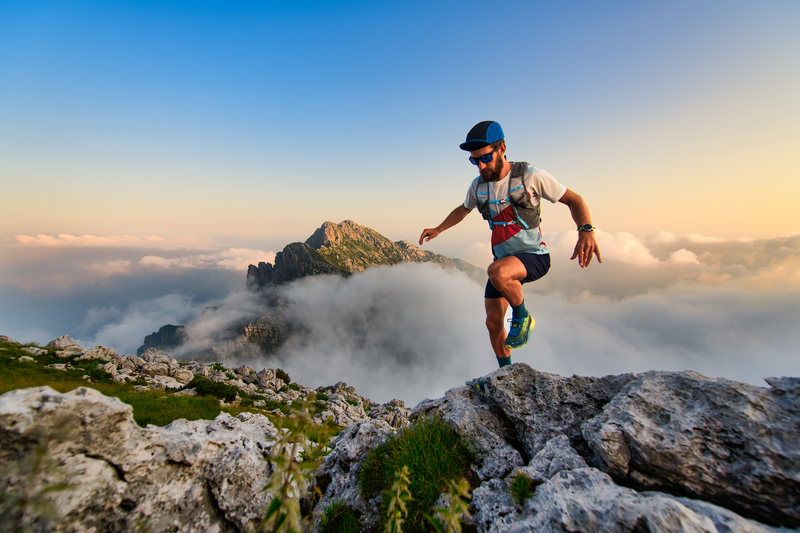
95% of researchers rate our articles as excellent or good
Learn more about the work of our research integrity team to safeguard the quality of each article we publish.
Find out more
ORIGINAL RESEARCH article
Front. Genet. , 11 April 2022
Sec. Livestock Genomics
Volume 13 - 2022 | https://doi.org/10.3389/fgene.2022.809741
This article is part of the Research Topic Omics Technologies in Livestock Improvement: From Selection to Breeding Decisions View all 30 articles
Water buffalo (Bubalus bubalis), belonging to the Bovidae family, is an economically important animal as it is the major source of milk, meat, and drought in numerous countries. It is mainly distributed in tropical and subtropical regions with a global population of approximately 202 million. The advent of low cost and rapid sequencing technologies has opened a new vista for global buffalo researchers. In this study, we utilized the genomic data of five commercially important buffalo breeds, distributed globally, namely, Mediterranean, Egyptian, Bangladesh, Jaffrarabadi, and Murrah. Since there is no whole-genome sequence analysis of these five distinct buffalo breeds, which represent a highly diverse ecosystem, we made an attempt for the same. We report the first comprehensive, holistic, and user-friendly web genomic resource of buffalo (BuffGR) accessible at http://backlin.cabgrid.res.in/buffgr/, that catalogues 6028881 SNPs and 613403 InDels extracted from a set of 31 buffalo tissues. We found a total of 7727122 SNPs and 634124 InDels distributed in four breeds of buffalo (Murrah, Bangladesh, Jaffarabadi, and Egyptian) with reference to the Mediterranean breed. It also houses 4504691 SSR markers from all the breeds along with 1458 unique circRNAs, 37712 lncRNAs, and 938 miRNAs. This comprehensive web resource can be widely used by buffalo researchers across the globe for use of markers in marker trait association, genetic diversity among the different breeds of buffalo, use of ncRNAs as regulatory molecules, post-transcriptional regulations, and role in various diseases/stresses. These SNPs and InDelscan also be used as biomarkers to address adulteration and traceability. This resource can also be useful in buffalo improvement programs and disease/breed management.
Water buffalo, scientifically known as Bubalus bubalis, is the major source of milk, meat, and drought in various countries, making it an economically important animal. This livestock species belonging to the Bovidae family is mainly distributed in tropical and subtropical regions. Based on morphology and behavior, the two categories of domestic Asian water buffalo are river buffalo (2n = 50) and swamp buffalo (2n = 48) (Iannuzzi, 1994). The global population of water buffalo is ∼217 million in 34 countries (FAOSTAT, 2020) with ∼82% and ∼18% river buffalo and swamp buffalo, respectively. South Asia holds the majority of water buffalo as India ranks first in buffalo breeding with a share of 50.5%, followed by Pakistan and China (Taşcioğlu et al., 2020). Buffalo are largely domesticated by small farmers in Asia. This indicates the popularity and dependence of water buffalo as compared to any other species that are domesticated. India has the lion’s share (69%) in river buffalo. Milk yield is more in buffalo than cattle. Also, buffalo milk has a higher nutritional value than cattle on account of higher fat content (8.0%), higher unsaturated fatty acid levels, higher protein content (4.5%), and lower phospholipid and cholesterol levels. It is a more preferred milk for dairy products (Du et al., 2019).
The fast decline in DNA sequencing costs has paved the way for researchers across the globe to revolutionize genome analysis. Assembly and decoding of the genome of water buffalo is in continuous progress. The following five genome assemblies of water buffalo exist on the NCBI database (https://www.ncbi.nlm.nih.gov/assembly/?term=Bubalus) (Last accessed: July 2021): GCA_003121395.1 of the Mediterranean breed from University of Adelaide, Australia; GCA_019923935.1 of the Murrah breed from National Dairy Development Board, India; GCA_004794615.1 of the Bangladesh breed from BGI-Shenzhen, China; GCA_002993835.1 of the Egyptian buffalo breed from Agriculture Genetic Engineering Research Institute and Nile University, Egypt, and GCA_000180995.3 of the Jafarabadi breed from Anand Agricultural University, India. GCA_003121395.1 and GCA_019923935.1 are chromosome level assemblies with 25 chromosomes, however, RefSeq annotation has not yet been provided for GCA_019923935.1.
Whole-genome sequencing and transcriptome studies provide insights on genetic makeup, numerous trait markers, and their expression in organisms. Simple sequence repeats (SSR) are the information source for genetic diversity among different breeds/varieties of the same species (Patzak et al., 2012). There are 22 buffalo breeds (only river subspecies) distributed all over the world with different characteristics like shape, size, color, weight, and lactation period, etc. Genomic variation results in single nucleotide polymorphisms (SNPs), insertions, and deletions (Surya et al., 2018). These variations are stable and are transferred from one generation to the next. These variations impinge start codon gain or loss, stop codon gain or loss, or frame shift. The presence of such variations in protein coding regions culminates in synonymous or non-synonymous amino acid replacement.
Long non-coding RNAs are a group of RNAs which are greater than 200 nt and lack open reading frames or have <100 amino acids in length. lncRNAs regulate gene expression through methylation and demethylation (Bhat and Jones 2016; Fernandes et al., 2019) and through chromatin modifications by interfering with transcription factors [binding with DNA and regulating transcription (Griffiths et al., 2000)] and miRNAs. lncRNAs perform post-translational regulation through capping, alternative splicing, editing, transport, translation, degradation, and stability of mRNA targets. Apart from their biological roles, lncRNAs can also function as biomarkers. At the organism level, lncRNAs are known to be abnormally expressed in many diseases therefore playing a role in diagnosis (Kosinska-Selbi et al., 2020).
miRNAs are 18–25 nucleotide-long regulatory sequences, which play an important role in response reactions during anorganism’s exposure to biotic or abiotic conditions (O’Brien et al., 2018). They regulate gene expression by binding to the target sequence with the help of AGO protein and make an miRNA-induced silencing complex (mi-RISC) (Kawamata and Tomari, 2010). Water buffalo are adapted to higher to lower altitudes, hence they face a wide range of stresses like low/high temperatures (Liu et al., 2019), pathogens (Dhanoa et al., 2019; Lecchi et al., 2019), etc. Previously known miRNAs specific to buffalo have been reported from various transcriptome studies involving such stress conditions (Dhanoa et al., 2019; Lecchi et al., 2019; Liu et al., 2019).
