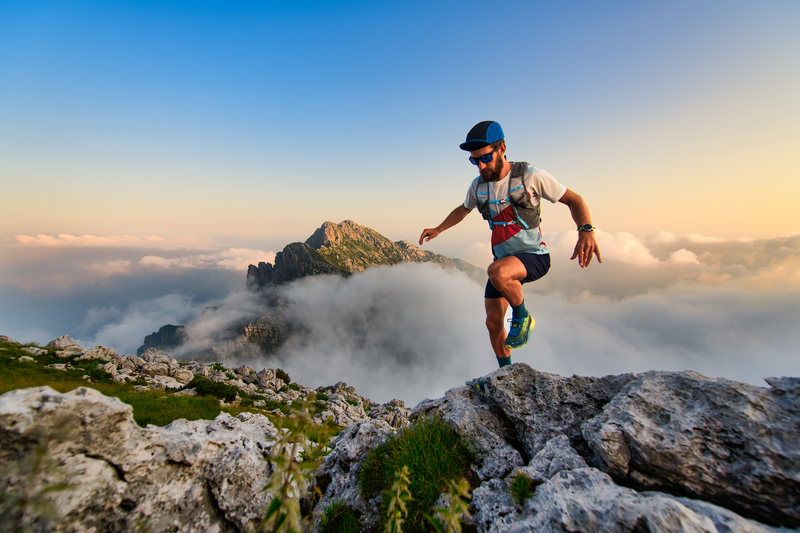
95% of researchers rate our articles as excellent or good
Learn more about the work of our research integrity team to safeguard the quality of each article we publish.
Find out more
ORIGINAL RESEARCH article
Front. Genet. , 04 March 2022
Sec. Statistical Genetics and Methodology
Volume 13 - 2022 | https://doi.org/10.3389/fgene.2022.799913
This article is part of the Research Topic Systems Genetics of Human Complex Diseases, Volume II View all 24 articles
Glioma is a primary high malignant intracranial tumor with poorly understood molecular mechanisms. Previous studies found that both DNA methylation modification and gene alternative splicing (AS) play a key role in tumorigenesis of glioma, and there is an obvious regulatory relationship between them. However, to date, no comprehensive study has been performed to analyze the influence of DNA methylation level on gene AS in glioma on a genome-wide scale. Here, we performed this study by integrating DNA methylation, gene expression, AS, disease risk methylation at position, and clinical data from 537 low-grade glioma (LGG) and glioblastoma (GBM) individuals. We first conducted a differential analysis of AS events and DNA methylation positions between LGG and GBM subjects, respectively. Then, we evaluated the influence of differential methylation positions on differential AS events. Further, Fisher’s exact test was used to verify our findings and identify potential key genes in glioma. Finally, we performed a series of analyses to investigate influence of these genes on the clinical prognosis of glioma. In total, we identified 130 glioma-related genes whose AS significantly affected by DNA methylation level. Eleven of them play an important role in glioma prognosis. In short, these results will help to better understand the pathogenesis of glioma.
Glioma is the most common and highly malignant primary intracranial tumor which is characterized by substantial heterogeneity and extremely poor prognosis in central nervous system (CNS) (Dong and Cui 2020; Pan et al., 2021). The World Health Organization (WHO) defines grade IV glioma as the glioblastoma (GBM). The annual incidence of this disease worldwide is about 5 cases per 100,000 people (Hottinger et al., 2014), and shows a significant mortality and unclarified molecular mechanism of the occurrence and development (Hottinger et al., 2014; Dong and Cui 2020). Although the etiology of glioma has been extensively studied, there are still many challenges and unknowns in the epigenetic mechanism of its pathogenesis and progress (Molinaro et al., 2019).
Recently, the DNA methylation has been demonstrated to extensively participate in the epigenetic mechanisms of CNS (Hwang et al., 2017), and many methyltransferase and demethylase-related genes (e.g., MGMT, CD44, HYAL2, SPP1, MMP2) contribute to the pathogenesis of glioma (Weller et al., 2010; Wiestler et al., 2014; Xiao et al., 2020). A large amount of the evidence showed that DNA methylation is involved in the occurrence and development of glioma tumors (Etcheverry et al., 2010; Chen et al., 2020; Dong and Cui 2020). For example, in GBM patients, the disease-related important signaling pathways (e.g., RB1 and TP53) are affected by CpG island promoter hyper-methylation (Etcheverry et al., 2010). The promoter methylation of DNA repair enzymes (O6-methylguanine-DNA methyltransferase) has been identified as a significant prognostic factor for temozolomide resistance in GBM patients (Chen et al., 2020).
Conversely, the previous studies reported that pathogenesis of glioma is significantly associated with the dysregulated alternative splicing (AS) in the brain (Mogilevsky et al., 2018; Pattwell et al., 2020; Zeng et al., 2020). AS is the primary driving force behind generating diverse proteins, which is the basis for the remarkable and complex functional regulation seen in eukaryotic cells (Xie et al., 2019). Genome-wide studies showed that 90–95% of human genes undergo some level of AS, and almost one-third of them were proved to be generated multiple protein isoforms (Kim et al., 2014; Wang et al., 2021). These processes usually show an extreme complexity in brain tissues and can play an important role in the progression of many CNS diseases (Merkin et al., 2012; Galarza-Munoz et al., 2017; Consortium 2020). For glioma, for instance, Mogilevsky et al. discovered that the manipulation of MKNK2 AS significantly suppressed the oncogenic properties of GBM cells and resensitized the cells to chemotherapy (Mogilevsky et al., 2018). Pattwell et al. found that a truncated splice variant, TrkB.T1, increases PDGF-induced Akt and STAT3 signaling and further enhances PDGF-driven GBM in vivo (Pattwell et al., 2020). Moreover, many previous studies indicate that there is a strong link between DNA methylation and AS and it generally contributes to the pathogenesis of CNS disorders, including glioma (Feng et al., 2019; Li et al., 2019). For example, transcriptome analysis revealed that PTEN methylation influences mature mRNA transcripts through modulation of pre-mRNA AS, and the methylation-defective PTEN R159K mutant is found most frequently in glioma patients. There was mark dysregulation of splicing factors in the PTEN-deficient GBM samples (Feng et al., 2019). The important oncogene METTL3 is a methyltransferase and it is found to modulate the nonsense-mediated mRNA decay of splicing factors and AS isoform switches in GBM. The methylation modification of serine- and arginine-rich splicing factors by METTL3 promotes GBM tumor growth and progression (Li et al., 2019).
