT2, TET1, TET2, TET3, ALKBH1, ALYREF, and YBX1 were used in our study. DNMT2 was not found to be expressed in COAD from TCGA datasets. Therefore, the remaining 13 m5C-related regulators were used for further analysis.
Tumor Classification and Principal Component Analysis
To explore the function of m5C-related regulators in COAD, a consistent clustering algorithm was used to determine the clustering of samples and estimate the stability of the clustering. Using the “Consensus ClusterPlus” R package (Wilkerson and Hayes, 2010), two different subgroups (cluster Ⅰ and cluster Ⅱ) were identified based on the following classification parameters: 1) slow growth rate of the cumulative distribution function value; 2) high correlation in the subgroup; and 3) no small clusters in the clustering data. Furthermore, principal component analysis (PCA) was used to assess gene expression patterns in different subgroups using the “Limma” R package (Ritchie et al., 2015).
Analysis of Clinicopathological Features and Prognosis
The correlation between m5C-related regulators and clinicopathological features was analyzed. Then, to filter the m5C-related regulators that were highly correlated with overall survival (OS), univariate Cox regression analysis was performed. Next, the Lasso Cox regression algorithm was used to identify m5C-related regulators with powerful prognostic significance. According to the best penalty parameter λ, the selected regulators’ coefficients were calculated. The risk score (RS) was estimated using the following formula:
Where Coef(i) is the coefficient and X(i) represents the expression levels of the selected m5C-related regulators. Using the obtained median risk score as the demarcation value, patients with COAD were classified in two groups: high-risk group and low-risk group. The OS and clinicopathological features were compared between these subgroups. Kaplan-Meier analysis and the receiver operating characteristic (ROC) curves were used to validate the predictive efficiency (Hanley and McNeil, 1982). Additionally, the prognostic value of the RS was verified using univariate and multivariate Cox regression analyses. The hazard ratio (HR) with 95% confidence intervals and log-rank p-value were calculated using the “glmnet” and “survival” R packages (Simon et al., 2011).
Biological Function Analysis
To explore the biological functions associated with m5C RNA modification, Kyoto Encyclopedia of Genes and Genomes (KEGG) pathway analysis, Gene Ontology (GO) analysis and Gene Set Enrichment Analysis (GSEA) were performed. The genes that were differentially expressed between the high-risk group and the low-risk group were functionally annotated using GO analysis and KEGG pathway analysis. Next, GSEA was conducted to determine the signaling pathways related to different clusters. Later, to explore the latent biological function of the m5C-related genes in COAD, GSEA for the m5C-related regulatory genes with powerful prognostic value was performed. The flow chart of bioinformatic analysis was shown in Figure 1.
Cell Culture
The COAD cell lines LS174T and normal colon mucosal epithelial cell line NCM460 were purchased from the American Type Culture Collection (ATCC, Manassas, VA, United States). All cells were cultured in RPMI-1640 medium (Life Technologies, Grand Island, NY, United States) supplemented with 10% fetal bovine serum (Life Technologies) at 37°C in a humidified atmosphere with 5% CO2.
Quantitative Reverse Transcription-Polymerase Chain Reaction (qRT-PCR)
Total RNA was extracted from cells with TRIzol reagent (Invitrogen, China) according to manufacturer’s instruction. Reverse transcription was carried out according to the manufacturer’s instructions using the PrimeScript RT Reagent Kit (Takara, China). The SYBR PrimeScript RT-PCR Kit (Takara) was applied for the analysis of quantitative reverse transcription-polymerase chain reaction (qRT-PCR). Related mRNAs expression levels were calculated using the 2-ΔΔCT method and the related GAPDH mRNA expression was used as an endogenous control. Primers sequences used in our study were as follows: GAPDH forward 5′-GGACCTGACCTGCCGTCTAG-3′, and reverse 5′-GTAGCCCAGGATGCCCTTGA-3′; NSUN6 forward 5′-TTTGCCATCTGCCTTAGT-3′, and reverse 5′-GTGTGTTGTTTTCCCTCC-3′; ALYREF forward 5′-GCAGGCCAAAACAACTTCCC-3′, and reverse 5′-AGTTCCTGAATATCGGCGTCT-3′.
Validation of the Protein Expression Levels of the m5C-Related Regulators via the Human Protein Atlas
To verify the protein expression levels of NSUN6 and ALYREF in COAD and normal tissues, immunohistochemistry (IHC) data were downloaded from the Human Protein Atlas (HPA, http://www.proteinatlas.org). The HPA online database provides IHC expression data for nearly 20 different cancers (Asplund et al., 2012) and enables the validation of the differential protein expression levels between tumor and normal tissues.
Statistical Analysis
The expression data of m5C-related regulators in tumor tissues and adjacent mucosa of COAD obtained from TCGA were compared using one-way analysis of variance (ANOVA); the clinical characteristics and m5C-related regulators of different groups were compared using the chi-square test; the Kaplan-Meier method was used to perform a bilateral logarithmic rank test in overall survival analysis; p-values < 0.05 were regarded as statistically significant. All statistical analyses were implemented using Rv4.0.3 (https://www.r-project.org/).
Results
RNA-Seq Transcriptome Data of m5C-Related Regulators in COAD
Based on RNA-seq transcriptome data of COAD from TCGA database, the expression of 13 m5C-related regulators between tumor tissues and adjacent mucosa was compared (Figure 2). With the exceptions of TET1 and TET3, the expression levels of the other 11 factors were significantly different in the tumor tissues and the adjacent mucosal tissues. Compared with the adjacent mucosa, the expression of NSUN3 (p < 0.001) and TET2 (p < 0.001) in the tumor group was significantly downregulated. The expression of ALKBH1 (p = 0.036), ALYREF (p < 0.001), NOP2 (p < 0.001), NSUN2 (p < 0.001), NSUN4 (p < 0.001), NSUN5 (p < 0.001), NSUN6 (p < 0.001), NSUN7 (p = 0.006), and YBX1(p < 0.001) were significantly upregulated in tumor tissues compared with the adjacent mucosa.
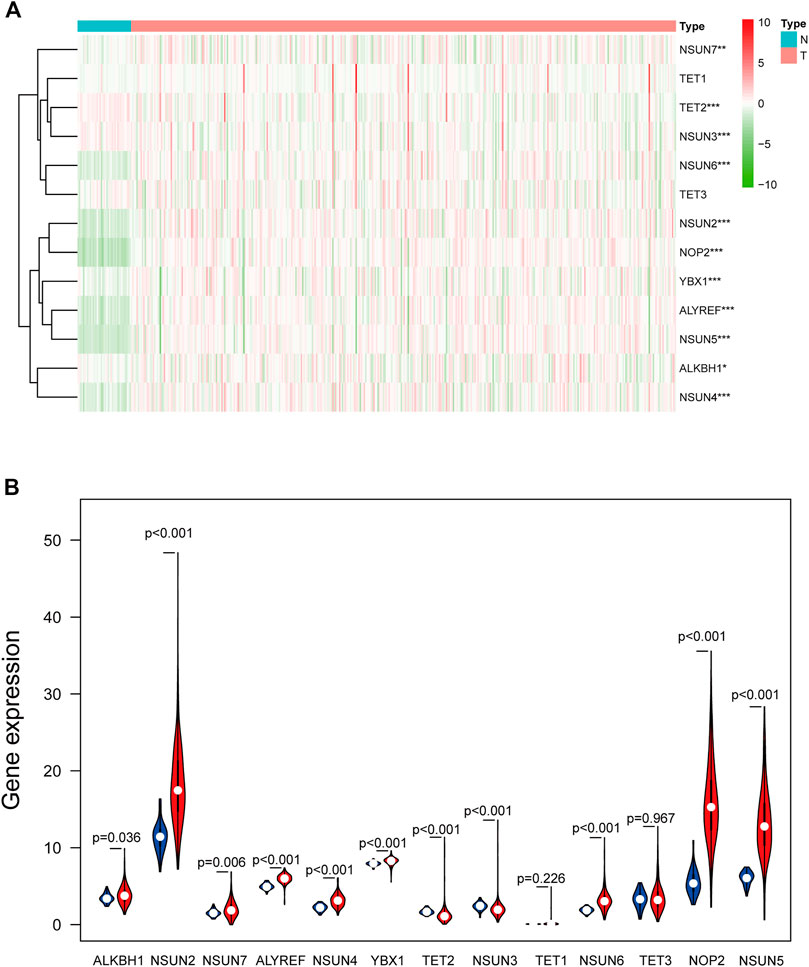
FIGURE 2. The expression of 13 m5C-related regulators in TCGA database between the tumor group and the normal group. (A) Heatmap of the expression of 13 m5C-related regulators. The depth of red represents the level of high expression, and the depth of green represents the level of low expression *p < 0.05, **p < 0.01, ***p < 0.001. (B) The violin diagram showed the median expression of 13 m5C-related regulators in COAD, and the position of white spots on the way represented the median value of the expression.
Correlation and Interaction of m5C-Related Regulators in COAD
The correlations between the m5C-related regulators were analyzed using the “corrplot” package in R and their interrelationships were retrieved from the STRING database (https://string-db.org/). The expression levels of the seven “writers” were correlated with each other, except for NSUN2 and NSUN7, NSUN5 and NSUN7, NSUN2 and NSUN3, and NSUN5 and NSUN6. There were also close and complicated relationships between each regulator in the protein-protein interaction (PPI) network. We also found that the expression of TET family genes (TET1, TET2, TET3) were highly related to each other and had little correlation with ALKBH1. However, the TET family was associated with ALKBH1 in the PPI network and had interrelationships with the “writer” genes via ALKBH1. In addition, there was evidence supporting the interaction between the “reader” genes ALYREF and YBX1 in the PPI network. The expression of these genes was also positively associated with each other (Figure 3).
