- 1Department of Animal Sciences, Purdue University, West Lafayette, IN, United States
- 2Centre for Genetic Improvement of Livestock, Department of Animal Biosciences, University of Guelph, Guelph, ON, Canada
- 3American Angus Association, Angus Genetics Inc., St Joseph, MO, United States
- 4Department of Animal Sciences, Federal University of Vicosa, Viçosa, Brazil
Cattle temperament has been considered by farmers as a key breeding goal due to its relevance for cattlemen’s safety, animal welfare, resilience, and longevity and its association with many economically important traits (e.g., production and meat quality). The definition of proper statistical models, accurate variance component estimates, and knowledge on the genetic background of the indicator trait evaluated are of great importance for accurately predicting the genetic merit of breeding animals. Therefore, 266,029 American Angus cattle with yearling temperament records (1–6 score) were used to evaluate statistical models and estimate variance components; investigate the association of sex and farm management with temperament; assess the weighted correlation of estimated breeding values for temperament and productive, reproductive efficiency and resilience traits; and perform a weighted single-step genome-wide association analysis using 69,559 animals genotyped for 54,609 single-nucleotide polymorphisms. Sex and extrinsic factors were significantly associated with temperament, including conception type, age of dam, birth season, and additional animal–human interactions. Similar results were observed among models including only the direct additive genetic effect and when adding other maternal effects. Estimated heritability of temperament was equal to 0.39 on the liability scale. Favorable genetic correlations were observed between temperament and other relevant traits, including growth, feed efficiency, meat quality, and reproductive traits. The highest approximated genetic correlations were observed between temperament and growth traits (weaning weight, 0.28; yearling weight, 0.28). Altogether, we identified 11 genomic regions, located across nine chromosomes including BTAX, explaining 3.33% of the total additive genetic variance. The candidate genes identified were enriched in pathways related to vision, which could be associated with reception of stimulus and/or cognitive abilities. This study encompasses large and diverse phenotypic, genomic, and pedigree datasets of US Angus cattle. Yearling temperament is a highly heritable and polygenic trait that can be improved through genetic selection. Direct selection for temperament is not expected to result in unfavorable responses on other relevant traits due to the favorable or low genetic correlations observed. In summary, this study contributes to a better understanding of the impact of maternal effects, extrinsic factors, and various genomic regions associated with yearling temperament in North American Angus cattle.
Introduction
Genetic evaluation schemes have been refined over time concomitantly with consumers, producers, and industry requirements. A recent American Angus Association (AAA) producers’ survey revealed docility (or temperament) as one of the top three traits to be prioritized in US Angus cattle breeding programs (Santos et al., 2019). Behavioral traits are important due to their impact on animal performance [e.g., growth (Hoppe et al., 2010)], meat quality (King et al., 2006; Coutinho et al., 2017; Sant’Anna et al., 2019), reproduction (Cooke et al., 2011; Valente et al., 2015; Cziszter et al., 2016), and immunity (Burdick et al., 2011). Other substantial influences include handler safety, animal welfare, longevity (Neja et al., 2015), efficiency of management systems, and economic profit (Carlstrom et al., 2014). For instance, Busby (2015) has reported an average profit of $46.63 per docile animal compared to $7.62 per animal with aggressive behavior. Temperament is one of a plethora of behavioral measurements, and it is defined as “the animal response to handling or forced movement by humans” (Burrow et al., 1988). Temperament can be assessed in multiple ways, including objective measurements [e.g., flight time (Burrow et al., 1988)] and subjective evaluations (Tulloh, 1961; Dickson et al., 1970; Kilgour, 1975).
A wide range of heritability estimates [average equal to 0.27, ranging from 0.03 to 0.67 (Sato, 1981; Hearnshaw and Morris, 1984; Hoppe et al., 2010; Rolfe et al., 2011)] has been reported for beef cattle temperament traits, supporting the idea that cattle temperament can be improved through genetic or genomic selection. Studies underlying the genetic background of temperament have also been performed (Esmailizadeh et al., 2008; Chan, 2012; Hanna et al., 2014; Riley et al., 2016; Garza-Brenner et al., 2017; Macleod et al., 2019; Costilla et al., 2020). In total, 29 quantitative trait loci (QTL) have been reported, in which 19 were identified for temperament in beef or dual-purpose cattle (Esmailizadeh et al., 2008; Glenske et al., 2011; Riley et al., 2016; Dos Santos et al., 2017; Garza-Brenner et al., 2017; Hu et al., 2019). Additionally, orthologous genes have been reported to be controlling behavioral indicator traits across various species, including humans (Costilla et al., 2020; Alvarenga et al., 2021). However, to the best of our knowledge, none of the mentioned studies have used large datasets [the maximum number of records in previous studies was 9,223 individuals (Costilla et al., 2020)] recorded in a broad array of geographic, environmental, and climatic zones, nor genetically evaluated temperament in the US Angus population, which is the most predominant breed in the largest producer of beef cattle in the world (Greenwood, 2021). Along with the breeding goals, environmental conditions, management practices, genetic population parameters (Hidalgo et al., 2020), and trait definitions change over time. Herein, routine updates of the statistical models and re-estimation of variance components are required, as the accuracy of estimated breeding values (EBVs) relies on properly defined genetic models and accurate genetic parameter estimates.
Therefore, our overarching goal was to better understand the variation of temperament at the yearling age across the US using a large dataset provided by the AAA through the Angus Genetics Inc. (AGI—American Angus Association; Saint Joseph, MO, US), from the non-genetic, genetic, and genomic aspects. The understanding of the non-genetic factors impacting temperament can guide the statistical modeling and provide a better understanding of nurture impacting temperament. Thereafter, up-to-date statistical models and variance components will allow more accurate EBV estimates and a more powerful genome-wide association to understand the genetic background and the biological mechanisms associated with cattle temperament. With that, the specific objectives of this study were to 1) identify the non-genetic effects (e.g., management events) influencing yearling temperament; 2) define an optimal statistical model (in terms of systematic and random effects) to be used in routine genetic and genomic evaluations of yearling temperament in North American Angus cattle; 3) estimate variance components for yearling temperament; 4) estimate approximated genetic correlations between yearling temperament and 20 productive efficiency and resilience traits, including calving ease, growth traits, carcass measurements, body conformation, susceptibility to high altitude disease, hair shedding score, and other mature cow measurements; and 5) identify genomic regions and candidate genes controlling yearling temperament in Angus cattle based on weighted single-step genome-wide association studies, followed by metabolic pathway (functional enrichment) analyses and a comparison with previously reported QTLs.
Materials and Methods
Data
All the data used in this study are from purebred and commercial Angus cattle registered in the AAA, born between 1990 and 2018, and recorded for temperament score at yearling age. Yearling temperament score is a subjective measurement (i.e., visually evaluated by the farmers/handlers), and it is recorded from 320 to 440 days of age (yearling) when the animal exits a chute, which is consistent within contemporary groups (Northcutt and Bowman, 2007). Temperament is scored on a one-to-six scale, in which a score of 1 represents a docile animal (i.e., desirable behavior) and a score of 6 represents a very aggressive (more temperamental) animal. A complete description of the scores and measurement guidelines used by the AAA are shown in Table 1.
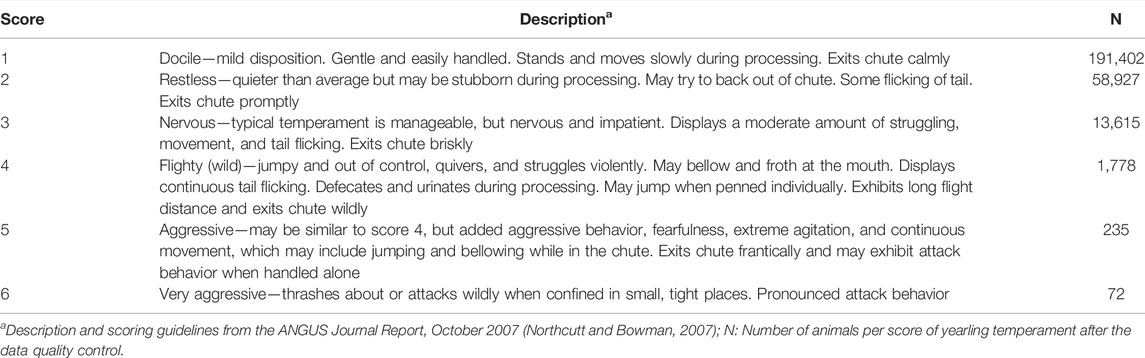
TABLE 1. Descriptiona of yearling temperament scores and number of animals per level in American Angus cattle.