Other regulatory non-coding RNAs, known as circular RNAs (circRNAs), spawn through back-splicing of RNAs. They are more stable than RNAs (Chen et al., 2017; Wang et al., 2017). The functions of circRNAs are not well known but still it is reported that they play a significant role in post-transcriptional regulation of gene expression (Lukiw, 2013). CircRNAs function as a sponge of miRNAs by sequestering them by binding and interacting with lncRNAs (Lei et al., 2021). These are being employed as biomarkers for controlling and treating diseases (Meng et al., 2017; Lu, 2020).
Before release of the buffalo reference genome, most of the studies related to buffalo involving omics analyses were based on the Bos taurus reference genome. The available whole-genome assemblies of five buffalo breeds represent a highly diverse ecosystem. Their utilization in whole-genome sequence analyses and in extraction of rapid polymorphic markers at lower costs for the breeders is warranted. In 2018, a buffalo reference genome with 24 chromosomes along with X and MT chromosomes was released by the Italian Buffalo Genome Consortium (https://www.ncbi.nlm.nih.gov/assembly/GCA_003121395.1). For the current study, the different omics studies in buffalo were performed using the GCA_003121395.1 buffalo reference genome to extract non-coding RNAs such as miRNAs, lncRNAs, and circRNAs in the 31 buffalo tissues, which had not been attempted earlier. Also, the various genetic markers such as SSRs, SNPs, and InDels from five breeds of buffalo (Mediterranean, Egyptian, Bangladesh, Jaffrarabadi and Murrah) were mined. After extraction of the mentioned molecular markers and non-coding RNAs, a web-based genomic resource, BuffGR was developed to facilitate the buffalo research community with user-friendly, single-window retrieval of buffalo omics data to be utilized for further scientific research and studies. This buffalo web resource is state-of-the-art, holistic, and currently the largest collection related to buffalo including the most important breed of India, i.e., Murrah from the latest 2021 assembly as well as the world, i.e., Mediterranean from the latest 2018 assembly.
In order to extract the breed-wise molecular markers and variants like SSRs, SNPs, and InDels, in five buffalo breeds, namely, Mediterranean, Egyptian, Bangladesh, Jaffrabadi, and Murrah, their genome assemblies were retrieved from NCBI (Table 1).
For extraction of cirRNAs, SNPs, and InDels, RNA-seq data of a total of 31 buffalo tissues were retrieved from NCBI, which were mapped with the GCF_003121435.1 genome assembly of the Mediterranean breed using Bowtie2 (Langmead and Salzberg, 2012), while HISAT2 (Kim et al., 2019) was used in the case of lncRNAs (Table 2). For the extraction of miRNAs, cirRNAs, and lncRNAs, the genome assembly of the Mediterranean breed was used (GCF_003121435.1).
TABLE 2. The details of RNA-seq data from the International Water Buffalo Genome Project representing different buffalo tissues along with SRA IDs and mapping %.
For extraction of variants, namely, SNPs and InDels, the four buffalo breeds (Murrah, Jaffrabadi, Bangladesh, and Egyptian) were mapped to the water buffalo reference genome of the Mediterranean breed (GCA_003121395.1, the UOA_WB_1 assembly). These mapped reads of RNA-seq data were first sorted and indexed using Samtools (Li et al., 2009; Li, 2011) along with the indexed reference genome (GCA_003121395.1). Then, coverage extraction of each nucleotide was performed using Samtools mpileup. Further, SNPs and InDels were extracted using bcftools (Danecek et al., 2021) call. Finally, significant SNPs were filtered using bcftools view at p-value <0.05, read depth >10, quality depth >30, minimum root mean square mapping >40, and flanking sequence length =50. This was followed by functional annotation of extracted SNPs and InDels using Perl script utilizing the annotation file of the genome of Mediterranean buffalo (GCA_003121395.1).
MIcroSAtellite (MISA) (Beier et al., 2017) was used to extract SSRs from genome assemblies of all the five breeds utilizing parameters such as ≥10, ≥6, ≥5, ≥4, and ≥4 repeats for mono, di, tri, tetra, and penta nucleotide (nt) motifs, respectively along with length of compound SSRs ≤100 nt and minimum distance between two SSRs ≥50 nt (Zhao et al., 2017). The functional annotation of mined SSR markers was performed using Perl scripts utilizing the annotation of the Mediterranean buffalo RefSeq genome (GCA_003121395.1). Finally, based on the result of MISA, primer3 software (Untergasser et al., 2012) was used to design the primer pairs at default parameters, taking the flanking sequences of SSRs of the Mediterranean breed.
For the prediction of miRNAs, first-known miRNAs and pre-miRNAs of Bos tauras from miRBase (Griffiths-Jones et al., 2006) were collected and duplicates were removed using CD-HIT (Huang et al., 2010). The pre-miRNA sequences of non-redundant Bos tauras miRNAs were aligned with the buffalo RefSeq genome (GCA_003121395.1) using BLASTn and sequences with 0 gap and ≤3 mismatches were taken along with 500 nt up and downstream stretches, making these >1000 nt length sequences (Altschul et al., 1990). Further, 200 nt fragments were taken from these sequences by using 25 nt sliding windows using the SegKit tool (Shen et al., 2016). The obtained sequences were again clustered using CD-HIT to obtain non-redundant sequences. Non-redundant sequences were used to predict the secondary structure by RNAfold (Lorenz et al., 2011) at minimum free energy (MFE) > −20. Further, sequences with <60 nt, non-AUGC, and multi-loop in structure, and pseudo pre-miRNAs were removed by Triplet-SVM classifier (Xue et al., 2005). These putative pre-miRNAs were used for further prediction of mature miRNAs using MiRdup (Leclercq et al., 2013). Finally, psRANTarget (Dai and Zhao, 2011) was used at an expectation value of 2 to predict mRNA targets of predicted miRNAs.
For the identification of circRNAs from the mapped RNA-seq reads of 31 buffalo tissues, CIRI v2.0.4 (Gao et al., 2015) was used. As circRNA-looping sites cannot be aligned directly to the genome, find_circ (Memczak et al., 2013) was used for the first 20 base pairs of each read end that were incompatible with the genome to anchor independent reads, thus map them with the buffalo reference genome (GCA_003121395.1), and finally to find only the mapped site. If the two anchors aligned in the linear region were in the reverse direction, anchor reads were extended until circRNA junctions were found. The sequence was considered a circRNA if the two sides of sequences corresponded to GT/AG splicing signals as mentioned by Fu et al. (2018). CIRI was also used to annotate circRNAs by using the annotation file of the GCF_003121395.1 genome assembly.
For the identification of lncRNAs, from RNA-seq data of 31 buffalo tissues, first, mapping was performed using HISAT2 (Kim et al., 2019), followed by assembly using Stringtie v1.3.5 (Pertea et al., 2015). Then, putative lncRNAs were predicted from assembled reads using CPC2 (Kang et al., 2017) and passed through subsequent steps (a and b) for further validation as non-coding transcripts, i.e., 1) the transcripts with length ≥200bp, open reading frame (ORF) ≤100 aa, strand information (+/- strand), and CPC2 score <0.5 were selected using OrfPredictor (Min et al., 2005) and passed through annotation using the annotation file of the GCF_003121395.1 genome assembly by GffCompare (Burset and Guigo, 1996). 2) These were then searched against the NCBI-nr protein database through blastx (E value 0.01, coverage >80%, and identity >90%) and the Pfam protein database through HMMER (Finn et al., 2011). Finally, the validated lncRNAs were classified based on origin of lncRNAs as i (within a reference intron), j (alternative lncRNAs isoforms of known genes), o (lncRNAs with exonic overlap with a known transcript), u (intergenic lncRNAs), and x (exonic overlap on the opposite stand) as classified by Roberts et al. (2011). Transcripts with FPKM ≥0.5 for multi-exon transcripts and FPKM ≥1 for single-exon transcripts were selected as lncRNAs.