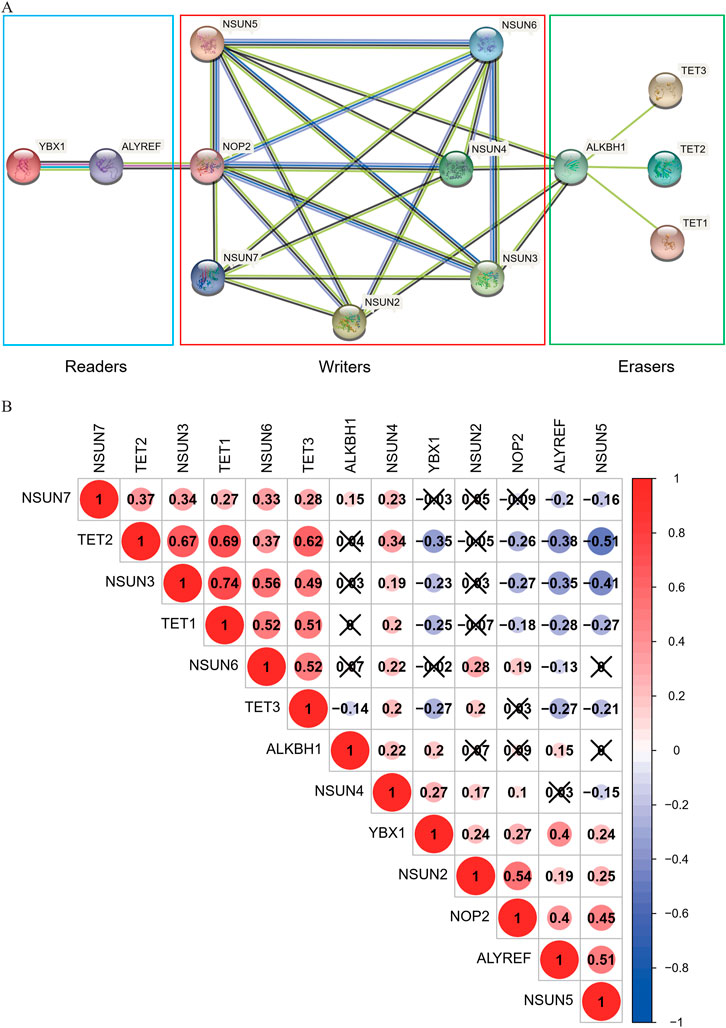
FIGURE 3. Correlation and interaction of m5C-related regulators in COAD. (A)The PPI network of the 13 m5C-related regulators was constructed using STRING. (B) Spearman correlation analysis of the 13 m5C-related regulators.
CNVs and SNPs of m5C-Related Regulators in COAD
Regarding CNVs, we found that 10 of the 13 m5C-related regulators were significantly different between the tumor tissue and the adjacent mucosa from 825 samples with CNV data. Furthermore, it was found that CNVs affect the expression of m5C-related regulators. The highest frequency of CNVs occurred in the “writer” gene NSUN5 (24.47%), followed by the “eraser” gene ALKBH1 (19.53%). The “eraser” gene TET3 had the lowest CNV frequency (2.35%) (Table 2). The “writer” genes NOP2, NSUN2, NSUN5, and NSUN7, the “eraser” genes TET2 and ALKBH, and the “reader” gene ALYREF displayed a significant difference in expression due to CNVs (Figure 4).
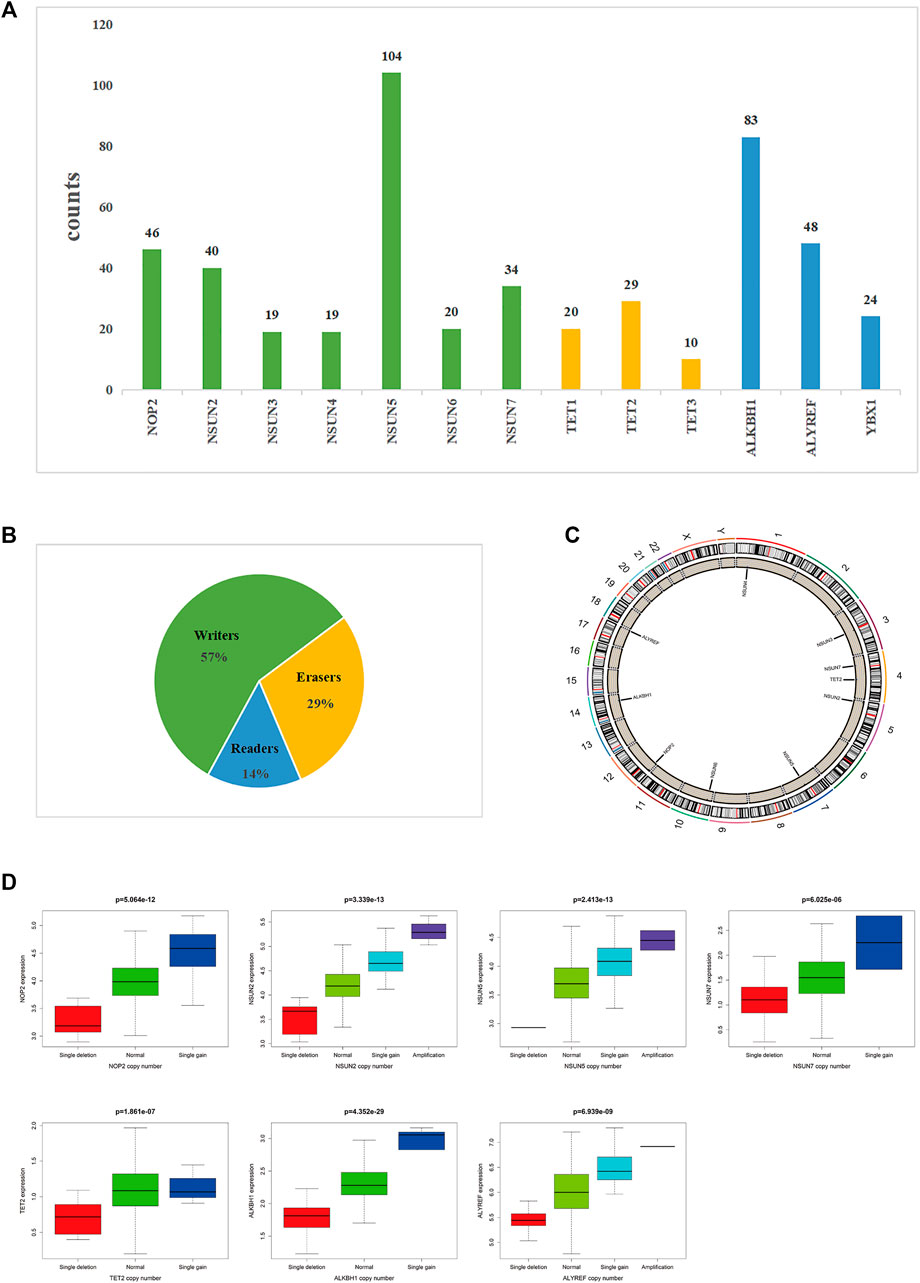
FIGURE 4. The landscape of CNV of m5C-related regulators in COAD.(A,B) Frequency of CNV of 13 m5C-related regulators in COAD. (B) Percentage of CNV of 13 m5C-related regulators in COAD. (C) Location of CNV alteration of 13 m5C-related regulators on chromosomes. (D) NOP2, NSUN2, NSUN5, NSUN7, TET2, ALKBH, and ALYREF displayed a significant difference in expression due to CNVs.
Regarding SNPs, we found that all of the m5C-related regulators had missense mutations, and missense mutations were the highest frequency mutation in 399 COAD cases with available sequencing data. Among them, the m5C “eraser” gene TET2 had the highest frequency of mutation events (96/399), followed by TET3 and TET1 (both 39/399). In addition, the “writer” genes NSUN2 and NSUN7, the “eraser” gene TET2, and the “reader” gene ALYREF displayed significant differences in expression levels due to SNPs. Next, we evaluated the effect of SNPs on patient prognosis, but no difference was observed due to the relatively few numbers of mutations (Figure 5).