However, so far, there has been no systematic study to explore the relationship between glioma-related DNA methylation and gene AS in the whole genome scale, and the influence of their interaction on the pathogenesis and progress of glioma. Therefore, in this study, we performed a genome-wide analysis by integrating the DNA methylation and AS data of 537 low-grade glioma (LGG) and GBM individuals. First, we downloaded the relevant data from the Cancer Genome Atlas (TCGA), TCGA SpliceSeq and EWASdb database, respectively. Second, we conducted the differential analysis between LGG and GBM samples to identify the glioma-related methylation positions and AS events. Third, based on the results, we performed a splicing quantitative trait methylation loci (defined as me-sQTL (Gutierrez-Arcelus et al., 2015; Han and Lee 2017)) analysis to explore the influence of DNA methylation level on gene AS in glioma. Fourth, we further explored the characteristics of these me-sQTLs and affected AS events. Fifth, combining the data of disease risk methylation positions from EWASdb, we performed the two-tailed Fisher’s exact test to investigate the disease specificity of the me-sQTLs and identify the potential key genes related to them in glioma. Finally, based on these potential key genes and clinical data, we conducted the least absolute shrinkage, univariate Cox regression, selection operator (LASSO) regression, clinical correlation and survival analysis to explore the influence of these genes whose AS events affected by DNA methylation on clinical prognosis of glioma. The flow chart is shown in Figure 1.
FIGURE 1. The flow chart of the study design for exploring the influence of DNA methylation level on gene AS in glioma and its impact on disease prognosis.
Clinical and methylation information of glioma patients was downloaded from the TCGA database (http://cancergenome.nih.gov), a comprehensive resource containing multi-omics data from various cancers. According to the annotation of TCGA, glioma is classified as the LGG and the GBM. TCGA is a global genomic profiling project that utilizes high-throughput microarray technologies to identify molecular subtype classifications of cancers, multigene clinical predictors, new targets for drug therapy, and predictive markers for these therapies (Vigneswaran et al., 2015). The International Classification of Diseases for Oncology has been used for nearly 25 years as a tool for coding diagnoses of neoplasms in tumor and cancer registrars and in pathology laboratories (Warzel et al., 2003). Data analysis was performed with the glioma classification LGG and GBM provided by the TCGA database. Current glioma classifications are based on the 2007 WHO grading scale, which separates gliomas based on cytologic features and degrees of malignancy after hematoxylin and eosin (H&E) staining (Erridge et al., 2011). According to the classification of gliomas in the TCGA database, data analysis is carried out by using the classifications LGG and GBM of gliomas provided by the TCGA database. We accessed these TCGA data using the Genomic Data Commons (GDC) data portal (https://portal. gdc. cancer.gov/). Particularly, based on our previous study (He et al., 2020), we first selected “DNA methylation” for the Data Category, “Illumina human methylation 450” for the Platform, “brain” for the Primary Site and “gliomas” in the Disease Type to screen out the suitable methylation array of patients in the GDC data portal. Then, the“clinical,” “brain” and “gliomas” were selected to the Data Category, Primary Site and Disease Type, respectively, to screen out the clinical information of patients in the GDC data portal. Finally, we removed samples that lacked methylation or clinical information.
The AS events of these samples were obtained from the TCGA SpliceSeq database (http://bioinformatics.mdanderson.org/TCGASpliceSeq), which identifies AS events and describes their genome profiles using the RNA-seq data of the TCGA samples (Ryan et al., 2016). Particularly, we downloaded the AS isoform average percent spliced-in (PSI) values of the LGG and GBM samples, respectively, from TCGA SpliceSeq database with the common parameter settings (i.e., the percentage of samples with PSI value >75%, minimum PSI range >0 and minimum PSI standard deviation >0.1) according to the previous studies (Yang et al., 2019; Rong et al., 2020; Wei et al., 2021). Based on the classification criteria of TCGA SpliceSeq, we classified the types of AS events into Alternate Acceptors (AA), Alternate Donors (AD), Exon Skip (ES), Retained Intron (RI), Alternate Promoters (AP), Alternate Terminators (AT) and Mutually Exclusive Exons (ME). The AS events that are not present in both LGG and GBM samples were removed.
Moreover, the information of disease risk methylation positions was obtained from the EWASdb database (http://www.bioapp.org/ewasdb/index.php/Index/index). EWASdb is a specialized epigenome-wide association database which stores the results of 1,319 epigenome-wide association study (EWAS) studies involved in the 302 diseases/phenotypes with the threshold for significance p < 1 × 10–7 (Liu et al., 2019). We downloaded the EWAS single epi-marker and annotation files (phenotype/disease info) and merged the files by the disease codes.
To obtain the glioma-related methylation positions, we performed differential methylation analysis between GBM and LGG samples. In particular, we used a Subset-quantile Within Array Normalization method to preprocess the methylation data by the R package “minfi,”, a specialized tool for the analysis of the Illumina methylation 450 array dataset (http://bioconductor.org/packages/release/bioc/html/minfi.html) (Aryee et al., 2014). Then, the quality control of methylation array was conducted “densityBeanPlot” function of this package. The characteristics of the qualified samples show that the methylation levels (beta values) of CpG positions are distributed around 0 and 1, respectively. Finally, based on the qualified methylation array data, we used a bump-hunting algorithm to identify the differentially methylated positions between GBM and LGG subjects by the “dmpFinder” function of this package. The parameter was set by its default value (i.e., type = “categorical”) and the significance level was set according to a common threshold for the absolute intercept ≥0.2 (i.e. 20% difference on the beta values) and the p value <1 × 10–3 (Guo et al., 2015).