Records from 675,678 Angus cattle with temperament score at yearling age were available. Few animals had scores of 4 (1,778 animals), 5 (425 animals), or 6 (151 animals). Therefore, these three categories were combined, which resulted in 83.8% of animals with score 1, 12.9% animals with score 2, 2.8% animals with score 3, and 0.5% animals with score 4+ (representing the scores 4, 5, and 6). A quality control procedure was performed to remove animals with missing information for the systematic effects evaluated and to warranty enough variability within their levels (i.e., at least three animals per level). Thereafter, the herds (where animals were located at birth, weaning, and yearling) exclusively represented by one score (e.g., all animals with score 1) were removed from the dataset. This criterion was used to avoid bias generated by the farmer/evaluator and allow variability within the levels. Additionally, data from animals younger than 320 days and older than 440 days at the time of temperament assessment were also removed. After quality control, there were 266,029 animals comprising 147,671 bulls, 3,332 steers, and 115,026 females with yearling temperament scores 1 (71.9%), 2 (22.2%), 3 (5.1%), or 4+ (0.8%). These remaining animals were born between 2001 and 2018. The number of animals per yearling temperament score after the quality control is shown in Table 1.
Statistical Model Definition and Genetic Parameter Estimation
Before consideration of the statistical model to be used, several options were tested for environmental (systematic) and genetic (direct and maternal genetic) effects. The statistical models used in this study were defined based on two steps: 1) definition of the systematic effects and contemporary group (CG) and 2) definition of direct genetic, maternal genetic, and/or maternal environmental effects. Concomitantly to the second step, the genetic parameters were estimated.
The first step was performed using the glm function available in R software (R Core Team, 2019), considering a quasi-binomial family distribution. Stepwise (i.e., backward elimination) subroutines for selecting the best subset of covariates were used for the systematic model definition and to define the CG. The best fitted non-genetic model for yearling temperament rendered a coefficient of determination equal to 0.35. The model included age of dam (AOD; 10 levels), CG (22,322 levels), and conception type (ET; two levels: embryo transference or natural conception) as categorical systematic effects and calf age deviation from 365 days (CALFDEV) as a linear covariate. AOD was classified following the Beef Improvement Federation Guidelines [BIF (Cundiff et al., 2018)]. CG was defined as the concatenation of birth date (month-year), birth herd, weaning date (month-year), weaning herd, if the animal was submitted to a creep feeding system, if the animal had ultrasound information, and date (month-year), herd, sex, and group age deviation when yearling temperament was recorded.
After defining the systematic model and the optimal CG, four mixed animal models were tested by incorporating four random effects: CG, additive direct genetic, maternal genetic, and/or maternal environmental effects. The four models and their corresponding components are listed in Table 2.
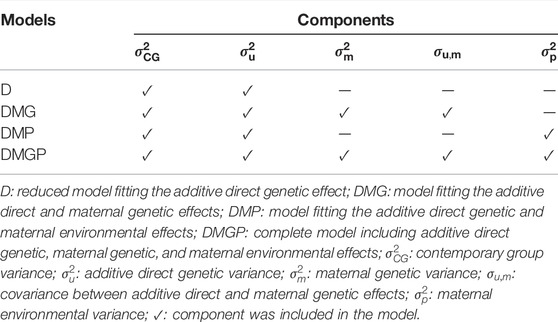
TABLE 2. Random effects included in the four animal models for yearling temperament in American Angus cattle.
Bayesian threshold models based on the Gibbs sampler and Markov Chain Monte Carlo (MCMC) algorithm were used to estimate the genetic parameters and, thereafter, compare the models. thrgibbs1f90 software (Tsuruta and Misztal, 2006) was used with a chain length varying from 500,000 to 1,000,000 iterations, assuming a burn-in from 50% to 75%, and a thin of 50 or 100. Non-informative prior information based on the inverse-chi-squared distribution was assumed for variance components. The models are presented below. The reduced model is defined as follows:
where
where
where
where all the components of the DMGP model were previously defined in Eqs 1–3. The systematic effects were the same for all four models.
A total of 819,303 animals (242,570 bulls, 298 steers, and 576,435 cows) born between 1928 and 2018 were included in the
where
The models were compared based on the linear regression method (Legarra and Reverter, 2018), which estimates bias, dispersion, and accuracies comparing two subsets of breeding values (EBVs) estimated using a partial and whole dataset. The whole dataset comprises all animals with temperament records (i.e., 266,029). The partial dataset was defined as animals in the whole dataset but masking the phenotype of the animals born in 2018 (13,202 animals), which was defined as the validation group. The EBVs from the partial and whole datasets were obtained fixing the variance components in thrgibbs1f90 with 10,000 iterations, 1,000 burn-in, and 5 thin. Bias, dispersion, and predictive accuracies were calculated for the validation animals.
Effect of Sex and Extrinsic Variables on Yearling Temperament
Sex and extrinsic factors available in the AAA dataset were submitted to a pairwise comparison using the lsmeans package (Lenth, 2016) implemented in R software (R Core Team, 2019). A linear model including calf age deviation from 365 days as a linear covariate and the following target independent variables as a categorical fixed effect, such as age of dam, conception type (embryo transfer or natural conception), parity type (single or twin), sex, birth season, if the animal was under a creep-feeding system, and if the animal had ultrasound and feed intake information, was used. In other words, the mean presented for each level of the fixed effect was adjusted for the remaining factors.
Approximated Genetic Correlations
The genetic relationship between yearling temperament and other productive efficiency and resilience traits is of great importance for the design of breeding programs and development of selection indexes. Due to limited access to the raw datasets and computational limitations, the genetic correlation between yearling temperament and 20 other relevant traits (Table 3) was assessed based on the correlation between the EBVs. The EBVs for yearling temperament were from the Statistical Model Definition and Genetic Parameter Estimation while fixing the variance components in thrgibbs1f90. The EBVs for the other traits were from the official genetic evaluation performed by the AAA, which includes the following: calving ease [calving ease direct (CED) and maternal calving ease (CEM)], body weight [birth weight (BW), weaning weight (WW), and yearling weight (YW)], residual average daily gain (RADG), dry-matter intake (DMI), yearling height (YH), scrotal circumference (SC), carcass and meat quality measurements [carcass weight (CW), marbling score (MARB), ribeye area (REA), and fat thickness (FAT)], conformation [foot angle (FOOT)], adaptation [high altitude disease susceptibility—pulmonary artery pressure (PAP) and hair shedding score (HS)], and other mature cow measurements [fertility—heifer pregnancy (HP), mature weight (MW), mature height (MH), and maternal milk (MILK)]. A complete description of the traits is shown in Table 3. Data from animals with EBV accuracies lower than 0.25 for both evaluated traits were disregarded for the correlation. A weighted Pearson correlation (
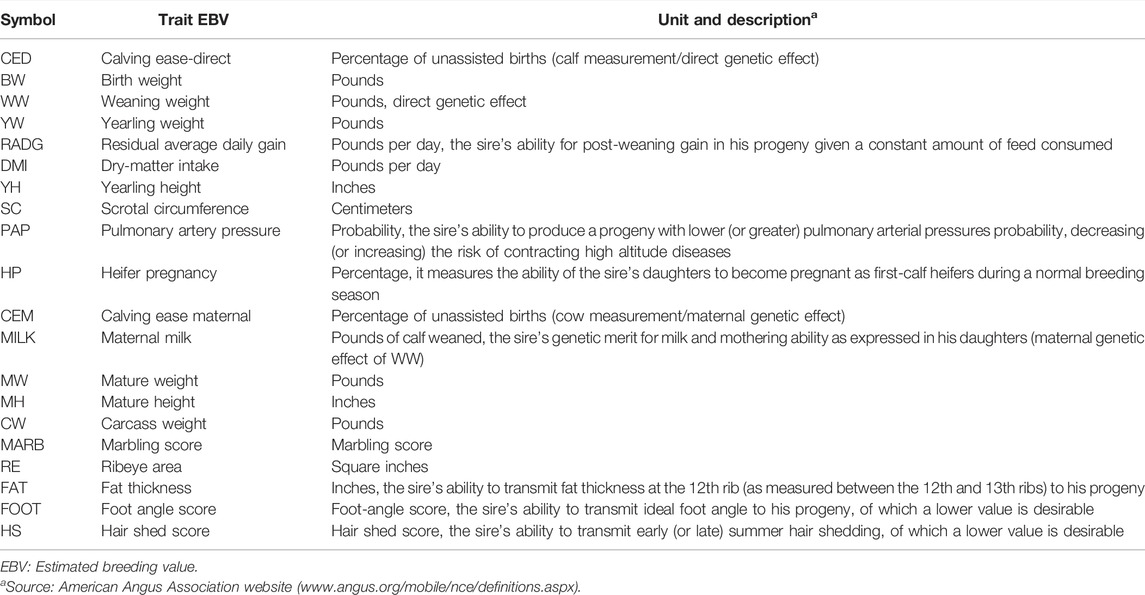
TABLE 3. Description of production traits evaluated to be genetically correlated with yearling temperament score, as defined by the American Angus Association.