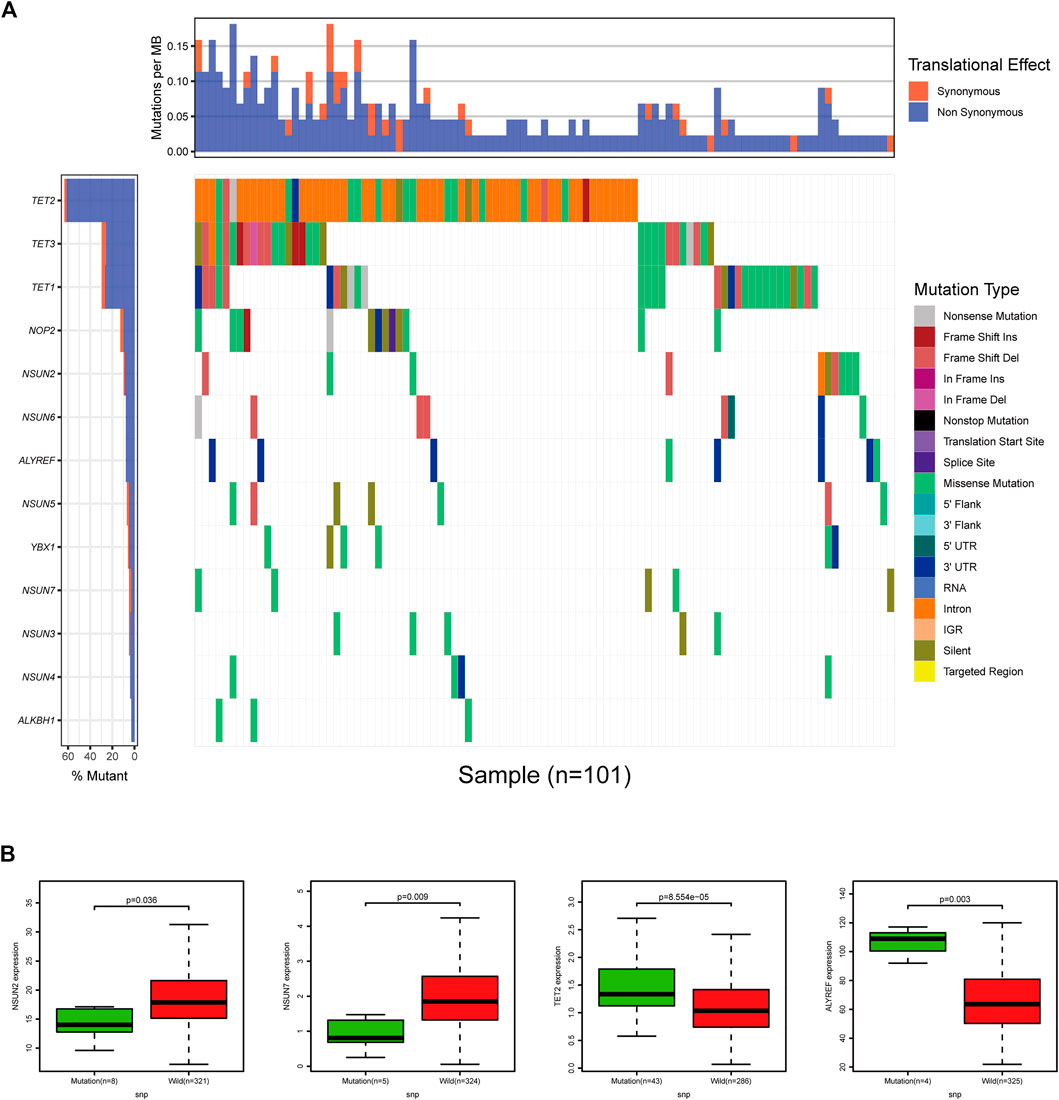
FIGURE 5. The landscape of SNP of m5C-related regulators in COAD. (A) Waterfall plot of SNP of 13 m5C-related regulators in COAD. (B) NSUN2, NSUN7, TET2, and ALYREF displayed significant differences in expression levels due to SNPs.
Consensus Clustering of Patients With COAD
Based on the expression levels of 13 m5C-related regulators, consistent clustering analysis of patients with COAD was performed, and they were clustered into two subgroups because there was minimal interference between the two subgroups (Figures 6A–D).
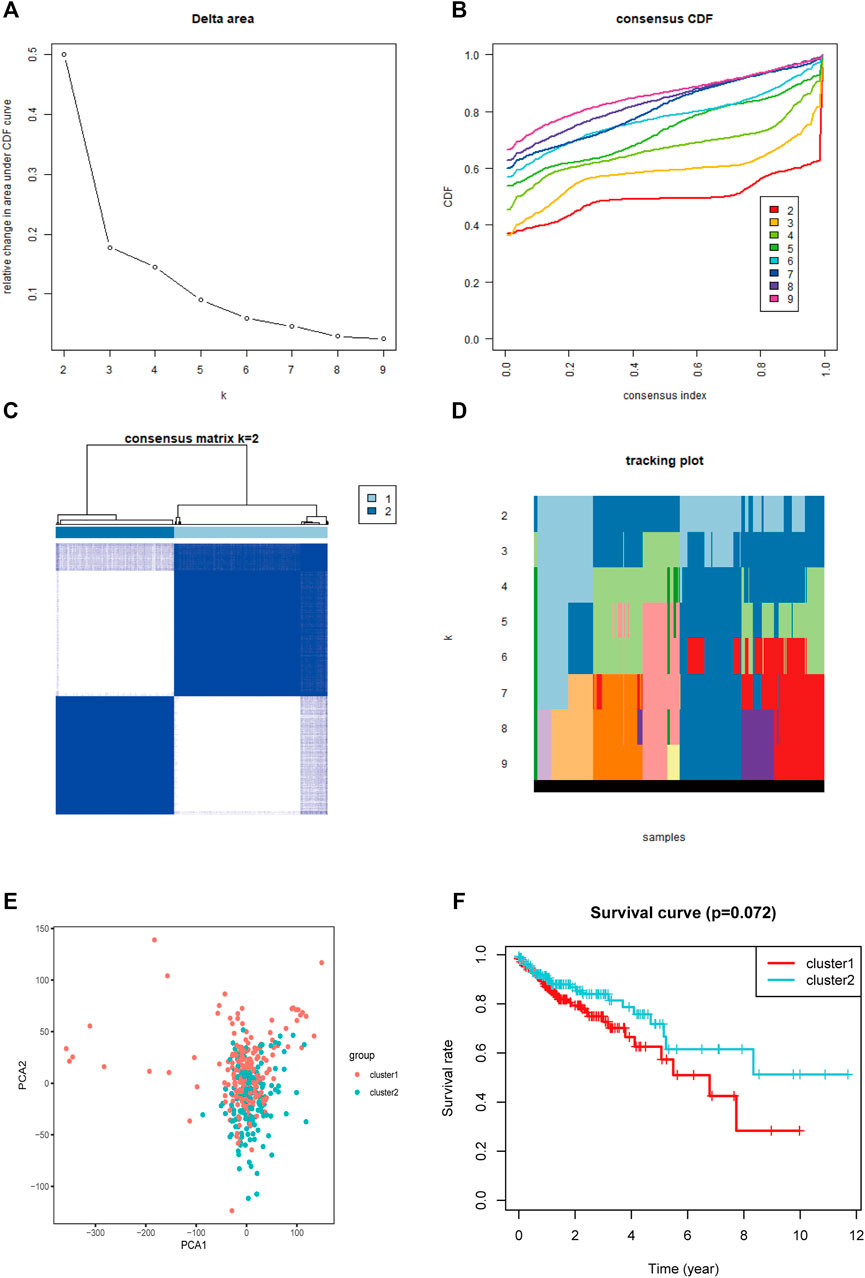
FIGURE 6. Consistent cluster analysis and principal component analysis of COAD. (A) The consistency clustering cumulative distribution function (CDF) when k is between 2 and 10. (B) The relative change of the area under the CDF curve from 2 to 10 of k. (C) At k = 2, the correlation between groups. (D) The distribution of the sample when k is between 2 and 10. (E) Principal component analysis of 2 clusters of total RNA expression profile after consistency analysis. (F) Comparison of Kaplan-Meier overall survival curves for COAD patients in cluster Ⅰ and Ⅱ.
PCA showed that the RNA expression levels in patients with COAD in clusters I and II were specific (Figure 6E). Nevertheless, there were many overlapping areas between each cluster on the whole, indicating that the clusters had something in common. The cluster II had a longer survival time than cluster I when analyzed using the Kaplan-Meier method, but they had no significant different (Figure 6F).
Prognostic Value of m5C-Related Regulators in COAD Prognosis
To evaluate the prognostic value of these 13 m5C-related regulators in COAD, univariate Cox regression analysis was used to identify m5C-related regulators that were highly correlated with the OS in patients with COAD, and two regulators with prognostic significance (p < 0.05) were found: NSUN6 and ALYREF. Specifically, ALYREF was considered a protective factor with HR < 1 in patients with COAD, and NSUN6 was considered as a risk factor with HR > 1 (Figure 7A). To further evaluate the prognostic significance of these two m5C-related regulators, LASSO Cox regression analysis was performed and it was revealed that NSUN6 (Coef = 0.300256795278519) and ALYREF (Coef = 0.00796895949684636) could serve as powerful prognostic factors in COAD (Figures 7B–C).