To identify the glioma-related AS events and corresponding genes, we performed the differential AS events analysis and gene annotation. Particularly, the differential AS events analysis was conducted by the vast-tools software (Irimia et al., 2014). Based on the PSI of each AS event, we performed a Bayesian inference-based differential AS analysis by the “diff” function of vast-tools software with its default parameters. According to the previous studies, we set the threshold for significance at the minimum value for absolute value of differential PSI between GBM and LGG samples (MV|ΔPSI|) at 0.95 confidence level greater than 10% (Ha et al., 2021; Hekman et al., 2021). The gene annotation was conducted by g:Profiler toolset, a web server for conversions between gene identifiers and functional annotation (Raudvere et al., 2019). We used the g:Profiler to identify these AS events corresponding genes, convert their ID and annotate the genome location and type of the genes. The annotation file (hg19) from the database (release 75) were used for these analyses (Aken et al., 2017).
To explore the effect of methylation on AS events in glioma, we performed a cis me-sQTL analysis by combining the PSI values of differential AS events and the beta values of differentially methylated positions from the same samples. Particularly, we first considered the distance between the differentially methylated positions and the transcription initiation site (TSS) of differential AS events corresponding genes less than 1 M as the cis region, and selected all methylation positions and AS event pairs that met the conditions for the cis me-sQTL analysis. The annotation files of the Illumina methylation 450 array dataset (hg19) and Ensembl database (release 75) were used to locate the genomic locations of the methylated positions and the TSS of AS events corresponding genes, respectively. Then, based on the beta values of the differentially methylated positions in combination with the PSI values of the corresponding differential AS events, we used a linear regression model to perform a cis me-sQTL analysis by the R package “Matrix eQTL” with the parameters, age, and gender as covariates (Shabalin 2012). Finally, we conducted a multiple testing by Benjamini–Hochberg method to correct the p values of the cis me-sQTL analysis and set false discovery rate (FDR) q value less than 0.05 as the threshold for significance level according to the previous studies (Gillies et al., 2018; Drag et al., 2019; Han et al., 2020).
In order to explore the disease specificity of these cis me-sQTLs and further verify our findings as well as identify the potential key glioma-related genes with affected AS events by methylation level, we performed the two-tailed Fisher’s exact test by combining the disease risk methylation positions and the results of cis me-sQTLs analysis. Particularly, we first produced the disease risk methylation position datasets for various disorders including glioma from EWASdb database (Liu et al., 2019). Then, we defined the methylation positions which were unlikely to have an effect on the AS events in cis region (p > 0.05) as the non me-sQTLs. Next, by the two-tailed Fisher’s exact test, we compared the proportions of all these cis and non me-sQTLs in the disease risk methylation positions for each of the disorders to explore the disease specificity and further verify previous findings. The threshold for significance level was set as the p value <0.05. Finally, to identify the potential key glioma-related genes at the me-sQTL level, we compared the proportions of cis and non me-sQTLs in glioma-related methylation positions for each gene using the two-tailed Fisher’s exact test (the threshold of p < 0.05). The “fisher.test” function of R was used for these calculations.
We further analyzed the influence of these potential key genes whose AS events are affected by DNA methylation on clinical prognosis of glioma. First, we calculated the average expression of these genes in each individual and separated the samples into low and high expression groups according to the median of average expression. Then, we used the Kaplan-Meier overall survival curves to compare prognosis between the high expression and low expression individuals. Next, we performed a univariate Cox regression analysis to assess the association between these me-sQTL genes and the prognosis of glioma. The threshold of significance was set at 95% confidence interval (CI) of hazard ratio (HR) ⊉ 1 and p < 0.05. Then, based on the results of univariate Cox regression analysis, the R package “glmnet” was used to perform the LASSO regression analysis, a fit algorithm based on cyclical coordinate descent and warm start search along a regularization path, to identify the main glioma prognosis-related genes (Simon et al., 2011). According to the common parameter settings, the maxit and alpha were set at 1,000 and 1, respectively, and others were set by their default values. Based on the results, the risk scores were calculated for each subject by the R package “survival” (http://CRAN.R-project.org/package=survival). Further, the receiver operator characteristic (ROC) curve was used to verify the reliability of these risk scores by the R package “survivalROC” (https://CRAN.R-project.org/package=survivalROC). Finally, we used the chi-square test to assess the association between the expression of these glioma prognosis-related genes and other clinical features of the patients, which included the age at initial pathologic diagnosis, the vital status, and the gender. The threshold for significance was set at the p value <0.05.
In total, we obtained the datasets of DNA methylation values, AS events PSI values, gene expression levels and clinical information from 537 glioma samples (including 486 LGG and 51 GBM patients). The summary of these glioma samples was shown in Table 1. Particularly, after the missing value filtering and normalization processing, we quantified a total of 369,531 CpGs methylation positions with the normalized values according to the annotation files of Illumina human methylation 450 array. The results of normalization processing were shown in the Supplementary Figure S1 and our previous study (He et al., 2020). We obtained 7,414 AS events with the PSI values from 537 glioma samples the TCGA SpliceSeq database. These AS events are composed of about 39.0% ES, 27.8% AP, 11.3% AT, 8.4% RI, 6.9% AD, 5.9% AA and 0.5% ME types (Figure 2A). The expression data of 20,530 genes of the glioma samples was downloaded from the TCGA database and quantified by RSEM values. The clinical information of these samples contains age, gender, survival time, and vital status. Moreover, after the combination of same disease types and missing value filtering, we obtained a total of 141 disease risk methylation position data sets from the EWASdb database.