1st. Calculation of the weights (
where
2nd. Calculation of the weighted mean for traits 1 and 2:
where
3rd. Calculation of the weighted variances:
where
4th. Calculation of the weighted covariance:
where
5th. Calculation of weighted correlation:
where
Finally, the standard errors (± SE) of the genetic correlation metric (i.e.,
where all the parameters have been described. The 95% confidence intervals were obtained as mean
Weighted Single-Step Genome-Wide Association Analyses
A weighted single-step genome-wide association study [WssGWAS (Wang et al., 2012)] was performed to identify candidate regions controlling yearling temperament. From the 266,029 animals with phenotypic information for yearling temperament, 69,559 were genotyped. Animals were genotyped with various SNP arrays as part of ongoing commercial genotyping activities by breeders for genetic evaluation purposes, resulting in a market set of 54,609 SNPs. Commercial genotyping products were from Zoetis, including i50K (www.zoetisus.com/animal-genetics/media/documents/i50k-00001_50k-sellsheet.pdf) and HD50K (www.zoetisus.com/animal-genetics/beef/hd-50k/hd-50k-for-black-angus.aspx), and Neogen GeneSeek, including GeneSeek Genomic Profile Low-Density (GGPLD; 40 K SNPs), High-Density (GGPHD; 80 K SNPs), GGPUHD (150 K SNPs), and AngusGS. Both Zoetis and Neogen provided, for genomic evaluation purposes, an imputed (average and standard deviation imputation accuracy equal to 99.72% and 0.87) SNP set similar to the Illumina BovineSNP50V2 and Illumina BovineSNP50V3 (Illumina, Inc., San Diego, CA), respectively, and mapped to the bovine genome assembly UMD3.1. However, the genomic coordinates were converted to ARS-UCD1.2 bovine genome assembly (Medrano, 2017; Rosen et al., 2018) using the biomaRt R package (Durinck et al., 2009).
Quality control (QC) procedures were applied to remove genotyped individuals with a call rate lower than 90% and pedigree errors. SNP genotypes with a call rate lower than 90%, an MAF lower than 0.01, and a deviance of heterozygous genotype from the Hardy Weinberg Equilibrium higher than 0.15 were also removed. Both autosomal chromosomes and pseudo-autosomal regions of the X chromosome were used in the WssGWAS. SNPs located on approximated pseudo-autosomal regions [PAR; SNP with a genomic coordinate above X:133,300,518 bp; 109 SNPs; (Johnson et al., 2019)] represented the X chromosome because it follows an autosomal-chromosome inherence and, therefore, were analyzed as autosomal SNPs (Johnson et al., 2019). preGSf90 software (Aguilar et al., 2014) was used to perform the QC, in which 69,441 genotyped animals and 42,662 SNPs remained for further analyses. As a WssGWAS was performed, the pedigree-based relationship matrix (
where
where R is a matrix of gene content adjusted for observed allele frequencies and D is a diagonal matrix of SNP weights. The weights were derived from the third iteration’s SNP solutions using a nonlinear approach described by VanRaden (2008) and Cole et al. (2009).
The optimal or selected statistical model was used to perform the WssGWAS. The vector of direct genetic effects was assumed to follow a normal distribution,
The genomic coordinates were based on the ARS-UCD1.2 bovine genome assembly (Medrano, 2017; Rosen et al., 2018). Quantitative trait loci (QTL) curated in the Cattle QTL DataBase [Cattle QTLdb (Hu et al., 2019); www.animalgenome.org; access date: September 27, 2021] and located within the selected genomic windows were identified. Gene annotation information was retrieved from Ensembl using the biomaRt R package (Durinck et al., 2009). Functional annotation was performed in terms of Gene Ontology (GO) biological processes [GO_BP; (Blake et al., 2013)] and metabolic pathways of the Kyoto Encyclopedia of Genes and Genomes [KEGG; (Kanehisa and Goto, 2000)] available in the DAVID database [david.ncifcrf.gov/tools.jsp; (Dennis et al., 2003); access date: September 27, 2021].
Results
Sex and Extrinsic Variables Affecting Yearling Temperament
Yearling temperament was significantly associated with sex and extrinsic factors, which includes reproductive conception type, age of dam, birth season, creep-fed or non–creep-fed animals, and if there is ultrasound and/or feed intake recording (Supplementary Table S1). Parity type (i.e., single or twin) was the only factor not significantly associated with temperament.
Embryo-transferred animals were statistically more docile (average equal to 1.36) than naturally conceived animals (average equal to 1.37; Supplementary Table S1; Figure 1A). Small differences in the averages are probably due to a skewed distribution toward docile scores (Table 1). The age of dam categorized by years (from three to 12 years of age) also influenced yearling temperament (Figure 1B; Table 2; Supplementary Table S1). Dams up to 4 years old raised more docile progenies (average equal to 1.34) than older dams (average equal to 1.41).
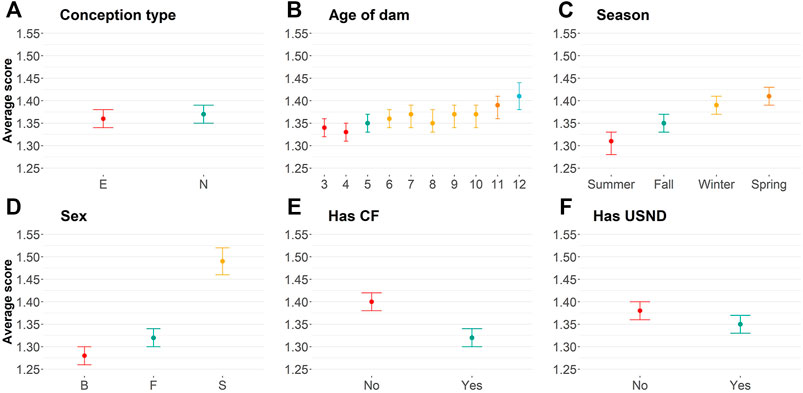
FIGURE 1. Average of temperament score using least-square means for (A) conception type [naturally conceived (N) or embryo transference (E)]; (B) Age of the dam in years; (C): birth season; (D): sex [bull (B), steers (S), and female (F)]; (E) if the animal was creep-fed; and (F): if the animal had ultrasound information.