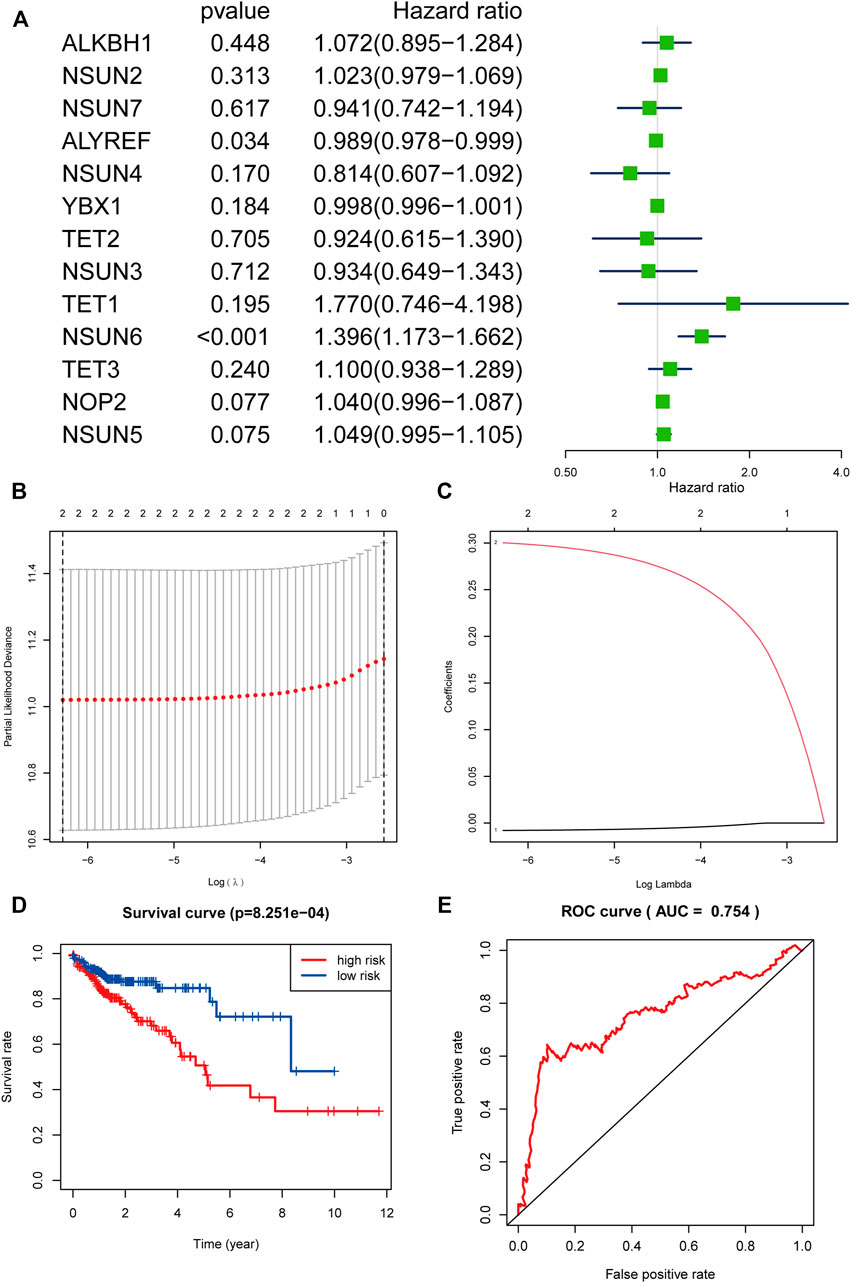
FIGURE 7. The process of constructing the signature based on NSUN6 and ALYREF and evaluating its prognostic value. (A) The Hazard ratio (HR), 95% confidence interval (CI) of 13 m5C-related regulators estimated by univariate Cox regression. (B) The point with the smallest cross verification error corresponds to the number of factors included in the Lasso regression model. (C) The lines of different colors represent the trajectory of the correlation coefficient of different factors in the model with the increase of Log Lamda. (D) Kaplan-Meier overall survival curves for patients in high-risk group- and low-risk group divided according to the risk score. (E) ROC analysis and AUC value of the ROC curve suggested the sensitivity and specificity for risk signature.
Based on NSUN6 and ALYREF, a risk signature was constructed and the risk score was calculated. Using the median risk score as the demarcation value, patients with COAD (n = 525) were classified into two groups, namely the high-risk and low-risk groups. To test the prognostic role of the two gene risk signatures, survival and ROC curve analyses were conducted. Based on the Kaplan-Meier (KM) survival analysis, the low-risk group had significantly longer survival time than the high-risk group (Figure 7D). In particular, compared with the 46.4% 5-year survival rate in the high-risk group, that of the low-risk group was 78.7%. The area under the curve (AUC) value in the time-dependent ROC curve was 0.754, suggesting good prediction performance of the survival model (Figure 7E).
Correlation Between the Two m5C-Related Regulators, Risk Score, and Clinicopathological Characteristics in COAD
We further analyzed the relationship between the two m5C-related regulators, risk score, and different clinical variables. KM survival analysis showed a close association of the two m5C-related regulators (NSUN6 and ALYREF) with the OS of patients with COAD (Figures 8A,B). In terms of TMN stage, the expression of ALYREF was differentially expressed between T3 stage and T4 stage and between M0 stage and M1 stage (Figure 8C). However, the expression of NSUN6 was not significantly different across groups in the TMN stage (Figure 8D). The expression of the two m5C-related regulators and the distribution of clinicopathological characteristics in the high-risk and low-risk groups are displayed as a heatmap (Figure 8E). Evident differences between the two groups according to stage T (p < 0.05) and fustat (p < 0.01) were observed.
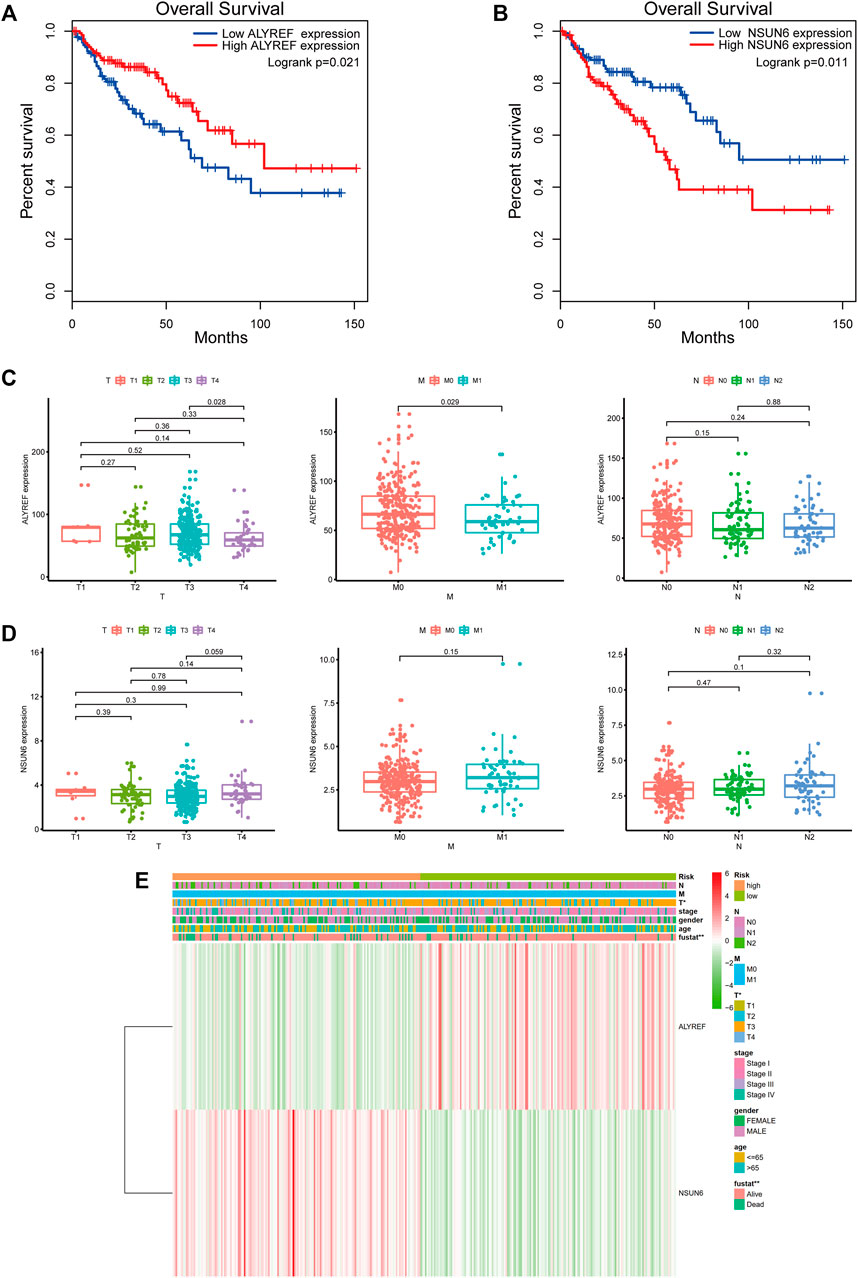
FIGURE 8. Survival analysis and clinicopathological characteristics of the two m5C-related regulators. (A) Kaplan-Meier survival curve of ALYREF in high- and low-expression groups. (B) Kaplan-Meier survival curve of NSUN6 in high- and low-expression groups. (C) Analysis of the relationship between the expression of ALYREF and TMN stage. (D) Analysis of the relationship between the expression of NSUN6 and TMN stage. (E) The heatmap shows the expression of NSUN6 and ALYRE in high-risk and low-risk. The distribution of clinicopathological characteristics was compared between the high-risk and low-risk groups. *p < 0.05, **p < 0.01.
To evaluate whether the risk score could serve as a prognostic indicator for OS in subgroups of patients with different clinical characteristics, we stratified subgroups by age (age ≤ 65 and age > 65), gender (female and male), clinical stage (stage I-II and stage III-IV), stage T (T1-2 and T3-4), stage M (M0 and M1) and stage N (N0 and N1-2). As the result shown in Figures 9A–D, the OS of the low-risk patients based on age (p < 0.001 in age ≤ 65), sex (p < 0.001 in male), and stage T (p < 0.005 in stage T1-2 and T3-4) was significantly higher than those of the high-risk patients.