The Buffalo Genomic Resource Database, BuffGR is a ‘three tier architecture’ relational database developed using client, server, and database tiers. The analyzed datasets were catalogued in BuffGR on a Linux server. The following steps were involved in the development of BuffGR (Figure 1A): 1) Extraction of SNPs/InDels, SSR markers, lncRNAs, miRNAs, and circRNAs from the reference genomes of different breeds of buffalo and SRA data of 31 tissues of buffalo. These data are absolute, rather than having relative quantification. 2) Development of relational database in MySQL version 10.4.17, which includes 11 tables for all the fields, namely, for SNPs/InDels, SSR markers, lncRNAs, miRNAs, and circRNAs (Figure 1B); 3) development of web interface in PHP, HTML, and Java. Web hosting of this interface was done by Apache2 server version 3.2.4. A request was sent to the web server from the user’s system in PHP. A query was generated following the request of the user on the web server and sent to MySQL. The database response was prepared in MySQL and sent back to the web server. Finally, a response prepared in PHP was displayed in the user’s system.
FIGURE 1. (A) Database preparation and data retrieval for BuffGR; (B) Layout of data, data options, and data tables of BuffGR.
A total of 6028881 SNPs and 613403 InDels were extracted from the set of 31 buffalo tissues. The highest number of SNPs and InDels was extracted from milk tissue (1625901 SNPs/174256 InDels) followed by testis (448640 SNPs/46172 InDels) and large intestine (152608 SNPs/17552 InDels) (Figure 2A). However, the variants detected breed-wise showed a maximum number of SNPs and InDels in the Murrah breed (6313245 SNPs/510515 InDels), followed by Bangladesh (906446 SNPs/114319 InDels) and Egyptian (447224 SNPs/5920 InDels), while the least was seen in Jaffarabadi (60207 SNPs/3370 InDels) (Figure 2B). Table 3 represents the extracted tissue-wise genes showing abundance of SNPs and InDels by functional annotation. A total of 7727122 SNPs and 634124 InDels were collectively distributed in the four breeds of buffalo (Murrah, Bangladesh, Jaffarabadi, and Egyptian) with reference to the Mediterranean breed. From functional annotation of breed-wise SNP/InDels, 12326/8469, 15152/2044, 4798/1100, and 21762/17222 genes were found to have abundance of SNPs/InDels in Bangladesh, Egyptian, Jaffrabadi, and Murrah breeds, respectively (Figure 2C for SNPs and Figure 2D for InDels). SNP discovery plays an important role in obtaining varying alleles associated with different traits of interest (Mishra et al., 2020). This can be useful in marker trait association studies for various traits (Pareek et al., 2008).
FIGURE 2. Frequencies of SNP/InDels in (A) 31 different buffalo tissues (B) different breeds of buffalo: Common and unique genes with abundance of (C) SNPs and (D) InDels in different breeds of buffalo.
A total of 12 genes (SPP1: chr7, SCD: chr23, SREBF1: chr3, STAT1: chr2, TG: chr15, LALBA: chr4, INSIG2: chr2, GHRL: chr21, DGAT1: chr15, CSN1S1: chr7, BTN1A1: chr2, ADRA1A: chr3) with abundance of milk tissue SNPs from the present study were found to be common out of 19 candidate genes reported to be associated with milk production trait by Du et al. (2019) (Table 4). We also found 10 genes (COL1A2, APOB, GDF7, KLHL29, NRXN1, RGS22, VPS13B, MFSD14A, SLC35A3, PALMD) with abundance of SNPs of different breeds from the present study to be common out of 12 candidate genes for different QTL traits such as milk yield, fat yield, protein yield, fat %, and protein % identified from GWAS analysis of Italian Mediterranean buffalo using the SNP-ChIP technique by Iamartino et al. (2017) and Liu et al. (2017) (Table 4).
TABLE 4. Genes with abundance of extracted tissue/breed SNPs found to be common within the reported candidate genes of QTL traits.
Maximum number of SSRs were observed in Jaffrabadi (1028180), followed by Bangladesh (9463410) and Mediterranean (908402), while the least was found in Egyptian (726405) (Figure 3A). The number of SSRs based on repeat types (mono, di, tri, tetra, penta, hexa-nucleotide repeats) along with their proportions, frequency of SSRs per Mb, and distance between the two SSRs are listed in Table 5. In all the breeds, abundance of mononucleotides was observed which might be because of the inherent limitation of the chemistry employed in next-generation sequencing for data generation (Haseneyer et al., 2011) (Figure 3B). A similar higher proportion of mono repeats has been found in other animals like cattle, horse, and camel (Ma, 2016; Khalkhali-Evrigh et al., 2019). The relative distributions of various SSR motif lengths in genomes differ from species to species (Sharma et al., 2007). A total of 4329, 4284, 1435, 29822, and 4326 putative genes with an abundance of SSRs in Bangladesh, Egyptian, Jaffrabadi, Mediterranean, and Murrah breeds, respectively, were annotated. Figure 3C shows the common genes with abundance of SSRs in different breeds. The reported putative molecular markers can be used in marker trait association studies for buffalo genetic improvement programs (Sikka and Sethi, 2008; Bhuyan et al., 2010; Kannur et al., 2017).
TABLE 5. Breed-wise frequencies of SSRs, their proportions, SSR density, and distance between two SSRs in different repeat motifs.
FIGURE 3. (A) Breed-wise frequencies of SSRs. (B) Breed-wise representation of different repeat motifs. (C) Common and unique genes with abundance of SSRs in the five breeds of buffalo.
We identified a total of 938 miRNAs from the genome assembly of the Mediterranean breed. The pre-miRNA sequences, secondary structure, target information, and location of origin were extracted for each miRNA along with mature miRNA sequence and anti-miRNA star sequence. It was observed that chromosome 11 had the maximum frequency of miRNAs (132 miRNAs) followed by chromosomes 23 (81 miRNAs) and 13 (80 miRNAs) (Figure 4B). A target search for 938 miRNAs was performed, out of which 88 miRNAs were found to have 3451 mRNA targets (predicted mode of action of miRNAs was cleavage of mRNA targets to destroy them or binding with mRNA targets to sequester them) and included in the web resource. Protein encoded by target mRNA, aligned as paired-unpaired sequences of the binding site between mRNA target and miRNA, were also mentioned in the web resource. The miRNAs have the future prospective to be used as biomarkers and for disease management and treatment. miRNAs can be used as a powerful tool to understand the regulatory mechanisms related to disease pathogenesis (Singh et al., 2020; Do et al., 2021).
FIGURE 4. (A) Tissue-wise frequencies of circRNAs and lncRNAs (B) chromosome-wise frequencies of miRNAs and circRNAs; (C) length-wise frequencies of lncRNAs in buffalo.
Out of the total 1702 circRNAs extracted from the 31 buffalo tissues, 1458 were unique circRNAs. Figure 4A shows that the maximum number of circRNAs was found in milk (833) tissues followed by embryo pool (153), testis (88), and tongue (52) tissues. Information of genomic localization into intron, exon, and intergenic regions of circRNAs along with genes of origin and strand of origin was extracted by functional annotation of circRNAs from different tissues which were catalogued in the web resource. The chromosome-wise distribution of circRNAs showed that most of the circRNAs originated from chromosome 2 (227), followed by chromosomes 3 (160) and 4 (155) (Figure 4B). circRNAs have multiple regulatory roles which can enrich breeding and improve economic traits related to buffalo (Fu et al., 2018; He et al., 2021; Yang et al., 2021).