FIGURE 2. The characteristic of the cis me-sQTLs and the affected AS events. (A) The pie charts show the proportion in all (left), differential (middle) and DNA methylation affected AS events (right) annotated with each class (AA, AD, ES, RI, AP, AT and ME), respectively. (B) The blue bar graphs indicate the relationship between the abundance of the cis me-sQTLs and the distance of them to TSS of corresponding AS events. The red dots indicate the relationship between the statistical significance of the cis me-sQTLs associated with AS and the distance of them to TSS of corresponding AS events. (C) The disease specificity of the cis me-sQTLs by the two-tailed Fisher’s exact test. (D) The glioma specificity of the cis me-sQTLs in each gene by the two-tailed Fisher’s exact test. The black bars in histogram represent 95% confidence intervals.
We performed a differential methylation analysis between the LGG and GBM subjects to identify the glioma-related DNA methylation positions. All of the methylation array data met quality control metrics. The results showed that the beta values of DNA methylation positions are mainly distributed around 0 and 1, respectively, for each sample. The details are described in the Supplementary Figure S2 and our previous study (He et al., 2020). By the differential methylation analysis, we identified a total of 208,138 positions with a significantly different methylation level between LGG and GBM subjects. The results are shown in the Supplementary Table S1 and our previous study (He et al., 2020).
To identify the glioma-related AS events, we further conducted differential AS events between the LGG and GBM subjects. According to the significance threshold MV|ΔPSI| at 0.95 confidence level ≥10%, we identified a total of 287 differential AS events between LGG and GBM subjects. These differential AS events belonged to 263 genes (Supplementary Table S2). Figure 3 shows the most significant differential AS events (SpliceSeq ID: 96726) of LPHN3 gene (MV|ΔPSI| at 0.95 confidence level = 0.25). A recent study reported that LPHN3 was an important paralog of EVA1C which leads to the high infiltration levels of multiple immune cells in glioma (Hu and Qu 2021). Moreover, according to the classification criteria for SpliceSeq database, about 35.5%, 31.0%, 14.0%, 9.1%, 5.2% and 5.2% of these identified AS events are categorized into ES, AP, AT, RI, AD and AA types, respectively (Figure 2A). We did not find a significant difference in the proportion of AS event types when compared with the original AS event type proportion by the two-tailed Fisher’s exact test (Figure 2A). This revealed a typological universality of the differential AS events in glioma.
FIGURE 3. The results of differential analysis for the AS event 96726 of LPHN3 gene. (A) The red line indicates that the maximum probability of ΔPSI of AS event 96726 between LGG and GBM subjects is greater than 0.25. (B) The histogram shows the two joint posterior distributions over PSI and the point estimates for each replicate.
Combining the PSI values of differential AS events with the beta values of differentially methylated positions from the same samples, we used a linear regression model to perform the cis me-sQTL analysis by R package “Matrix eQTL” with the parameters, age, and gender serving as covariates. In total, we identified 19,345 methylated positions affecting 256 AS events which are involved in 233 genes (over 88% of the total differential genes) with a significance level of FDR q < 0.05. This revealed a general influence of DNA methylation level on gene AS in glioma. The top 25 significant results are shown in Table 2 (the full information is listed in the Supplementary Table S3). Among the 256 affected AS events, we found that about 34.1%, 32.2%, 14.6%, 9.6%, 4.6% and 5.0% of these affected AS events are categorized into ES, AP, AT, RI, AD and AA types, respectively. By the two-tailed Fisher’s exact test, we also did not find a significant difference of percentage between the affected and the original AS event types (Figure 2A). This revealed a typological non-specific regulation of gene AS by DNA methylation level in glioma. Further, we explored the relationship between the significance of regulation of the cis me-sQTLs and the distance of them to the TSS of the corresponding affected gene, and their distribution characteristics in genome. The results showed that these cis me-sQTLs tended to be distributed in the proximity of the corresponding affected gene TSS, and there were more significant regulatory effects of them in these regions (Figure 2B). This was consistent with the findings of previous studies (Pangeni et al., 2018; Chen and Elnitski 2019).
TABLE 2. The top 25 significant results of the me-sQTLs and the differential AS events affected by the methylated position.
To explore the disease specificity of these cis me-sQTLs and verify our findings, as well as further identify the potential key glioma-related genes at the me-sQTL level, we performed the two-tailed Fisher’s exact test using the disease risk methylation position data from the EWASdb database. We found that the risk methylation positions of all the 141 diseases are overlapped with the cis me-sQTLs and non me-sQTLs. By comparing the proportions of cis me-sQTLs and non me-sQTLs in each disease risk methylation position dataset (the threshold of Fisher’s p value <0.05), we found that the cis me-sQTLs significantly enriched the risk methylation position dataset of 103 diseases, which are mainly composed of CNS disorders and malignant tumor diseases including glioma (odds ratio (OR) = 2.49, p = 0). In contrast, the remaining 38 diseases, whose risk methylation positions are not significantly enriched by the cis me-sQTLs, are mainly composed of the other types of disorders, e.g., the rheumatic heart disease (p = 5.99 × 10–1), septicemia (p = 6.97 × 10–1), and Infertile (p = 1). The top 5 most and least significant results are shown in Figure 2C (the full information is listed in the Supplementary Table S4). The results revealed the specificity and similarity of neuro-oncological disorders at the me-sQTL level and verified the association of the cis me-sQTLs we identified with glioma. Further, for each type of AS event and each gene, we compared the proportions of their cis and non me-sQTLs in glioma risk methylation position dataset, respectively. The results showed that the cis me-sQTLs of almost all types of AS events are significantly enriched in glioma risk methylation position dataset, i.e., AA (OR = 4.76, p = 1.18 × 10–36), AT (OR = 2.85, p = 9.63 × 10–66), ES (OR = 2.39, p = 6.26 × 10–112), RI (OR = 2.05, p = 2.85 × 10–30), AD (OR = 2.55, p = 4.78 × 10–21), and AP (OR = 2.88, p = 8.69 × 10–191), and there are a total of 130 genes whose cis me-sQTLs are significantly enriched in glioma risk methylation position dataset (p < 0.05). Figure 2D shows the top 20 significant results and full information is listed in the Supplementary Table S5. We considered that these genes are more correlated with the pathogenesis of glioma at the me-sQTL level and selected them for the following prognosis analysis of glioma.