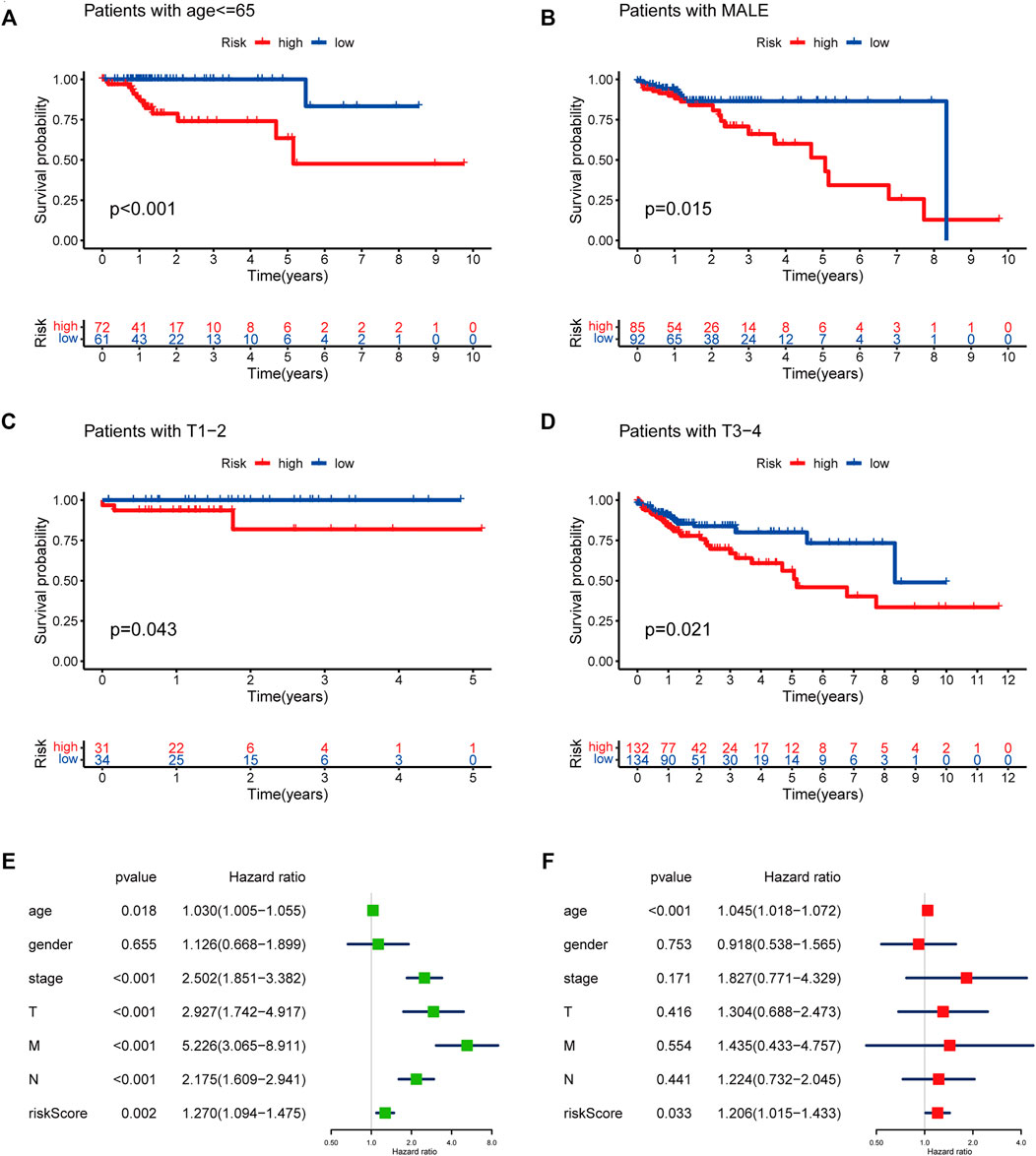
FIGURE 9. Subgroup analysis with risk score in different clinicopathological features and Prognostic risk model verification. (A) age ≤ 65. (B) male. (C) T1-T2. (D) T3-T4. (E) Univariate Cox regression analysis of risk score combined with clinicopathological factors. (F) Multivariate Cox regression analysis of risk score combined with clinicopathological factors.
To further examine whether the risk score was an independent prognostic factor, univariate and multivariate Cox regression analyses were conducted. This revealed that the risk score was significantly associated with OS in univariate analysis, in addition to age at diagnosis, pathological stage, and TNM stage (p < 0.05). However, only the age at diagnosis and risk score were correlated with OS (p < 0.05) in the multivariate Cox regression analysis (Figures 9E,F).
Biological Functional Analysis
As we clustered the patients with COAD into cluster Ⅰ and cluster Ⅱ, genes that were significantly upregulated (fold change >1 and p < 0.05) or downregulated (fold change <1 and p < 0.05) between the high-risk group and low-risk group were identified using the “edgeR” package in R. GO and KEGG pathway analysis were used for biological functional analysis.
Concerning GO analysis, the differentially expressed genes were associated with immune-related biological processes, such as “antigen binding” and “immunoglobulin receptor binding,” and pre-mRNA-related biological processes, such as “pre-mRNA 5′-splice site binding” and “pre-mRNA binding.” (Figure 10A). KEGG pathway analysis results were correlated with immune-related pathways, including “complement and coagulation cascades” and “NOD-like receptor signaling pathway,” and RNA-related pathways, including “RNA transport” and “spliceosome.” Moreover, cancer-related pathways were enriched, such as “transcriptional misregulation in cancer” and “MAPK signaling pathway” (Figure 10B).
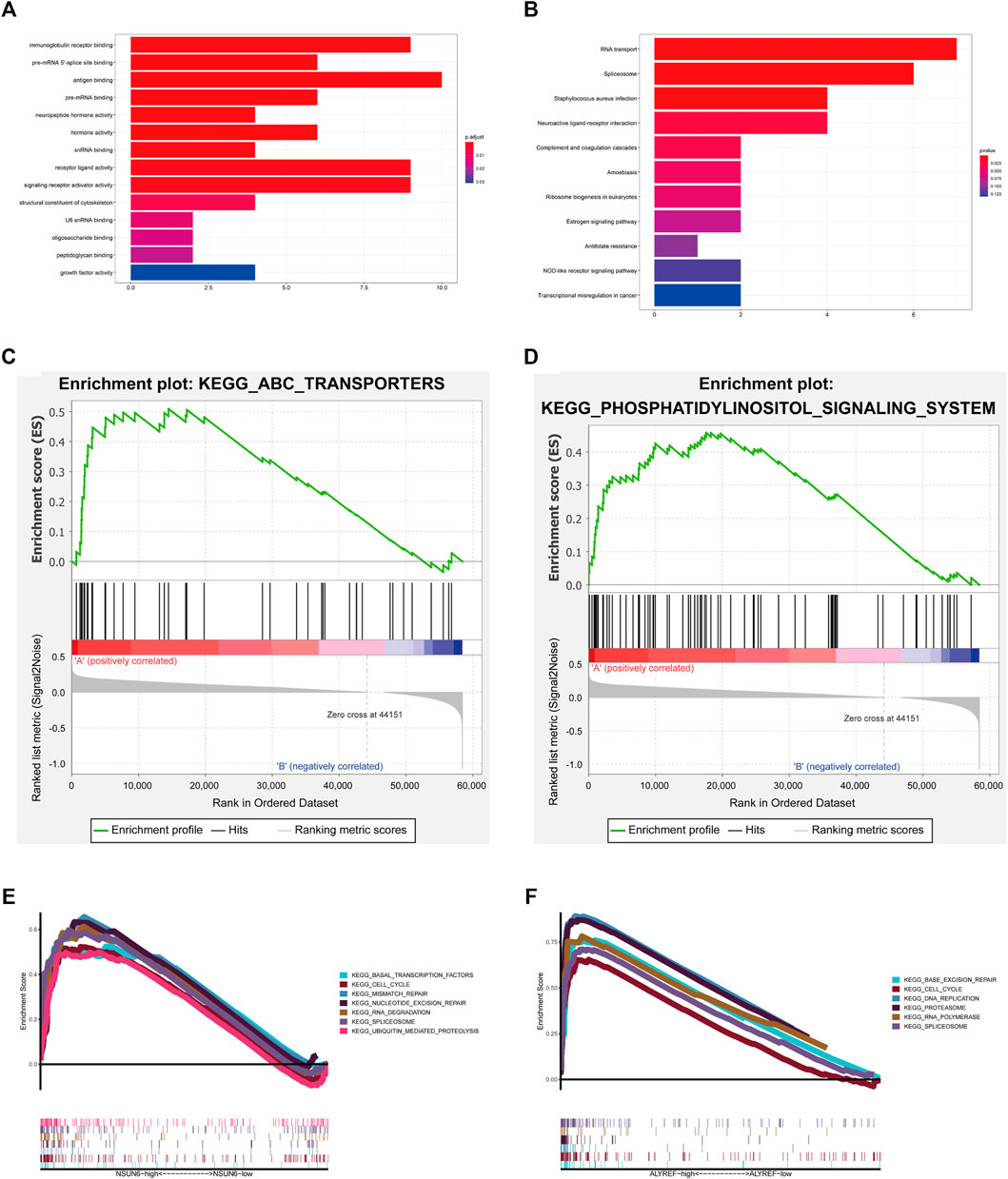
FIGURE 10. Biological functional analysis. (A,B) GO analysis and KEEG pathways analysis of the genes significantly upregulated or downregulated between cluster Ⅰ and cluster Ⅱ. (C,D) Cluster I had a worse overall survival and lower 5-year survival rate associated with malignancy-associated pathways, including the ATP-binding cassette transporter and phosphatidylinositol signaling system. (E) GSEA results for NSUN6 in COAD. (F) GSEA results for ALYREF in COAD.