A total of 44221 lncRNAs were identified in the 31 buffalo tissues. Abundance of lncRNAs was observed in milk tissue 17387) followed by testis (5048) and pooled embryo 4419) (Figure 4A). Genomic annotation based on the site of origin of lncRNAs found distribution of 37712 unique lncRNAs into five classes such as intron (14252), isoform/pseudogene (1308), exon (1358), intergenic (17134), and antisense exon (3659) regions. Protein and transcript information was also included for genic origin of lncRNAs. Genomic annotation of unique lncRNAs from all tissues depicted abundance in the intergenic (17134), followed by intron 14252) regions in our study. The graphical representation of lncRNA frequencies based on their length showed that most lncRNAs had a length of 200–399 bps and had a decreasing trend in frequency with increase in lncRNA length (Figure 4C). The role of lncRNAs in genomic studies has been found to be critical in linking the gap between livestock genotype and phenotype (Kosinska-Selbi et al., 2020).
BuffGR is a comprehensive, first-of-its-kind web resource, with a holistic collection of buffalo molecular markers and variants of five buffalo breeds (Murrah, Mediterranean, Jaffarabadi, Bangladesh, and Egyptian). It is a user-friendly web resource, which catalogues SNPs, InDels, and SSRs along with ncRNAs such as mircoRNAs, lncRNAs, and circRNAs from the five buffalo breeds and 31 tissues. It has a left vertical section which provides access to varying sections of the web page including Home, Statistics, Data, and Team. The Home page includes the brief introduction of the buffalo web genomic resource along with a description about RNA/transcripts and molecular markers of buffalo. The Statistics section provides the statistics of extracted buffalo genomic data represented in the form of various graphs and pie charts.
The Data section includes hyperlinked images of each data point included in the web resource, and by clicking on the image, the user navigates to the next page of the respective data which provides the user varying options including type of tissue or chromosome number or breed, etc. (as shown in detail in Figure 1B). After selecting the combination of options, the user gets a complete table of the related data. The last column of each table provides a hyperlink to the genome browser, which navigates to the genomic location of the respective marker or ncRNA. In the case of miRNAs, each miRNA sequence is hyperlinked, which navigates to its mRNA target/s wherever available; the Team page includes the name of the team members with their profile. The Tutorial page guides users regarding the use of this web genomic resource (Figure 5).
The computational approach of discovery of SSR markers, SNPs, and InDels along with miRNAs, lncRNAs, and circRNAs utilizing the available genomic data of different breeds resulted in a ready-to-use, user-friendly, rapid, and economical approach for genomic resource development. The developed web resource, BuffGR can be of immense use to the international buffalo research community, which can utilize the information of genomic attributes from five breeds from India (Murrah and Jaffrabadi), Italy (Mediterranean), Bangladesh (Bangladesh), and Egypt (Egyptian). The catalogued SNP/InDel markers from different breeds could be used to study genetic diversity among different breeds of buffalo (Camargo et al., 2015; Deng et al., 2016; El-Halawany et al., 2017; Iamartino et al., 2017; Liu et al., 2017; Dutta et al., 2020). Highly variable SSR markers extracted in the present study could be utilized to find genetic diversity (Barker et al., 1997; Zhang et al., 2020). The SSR markers from different breeds could be used to find polymorphic SSRs (Moore et al., 1995) and their utilization in the study of genetic diversity of respective breeds (Moioli et al., 2001; Merdan et al., 2019; Vohra et al., 2021; Ünal et al., 2021). We also extracted ∼270000 polymorphic SSRs in the Mediterranean buffalo breed with respect to Murrah, Bangladesh, Jaffrabadi, and Egyptian breeds. The species-specific genetic markers (SNP/InDels and SSRs) can also be used as biomarkers of species to be used in the meat industry to trace adulteration or trafficking/traceability (Kannur et al., 2017).
Two coding variants were detected in the ASIP gene by Dutta et al. (2020), one synonymous variant at chr14:19947421 and another non-synonymous variant at chr14:19947429. Dutta et al. (2020) also reported that the alternative allele at the synonymous variant was not observed in Murrah, Surti, or Mediterranean breeds. The potential of extracted SNPs from this study as biomarkers can be seen from the example that the Murrah and Mediterranean breeds in the present study only had one non-synonymous SNP at 19947429 in the ASIP gene on chr14 as reported by Dutta et al. (2020). Significant SNPs could be utilized to find candidate genes specific to a certain function. The variants and SSRs can also be utilized in GWAS (El–Halawany et al., 2017) and later in MTA (marker trait association) analysis and QTL analysis by interval mapping (Deng et al., 2016; Mishra et al., 2020). The present study also shows the potential utilization of extracted markers in marker trait association as few of the genes with abundance of extracted tissue/breed SNPs were found in common with the candidate genes of the few reported QTL traits determined from GWAS studies. We found 12 genes with abundance of milk tissue SNPs to be in common with candidate genes of milk trait, and 10 genes with abundance of SNPs from different breeds to be in common with candidate genes of QTL traits such as milk yield, fat yield, protein yield, fat %, and protein % from other GWAS analyses (Iamartino et al., 2017; Liu et al., 2017; Du et al., 2020).
Tissue-specific lncRNAs could be helpful in studying post-transcriptional regulation by targeting certain mRNAs by cleaving or binding (Zhang et al., 2021) with target mRNAs. lncRNAs could be competitors of miRNAs, which targeted certain mRNAs, where lncRNAs sequestered miRNAs by binding to them and preventing miRNA from cleaving the respective mRNA (Li et al., 2020). Also, tissue-specific lncRNAs could be helpful in utilization in transcriptional regulation by targeting or modulating transcription regulatory proteins by facilitating their binding to a certain site or blocking binding at their target site (Cai et al., 2019; Pan et al., 2021). Another important fact is that the provided tissue-wise lncRNAs are the largest reported group of annotated lncRNAs of buffalo in a single study while several studies report tissue-specific lncRNAs in various species of livestock such as Bos taurus, Gallus gallus, Sus scrofa (Kosinska-Selbi et al., 2020), and Bos indicus (Alexandre et al., 2020). The TCONS_00011978 lncRNA, identified from muscle tissue in the present study, was reported to have regulatory potential in muscle with the highest degree of connectivity within the muscle network by Alexandre et al. (2020), reaffirming the potential of our extracted lncRNAs to be utilized in various future studies of buffalo. The buffalo miRNAs and their target mRNAs extracted in the present study can be utilized in post-transcriptional regulation of certain mRNAs and their encoding proteins by cleaving or binding with their target mRNAs (MacFarlane and Murphy, 2010; Hammond, 2015; Chen et al., 2020; Singh et al., 2020) along with recognition, de-capping, and degradation of 3′ UTR, and de-adenylation and adenylation of 3’ UTR of mRNAs (Shukla et al., 2011). The miRNAs could be used to find their lncRNAs target; action of miRNAs on lncRNAs could be sequestering them by binding or destroying them by cleaving (Assmann et al., 2019; Xie et al., 2020). The tissue-wise extracted circRNAs in the present study could be utilized in the studies of tissue-specific post-transcriptional regulation involving circRNAs and their role in various buffalo diseases (Gao et al., 2018; He et al., 2021; Lei et al., 2021; Yang et al., 2021).
SNP and SSR markers can also be used in parentage and relatedness testing required in breeding and conservation programs (Labuschagne et al., 2015). SNP markers can also be used in estimating inbreeding and effective population sizes required in conservation management monitoring genetic diversity (Panetta et al., 2017). They can be used to compute global co-ancestries of un-pedigreed populations. Such an approach can be of immense use in formulation of selective mating plans based on minimum co-ancestry mating and minimizing inbreeding (Fernández et al., 2005). Both SSR and SNP markers can be used in individual animal identification and breed traceability (Zhao et al., 2020). Water buffalo miRNAs and SNPs can be further used as genomic resources. Such use has been reported in cattle where SNPs and miRNAs have been found associated with bovine phenotypes to be used in breed improvement (Sousa et al., 2021).