We further analyzed the influence of the potential key genes which are associated with glioma in me-sQTL level on the clinical prognosis of glioma. The expression data were obtained from TCGA database and these data are involved in 117 of the 130 potential key genes. We found that the overall survival curve of the subjects with high expression of these genes is significantly longer than the subjects with low expression (p = 7.56 × 10–1 (Figure 4A). This revealed that the expression dysregulation of these potential key genes is significantly associated with the bad prognosis of glioma patients. To avoid dependence between the 117 genes and identify the main glioma prognosis-related genes, we performed the univariate Cox regression analysis of the 117 genes. However, the results showed that 61me-sQTL genes identified are high-risk factors for the prognosis of glioma subjects (i.e. 95% CI HR ⊉ 1 and p < 0.001) (Supplementary Figure S3). We discover that both over-expression of those 30 genes and under-expression of the other 31 genes can lead to a poor prognosis in glioma patients, which is also consistent with common sense. given that patients are in advanced stages of the disease and their survival may be affected by other complications or factors. Then, we further applied the LASSO regression algorithm to conduct the selection and calculate the risk score of each subject to univariate Cox regression results. The results showed that there are 11 genes (i.e., KIF3A, HAUS1, TMCC1, BEND7, B3GNT5, MTMR3, ITGB3, BICD1, EXTL3, SUN1 and MXRA8) identified when the cross-validated partial likelihood deviance reaches its minimum value (Figures 4B,C). Among the 11 genes, the coefficients of 7 were positive (i.e., increase risk of disease), and others were negative (i.e., decrease risk of disease). A previous study reported that the low expression of the TMCC1 gene confers poor clinical prognoses of glioma patients which is in accordance with our findings (Pangeni et al., 2018). The area under the curve (AUC) of the ROC is 0.988, which reveals the reliability of the risk score (Figure 4D). According to the median of risk scores, the patients were separated into the low and high-risk groups. We found that the LGG and GBM subjects are mainly distributed in the low and high-risk group, respectively, which reflects the consistency between the risk scores calculated by the prognosis-related me-sQTL genes and the severity of glioma. Moreover, the results of chi-square test showed that the risk scores of the prognosis-related me-sQTL genes are also associated with the age at initial pathologic diagnosis (p = 4.89 × 10–2) and vital status (p = 1.81 × 10–9), but not with the gender of the patients (p = 4.60 × 10–1) (Figure 4E). This proves that the 11 key genes we found are meaningful for clinical prognosis of glioma. Among the 11 genes, 7 of them (i.e., B3GNT5, BICD1, KIF3A, HAUS1, MTMR3, ITGB3 and EXTL3) have been confirmed to be associated with the prognosis of glioma (Kim et al., 2011; Sumazin et al., 2011; Huang et al., 2017; Zhou et al., 2018; Jeong et al., 2020; Li et al., 2020; Wang et al., 2020). Our findings imply that the functions of these genes in glioma prognosis may be related to the methylation regulation of their AS events.
FIGURE 4. The influence of the glioma-related genes whose AS significantly affected by DNA methylation level on the disease prognosis. (A) The Kaplan-Meier overall survival curves of the low (red) and high (blue) expression groups. (B) and (C) show the results of LASSO regression. There are 11 independent genes with their coefficient when the partial likelihood deviance reaches its minimum value. (D) The ROC curve reveals the reliability of the risk score by comparing the true and false positive rate. (E) The heatmap shows the association between the risk scores of the prognosis-related me-sQTL genes and the clinical features of glioma patients.
In this study, we used the TCGA data to explore the role of the me-sQTL process on pathogenesis of glioma and identify the affected genes and further analyze the influence of them on the clinical prognosis of glioma. In total, we identified 130 such genes which have the following three characteristics: 1) they are significantly differentially expressed between the LGG and GBM subjects; 2) their AS events are significantly regulated by DNA methylation level in the cis regions; and 3) the cis me-sQTLs of them are significantly enriched in glioma risk methylation position dataset. Further, the results of clinical data analysis show a significant association between the expression of these genes and the clinical prognosis of glioma, and among them, 11 (i.e., KIF3A, HAUS1, TMCC1, BEND7, B3GNT5, MTMR3, ITGB3, BICD1, EXTL3, SUN1 and MXRA8) are considered the key risk factors for the prognosis and severity of glioma. At the same time, these 130 genes provide new ideas for the study of the interaction between DNA methylation and alternative splicing in gliomas and similar diseases and provide reference for future research on the study of DNA methylation and variable splicing in neurological diseases in the whole genome. In summary, we performed a strategy to explore the influence of DNA methylation level on gene AS in glioma and these findings will help to better understand pathogenesis of glioma.
The original contributions presented in the study are included in the article/Supplementary Material, further inquiries can be directed to the corresponding author.
ZH and ZY designed the research. ZY, YH, YW, LH, YT, YH, and YC collected the data. ZY and ZH performed the research, analyzed data, and wrote the paper. ZH reviewed and modified the manuscript. All authors discussed the results, and contributed to the final manuscript. All authors read and approved the final manuscript.
This research is financially supported by Start-up fund of Chongqing Medical University (Grant No. R1017) and Science and Technology Research Program of Chongqing Municipal Education Commission (Grant No. KJQN202100402).
The authors declare that the research was conducted in the absence of any commercial or financial relationships that could be construed as a potential conflict of interest.
All claims expressed in this article are solely those of the authors and do not necessarily represent those of their affiliated organizations, or those of the publisher, the editors, and the reviewers. Any product that may be evaluated in this article, or claim that may be made by its manufacturer, is not guaranteed or endorsed by the publisher.