Next, we used GSEA to predict the functional difference between clusters I and II. The results showed that cluster I had a worse OS and lower 5-year survival rate associated with malignancy-associated pathways, including the ATP-binding cassette transporter (NES = 1.79, normalized p = 0.006) and phosphatidylinositol signaling system (NES = 1.63, normalized p = 0.03) (Figures 10C,D).
Furthermore, as NSUN6 and ALYREF were shown to be important regulators of m5C in our study, GSEA was performed to investigate the potential biological processes associated with NSUN6 and ALYREF in COAD pathogenesis. GSEA suggested that increased expression of NSUN6 and ALYREF is involved in various biological functions in RNA processing, such as spliceosome, RNA polymerase, and RNA degradation. Upregulation of these genes was associated with malignancy-associated pathways, such as the cell cycle (Figures 10E,F).
Validation of the Expression Levels of the m5C-Related Regulators in Cell Lines and Clinical Samples
For validating the expression levels of the two m5C-related prognostic regulators from prognostic signature, we detected the expression levels in the COAD cell lines LS174T and normal colon mucosal epithelial cell line NCM460 by qRT-PCR. Our results showed that NSUN6 and ALYREF were significantly upregulated in LS174T compared with NCM460 (Figures 11A,B). IHC data from the HPA online database also demonstrated that the protein levels of NSUN6 and ALYREF were more highly expressed in cancer tissues than in normal tissues (Figure 11C).
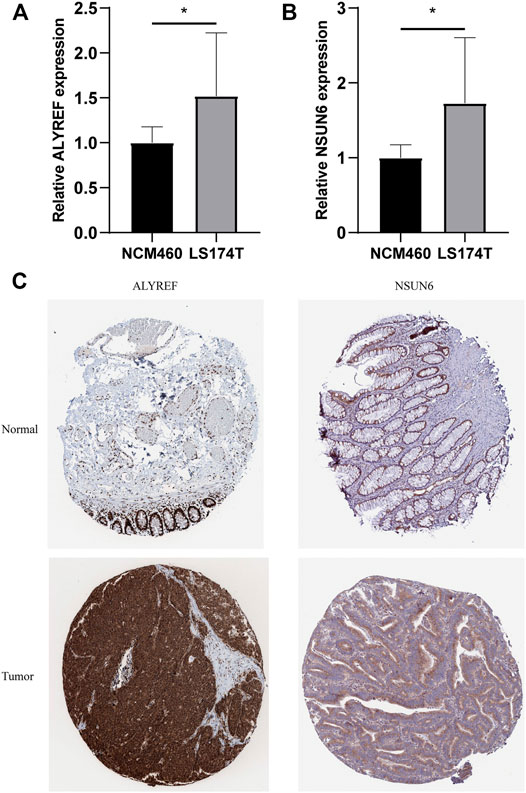
FIGURE 11. Validation of the Expression Levels of the m5C-Related Regulators in Cell Lines and Clinical Samples. (A,B) Expression of ALYREF and NSUN6 in COAD cell lines LS174T and normal colon mucosal epithelial cell line NCM460. *p < 0.01. (C) IHC analysis of the protein level expression of ALYREF and NSUN6 in COAD and normal tissues in HPA online database.
Discussion
RNA modifications have been increasingly demonstrated in tumorigenesis and tumor progression, suggesting that RNA epigenetic regulators may play an important role in COAD. Previous studies have shown that m6A RNA modification not only plays a critical role in the tumorigenesis and progression of CRC, but also has powerful significance in the diagnosis and prognosis of CRC patients (Li et al., 2021). Additionally, a growing body of evidence shows that m5C-related regulators could be latent predictive biomarkers in a variety of cancer (Huang et al., 2021a; Huang et al., 2021b; Pan et al., 2021). However, the literature on CRC and m5C has largely focused on DNA methylation (Zhu et al., 2018). Little is known about the relationship between m5C-related RNA modifications and CRC, which calls our attention to investigate the aberrant expression of m5C-related regulators in COAD and explore whether m5C-related regulators could serve as ideal biomarkers for COAD prognosis and participate in COAD initiation and progression.
In our study, we showed that the expressions of m5C-related regulators were significantly altered between tumor tissues and adjacent mucosa and had a strong correlation with the tumor progression and prognosis. This indicated that m5C-related regulators play a crucial role in COAD. First, the “writer” genes NSUN1-NSUN7, the “eraser” genes TET2 and ALKBH1, and the “reader” genes ALYREF and YBX1 were significantly upregulated or downregulated in tumor tissues, suggesting these genes may be critical in m5C-related occurrence and progression of COAD. To investigate the relationship between CNVs or SNPs of m5C-related regulators and their mRNA expression levels, COAD samples with CNV or SNP data from TCGA were analyzed. Regarding CNVs, the copy number of seven m5C-related regulators increased or was lost, and their mRNA expression was upregulated or downregulated accordingly and was significantly correlated. SNPs in TET2 and ALYREF were highly correlated with their high mRNA expression, while SNPs of NSUN2 and NSUN7 were significantly correlated with their low mRNA expression levels. Additionally, m5C-associated mutations in COAD could be studied in RMVar and RMdisease database, which were recently constructed and focused on genetic variants in RNA modifications (Kunqi Chenet al., 2021; Xin Luo et al., 2021).
Thereafter, based on the expression of the m5C-related regulators, patients with COAD were clustered into two subgroups (cluster Ⅰ and cluster Ⅱ), an the cluster II had a longer survival time than cluster I. To further study the effect of m5C-related regulators on the prognosis and clinicopathological characteristics of COAD, we constructed a prognostic risk signature using two identified m5C-related regulators (NSUN6 and ALYREF) and were able to assign patients with COAD into high- and low-risk groups. The correlation between the groups and clinicopathological characteristics was assessed, which revealed that the high-risk group was linked with stage T and fustat. Based on the risk value, the established ROC curve showed a satisfactory prediction performance. Moreover, the risk score can be used as an independent prognostic factor for COAD, suggesting that NSUN6 and ALYREF may be vital m5C-related regulators and significant prognostic factors for patients with COAD. Furthermore, this m5C-related regulators prognostic model could serve as a prognostic indicator for OS in subgroups of patients with different clinical characteristics, especially age ≤65, male, and stage T. The results presented above indicated that NSUN6 and ALYREF can be used as potential biomarkers, and a reliable risk model is critical for providing the necessary evidence for clinical adoption. Apart from our results, there was another study similarly demonstrated that a risk score developed from the three-m5C signature represented an independent prognostic factor for patients with COAD (Geng et al., 2021).
Recently, many studies have indicated that m5C RNA modification is involved in all types of human cancer. NSUN2 is the most studied m5C methyltransferase and participates in various cancers, such as bladder cancer, gallbladder carcinoma, and hepatocellular carcinoma (Chen et al., 2019; Gao et al., 2019; Sun et al., 2020). It was reported that NSUN2 is highly expressed in colon cancers (Okamoto et al., 2012), which was corroborated in our results. NSUN2 mainly exerts an oncogenic role by maintaining the stability of oncogenic RNA (Chellamuthu and Gray, 2020), but whether NSUN2 plays the same role in COAD requires further research. With respect to the two m5C-related regulators (NSUN6 and ALYREF) identified in our results, there have been some studies on cancer and related mechanisms. The role of NSUN6 in regulating cell proliferation and pancreatic cancer tumor growth was recently confirmed, and NSUN6 performs well in evaluating tumor recurrence and survival among pancreatic cancer patients (Yang et al., 2021). Next, ALYREF was found to be upregulated in hepatocellular carcinoma and oral squamous cell carcinoma, and it may have an effect on tumorigenesis via cell cycle regulation and mitosis (Saito et al., 2013; He et al., 2020).
To provide a comprehensive analysis, GO, KEEG pathway, and GSEA analyses of m5C-related regulators were also conducted. Several biological processes and pathways associated with the occurrence and progression of COAD were enriched, including “MAPK signaling pathway” and “cell cycle” (Koveitypour et al., 2019; Malki et al., 2020). Moreover, previous studies have reported that m5C-related RNA modifications are closely associated with mRNA translation, transport, and stability. Here, we found that the m5C-related regulators were associated with “pre-mRNA 5′-splice site binding” and “spliceosome,” suggesting they play important roles in RNA processing. In addition, it should be noted that a number of biological processes and pathways associated with immune response were identified. While extensive literature reports have demonstrated that N6-methyladenosine plays important role in immune evasion and immune response (Xiaoting Lou et al., 2021; Shulman and Stern-Ginossar, 2020) and bioinformatic analysis have shown that the m5C-related regulators were related to tumor immune microenvironment and affected the abundance of tumor-infiltrating immune cells in COAD (Geng et al., 2021), there have been few experimental reports about the relationship between m5C-related RNA modifications and immune response, suggesting that further research is required.