Birth season was statistically associated with yearling temperament score (p
Our phenotypic analyses have also shown a positive relationship between implementation of the creep feeding system and animal temperament (Supplementary Table S1; Figure 1E). In short, creep-fed calves tend to be more docile (average of 1.32) than non–creep-fed calves (average of 1.40). Finally, animals that, in general, had additional recorded phenotypic collections were more docile (p
Model Choice and Parameter Estimation
We tested four animal models, which included direct genetic and maternal effects (Table 2). The results of the model incorporating both maternal effects (DMGP model including direct genetic, maternal genetic, and maternal environmental effects) are not presented because it did not converge even using up to 1 million iterations. The fitness measures of the models and genetic parameters are shown in Table 4. The DMG model, including direct and maternal genetic effect, provided a slight lower residual variance (
Total yearling temperament heritability estimates ranged from 0.38 (DMP model) to 0.39 (D model) on the liability scale. Maternal genetic effects contributed to 4% of the total variation in yearling temperament on the liability scale (Table 4). Additionally, a strong negative genetic correlation was observed between direct and maternal genetic effects (−0.40; Table 4).
Approximated Genetic Correlation Between Yearling Temperament and Other Relevant Traits
Weighted correlations between the EBVs of yearling temperament and other key traits is shown in Figure 2. Additional correlations between all pairs of traits are provided for comparison. Other metrics, including the number of animals, and descriptive statistics (average, minimum, and maximum) of EBVs’ theoretical accuracy are shown in Supplementary Table S3. Low-to-moderate genetic correlation was observed between temperament and all other relevant traits, which varied from −0.05 (yearling temperament vs. hair shedding) to 0.28 (yearling temperament vs. weaning or yearling weight). Other approximated genetic correlation between pairs of traits was similar to the genetic correlation estimated using multiple-trait models used by the AAA (please see www.angus.org/Nce/Heritabilities).
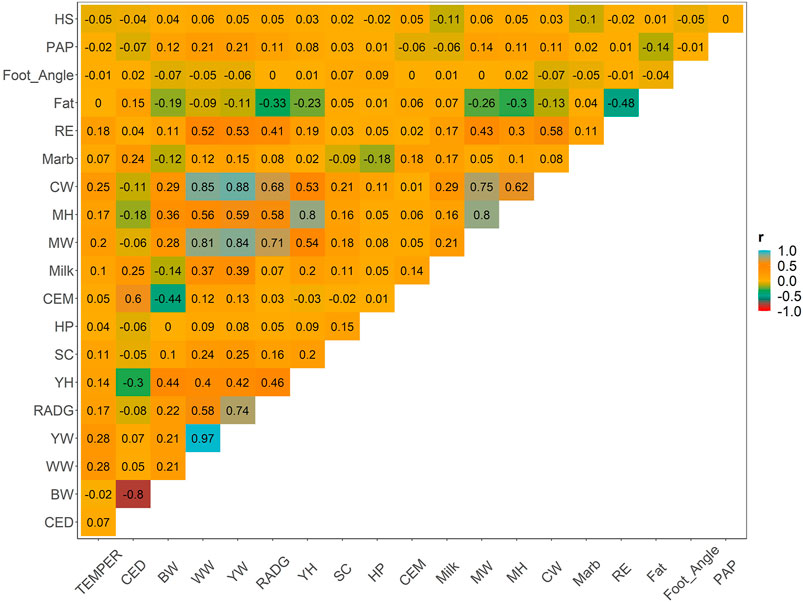
FIGURE 2. Weighted Pearson correlation among all estimated breeding values for relevant traits in the beef cattle industry.
Positive and favorable association between yearling temperament and growth traits (i.e., direct weaning, yearling, and mature weight; correlation from 0.20 to 0.28), feed efficiency (i.e., RADG; 0.17), precocity (i.e., scrotal score; 0.11), and carcass traits (i.e., carcass weight, ribeye by area; varying from 0.18 to 0.25) were observed. The remaining traits had low genetic correlations, varying from −0.05 (i.e., hair shedding) to 0.10 (i.e., maternal weaning weight).
Weighted Single-Step Genome-Wide Association Analyses
The GEBVs from the D model were back-solved to generate the SNP window effects and the percentage of the total additive genetic variance explained by them. The WssGWAS was performed using 266,029 animals with phenotype, 69,441 animals with genotype for 42,662 SNPs located on autosomal and X chromosomes, and a pedigree containing 578,821 animals. The distribution of MAF is presented in Supplementary Figure S1, which was equally distributed across MAF intervals of 0.05.
Eleven independent genomic regions (i.e., non-overlapping regions of five SNPs) were identified, in which each genomic region explained more than 0.20% of the total additive genetic variance. All genomic regions together explained 3.33% of the total additive genetic variance for temperament. Relevant genomic regions were located on BTA2, BTA4, BTA8, BTA10, BTA11, BTA14, BTA26, BTA29, and X chromosomes (Supplementary Table S4). The Manhattan plot of the additive genetic variance explained by genomic windows for yearling temperament is shown in Figure 3.
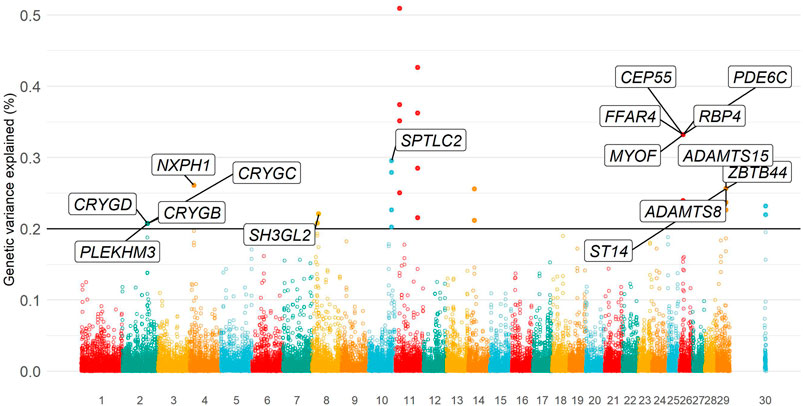
FIGURE 3. Manhattan plot of additive genetic variance explained by genomic windows for yearling temperament using the D model.
Twenty-five positional candidate genes were identified surrounding the 11 genomic windows, in which 18 genes were annotated and their biotype was classified as protein coding (17 genes) and noncoding RNA genes (one gene; Table 5; Supplementary Table S4). The two regions with the largest variance explained (i.e., 0.50%) are located on BTA11, and no annotated gene has been observed in that region. However, one of the regions had one gene categorized as long noncoding RNA (lncRNA; Supplementary Table S4). The richest genomic regions for identified genes were BTA2, BTA26, and BTA29. The main genes annotated in these regions are PLEKHM3, CRYGD, CRYGC, CRYGB, RBP4, MYOF, CEP55, FFAR4, PDE6C, ST14, ZBTB44, ADAMTS8, and ADAMTS15.
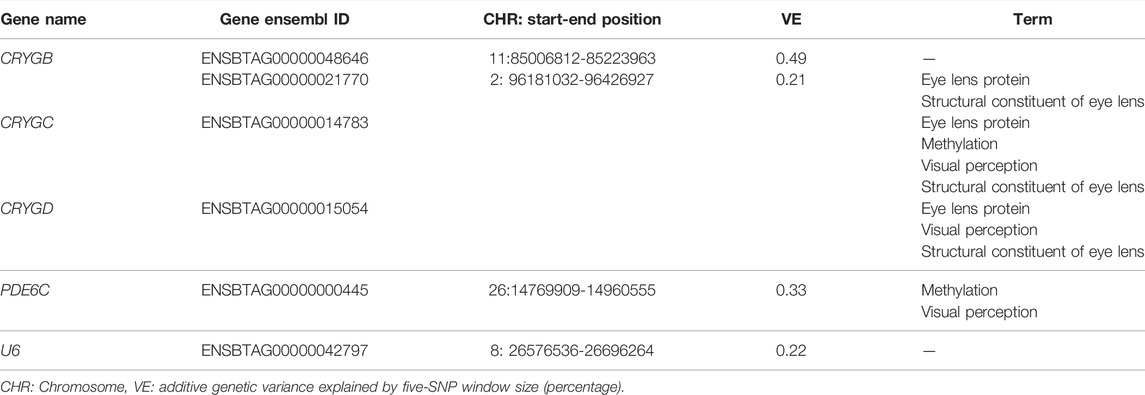
TABLE 5. Sample of the top genomic regions, the genes, and biological processes involved in these regions.