Through this study, we report the first comprehensive and user-friendly web genomic resource for buffalo (BuffGR) including genomic findings of five commercially important buffalo breeds, namely Mediterranean, Egyptian, Bangladesh, Jaffrarabadi, and Murrah. BuffGR catalogues a total of 6028881 SNPs and 613403 InDeLs extracted from the set of 31 buffalo tissues. Collectively, a total of 7727122 SNPs and 634124 InDels were distributed in the four breeds of buffalo (Murrah, Bangladesh, Jaffarabadi, and Egyptian) with reference to the Mediterranean breed. The web resource has 4504691 SSR markers from all the breeds, 1458 unique circRNAs and 37712 lncRNAs from 31 buffalo tissues, and 938 miRNAs from the genome assembly of the Mediterranean breed. This information can be widely used by the buffalo researchers across the globe for studying the genetic diversity among the different breeds of buffalo, studies involving post-transcriptional regulation, and their role in various buffalo diseases. The provided markers can be used as biomarkers in the meat industry to trace adulteration, trafficking, and breed traceability. These can be used not only for knowledge discovery research but also for marker trait association, which will be helpful in the improvement and management of buffalo breeds.
The datasets presented in this study can be found in online repositories. The names of the repository/repositories and accession number(s) can be found in the article/Supplementary Material.
MI, DK, and SJ conceived the theme of the study. AaK, KS, SJ, MR, RJ, AnK, AG, JK, MI, and UA performed the computational analysis and developed genomic resources. KS, AaK, MI, SJ, and DK drafted the manuscript. VN, AR, TD, and DK edited the manuscript. All co-authors read and approved the final manuscript.
The authors declare that the research was conducted in the absence of any commercial or financial relationships that could be construed as a potential conflict of interest.
All claims expressed in this article are solely those of the authors and do not necessarily represent those of their affiliated organizations, or those of the publisher, the editors and the reviewers. Any product that may be evaluated in this article, or claim that may be made by its manufacturer, is not guaranteed or endorsed by the publisher.
The authors are thankful to the Indian Council of Agricultural Research (ICAR), Ministry of Agriculture and Farmers’ Welfare, Government of India for providing financial assistance in the form of a CABin grant as well as the use of the Advanced Super Computing Hub for Omics Knowledge in Agriculture (ASHOKA) facility at ICAR-IASRI, New Delhi, India created under the National Agricultural Innovation Project, and funded by the World Bank. The authors further acknowledge the supportive role of the Director of ICAR-IASRI, New Delhi and Director, ICAR-CIRB, Hisar, India. The grant of Junior Research Fellowship to AaK by the Indian Council of Agricultural Research is duly acknowledged. Authors are also thankful to Lal Bahadur Shastri Outstanding Young Scientist Scheme, ICAR for necessary support.
Alexandre, P. A., Reverter, A., Berezin, R. B., Porto-Neto, L. R., Ribeiro, G., Santana, M. H. A., et al. (2020). Exploring the Regulatory Potential of Long Non-coding RNA in Feed Efficiency of Indicine Cattle. Genes 11 (9), 997. doi:10.3390/genes11090997
Altschul, S. F., Gish, W., Miller, W., Myers, E. W., and Lipman, D. J. (1990). Basic Local Alignment Search Tool. J. Mol. Biol. 215 (3), 403–410. doi:10.1016/s0022-2836(05)80360-2
Assmann, T. S., Milagro, F. N., and Martínez, J. (2019). Crosstalk between microRNAs, the Putative Target Genes and the lncRNA Network in Metabolic Diseases. Mol. Med. Rep. 20 (4), 3543–3554. doi:10.3892/mmr.2019.10595
Barker, J. S. F., Moore, S. S., Hetzel, D. J. S., Evans, D., Byrne, K., and Tan, S. G. (1997). Genetic Diversity of Asian Water buffalo (Bubalus Bubalis): Microsatellite Variation and a Comparison with Protein-Coding Loci. Anim. Genet. 28 (2), 103–115. doi:10.1111/j.1365-2052.1997.00085.x
Beier, S., Thiel, T., Münch, T., Scholz, U., and Mascher, M. (2017). MISA-web: a Web Server for Microsatellite Prediction. Bioinformatics 33 (16), 2583–2585. doi:10.1093/bioinformatics/btx198
Bhat, S., and Jones, W. D. (2016). An Accelerated miRNA-Based Screen Implicates Atf-3 in Drosophila Odorant Receptor Expression. Sci. Rep. 6 (1), 1–8. doi:10.1038/srep20109
Bhuyan, D. K., Sangwan, M. L., Gole, V. C., and Sethi, R. K. (2010). Studies on DNA Fingerprinting in Murrah Buffaloes Using Microsatellite Markers. Indian J. Biotechnol. 9 (4), 367–370.
Burset, M., and Guigó, R. (1996). Evaluation of Gene Structure Prediction Programs. Genomics 34 (3), 353–367. doi:10.1006/geno.1996.0298
Cai, R., Tang, G., Zhang, Q., Yong, W., Zhang, W., Xiao, J., et al. (2019). A Novel Lnc-RNA, Named Lnc-ORA, Is Identified by RNA-Seq Analysis, and its Knockdown Inhibits Adipogenesis by Regulating the PI3K/AKT/mTOR Signaling Pathway. Cells 8 (5), 477. doi:10.3390/cells8050477
Chen, L., Zhang, S., Wu, J., Cui, J., Zhong, L., Zeng, L., et al. (2017). circRNA_100290 Plays a Role in Oral Cancer by Functioning as a Sponge of the miR-29 Family. Oncogene 36 (32), 4551–4561. doi:10.1038/onc.2017.89
Chen, Z., Xie, Y., Luo, J., Chen, T., Xi, Q., Zhang, Y., et al. (2020). Milk Exosome-Derived miRNAs from Water buffalo Are Implicated in Immune Response and Metabolism Process. BMC Vet. Res. 16 (1), 123–125. doi:10.1186/s12917-020-02339-x
Dai, X., and Zhao, P. X. (2011). psRNATarget: a Plant Small RNA Target Analysis Server. Nucleic Acids Res. 39 (Suppl. l_2), W155–W159. doi:10.1093/nar/gkr319
Danecek, P., Bonfield, J. K., Liddle, J., Marshall, J., Ohan, V., Pollard, M. O., et al. (2021). Twelve Years of SAMtools and BCFtools. GigaScience 10 (2), giab008. doi:10.1093/gigascience/giab008
de Camargo, G., Aspilcueta-Borquis, R., Fortes, M., Porto-Neto, R., Cardoso, D., Santos, D., et al. (2015). Prospecting Major Genes in Dairy Buffaloes. BMC Genomics 16 (1), 1–14. doi:10.1186/s12864-015-1986-2
Deng, T. X., Pang, C. Y., Liu, M. Q., Zhang, C., and Liang, X. W. (2016). Synonymous Single Nucleotide Polymorphisms in the MC4R Gene that Are Significantly Associated with Milk Production Traits in Water Buffaloes. Genet. Mol. Res. 15, 1–8. doi:10.4238/gmr.15028153
Dhanoa, J. K., Singh, J., Singh, A., Arora, J. S., Sethi, R. S., and Mukhopadhyay, C. S. (2019). Discovery of isomiRs in PBMCs of Diseased Vis-À-Vis Healthy Indian Water Buffaloes. ExRNA 1 (1), 1–12. doi:10.1186/s41544-019-0013-1
Do, D. N., Dudemaine, P.-L., Mathur, M., Suravajhala, P., Zhao, X., and Ibeagha-Awemu, E. M. (2021). miRNA Regulatory Functions in Farm Animal Diseases, and Biomarker Potentials for Effective Therapies. Ijms 22 (6), 3080. doi:10.3390/ijms22063080
Du, C., Deng, T., Zhou, Y., Ye, T., Zhou, Z., Zhang, S., et al. (2019). Systematic Analyses for Candidate Genes of Milk Production Traits in Water buffalo(Bubalus Bubalis). Anim. Genet. 50 (3), 207–216. doi:10.1111/age.12739
Dutta, P., Talenti, A., Young, R., Jayaraman, S., Callaby, R., Jadhav, S. K., et al. (2020). Whole Genome Analysis of Water buffalo and Global Cattle Breeds Highlights Convergent Signatures of Domestication. Nat. Commun. 11 (1), 1–13. doi:10.1038/s41467-020-18550-1
El-Halawany, N., Abdel-Shafy, H., Shawky, A.-E. -M. A., Abdel-Latif, M. A., Al-Tohamy, A. F. M., and Abd El-Moneim, O. M. (2017). Genome-wide Association Study for Milk Production in Egyptian buffalo. Livestock Sci. 198, 10–16. doi:10.1016/j.livsci.2017.01.019
FAOSTAT (2020). The Food and Agriculture Organization (FAO) of the United Nations Statistics Division. Available at: https://www.fao.org/faostat/en/#home (Accessed August 2021).