However, there are some limitations associated with our research. Firstly, the m5C-related regulators we selected included some DNA demethylase, such as TET1, TET2, TET3, and ALKBH1. The specific role of these genes in m5C RNA modification and DNA methylation of COAD and the crosstalk between m5C RNA modification and DNA methylation in COAD need to be further explored. Secondly, our research mainly focused on bioinformatic analysis, more experimental studies exploring the function of m5C on the different types of RNA and sites in COAD are in urgent need in future work. The m5C-Atlas database, a comprehensive database for decoding and annotating the m5C epitranscriptome, may be useful in the research (Ma et al., 2022). Thirdly, overall survival between cluster Ⅰ and cluster Ⅱ had no significant difference, more m5C-related regulators and more cohort need to be included in future analysis.
Conclusion
In this study, we first found that there was a significant correlation between the expression of m5C-related regulators and clinicopathological features and OS of patients with COAD. This revealed that a prognostic signature obtained using m5C-related regulators (NSUN6 and ALYREF) had significant value in COAD and could effectively predict the survival of patients with COAD. Additionally, biological processes and pathways associated with m5C-related RNA modifications were identified, which may facilitate the malignant development of COAD, thus improving our understanding of the role of m5C-related RNA modifications in the occurrence and progression of COAD. This work also provides important evidence towards the development of predictive biomarkers and molecular targeted therapy for COAD Bray et al., 2018.
Data Availability Statement
Publicly available datasets were analyzed in this study, which can be found in the Cancer Genome Atlas (TCGA) database and the Human Protein Atlas (HPA) online database.
Ethics Statement
This study met the publication guidelines stated by TCGA (https://cancergenome.nih.Gov/publications/publicationguidelines) HPA (https://www.proteinatlas.org/about/publications). All data used in the study were obtained from TCGA and HPA, and ethics approval and informed consent were not required.
Author Contributions
YH and YW conceived and designed the study. CH, XJ, and YY organized the database and performed statistical analyses. YH wrote the first draft of the manuscript. CH, XJ, and YY prepared the figures and tables and were involved in manuscript writing. KZ, PL, FL, and YW revised and proofread the manuscript. All authors contributed to manuscript revision and approved the submitted version.
Funding
This study was supported by The National Natural Science Foundation (No. 81904139, No. 81973819, No. 81904145 and No. 82104602), Collaborative Innovation Team Project of First-Rate Universities and Disciplines and High-level University Discipline of Guangzhou University of Chinese Medicine (No. 2021xk47), The Natural Science Foundation of Guangdong Province (No. 2019A1515011145), Guangdong Medical Science and Technology Research Fund (No. B2021089, No. A2020186), Guangzhou Science and Technology Project (No. 202102020535), Clinical Research Project of Innovation Hospital in the First Affiliated Hospital of Guangzhou University of Chinese Medicine (No. 2019ⅡT19) and Innovation Development project of the First Affiliated Hospital of Guangzhou University of Chinese Medicine (No. 2019QN01).
Conflict of Interest
The authors declare that the research was conducted in the absence of any commercial or financial relationships that could be construed as a potential conflict of interest.
Publisher’s Note
All claims expressed in this article are solely those of the authors and do not necessarily represent those of their affiliated organizations, or those of the publisher, the editors and the reviewers. Any product that may be evaluated in this article, or claim that may be made by its manufacturer, is not guaranteed or endorsed by the publisher.
Acknowledgments
This study benefited from the Cancer Genome Atlas (TCGA) databases. We appreciate the data platform and the authors up-loaded their data. We would like to thank Editage (www.editage.cn) for English language editing.
References
Angelova, M. T., Dimitrova, D. G., Dinges, N., Lence, T., Worpenberg, L., Carré, C., et al. (2018). The Emerging Field of Epitranscriptomics in Neurodevelopmental and Neuronal Disorders. Front. Bioeng. Biotechnol. 6, 46. doi:10.3389/fbioe.2018.00046
Asplund, A., Edqvist, P.-H. D., Schwenk, J. M., and Pontén, F. (2012). Antibodies for Profiling the Human Proteome-The Human Protein Atlas as a Resource for Cancer Research. Proteomics 12 (13), 2067–2077. doi:10.1002/pmic.201100504
Barbieri, I., and Kouzarides, T. (2020). Role of RNA Modifications in Cancer. Nat. Rev. Cancer 20 (6), 303–322. doi:10.1038/s41568-020-0253-2
Begik, O., Lucas, M. C., Liu, H., Ramirez, J. M., Mattick, J. S., and Novoa, E. M. (2020). Integrative Analyses of the RNA Modification Machinery Reveal Tissue- and Cancer-specific Signatures. Genome Biol. 21 (1), 97. doi:10.1186/s13059-020-02009-z
Bray, F., Ferlay, J., Soerjomataram, I., Siegel, R. L., Torre, L. A., and Jemal, A. (2018). Global Cancer Statistics 2018: GLOBOCAN Estimates of Incidence and Mortality Worldwide for 36 Cancers in 185 Countries. CA: A Cancer J. Clinicians 68 (6), 394–424. doi:10.3322/caac.21492
Chellamuthu, A., and Gray, S. G. (2020). The RNA Methyltransferase NSUN2 and its Potential Roles in Cancer. Cells 9 (8), 1758. doi:10.3390/cells9081758
Chen, X., Li, A., Sun, B.-F., Yang, Y., Han, Y.-N., Yuan, X., et al. (2019). 5-Methylcytosine Promotes Pathogenesis of Bladder Cancer through Stabilizing mRNAs. Nat. Cel Biol. 21 (8), 978–990. doi:10.1038/s41556-019-0361-y
Darwiche, N. (2020). Epigenetic Mechanisms and the Hallmarks of Cancer: An Intimate Affair. Am. J. Cancer Res. 10 (7), 1954–1978.
Dawson, M. A., and Kouzarides, T. (2012). Cancer Epigenetics: From Mechanism to Therapy. Cell 150 (1), 12–27. doi:10.1016/j.cell.2012.06.013
Gao, Y., Wang, Z., Zhu, Y., Zhu, Q., Yang, Y., Jin, Y., et al. (2019). NOP 2/Sun RNA Methyltransferase 2 Promotes Tumor Progression via its Interacting Partner RPL 6 in Gallbladder Carcinoma. Cancer Sci. 110 (11), 3510–3519. doi:10.1111/cas.14190
Geng, Q., Wei, Q., Shen, Z., Zheng, Y., Wang, L., Xue, W., et al. (2021). Comprehensive Analysis of the Prognostic Value and Immune Infiltrates of the Three-m5C Signature in colon Carcinoma. Cancer Manag. Res. 13, 7989–8002. doi:10.2147/CMAR.S331549
Grady, W. M., Yu, M., and Markowitz, S. D. (2021). Epigenetic Alterations in the Gastrointestinal Tract: Current and Emerging Use for Biomarkers of Cancer. Gastroenterology 160 (3), 690–709. doi:10.1053/j.gastro.2020.09.058
Hanley, J. A., and McNeil, B. J. (1982). The Meaning and Use of the Area under a Receiver Operating Characteristic (ROC) Curve. Radiology 143 (1), 29–36. doi:10.1148/radiology.143.1.7063747
He, Y., Yu, X., Li, J., Zhang, Q., Zheng, Q., and Guo, W. (2020). Role of m5C-Related Regulatory Genes in the Diagnosis and Prognosis of Hepatocellular Carcinoma. Am. J. Transl. Res. 12 (3), 912–922. doi:10.18632/aging.102669
Huang, Z., Li, J., Chen, J., and Chen, D. (2021a). Construction of Prognostic Risk Model of 5-Methylcytosine-Related Long Non-coding RNAs and Evaluation of the Characteristics of Tumor-Infiltrating Immune Cells in Breast Cancer. Front. Genet. 12, 748279. doi:10.3389/fgene.2021.748279
Huang, Z., Pan, J., Wang, H., Du, X., Xu, Y., Wang, Z., et al. (2021b). Prognostic Significance and Tumor Immune Microenvironment Heterogenicity of m5C RNA Methylation Regulators in Triple-Negative Breast Cancer. Front. Cel Dev. Biol. 9, 657547. doi:10.3389/fcell.2021.657547
Hussain, S. (2021). The Emerging Roles of Cytosine-5 Methylation in mRNAs. Trends Genet. 37, 498–500. doi:10.1016/j.tig.2021.02.001
Koveitypour, Z., Panahi, F., Vakilian, M., Peymani, M., Seyed Forootan, F., Nasr Esfahani, M. H., et al. (2019). Signaling Pathways Involved in Colorectal Cancer Progression. Cell Biosci. 9, 97. doi:10.1186/s13578-019-0361-4