There were 210 QTLs annotated in an overlap with the genomic windows associated with temperament. Figure 4 shows the distribution of trait-type that these QTLs were previously associated with. In total, 72% of QTLs were previously associated with milk content, followed by 8% with structural problems, 6% with reproduction and production, and 4% with meat and carcass traits. Two QTLs for milking speed are located on a BTA14 genomic region explaining 0.26% of the total genetic variation. One gene overlapped in this region: TOX gene (thymocyte selection associated high mobility group box). In addition, two overlapped QTLs were annotated for longevity on BTA2, where four genes were identified, including three from the crystalline gamma family (i.e., CRYGB, CRYGC, and CRYGD) and PLEKHM3 (pleckstrin homology domain containing M3).
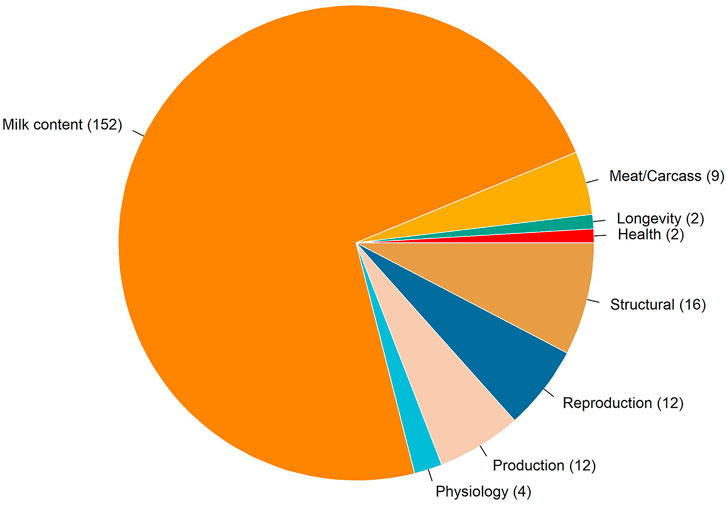
FIGURE 4. Chart of the trait-types associated with the quantitative trait loci overlapping with the genomic regions associated with temperament.
To better understand the functions of the genes identified, we performed a functional analysis in DAVID, in which one GO biological process, one GO molecular function, and one term were significantly enriched based on the Benjamini test (p < 0.10). All terms were associated with vision processes. Visual perception (GO:0007601) was enriched by three genes located on BTA2, including PDE6C (phosphodiesterase 6C), CRYGD, and CRYGC. The last two genes from the CRYG family were also enriched in the structural constituent of eye lens (GO:0005212) and eye lens protein terms, in addition to a third CRYG family gene: CRYGB (Supplementary Table S5). Finally, another interesting process that those genes also participate in is methylation (Supplementary Table S5). In Table 5 is presented the genes enriched in the GO terms, as well as the genes located in the top regions.
Discussion
Sex and Extrinsic Variables Affecting Yearling Temperament
Pointing out extrinsic factors influencing yearling temperament may inform future management studies and, hence, improve management strategies implemented in herds as well as handlers’ safety. The elucidation of levels within the extrinsic factors intermeddling behavioral responses is also paramount to define and fit appropriate effects in the models, which can directly impact the prediction of EBVs and marker effects. Therefore, we have reported the connectivity between yearling temperament and farm experiences that the animal was subjected to, for which we used one of the largest beef cattle datasets available worldwide.
The statistical differences observed for embryo-transferred animals are potentially due to the genetic superiority of donors for many economically important traits, including temperament. An alternative hypothesis is associated with maternal ability and behavior during rearing. Particularly, foster/recipient cows could be (directly or indirectly) selected based on their maternal ability and easy-to-handle characteristics (Busby, 2015) in order to rear the biological progeny from high–genetic merit animals, and therefore, the behavior of these foster calves could be influenced by their foster mother characteristics.
Still, at the maternal level, younger dams raised more docile progenies than older dams. Similar trends were observed in Nellore cattle, where the average of temperament score tended to increase (i.e., being more aggressive) as the dam gets older (Barrozo et al., 2012). Studies have reported the high cognitive abilities of cattle, in which animals tend to avoid certain environments based on previous negative experiences (Ede et al., 2019). Thereupon, calves could reflect upon the behavior of their dams, in which an older cow, through memory acquisition, can become more aggressive or fearful across the years due to previous negative experiences (Kasimanickam et al., 2018).
In general, bulls have more dominant and aggressive behaviors than females (Reinhardt et al., 1987). Controversially, in recent studies, female beef cattle had been classified as more temperamental (Gauly et al., 2001). In this study, female Angus were on average more temperamental than male Angus (p < 0.05).
The birth-seasonal animal temperament pattern hinges on climate, grazing conditions, production system, and, consequently, on the farm location (McBride and Mathews, 2011; USDA–APHIS, United States Department of Agriculture-Animal and Plant Health Inspection Service, 2017). For instance, a study conducted by USDA–APHIS, United States Department of Agriculture-Animal and Plant Health Inspection Service, 2017 reported higher percentages of calves born during the spring in the Central US (78.4% of the animals) and West (64.0% of the animals) regions compared to calves born in the East region (43.0%). Additionally, the same study reported that over one-fourth of calves (27.9%) were born during the fall in the East region of the US, while only 15.3% and 7.8% were born in the West and Central regions, respectively (USDA–APHIS, United States Department of Agriculture-Animal and Plant Health Inspection Service, 2017). In the same report, a small proportion of cows calved during the summer [2.7% (USDA–APHIS, United States Department of Agriculture-Animal and Plant Health Inspection Service, 2017)]. Ultimately, the pattern of calves born per season, as presented by the USDA–APHIS, United States Department of Agriculture-Animal and Plant Health Inspection Service, 2017, is in agreement with our findings (10% summer, 18% fall, 30% spring, and 42% winter; Supplementary Table S1). Therefore, the season might reflect the region where most calves were born. In other words, animals born during the fall, winter, and spring mainly represent animals from the East, North, and Central-West regions, respectively. Another theory has to do with the size of the herd and/or contemporary group. Animals born during low season probably have few lot-mates and, as a consequence, lower probability of stressful social interactions.
Animal behavior has been associated with memory ability, in which positive and frequent human–animal interaction could positively impact future behavioral responses (Boivin et al., 2009). The creep feeding system and additional phenotypic measurement (e.g., ultrasound information) are generally associated with additional interaction with humans (Broadhead et al., 2018). A temperament study in Angus cows in Bulgaria reported that more docile cows have frequent contact with people (Karamfilov, 2022). Our phenotypic analyses showed a positive relationship between the use of creep feeding and if the animal had additional measurements (i.e., ultrasound and feed intake). On the other hand, an experimental study reported that despite efforts to give positive animal–human experiences during the handling, cattle would still be averse to the handling process, and its fear would increase (Petherick et al., 2002).
These findings provide a framework for understanding how sex and extrinsic factors influence temperament at the phenotypic level. Furthermore, it can provide insights to improve farm management to guide future epigenetic experimental studies. Environmental conditions regulating gene expression are well known for many traits, including docility (Cantrell et al., 2019). Hence, the listed environmental factors affecting yearling temperament can also be indicative of a programmer of epigenetics modifications.
Model Choice and Parameter Estimation
The ultimate goal of model definition is to facilitate genetic improvement through more accurate estimation of variance components and breeding values (Bijma, 2006). Therefore, four animal models were tested in this study, including direct genetic and maternal effects (Tables 2, 4). Genetic parameters for the DMGP model, which includes direct genetic, maternal genetic, and maternal environmental effects, did not converge, which might be due to inherently problematic estimation of maternal effects (Meyer, 1994). The small average number of progenies per dam and grand-dam in our dataset represents an additional challenge for the estimation of maternal effects [i.e., average of 1.6 ± 1.82 progenies per dams (484,322 dams in the pedigree), 2.2 ± 4.9 grandchildren per grand-dam (total of 338,628 grand-dams in the pedigree), and 2.9 ± 10.2 great-grandchildren per great-grand-dam (total of 250,119 great-grand-dams in the pedigree)].