Fernandes, L. G. V., Guaman, L. P., Vasconcellos, S. A., Heinemann, M. B., Picardeau, M., and Nascimento, A. L. T. O. (2019). Gene Silencing Based on RNA-Guided Catalytically Inactive Cas9 (dCas9): a New Tool for Genetic Engineering in Leptospira. Sci. Rep. 9 (1), 1–14. doi:10.1038/s41598-018-37949-x
Fernández, J., Villanueva, B., Pong-Wong, R., and Toro, M. A. (2005). Efficiency of the Use of Pedigree and Molecular Marker Information in Conservation Programs. Genetics 170 (3), 1313–1321. doi:10.1534/genetics.104.037325
Finn, R. D., Clements, J., and Eddy, S. R. (2011). HMMER Web Server: Interactive Sequence Similarity Searching. Nucleic Acids Res. 39 (Suppl. l_2), W29–W37. doi:10.1093/nar/gkr367
Fu, Y., Jiang, H., Liu, J.-B., Sun, X.-L., Zhang, Z., Li, S., et al. (2018). Genome-wide Analysis of Circular RNAs in Bovine Cumulus Cells Treated with BMP15 and GDF9. Sci. Rep. 8 (1), 1–10. doi:10.1038/s41598-018-26157-2
Gao, Y., Wang, J., and Zhao, F. (2015). CIRI: an Efficient and Unbiased Algorithm for De Novo Circular RNA Identification. Genome Biol. 16 (1), 1–16. doi:10.1186/s13059-014-0571-3
Gao, Y., Wu, M., Fan, Y., Li, S., Lai, Z., Huang, Y., et al. (2018). Identification and Characterization of Circular RNAs in Qinchuan Cattle Testis. R. Soc. Open Sci. 5 (7), 180413. doi:10.1098/rsos.180413
Griffiths, A. J., Miller, J. H., Suzuki, D. T., Lewontin, R. C., and Gelbart, W. M. (2000). Transcription: An Overview of Gene Regulation in Eukaryotes. An Introduction to Genetic Analysis. 7th edition. New York: W. H. Freeman. Available at: https://www.ncbi.nlm.nih.gov/books/NBK21766/.
Griffiths-Jones, S., Grocock, R. J., Van Dongen, S., Bateman, A., and Enright, A. J. (2006). miRBase: microRNA Sequences, Targets and Gene Nomenclature. Nucleic Acids Res. 34 (Suppl. l_1), D140–D144. doi:10.1093/nar/gkj112
Hammond, S. M. (2015). An Overview of microRNAs. Adv. Drug Deliv. Rev. 87, 3–14. doi:10.1016/j.addr.2015.05.001
Haseneyer, G., Schmutzer, T., Seidel, M., Zhou, R., Mascher, M., Schön, C.-C., et al. (2011). From RNA-Seq to Large-Scale Genotyping - Genomics Resources for rye (Secale Cereale L.). BMC Plant Biol. 11 (1), 1–13. doi:10.1186/1471-2229-11-131
He, T., Chen, Q., Tian, K., Xia, Y., Dong, G., and Yang, Z. (2021). Functional Role of circRNAs in the Regulation of Fetal Development, Muscle Development, and Lactation in Livestock. Biomed. Res. Int. 2021, 5383210. doi:10.1155/2021/5383210
Huang, Y., Niu, B., Gao, Y., Fu, L., and Li, W. (2010). CD-HIT Suite: a Web Server for Clustering and Comparing Biological Sequences. Bioinformatics 26 (5), 680–682. doi:10.1093/bioinformatics/btq003
Iamartino, D., Nicolazzi, E. L., Van Tassell, C. P., Reecy, J. M., Fritz-Waters, E. R., Koltes, J. E., et al. (2017). Design and Validation of a 90K SNP Genotyping Assay for the Water buffalo (Bubalus Bubalis). PloS One 12 (10), e0185220. doi:10.1371/journal.pone.0185220
Iannuzzi, L. (1994). Standard Karyotype of the River buffalo (Bubalus Bubalis L., 2n = 50). Report of the Committee for the Standardization of Banded Karyotypes of the River buffalo. Cytogenet. Cel. Genet. 67 (2), 102–113. doi:10.1159/000133808
Kang, Y.-J., Yang, D.-C., Kong, L., Hou, M., Meng, Y.-Q., Wei, L., et al. (2017). CPC2: a Fast and Accurate Coding Potential Calculator Based on Sequence Intrinsic Features. Nucleic Acids Res. 45 (W1), W12–W16. doi:10.1093/nar/gkx428https://www.ncbi.nlm.nih.gov/assembly/GCF_003121395.1/ https://www.ncbi.nlm.nih.gov/assembly/GCA_000180995.3/ https://www.ncbi.nlm.nih.gov/assembly/GCA_004794615.1/ https://www.ncbi.nlm.nih.gov/assembly/GCA_002993835.1/ https://www.ncbi.nlm.nih.gov/assembly/GCF_019923935.1/
Kannur, B. H., Fairoze, M. N., Girish, P. S., Karabasanavar, N., and Rudresh, B. H. (2017). Breed Traceability of buffalo Meat Using Microsatellite Genotyping Technique. J. Food Sci. Technol. 54 (2), 558–563. doi:10.1007/s13197-017-2500-4
Kawamata, T., and Tomari, Y. (2010). Making Risc. Trends Biochemical Sciences 35 (7), 368–376. doi:10.1016/j.tibs.2010.03.009
Khalkhali-Evrigh, R., Hedayat-Evrigh, N., Hafezian, S. H., Farhadi, A., and Bakhtiarizadeh, M. R. (2019). Genome-wide Identification of Microsatellites and Transposable Elements in the Dromedary Camel Genome Using Whole-Genome Sequencing Data. Front. Genet. 10, 692. doi:10.3389/fgene.2019.00692
Kim, D., Paggi, J. M., Park, C., Bennett, C., and Salzberg, S. L. (2019). Graph-based Genome Alignment and Genotyping with HISAT2 and HISAT-Genotype. Nat. Biotechnol. 37, 907–915. doi:10.1038/s41587-019-0201-4
Kosinska-Selbi, B., Mielczarek, M., and Szyda, J. (2020). Review: Long Non-coding RNA in Livestock. Animal 14 (10), 2003–2013. doi:10.1017/s1751731120000841
Labuschagne, C., Nupen, L., Kotzé, A., Grobler, P. J., and Dalton, D. L. (2015). Assessment of Microsatellite and SNP Markers for Parentage Assignment in Ex Situ African Penguin ( Spheniscus demersus ) Populations. Ecol. Evol. 5 (19), 4389–4399. doi:10.1002/ece3.1600
Langmead, B., and Salzberg, S. (2012). Fast Gapped-Read Alignment with Bowtie 2. Nat. Methods 9, 357–359. doi:10.1038/nmeth.1923