Kunqi Chen, K., Song, B., Tang, Y., Wei, Z., Xu, Q., Su, J., et al. (2021). RMDisease: A Database of Genetic Variants that Affect RNA Modifications, with Implications for Epitranscriptome Pathogenesis. Nucleic Acids Res. 49 (D1), D1396–D1404. doi:10.1093/nar/gkaa790
Li, J., Liang, L., Yang, Y., Li, X., and Ma, Y. (2021). N6-methyladenosine as a Biological and Clinical Determinant in Colorectal Cancer: Progression and Future Direction. Theranostics 11 (6), 2581–2593. doi:10.7150/thno.52366
Ma, J., Song, B., Wei, Z., Huang, D., Zhang, Y., Su, J., et al. (2022). M5C-Atlas: A Comprehensive Database for Decoding and Annotating the 5-methylcytosine (m5C) Epitranscriptome. Nucleic Acids Res. 50 (D1), D196–D203. doi:10.1093/nar/gkab1075
Malki, A., ElRuz, R. A., Gupta, I., Allouch, A., Vranic, S., and Al Moustafa, A.-E. (2020). Molecular Mechanisms of colon Cancer Progression and Metastasis: Recent Insights and Advancements. Int. J. Mol. Sci. 22 (1), 130. doi:10.3390/ijms22010130
Miller, K. D., Nogueira, L., Mariotto, A. B., Rowland, J. H., Yabroff, K. R., Alfano, C. M., et al. (2019). Cancer Treatment and Survivorship Statistics, 2019. CA A. Cancer J. Clin. 69 (5), 363–385. doi:10.3322/caac.21565
Minervini, C. F., Parciante, E., Impera, L., Anelli, L., Zagaria, A., Specchia, G., et al. (2020). Epitranscriptomics in normal and Malignant Hematopoiesis. Int. J. Mol. Sci. 21 (18), 6578. doi:10.3390/ijms21186578
Mortazavi, A., Williams, B. A., McCue, K., Schaeffer, L., and Wold, B. (2008). Mapping and Quantifying Mammalian Transcriptomes by RNA-Seq. Nat. Methods 5 (7), 621–628. doi:10.1038/nmeth.1226
Nombela, P., Miguel-López, B., and Blanco, S. (2021). The Role of m6A, m5C and Ψ RNA Modifications in Cancer: Novel Therapeutic Opportunities. Mol. Cancer 20 (1), 18. doi:10.1186/s12943-020-01263-w
Okamoto, M., Hirata, S., Sato, S., Koga, S., Fujii, M., Qi, G., et al. (2012). Frequent Increased Gene Copy Number and High Protein Expression of tRNA (Cytosine-5-)-methyltransferase (NSUN2) in Human Cancers. DNA Cel Biol. 31 (5), 660–671. doi:10.1089/dna.2011.1446
Pan, J., Huang, Z., and Xu, Y. (2021). M5C RNA Methylation Regulators Predict Prognosis and Regulate the Immune Microenvironment in Lung Squamous Cell Carcinoma. Front. Oncol. 11, 657466. doi:10.3389/fonc.2021.657466
Porcellini, E., Laprovitera, N., Riefolo, M., Ravaioli, M., Garajova, I., and Ferracin, M. (2018). Epigenetic and Epitranscriptomic Changes in Colorectal Cancer: Diagnostic, Prognostic, and Treatment Implications. Cancer Lett. 419, 84–95. doi:10.1016/j.canlet.2018.01.049
Ritchie, M. E., Phipson, B., Wu, D., Hu, Y., Law, C. W., Shi, W., et al. (2015). Limma powers Differential Expression Analyses for RNA-Sequencing and Microarray Studies. Nucleic Acids Res. 43 (7), e47. doi:10.1093/nar/gkv007
Roundtree, I. A., Evans, M. E., Pan, T., and He, C. (2017). Dynamic RNA Modifications in Gene Expression Regulation. Cell 169 (7), 1187–1200. doi:10.1016/j.cell.2017.05.045
Saito, Y., Kasamatsu, A., Yamamoto, A., Shimizu, T., Yokoe, H., Sakamoto, Y., et al. (2013). ALY as a Potential Contributor to Metastasis in Human Oral Squamous Cell Carcinoma. J. Cancer Res. Clin. Oncol. 139 (4), 585–594. doi:10.1007/s00432-012-1361-5
Schaefer, M. R. (2021). The Regulation of RNA Modification Systems: The Next Frontier in Epitranscriptomics. Genes 12 (3), 345. doi:10.3390/genes12030345
Shi, H., Chai, P., Jia, R., and Fan, X. (2020). Novel Insight into the Regulatory Roles of Diverse RNA Modifications: Re-defining the Bridge between Transcription and Translation. Mol. Cancer 19 (1), 78. doi:10.1186/s12943-020-01194-6
Shulman, Z., and Stern-Ginossar, N. (2020). The RNA Modification N6-Methyladenosine as a Novel Regulator of the Immune System. Nat. Immunol. 21 (5), 501–512. doi:10.1038/s41590-020-0650-4
Simon, N., Friedman, J., Hastie, T., and Tibshirani, R. (2011). Regularization Paths for Cox's Proportional Hazards Model via Coordinate Descent. J. Stat. Soft. 39 (5), 1–13. doi:10.18637/jss.v039.i05
Song, H., Liu, D., Dong, S., Zeng, L., Wu, Z., Zhao, P., et al. (2020). Epitranscriptomics and Epiproteomics in Cancer Drug Resistance: Therapeutic Implications. Sig Transduct Target. Ther. 5 (1), 193. doi:10.1038/s41392-020-00300-w
Sun, Z., Xue, S., Zhang, M., Xu, H., Hu, X., Chen, S., et al. (2020). Aberrant NSUN2-Mediated m5C Modification of H19 lncRNA Is Associated with Poor Differentiation of Hepatocellular Carcinoma. Oncogene 39 (45), 6906–6919. doi:10.1038/s41388-020-01475-w
White, A., Joseph, D., Rim, S. H., Johnson, C. J., Coleman, M. P., and Allemani, C. (2017). Colon Cancer Survival in the United States by Race and Stage (2001-2009): Findings from the CONCORD-2 Study. Cancer 123 (Suppl. 24), 5014–5036. doi:10.1002/cncr.31076
Wilkerson, M. D., and Hayes, D. N. (2010). ConsensusClusterPlus: A Class Discovery Tool with Confidence Assessments and Item Tracking. Bioinformatics 26 (12), 1572–1573. doi:10.1093/bioinformatics/btq170
Wu, C.-t., and Morris, J. R. (2001). Genes, Genetics, and Epigenetics: A Correspondence. Science 293 (5532), 1103–1105. doi:10.1126/science.293.5532.1103
Xiaoting Luo, X., Li, H., Liang, J., Zhao, Q., Xie, Y., Ren, J., et al. (2021). RMVar: An Updated Database of Functional Variants Involved in RNA Modifications. Nucleic Acids Res. 49 (D1), D1405–D1412. doi:10.1093/nar/gkaa811
Xie, S., Chen, W., Chen, K., Chang, Y., Yang, F., Lin, A., et al. (2020). Emerging Roles of RNA Methylation in Gastrointestinal Cancers. Cancer Cel Int 20 (1), 585. doi:10.1186/s12935-020-01679-w
Xin Lou, X., Wang, J.-J., Wei, Y.-Q., and Sun, J.-J. (2021). Emerging Role of RNA Modification N6-Methyladenosine in Immune Evasion. Cell Death Dis. 12 (4), 300. doi:10.1038/s41419-021-03585-z
Xue, C., Zhao, Y., and Li, L. (2020). Advances in RNA Cytosine-5 Methylation: Detection, Regulatory Mechanisms, Biological Functions and Links to Cancer. Biomark Res. 8, 43. doi:10.1186/s40364-020-00225-0
Yang, R., Liang, X., Wang, H., Guo, M., Shen, H., Shi, Y., et al. (2021). The RNA Methyltransferase NSUN6 Suppresses Pancreatic Cancer Development by Regulating Cell Proliferation. EBioMedicine 63, 103195. doi:10.1016/j.ebiom.2020.103195
Yu-Sheng Chen, Y. S., Yang, W. L., Zhao, Y. L., and Yang, Y. G. (2021). Dynamic Transcriptomic M(5) C and its Regulatory Role in RNA Processing. Wiley Interdiscip. Rev. RNA 12, e1639. doi:10.1002/wrna.1639
Zhao, Z., Meng, J., Su, R., Zhang, J., Chen, J., Ma, X., et al. (2020). Epitranscriptomics in Liver Disease: Basic Concepts and Therapeutic Potential. J. Hepatol. 73 (3), 664–679. doi:10.1016/j.jhep.2020.04.009
Keywords: colon adenocarcinoma, epigenetics, RNA modification, 5-methylcytosine, gene expression profile, prognostic signature, TCGA
Citation: Huang Y, Huang C, Jiang X, Yan Y, Zhuang K, Liu F, Li P and Wen Y (2022) Exploration of Potential Roles of m5C-Related Regulators in Colon Adenocarcinoma Prognosis. Front. Genet. 13:816173. doi: 10.3389/fgene.2022.816173
Received: 16 November 2021; Accepted: 26 January 2022;
Published: 24 February 2022.
Edited by:
Ritu Kulshreshtha, Indian Institute of Technology Delhi, IndiaReviewed by:
Hailin Wang, Research Center for Eco-environmental Sciences (CAS), ChinaKunqi Chen, Fujian Medical University, China
Copyright © 2022 Huang, Huang, Jiang, Yan, Zhuang, Liu, Li and Wen. This is an open-access article distributed under the terms of the Creative Commons Attribution License (CC BY). The use, distribution or reproduction in other forums is permitted, provided the original author(s) and the copyright owner(s) are credited and that the original publication in this journal is cited, in accordance with accepted academic practice. No use, distribution or reproduction is permitted which does not comply with these terms.
*Correspondence: Fengbin Liu, bGl1ZmIxNjNAMTYzLmNvbQ==; Peiwu Li, ZG9jdG9ybGlwd0BnenVjbS5lZHUuY24=; Yi Wen, MjAxNzIxMDEwMzFAc3R1Lmd6dWNtLmVkdS5jbg==