Among the converged models, the DMG model (i.e., including direct and maternal genetic effects) provided a slightly lower residual variance, suggesting the components are better capturing the total variance. Beckman et al. (2007) have also observed a positive effect of maternal effects (i.e., genetic and environment) on temperament in Limousin cattle. On the other hand, the other models presented slightly desirable dispersion and accuracy. Nevertheless, small differences were observed among the models considering the genetic parameters and linear regression metrics for the best fit model. Thereupon, in routine genetic evaluations, we suggest the use of the D model due to its computational efficiency (time of analysis required), and it was as suitable as the other models. However, as mentioned before, the current data structure might be suboptimal for maternal effect estimation. Therefore, when more data are available, the re-estimation of maternal effects for docility is recommended.
Medium-to-high heritability are in close agreement with previous heritability estimates for cattle temperament using pedigree (Burrow, 2001; Beckman et al., 2007; Haskell et al., 2014) or genomic information (Costilla et al., 2020). Therefore, yearling temperament measured on a 1–6 scale presents sufficient variability to respond to genetic selection, even though a small genetic improvement has been observed across the years in Angus cattle (Supplementary Figure S3). Finally, the maternal genetic effects contributed to 4% of the total variation in yearling temperament on the liability scale. Previous studies have also reported low maternal genetic contribution on temperament variation (Burrow, 2001; Beckman et al., 2007). Additionally, a strong negative genetic correlation was observed between additive direct and maternal genetic effects (−0.40 ± 0.039; Table 4), which can be explained by the environmental covariances between dam and offspring records and on the fixed effects’ structure used in the statistical models for the data analyses (Bijma, 2006). Additionally, the effect of sire-by-herd interaction was not included, which could potentially adjust the negative correlation (Willham, 2000). Negative genetic correlation estimates between direct and maternal additive genetic effects for other traits were also reported in the literature (Dodenhoff et al., 1999; Burrow, 2001; Bijma, 2006; Beckman et al., 2007). However, Willham (2000) stated that there is a possibility of negative correlation between maternal and direct genetic components. The aggressiveness of the dams during the nursery event could be associated with higher protectiveness of the progeny and not necessarily with overall temperament of the cow.
Approximated Genetic Correlation Between Yearling Temperament and Economically Relevant and Indicator Traits
In general, Pearson correlation between EBVs (or EPDs) does not properly represent the genetic correlation, especially between lowly accurate values (Calo et al., 1973). Therefore, Calo et al. (1973) proposed an approach to adjust for the EBV accuracies, which has been extensively used in dairy cattle studies (Dikmen et al., 2012; Costa et al., 2020; Dechow et al., 2020). Traditionally, dairy cattle have higher EBV accuracies than beef cattle populations. Thereof, the method used by Calo et al. (1973) might penalize Pearson correlations when correlated traits have low accuracies. For this study, we calculated a correlation of EBVs weighted for the accuracies.
Pairwise genetic correlation among all other relevant traits had been performed, and similar direction and scale were observed between genetic correlation using the weighted Pearson correlation and multi-trait animal model, considering the same population (www.angus.org/Nce/Heritabilities). For completeness, we have presented the approximated genetic correlations between all pairs of traits. However, only associations between yearling temperament and the other traits are discussed in this article.
Positive and favorable genetic correlation was observed between temperament and growth, feed efficiency, precocity, and carcass traits. Similar results were observed in cattle. For instance, temperament was genetically associated with higher average daily gain, better conformation scores, finishing precocity, and muscling (Burrow, 2001; King et al., 2006; Phocas et al., 2006; Hoppe et al., 2010; Sant’Anna et al., 2012, 2019; Cooke, 2014; Coutinho et al., 2017). Nervous cattle tend to allocate more energy into the state of excitement instead of using it for other physiological functions such as growth and reproduction (Petherick et al., 2002). In terms of carcass quality, the study observed alterations in the carcass pH based on the temperament groups [i.e., more docile vs. more aggressive; (Petherick et al., 2002)]. The pH variations by animal temperament were speculated to be caused by differences in lactic acid concentrations, as nervous animals have higher levels of lactic acid as a consequence of the distress (Petherick et al., 2002). Other traits (e.g., foot score and hair shedding) had low genetic correlation with temperament, suggesting its partially independent genetic responses.
In summary, there were no unfavorable correlations between temperament and other relevant traits in livestock. This suggests that direct selection for temperament would not negatively impact the genetic improvement of other relevant traits. Actually, the long-term selection for growth, feed efficiency, precocity, and carcass traits has favorable effect on the indirect selection for temperament, or vice versa.
Weighted Single-Step Genome-Wide Association Analyses
Animal temperament is a behavioral response from a multifactorial process. The starting point is a stimulus, which could be from a simple touch, sight, sound, smell, or taste, until more complex levels, for instance, an intuitive feeling of dominance. The complexity extends to the genetic level, in which behavior is controlled by many genes with small effect (Costilla et al., 2020; Alvarenga et al., 2021). Despite the high heritability estimate, our study found three pieces of evidence of a polygenic nature of yearling temperament in North American Angus. First, an even distribution of variance explained by genomic windows was observed across the genome (Figure 3). Second, none of the genomic windows explained the large proportion of the total additive genetic variance for yearling temperament—the maximum variance explained was 0.51% on BTA11 (Supplementary Table S4). Finally, at the modeling level, small differences were observed in the distribution of the genomic window effect and variance explained when using ssGWAS or WssGWAS approaches (Supplementary Figure S2). Similar findings were observed by Araujo et al. (2022) while fitting haplotype groups instead of SNP effects using the AAA dataset for temperament. In general, a weighting method (e.g., WssGWAS) is expected to prioritize major regions when a less polygenic trait is being analyzed (Wang et al., 2014). In summary, the weighting process for genomic associations using SNPs is not necessary if the target trait underlies a polygenic architecture.
At the genomic level, another characteristic of yearling temperament is the influence of the X-chromosome. In comparison with other chromosomes, X was underrepresented with a total of 97 SNPs (59.13
The QTL annotation within the selected genomic regions corroborates and potentially justifies the genetic correlation between temperament and growth, carcass traits, precocity, reproduction, and maternal traits (Figure 2). The overlapping regions offer insights into mechanisms that can cause the genetic correlation, such as pleiotropy, LD between trait-specific QTLs, and/or LD between QTL and marker (Gianola et al., 2015). The majority of the QTLs were annotated for milk content and yield, which goes in line with the extended list of association studies for milk traits and annotated in the animal QTL database. No straightforward relationship between behavioral traits and milk yield have been drawn (Hedlund and Lovlie, 2015). However, many studies observed that nervous cows tend to have lower milk production, justified by differential hormonal functions (Hedlund and Lovlie, 2015). One gene annotated for milking speed (i.e., TOX) is involved in the immune system (Aliahmad et al., 2012). So far, to the best of our knowledge, no direct connection has been made between behavioral traits and the TOX gene. In human studies, there are reports indicating that the immune system can be a mediator of adulthood behavior, for example, dysregulation of immune responses was associated with higher aggression (Takahashi et al., 2018). In cattle, TOX was reported in association with puberty in Brahman beef cattle (Fortes et al., 2012) and carcass traits in Korean Hanwoo cattle (Bhuiyan et al., 2018), and it has been identified as a signature of selection in tropical adapted crossbred cattle (Cheruiyot et al., 2018).
In beef cattle, yearling temperament is associated with the length of productive life of the animal (Oliveira et al., 2020), as it is one of the voluntary culling reasons. QTLs annotated for longevity also overlapped with regions for yearling temperament. The genes located in regions for longevity and yearling temperament are mainly associated and enriched in visual mechanisms (Chang et al., 2009). The majority of the genes are from the crystalline gamma family genes (e.g., CRYGB, CRYGC, and CRYGD). In behavior, visual perception is required for the reception of a stimulus, which is subsequently converted to a physiological messenger, and properly recognized as a signal (Chang et al., 2009).