Lecchi, C., Catozzi, C., Zamarian, V., Poggi, G., Borriello, G., Martucciello, A., et al. (2019). Characterization of Circulating miRNA Signature in Water Buffaloes (Bubalus Bubalis) during Brucella Abortus Infection and Evaluation as Potential Biomarkers for Non-invasive Diagnosis in Vaginal Fluid. Sci. Rep. 9 (1), 1945. doi:10.1038/s41598-018-38365-x
Leclercq, M., Diallo, A. B., and Blanchette, M. (2013). Computational Prediction of the Localization of microRNAs within Their Pre-miRNA. Nucleic Acids Res. 41 (15), 7200–7211. doi:10.1093/nar/gkt466
Lei, Z., Wu, H., Xiong, Y., Wei, D., Wang, X., Luoreng, Z., et al. (2021). ncRNAs Regulate Bovine Adipose Tissue Deposition. Mol. Cel Biochem 476 (7), 2837–2845. doi:10.1007/s11010-021-04132-2
Li, H., Handsaker, B., Wysoker, A., Fennell, T., Ruan, J., Homer, N., et al. (2009). The Sequence Alignment/Map Format and SAMtools. Bioinformatics 25, 2078–2079. doi:10.1093/bioinformatics/btp352
Li, H. (2011). A Statistical Framework for SNP Calling, Mutation Discovery, Association Mapping and Population Genetical Parameter Estimation from Sequencing Data. Bioinformatics 27 (21), 2987–2993. doi:10.1093/bioinformatics/btr509
Li, H., Huang, K., Wang, P., Feng, T., Shi, D., Cui, K., et al. (2020). Comparison of Long Non-coding RNA Expression Profiles of Cattle and buffalo Differing in Muscle Characteristics. Front. Genet. 11, 98. doi:10.3389/fgene.2020.00098
Liu, J. J., Liang, A. X., Campanile, G., Plastow, G., Zhang, C., Wang, Z., et al. (2017). Genome-wide Association Studies to Identify Quantitative Trait Loci Affecting Milk Production Traits in Water buffalo. J. Dairy Sci. 101, 433–444. doi:10.3168/jds.2017-13246
Liu, S., Ye, T., Li, Z., Li, J., Jamil, A. M., Zhou, Y., et al. (2019). Identifying Hub Genes for Heat Tolerance in Water buffalo (Bubalus Bubalis) Using Transcriptome Data. Front. Genet. 10, 209. doi:10.3389/fgene.2019.00209
Lorenz, R., Bernhart, S. H., Höner Zu Siederdissen, C., Tafer, H., Flamm, C., Stadler, P. F., et al. (2011). ViennaRNA Package 2.0. Algorithms Mol. Biol. 6 (1), 26–14. doi:10.1186/1748-7188-6-26
Lu, M. (2020). Circular RNA: Functions, Applications and Prospects. ExRNA 2 (1), 1–7. doi:10.1186/s41544-019-0046-5
Lukiw, W. J. (2013). Circular RNA (circRNA) in Alzheimer's Disease (AD). Front. Genet. 4, 307. doi:10.3389/fgene.2013.00307
Ma, Z.-J. (2016). Abundance and Characterization of Perfect Microsatellites on the Cattle Y Chromosome. Anim. Biotechnol. 28 (3), 157–162. doi:10.1080/10495398.2016.1243551
MacFarlane, L.-A., and Murphy, P. R. (2010). MicroRNA: Biogenesis, Function and Role in Cancer. Cg 11 (7), 537–561. doi:10.2174/138920210793175895
Memczak, S., Jens, M., Elefsinioti, A., Torti, F., Krueger, J., Rybak, A., et al. (2013). Circular RNAs Are a Large Class of Animal RNAs with Regulatory Potency. Nature 495 (7441), 333–338. doi:10.1038/nature11928
Meng, S., Zhou, H., Feng, Z., Xu, Z., Tang, Y., Li, P., et al. (2017). CircRNA: Functions and Properties of a Novel Potential Biomarker for Cancer. Mol. Cancer 16 (1), 1–8. doi:10.1186/s12943-017-0663-2
Merdan, S. M., El-Zarei, M. F., Ghazy, A., Ayoub, M. A., Al-Shawa, Z. M., and Mokhtar, S. A. (2019). Genetic Differentiation between Egyptian Buffalo Populations Using Microsatellite Markers. J. Anim. Poult. Fish Prod. 8 (1), 21–28.
Min, X. J., Butler, G., Storms, R., and Tsang, A. (2005). OrfPredictor: Predicting Protein-Coding Regions in EST-Derived Sequences. Nucleic Acids Res. 33 (Suppl. l_2), W677–W680. doi:10.1093/nar/gki394
Mishra, D. C., Sikka, P., Yadav, S., Bhati, J., Paul, S. S., Jerome, A., et al. (2020). Identification and Characterization of Trait-specific SNPs Using ddRAD Sequencing in Water buffalo. Genomics 112 (5), 3571–3578. doi:10.1016/j.ygeno.2020.04.012
Moioli, B., Georgoudis, A., Napolitano, F., Catillo, G., Giubilei, E., Ligda, C., et al. (2001). Genetic Diversity between Italian, Greek and Egyptian buffalo Populations. Livestock Prod. Sci. 70 (3), 203–211. doi:10.1016/S0301-6226(01)00175-0
Moore, S. S., Evans, D., Byrne, K., Barker, J. S. F., Tan, S. G., Vankan, D., et al. (1995). A Set of Polymorphic DNA Microsatellites Useful in Swamp and River buffalo (Bubalus Bubalis). Anim. Genet. 26 (5), 355–359. doi:10.1111/j.1365-2052.1995.tb02674.x
O'Brien, J., Hayder, H., Zayed, Y., and Peng, C. (2018). Overview of microRNA Biogenesis, Mechanisms of Actions, and Circulation. Front. Endocrinol. 9, 402. doi:10.3389/fendo.2018.00402
Pan, Y., Yang, S., Cheng, J., Lv, Q., Xing, Q., Zhang, R., et al. (2021). Whole-Transcriptome Analysis of LncRNAs Mediated ceRNA Regulation in Granulosa Cells Isolated from Healthy and Atresia Follicles of Chinese Buffalo. Front. Vet. Sci. 8, 680182. doi:10.3389/fvets.2021.680182
Panetto, J. C. D. C., Machado, M. A., da Silva, M. V. G. B., Barbosa, R. S., dos Santos, G. G., Leite, R. d. M. H., et al. (2017). Parentage Assignment Using SNP Markers, Inbreeding and Population Size for the Brazilian Red Sindhi Cattle. Livestock Sci. 204, 33–38. doi:10.1016/j.livsci.2017.08.008
Pareek, C. S., Czarnik, U., Pierzchała, M., and Zwierzchowski, L. (2008). An Association between the C> T Single Nucleotide Polymorphism within Intron IV of Osteopontin Encoding Gene (SPP1) and Body Weight of Growing Polish Holstein-Friesian Cattle. Anim. Sci. Pap. Rep. 26 (4), 251–257.