The Supplementary Material for this article can be found online at: https://www.frontiersin.org/articles/10.3389/fgene.2022.799913/full#supplementary-material
Aken, B. L., Achuthan, P., Akanni, W., Amode, M. R., Bernsdorff, F., Bhai, J., et al. (2017). Ensembl 2017. Nucleic Acids Res. 45, D635–D642. doi:10.1093/nar/gkw1104
Aryee, M. J., Jaffe, A. E., Corrada-Bravo, H., Ladd-Acosta, C., Feinberg, A. P., Hansen, K. D., et al. (2014). Minfi: a Flexible and Comprehensive Bioconductor Package for the Analysis of Infinium DNA Methylation Microarrays. Bioinformatics 30, 1363–1369. doi:10.1093/bioinformatics/btu049
Chen, W.-j., Zhang, X., Han, H., Lv, J.-n., Kang, E.-m., Zhang, Y.-l., et al. (2020). The Different Role of YKL-40 in Glioblastoma Is a Function of MGMT Promoter Methylation Status. Cell Death Dis 11, 668. doi:10.1038/s41419-020-02909-9
Chen, Y.-C., and Elnitski, L. (2019). Aberrant DNA Methylation Defines Isoform Usage in Cancer, with Functional Implications. Plos Comput. Biol. 15, e1007095. doi:10.1371/journal.pcbi.1007095
Consortium, G. T. (2020). The GTEx Consortium Atlas of Genetic Regulatory Effects across Human Tissues. Science 369, 1318–1330. doi:10.1126/science.aaz1776
Dong, Z., and Cui, H. (2020). The Emerging Roles of RNA Modifications in Glioblastoma. Cancers 12, 736. doi:10.3390/cancers12030736
Drag, M. H., Kogelman, L. J. A., Maribo, H., Meinert, L., Thomsen, P. D., and Kadarmideen, H. N. (2019). Characterization of eQTLs Associated with Androstenone by RNA Sequencing in Porcine Testis. Physiol. Genomics 51, 488–499. doi:10.1152/physiolgenomics.00125.2018
Erridge, S. C., Hart, M. G., Kerr, G. R., Smith, C., McNamara, S., Grant, R., et al. (2011). Trends in Classification, Referral and Treatment and the Effect on Outcome of Patients with Glioma: a 20 Year Cohort. J. Neurooncol. 104, 789–800. doi:10.1007/s11060-011-0546-0
Etcheverry, A., Aubry, M., de Tayrac, M., Vauleon, E., Boniface, R., Guenot, F., et al. (2010). DNA Methylation in Glioblastoma: Impact on Gene Expression and Clinical Outcome. BMC Genomics 11, 701. doi:10.1186/1471-2164-11-701
Feng, J., Dang, Y., Zhang, W., Zhao, X., Zhang, C., Hou, Z., et al. (2019). PTEN Arginine Methylation by PRMT6 Suppresses PI3K-AKT Signaling and Modulates Pre-mRNA Splicing. Proc. Natl. Acad. Sci. USA 116, 6868–6877. doi:10.1073/pnas.1811028116
Galarza-Muñoz, G., Briggs, F. B. S., Evsyukova, I., Schott-Lerner, G., Kennedy, E. M., Nyanhete, T., et al. (2017). Human Epistatic Interaction Controls IL7R Splicing and Increases Multiple Sclerosis Risk. Cell 169, 72–84. e13. doi:10.1016/j.cell.2017.03.007
Gillies, C. E., Putler, R., Menon, R., Otto, E., Yasutake, K., Nair, V., et al. (2018). An eQTL Landscape of Kidney Tissue in Human Nephrotic Syndrome. Am. J. Hum. Genet. 103, 232–244. doi:10.1016/j.ajhg.2018.07.004
Guo, X., Xu, Y., and Zhao, Z. (2015). In-depth Genomic Data Analyses Revealed Complex Transcriptional and Epigenetic Dysregulations of BRAF V600E in Melanoma. Mol. Cancer 14, 60. doi:10.1186/s12943-015-0328-y
Gutierrez-Arcelus, M., Ongen, H., Lappalainen, T., Montgomery, S. B., Buil, A., Yurovsky, A., et al. (2015). Tissue-specific Effects of Genetic and Epigenetic Variation on Gene Regulation and Splicing. Plos Genet. 11, e1004958. doi:10.1371/journal.pgen.1004958
Ha, K. C. H., Sterne-Weiler, T., Morris, Q., Weatheritt, R. J., and Blencowe, B. J. (2021). Differential Contribution of Transcriptomic Regulatory Layers in the Definition of Neuronal Identity. Nat. Commun. 12, 335. doi:10.1038/s41467-020-20483-8
Han, S., and Lee, Y. (2017). IMAS: Integrative Analysis of Multi-Omics Data for Alternative Splicing, 1.