Another term identified in this study which has no significance (p = 0.42) but is biologically important is methylation (Supplementary Table S5). Altered cattle behavior has been linked with differential methylation of DNA (Corvo et al., 2020; Littlejohn et al., 2020). Three genes were involved in this methylation process (i.e., PDE6C, RBP4, and CRYGC), and out of those, PDE6C was also enriched in the visual perception. In animal models (i.e., mice), this gene has been reported in retinol progenitor regions to be highly methylated, which could impact photoreceptor mechanisms and, consequently, the signaling cascades (Dvoriantchikova et al., 2019). In summary, this study identified genes participating in the visual mechanism, in which the eyes, in addition to being responsible for stimulus reception, have been defined as the window to the brain. For instance, pupil dilation has been associated with cognitive ability and Alzheimer’s disease (Granholm et al., 2017; Ozeri-Rotstain et al., 2020).
Another gene participating in the methylation process is RBP4 (retinol binding protein gene), in which knockout mice for it showed impairment, reduced activity, and increased anxiety-like behavior (Buxbaum et al., 2014). The protein produced by the RBP4 gene is the principal retinol (i.e., vitamin A) serum transporter in the human body (Reay and Cairns, 2020). Disruption of the transport, metabolism, and signaling of vitamin A metabolites have been associated with mental and behavioral disorders in humans [reviewed by Reay and Cairns (2020)]. In cattle, RBP4 has been associated with growth traits, which might be related to insulin metabolism (Wang et al., 2010) and heifer fertility (Abdollahi-Arpanahi et al., 2019).
The region with the greatest additive genetic variance explained is located on BTA11, but no annotated gene was found in this region. However, there was one long noncoding RNA (lncRNA, ENSBTAG00000048646) located in a region explaining 0.49% of the total variation (Supplementary Table S4). Some lncRNA genes are specifically expressed in brain cells, which implies its roles in neuronal development and cognitive and behavioral regulation (Zhang et al., 2020). In cattle, this gene biotype has been associated with possible skin pigmentation (Weikard et al., 2013), meat quality (Billerey et al., 2014), and lipid metabolism and has been suggested as a possible heat-stress biomarker (Ibeagha-Awemu and Zhao, 2015). However, to the best of our knowledge, no study evaluated the impact of lncRNA on behavior in cattle.
Insulin metabolism is another pathway in which few of the genes identified in this study play an important role, for example, omega-3 Fatty Acid Receptor-1 gene (FFAR4) located on BTA26 (Marcotte et al., 2017). Insulin metabolism dysfunction could impact performance and affect the overall mood and cognitive ability of the individuals through dopaminergic abnormal functioning, as observed in humans (Kleinridders et al., 2015; Lyrae Silva et al., 2019). Similar to other genes discussed in this study (e.g., TOX), FFAR4 receptors have also been reported as triggers to inflammatory responses (Oh et al., 2010). In general, FFAR4 gene signals either a pathway involved in
Finally, Alvarenga et al. (2021) systematically reviewed genes associated with farmed mammals’ behavior. Among all genes catalogued by the authors, two (i.e., U6 and CEP55) genes previously reported to be associated with behavioral traits in livestock overlapped with the genes identified in this study (Alvarenga et al., 2021). Interestingly, the U6 spliceosomal RNA (U6) gene was previously reported to be associated with temperament (Hanna et al., 2014), maternal behavior (Michenet et al., 2016), and sucking reflex (Dreher et al., 2019) in cattle and feeding behavior (Cross et al., 2018) and adrenaline/creatine level (Terenina et al., 2013) in pigs. The U6 gene is a noncoding RNA gene; in this study, the paralog gene identified is on BTA8 explaining 0.22% of the total genetics. The U6 snRNA is highly conserved in eukaryotes, and it is located in the spliceosome, where it orchestrates the splicing function (Didychuk et al., 2018), which seems to have an evolutionary importance in terms of organism viability. Molecular and chemistry studies might be crucial to underpin the role of uridine-rich small nuclear RNAs (i.e., U6) in alternative animal behavior. However, more studies are required to elucidate the roles of the genes identified in this study, including in vitro gene knockout and gene editing studies.
Conclusions
Our study encompasses a large, diverse, and robust dataset from the North American Angus cattle population. Yearling temperament was shown to be heritable (0.38 ± 0.01), suggesting that genetic gains can be effectively obtained through direct genetic and genomic selection. Additionally, direct selection for yearling temperament is not expected to result in unfavorable effects on other economically important traits. Actually, the long-term selection for growth, feed efficiency, precocity, and carcass traits has a favorable effect on the indirect selection for temperament, or vice versa.
Sex and extrinsic factors affect animal behavior, such as age of dam, additional human–animal interaction, and birth region. Fitting maternal effects are not crucial for breeding value estimation of temperament in the US Angus population, suggesting the use of the reduced model due to its computational efficiency. Many of the genomic regions associated with yearling temperament were enriched in QTL regions linked with milking speed, longevity, and other economically important traits. Pseudo-autosomal regions of the X-chromosome seem to play a role in yearling temperament, as well as RNA gene biotypes, for example, long noncoding RNA genes. Finally, many of the genes were enriched in visual pathways, which in addition to being important for stimulus, have also been linked to cognitive abilities. The SNPs and genomic regions identified in this study can be used when designing customized SNP panels, to be further investigated in functional studies aiming to better understand the biological mechanisms influencing cattle temperament, as well as used for a biology-driven genomic prediction. In summary, the insights from our study will be useful for various practical applications and future research, including the breeding industry, farm management, and behavioral genomics studies.
Data Availability Statement
The data analyzed in this study are subject to the following licenses/restrictions: The data supporting the results of this article are included within the article and in its Supplementary Files. The raw data cannot be made available, as it is property of the American Angus Association (Saint Joseph, MO, United States) and this information is commercially sensitive. Requests to access these datasets should be directed to the corresponding author.
Author Contributions
AA partially conceptualized the article idea, analyzed the data, interpreted and discussed the results, and prepared the original draft, including writing and reviewing; HO provided technical support for the analysis, reviewed, and edited. SM participated in the conceptualization and reviewed the final version. FS provided technical support for the analysis and reviewed the final manuscript. LB conceptualized and supervised the project, provided training to the first author, and reviewed and edited the manuscript. All authors have read and agreed to the published version of the manuscript.
Funding
This project was partially funded by the American Angus Association and Angus Genetics Inc. (MO, United States).
Conflict of Interest
Author SM was employed by the Angus Genetics Inc.
The remaining authors declare that the research was conducted in the absence of any commercial or financial relationships that could be construed as a potential conflict of interest.
Publisher’s Note
All claims expressed in this article are solely those of the authors and do not necessarily represent those of their affiliated organizations, or those of the publisher, the editors, and the reviewers. Any product that may be evaluated in this article, or claim that may be made by its manufacturer, is not guaranteed or endorsed by the publisher.
Acknowledgments
The authors acknowledge American Angus Association and Angus Genetics Inc. staff for all the support in regard to data availability and computing resources.