Patzak, J., Paprštein, F., Henychová, A., and Sedlák, J. (2012). Comparison of Genetic Diversity Structure Analyses of SSR Molecular Marker Data within Apple (Malus×domestica) Genetic Resources. Genome 55 (9), 647–665. doi:10.1139/G2012-054
Pertea, M., Pertea, G. M., Antonescu, C. M., Chang, T.-C., Mendell, J. T., and Salzberg, S. L. (2015). StringTie Enables Improved Reconstruction of a Transcriptome from RNA-Seq Reads. Nat. Biotechnol. 33, 290–295. doi:10.1038/nbt.3122
Roberts, A., Pimentel, H., Trapnell, C., and Pachter, L. (2011). Identification of Novel Transcripts in Annotated Genomes Using RNA-Seq. Bioinformatics 27 (17), 2325–2329. doi:10.1093/bioinformatics/btr355
Sharma, P. C., Grover, A., and Kahl, G. (2007). Mining Microsatellites in Eukaryotic Genomes. Trends Biotechnology 25 (11), 490–498. doi:10.1016/j.tibtech.2007.07.013
Shen, W., Le, S., Li, Y., and Hu, F. (2016). SeqKit: A Cross-Platform and Ultrafast Toolkit for FASTA/Q File Manipulation. PloS One 11 (10), e0163962. doi:10.1371/journal.pone.0163962
Shukla, G. C., Singh, J., and Barik, S. (2011). MicroRNAs: Processing, Maturation, Target Recognition and Regulatory Functions. Mol. Cel. Pharmacol. 3 (3), 83–92. PMC3315687.
Sikka, P., and Sethi, R. K. (2008). Genetic Variability in Production Performance of Murrah Buffaloes (Bubalus Bubalis) Using Microsatellite Polymorphism. Indian J. Biotechnol. 7, 103–107.
Singh, J., Dhanoa, J. K., Choudhary, R. K., Singh, A., Sethi, R. S., Kaur, S., et al. (2020). MicroRNA Expression Profiling in PBMCs of Indian Water Buffalo (Bubalus Bubalis) Infected with Brucella and Johne's Disease. ExRNA 2, 1–13. doi:10.1186/s41544-020-00049-y
Sousa, M. A. P. D., de Athayde, F. R. F., Maldonado, M. B. C., Lima, A. O. D., Fortes, M. R. S., and Lopes, F. L. (2021). Single Nucleotide Polymorphisms Affect miRNA Target Prediction in Bovine. Plos One 16 (4), e0249406. doi:10.1371/journal.pone.0249406
Surya, T., Vineeth, M. R., Sivalingam, J., Tantia, M. S., Dixit, S. P., Niranjan, S. K., et al. (2019). Genomewide Identification and Annotation of SNPs in Bubalus Bubalis. Genomics 111 (6), 1695–1698. doi:10.1016/j.ygeno.2018.11.021
Taşcioğlu, Y., Akpinar, M. G., Gül, M., Karli, B., and Bozkurt, Y. (2020). Determination of Optimum Agricultural Policy for buffalo Breeding. Revista Brasileira de Zootecnia 49, 1–10. doi:10.37496/rbz4920200120
Ünal, E. Ö., Işık, R., Şen, A., Geyik Kuş, E., and Soysal, M. İ. (2021). Evaluation of Genetic Diversity and Structure of Turkish Water Buffalo Population by Using 20 Microsatellite Markers. Animals 11 (4), 1067. doi:10.3390/ani11041067
Untergasser, A., Cutcutache, I., Koressaar, T., Ye, J., Faircloth, B. C., Remm, M., et al. (2012). Primer3-new Capabilities and Interfaces. Nucleic Acids Res. 40, e115. doi:10.1093/nar/gks596
Vohra, V., Singh, N. P., Chhotaray, S., Raina, V. S., Chopra, A., and Kataria, R. S. (2021). Morphometric and Microsatellite-Based Comparative Genetic Diversity Analysis in Bubalus Bubalis from North India. PeerJ 9, e11846. doi:10.7717/peerj.11846
Wang, K., Gan, T.-Y., Li, N., Liu, C.-Y., Zhou, L.-Y., Gao, J.-N., et al. (2017). Circular RNA Mediates Cardiomyocyte Death via miRNA-dependent Upregulation of MTP18 Expression. Cell Death Differ. 24 (6), 1111–1120. doi:10.1038/cdd.2017.61
Xie, F., Liu, Y. L., Chen, X. Y., Li, Q., Zhong, J., Dai, B. Y., et al. (2020). Role of MicroRNA, LncRNA, and Exosomes in the Progression of Osteoarthritis: a Review of Recent Literature. Orthop. Surg. 12 (3), 708–716. doi:10.1111/os.12690
Xue, C., Li, F., He, T., Liu, G. P., Li, Y., and Zhang, X. (2005). Classification of Real and Pseudo microRNA Precursors Using Local Structure-Sequence Features and Support Vector Machine. BMC Bioinformatics 6 (1), 310–317. doi:10.1186/1471-2105-6-310
Yang, Z., He, T., and Chen, Q. (2021). The Roles of CircRNAs in Regulating Muscle Development of Livestock Animals. Front. Cel. Dev. Biol. 9, 163. doi:10.3389/fcell.2021.619329
Zhang, R., Wang, J., Xiao, Z., Zou, C., An, Q., Li, H., et al. (2021). The Expression Profiles of mRNAs and lncRNAs in Buffalo Muscle Stem Cells Driving Myogenic Differentiation. Front. Genet. 12, 1048. doi:10.3389/fgene.2021.643497
Zhang, Y., Colli, L., and Barker, J. S. F. (2020). Asian Water buffalo: Domestication, History and Genetics. Anim. Genet. 51 (2), 177–191. doi:10.1111/age.12911
Zhao, C., Qiu, J., Agarwal, G., Wang, J., Ren, X., Xia, H., et al. (2017). Genome-wide Discovery of Microsatellite Markers from Diploid Progenitor Species, Arachis Duranensis and A. Ipaensis, and Their Application in Cultivated Peanut (A. hypogaea). Front. Plant Sci. 8, 1209. doi:10.3389/fpls.2017.01209
Keywords: bovine, lncRNA, miRNA, molecular markers, web-resource, CircRNAs
Citation: Khan A, Singh K, Jaiswal S, Raza M, Jasrotia RS, Kumar A, Gurjar AKS, Kumari J, Nayan V, Iquebal MA, Angadi UB, Rai A, Datta TK and Kumar D (2022) Whole-Genome-Based Web Genomic Resource for Water Buffalo (Bubalus bubalis). Front. Genet. 13:809741. doi: 10.3389/fgene.2022.809741
Received: 05 November 2021; Accepted: 14 February 2022;
Published: 11 April 2022.
Edited by:
Basharat Ahmad Bhat, University of Otago, New ZealandReviewed by:
Qianjun Zhao, Institute of Animal Sciences (CAAS), ChinaCopyright © 2022 Khan, Singh, Jaiswal, Raza, Jasrotia, Kumar, Gurjar, Kumari, Nayan, Iquebal, Angadi, Rai, Datta and Kumar. This is an open-access article distributed under the terms of the Creative Commons Attribution License (CC BY). The use, distribution or reproduction in other forums is permitted, provided the original author(s) and the copyright owner(s) are credited and that the original publication in this journal is cited, in accordance with accepted academic practice. No use, distribution or reproduction is permitted which does not comply with these terms.
*Correspondence: Mir Asif Iquebal, bWEuaXF1ZWJhbEBpY2FyLmdvdi5pbg==
†These authors have contributed equally to this work
Disclaimer: All claims expressed in this article are solely those of the authors and do not necessarily represent those of their affiliated organizations, or those of the publisher, the editors and the reviewers. Any product that may be evaluated in this article or claim that may be made by its manufacturer is not guaranteed or endorsed by the publisher.
Research integrity at Frontiers
Learn more about the work of our research integrity team to safeguard the quality of each article we publish.