Han, Z., Xue, W., Tao, L., Lou, Y., Qiu, Y., and Zhu, F. (2020). Genome-wide Identification and Analysis of the eQTL lncRNAs in Multiple Sclerosis Based on RNA-Seq Data. Brief Bioinform 21, 1023–1037. doi:10.1093/bib/bbz036
He, Y., Wang, L., Tang, J., and Han, Z. (2020). Genome-Wide Identification and Analysis of the Methylation of lncRNAs and Prognostic Implications in the Glioma. Front. Oncol. 10, 607047. doi:10.3389/fonc.2020.607047
Hekman, R. M., Hume, A. J., Goel, R. K., Abo, K. M., Huang, J., Blum, B. C., et al. (2021). Actionable Cytopathogenic Host Responses of Human Alveolar Type 2 Cells to SARS-CoV-2. Mol. Cel 81, 212. doi:10.1016/j.molcel.2020.12.028
Hottinger, A. F., Stupp, R., and Homicsko, K. (2014). Standards of Care and Novel Approaches in the Management of Glioblastoma Multiforme. Chin. J. Cancer 33, 32–39. doi:10.5732/cjc.013.10207
Hu, Z., and Qu, S. (2021). EVA1C Is a Potential Prognostic Biomarker and Correlated with Immune Infiltration Levels in WHO Grade II/III Glioma. Front. Immunol. 12, 683572. doi:10.3389/fimmu.2021.683572
Huang, S.-P., Chang, Y.-C., Low, Q. H., Wu, A. T. H., Chen, C.-L., Lin, Y.-F., et al. (2017). BICD1 Expression, as a Potential Biomarker for Prognosis and Predicting Response to Therapy in Patients with Glioblastomas. Oncotarget 8, 113766–113791. doi:10.18632/oncotarget.22667
Hwang, J.-Y., Aromolaran, K. A., and Zukin, R. S. (2017). The Emerging Field of Epigenetics in Neurodegeneration and Neuroprotection. Nat. Rev. Neurosci. 18, 347–361. doi:10.1038/nrn.2017.46
Irimia, M., Weatheritt, R. J., Ellis, J. D., Parikshak, N. N., Gonatopoulos-Pournatzis, T., Babor, M., et al. (2014). A Highly Conserved Program of Neuronal Microexons Is Misregulated in Autistic Brains. Cell 159, 1511–1523. doi:10.1016/j.cell.2014.11.035
Jeong, H. Y., Park, S. Y., Kim, H. J., Moon, S., Lee, S., Lee, S. H., et al. (2020). B3GNT5 Is a Novel Marker Correlated with Stem-like Phenotype and Poor Clinical Outcome in Human Gliomas. CNS Neurosci. Ther. 26, 1147–1154. doi:10.1111/cns.13439
Kim, J.-H., Zheng, L. T., Lee, W.-H., and Suk, K. (2011). Pro-apoptotic Role of Integrin β3 in Glioma Cells. J. Neurochem. 117, 494–503. doi:10.1111/j.1471-4159.2011.07219.x
Kim, M.-S., Pinto, S. M., Getnet, D., Nirujogi, R. S., Manda, S. S., Chaerkady, R., et al. (2014). A Draft Map of the Human Proteome. Nature 509, 575–581. doi:10.1038/nature13302
Li, F., Yi, Y., Miao, Y., Long, W., Long, T., Chen, S., et al. (2019). N6-Methyladenosine Modulates Nonsense-Mediated mRNA Decay in Human Glioblastoma. Cancer Res. 79, 5785–5798. doi:10.1158/0008-5472.CAN-18-2868
Li, S., Wei, Z., Li, G., Zhang, Q., Niu, S., Xu, D., et al. (2020). Silica Perturbs Primary Cilia and Causes Myofibroblast Differentiation during Silicosis by Reduction of the KIF3A-Repressor GLI3 Complex. Theranostics 10, 1719–1732. doi:10.7150/thno.37049
Liu, D., Zhao, L., Wang, Z., Zhou, X., Fan, X., Li, Y., et al. (2019). EWASdb: Epigenome-wide Association Study Database. Nucleic Acids Res. 47, D989–D993. doi:10.1093/nar/gky942
Merkin, J., Russell, C., Chen, P., and Burge, C. B. (2012). Evolutionary Dynamics of Gene and Isoform Regulation in Mammalian Tissues. Science 338, 1593–1599. doi:10.1126/science.1228186
Mogilevsky, M., Shimshon, O., Kumar, S., Mogilevsky, A., Keshet, E., Yavin, E., et al. (2018). Modulation ofMKNK2alternative Splicing by Splice-Switching Oligonucleotides as a Novel Approach for Glioblastoma Treatment. Nucleic Acids Res. 46, 11396–11404. doi:10.1093/nar/gky921
Molinaro, A. M., Taylor, J. W., Wiencke, J. K., and Wrensch, M. R. (2019). Genetic and Molecular Epidemiology of Adult Diffuse Glioma. Nat. Rev. Neurol. 15, 405–417. doi:10.1038/s41582-019-0220-2
Pan, T., Wu, F., Li, L., Wu, S., Zhou, F., Zhang, P., et al. (2021). The Role m6A RNA Methylation Is CNS Development and Glioma Pathogenesis. Mol. Brain 14, 119. doi:10.1186/s13041-021-00831-5
Pangeni, R. P., Zhang, Z., Alvarez, A. A., Wan, X., Sastry, N., Lu, S., et al. (2018). Genome-wide Methylomic and Transcriptomic Analyses Identify Subtype-specific Epigenetic Signatures Commonly Dysregulated in Glioma Stem Cells and Glioblastoma. Epigenetics 13, 432–448. doi:10.1080/15592294.2018.1469892
Pattwell, S. S., Arora, S., Cimino, P. J., Ozawa, T., Szulzewsky, F., Hoellerbauer, P., et al. (2020). A Kinase-Deficient NTRK2 Splice Variant Predominates in Glioma and Amplifies Several Oncogenic Signaling Pathways. Nat. Commun. 11, 2977. doi:10.1038/s41467-020-16786-5
Raudvere, U., Kolberg, L., Kuzmin, I., Arak, T., Adler, P., Peterson, H., et al. (2019). g:Profiler: a Web Server for Functional Enrichment Analysis and Conversions of Gene Lists (2019 Update). Nucleic Acids Res. 47, W191–W198. doi:10.1093/nar/gkz369
Rong, M.-h., Zhu, Z.-h., Guan, Y., Li, M.-w., Zheng, J.-s., Huang, Y.-q., et al. (2020). Identification of Prognostic Splicing Factors and Exploration of Their Potential Regulatory Mechanisms in Pancreatic Adenocarcinoma. PeerJ 8, e8380. doi:10.7717/peerj.8380
Ryan, M., Wong, W. C., Brown, R., Akbani, R., Su, X., Broom, B., et al. (2016). TCGASpliceSeq a Compendium of Alternative mRNA Splicing in Cancer. Nucleic Acids Res. 44, D1018–D1022. doi:10.1093/nar/gkv1288
Shabalin, A. A. (2012). Matrix eQTL: Ultra Fast eQTL Analysis via Large Matrix Operations. Bioinformatics 28, 1353–1358. doi:10.1093/bioinformatics/bts163
Simon, N., Friedman, J., Hastie, T., and Tibshirani, R. (2011). Regularization Paths for Cox's Proportional Hazards Model via Coordinate Descent. J. Stat. Soft. 39, 1–13. doi:10.18637/jss.v039.i05
Sumazin, P., Yang, X., Chiu, H.-S., Chung, W.-J., Iyer, A., Llobet-Navas, D., et al. (2011). An Extensive microRNA-Mediated Network of RNA-RNA Interactions Regulates Established Oncogenic Pathways in Glioblastoma. Cell 147, 370–381. doi:10.1016/j.cell.2011.09.041
Vigneswaran, K., Neill, S., and Hadjipanayis, C. G. (2015). Beyond the World Health Organization Grading of Infiltrating Gliomas: Advances in the Molecular Genetics of Glioma Classification. Ann. Transl Med. 3, 95. doi:10.3978/j.issn.2305-5839.2015.03.57
Wang, B., Mao, J.-h., Wang, B.-y., Wang, L.-x., Wen, H.-y., Xu, L.-j., et al. (2020). Exosomal miR-1910-3p Promotes Proliferation, Metastasis, and Autophagy of Breast Cancer Cells by Targeting MTMR3 and Activating the NF-Κb Signaling Pathway. Cancer Lett. 489, 87–99. doi:10.1016/j.canlet.2020.05.038
Wang, M., Zhou, Z., Zheng, J., Xiao, W., Zhu, J., Zhang, C., et al. (2021). Identification and Validation of a Prognostic Immune-Related Alternative Splicing Events Signature for Glioma. Front. Oncol. 11, 650153. doi:10.3389/fonc.2021.650153
Warzel, D. B., Andonaydis, C., McCurry, B., Chilukuri, R., Ishmukhamedov, S., and Covitz, P. (2003). Common Data Element (CDE) Management and Deployment in Clinical Trials. AMIA Annu. Symp. Proc. 2003, 1048.
Wei, Y., Zhang, Z., Peng, R., Sun, Y., Zhang, L., and Liu, H. (2021). Systematic Identification of Survival-Associated Alternative Splicing Events in Kidney Renal Clear Cell Carcinoma. Comput. Math. Methods Med. 2021, 1–10. doi:10.1155/2021/5576933
Weller, M., Stupp, R., Reifenberger, G., Brandes, A. A., van den Bent, M. J., Wick, W., et al. (2010). MGMT Promoter Methylation in Malignant Gliomas: Ready for Personalized Medicine. Nat. Rev. Neurol. 6, 39–51. doi:10.1038/nrneurol.2009.197
Wiestler, B., Capper, D., Sill, M., Jones, D. T. W., Hovestadt, V., Sturm, D., et al. (2014). Integrated DNA Methylation and Copy-Number Profiling Identify Three Clinically and Biologically Relevant Groups of Anaplastic Glioma. Acta Neuropathol. 128, 561–571. doi:10.1007/s00401-014-1315-x
Xiao, Y., Cui, G., Ren, X., Hao, J., Zhang, Y., Yang, X., et al. (2020). A Novel Four-Gene Signature Associated with Immune Checkpoint for Predicting Prognosis in Lower-Grade Glioma. Front. Oncol. 10, 605737. doi:10.3389/fonc.2020.605737
Xie, Z. c., Wu, H. y., Dang, Y. w., and Chen, G. (2019). Role of Alternative Splicing Signatures in the Prognosis of Glioblastoma. Cancer Med. 8, 7623–7636. doi:10.1002/cam4.2666
Yang, C., Wu, Q., Huang, K., Wang, X., Yu, T., Liao, X., et al. (2019). Genome-Wide Profiling Reveals the Landscape of Prognostic Alternative Splicing Signatures in Pancreatic Ductal Adenocarcinoma. Front. Oncol. 9, 511. doi:10.3389/fonc.2019.00511
Zeng, Y., Zhang, P., Wang, X., Wang, K., Zhou, M., Long, H., et al. (2020). Identification of Prognostic Signatures of Alternative Splicing in Glioma. J. Mol. Neurosci. 70, 1484–1492. doi:10.1007/s12031-020-01581-0
Keywords: glioma, alternative splicing, methylation modification, clinical prognosis, TCGA
Citation: Yang Z, He Y, Wang Y, Huang L, Tang Y, He Y, Chen Y and Han Z (2022) Genome-Wide Analysis for the Regulation of Gene Alternative Splicing by DNA Methylation Level in Glioma and its Prognostic Implications. Front. Genet. 13:799913. doi: 10.3389/fgene.2022.799913
Received: 22 October 2021; Accepted: 20 January 2022;
Published: 04 March 2022.
Edited by:
Liangcai Zhang, Janssen Research and Development (United States), United StatesReviewed by:
Xuefeng Gu, Shanghai University of Medicine and Health Sciences, ChinaCopyright © 2022 Yang, He, Wang, Huang, Tang, He, Chen and Han. This is an open-access article distributed under the terms of the Creative Commons Attribution License (CC BY). The use, distribution or reproduction in other forums is permitted, provided the original author(s) and the copyright owner(s) are credited and that the original publication in this journal is cited, in accordance with accepted academic practice. No use, distribution or reproduction is permitted which does not comply with these terms.
*Correspondence: Zhijie Han, emhpamllaGFuQGNxbXUuZWR1LmNu
Disclaimer: All claims expressed in this article are solely those of the authors and do not necessarily represent those of their affiliated organizations, or those of the publisher, the editors and the reviewers. Any product that may be evaluated in this article or claim that may be made by its manufacturer is not guaranteed or endorsed by the publisher.
Research integrity at Frontiers
Learn more about the work of our research integrity team to safeguard the quality of each article we publish.