Supplementary Material
The Supplementary Material for this article can be found online at: https://www.frontiersin.org/articles/10.3389/fgene.2022.794625/full#supplementary-material
References
Abdollahi-Arpanahi, R., Carvalho, M. R., Ribeiro, E. S., and Peñagaricano, F. (2019). Association of Lipid-Related Genes Implicated in Conceptus Elongation with Female Fertility Traits in Dairy Cattle. J. Dairy Sci. 102, 10020–10029. doi:10.3168/jds.2019-17068
Aguilar, I., Misztal, I., Johnson, D. L., Legarra, A., Tsuruta, S., and Lawlor, T. J. (2010). Hot Topic: a Unified Approach to Utilize Phenotypic, Full Pedigree, and Genomic Information for Genetic Evaluation of Holstein Final Score. J. Dairy Sci. 93, 743–752. doi:10.3168/jds.2009-2730
Aguilar, I., Misztal, I., Tsuruta, S., Legarra, A., and Wang, H. (2014). “PREGSF90 – POSTGSF90: Computational Tools for the Implementation of Single-step Genomic Selection and Genome-wide Association with Ungenotyped Individuals in BLUPF90 Programs,” in Abstract retrieved from 10th World Congress of Genetics Applied to Livestock Production, Vancouver, Canada, August 17–22, 2014. doi:10.13140/2.1.4801.5045
Aliahmad, P., Seksenyan, A., and Kaye, J. (2012). The many Roles of TOX in the Immune System. Curr. Opin. Immunol. 24, 173–177. doi:10.1016/j.coi.2011.12.001
Altman, D. G., and Bland, J. M. (2005). Standard Deviations and Standard Errors. BMJ 331, 903. doi:10.1136/bmj.331.7521.903
Alvarenga, A. B., Oliveira, H. R., Chen, S.-Y., Miller, S. P., Marchant-Forde, J. N., Grigoletto, L., et al. (2021). A Systematic Review of Genomic Regions and Candidate Genes Underlying Behavioral Traits in Farmed Mammals and Their Link with Human Disorders. Animals 11, 715. doi:10.3390/ani11030715
Araujo, A. C., Carneiro, P. L. S., Alvarenga, A. B., Oliveira, H. R., Miller, S. P., Brito, L. B., et al. (2022). Haplotype-based Single-step GWAS for Yearling Temperament in American Angus Cattle. Genes 13, 17. doi:10.3390/genes13010017
Barrozo, D., Buzanskas, M. E., Oliveira, J. A., Munari, D. P., Neves, H. H. R., and Queiroz, S. A. (2012). Genetic Parameters and Environmental Effects on Temperament Score and Reproductive Traits of Nellore Cattle. Animal 6, 36–40. doi:10.1017/S1751731111001169
Beckman, D. W., Enns, R. M., Speidel, S. E., Brigham, B. W., and Garrick, D. J. (2007). Maternal Effects on Docility in Limousin Cattle1. J. Anim. Sci. 85, 650–657. doi:10.2527/jas.2006-450
Bhuiyan, M. S. A., Lim, D., Park, M., Lee, S., Kim, Y., Gondro, C., et al. (2018). Functional Partitioning of Genomic Variance and Genome-wide Association Study for Carcass Traits in Korean Hanwoo Cattle Using Imputed Sequence Level SNP Data. Front. Genet. 9, 1–14. doi:10.3389/fgene.2018.00217
Bijma, P. (2006). Estimating Maternal Genetic Effects in Livestock1. J. Anim. Sci. 84, 800–806. doi:10.2527/2006.844800x
Billerey, C., Boussaha, M., Esquerré, D., Rebours, E., Djari, A., Meersseman, C., et al. (2014). Identification of Large Intergenic Non-coding RNAs in Bovine Muscle Using Next-Generation Transcriptomic Sequencing. BMC Genomics 15, 1. doi:10.1186/1471-2164-15-499
Blake, J. A., Dolan, M., Drabkin, H., Hill, D. P., Ni, L., Sitnikov, D., et al. (2013). Gene Ontology Annotations and Resources. Nucleic Acids Res. 41, D530–D535. doi:10.1093/nar/gks1050
Boivin, X., Gilard, F., and Egal, D. (2009). The Effect of Early Human Contact and the Separation Method from the Dam on Responses of Beef Calves to Humans. Appl. Anim. Behav. Sci. 120, 132–139. doi:10.1016/j.applanim.2009.05.011
Broadhead, D. L., Stockton, M., Stalker, L. A., Musgrave, J. A., and Funston, R. N. (2018). 418 Economics of Creep Feeding a Spring Calving Beef Herd in the Nebraska Sandhills. J. Anim. Sci. 96, 224–225. doi:10.1093/jas/sky073.415
Burdick, N. C., Randel, R. D., Carroll, J. A., and Welsh, T. H. (2011). Interactions between Temperament, Stress, and Immune Function in Cattle. Int. J. Zoolog. 2011, 1–9. doi:10.1155/2011/373197
Burrow, H. M. (2001). Variances and Covariances between Productive and Adaptive Traits and Temperament in a Composite Breed of Tropical Beef Cattle. Livestock Prod. Sci. 70, 213–233. doi:10.1016/S0301-6226(01)00178-6
Burrow, H., Seifert, G., and Corbet, N. (1988). A New Technique for Measuring Temperament in Cattle. Soc. Anim. Prod. 17, 54.
Busby, D. (2015). “Lessons Learned from 32 Years of Retained Ownership – TCSCF Summary,” in Abstract retrieved from Driftless Region Beef Conference, Iowa, January 22–23, 2015, 29–31.
Buxbaum, J. N., Roberts, A. J., Adame, A., and Masliah, E. (2014). Silencing of Murine Transthyretin and Retinol Binding Protein Genes Has Distinct and Shared Behavioral and Neuropathologic Effects. Neuroscience 275, 352–364. doi:10.1016/j.neuroscience.2014.06.019
Calo, L. L., McDowell, R. E., VanVleck, L. D., and Miller, P. D. (1973). Genetic Aspects of Beef Production Among Holstein-Friesians Pedigree Selected for Milk Production1. J. Anim. Sci. 37, 676–682. doi:10.2527/jas1973.373676x
Cantrell, B., Lachance, H., Murdoch, B., Sjoquist, J., Funston, R., Weaber, R., et al. (2019). Global DNA Methylation in the Limbic System of Cattle. Epigenomes 3, 8. doi:10.3390/epigenomes3020008
Carlström, C., Strandberg, E., Johansson, K., Pettersson, G., Stålhammar, H., and Philipsson, J. (2014). Genetic Evaluation of In-Line Recorded Milkability from Milking Parlors and Automatic Milking Systems. J. Dairy Sci. 97, 497–506. doi:10.3168/jds.2013-6948
Chan, F. Y. (2012). “Genome Wide Association Studies for Temperament in New Zealand Dairy Cattle,” (Palmerston North, NZ: Massey University). Master’s thesis.
Chang, B., Grau, T., Dangel, S., Hurd, R., Jurklies, B., Sener, E. C., et al. (2009). A Homologous Genetic Basis of the Murine Cpfl1 Mutant and Human Achromatopsia Linked to Mutations in the PDE6C Gene. Proc. Natl. Acad. Sci. 106, 19581–19586. doi:10.1073/pnas.0907720106
Cheruiyot, E. K., Bett, R. C., Amimo, J. O., Zhang, Y., Mrode, R., and Mujibi, F. D. N. (2018). Signatures of Selection in Admixed Dairy Cattle in Tanzania. Front. Genet. 9, 1–15. doi:10.3389/fgene.2018.00607
Christensen, O. F., and Lund, M. S. (2010). Genomic Prediction when Some Animals Are Not Genotyped. Genet. Sel Evol. 42, 8. doi:10.1186/1297-9686-42-2
Cole, J. B., VanRaden, P. M., O’Connell, J. R., van Tassell, C. P., Sonstegard, T. S., Schnabel, R. D., et al. (2009). Distribution and Location of Genetic Effects for Dairy Traits. J. Dairy Sci. 92, 2931–2946. doi:10.3168/jds.2008-1762
Cooke, R. F. (2014). Bill E. Kunkle Interdisciplinary Beef Symposium: Temperament and Acclimation to Human Handling Influence Growth, Health, and Reproductive Responses in Bos taurus and Bos indicus Cattle1. J. Anim. Sci. 92, 5325–5333. doi:10.2527/jas.2014-8017
Cooke, R. F., Bohnert, D. W., Meneghetti, M., Losi, T. C., and Vasconcelos, J. L. M. (2011). Effects of Temperament on Pregnancy Rates to Fixed-Timed AI in Bos indicus Beef Cows. Livestock Sci. 142, 108–113. doi:10.1016/j.livsci.2011.06.024
Costa, A., Bovenhuis, H., and Penasa, M. (2020). Changes in Milk Lactose Content as Indicators for Longevity and Udder Health in Holstein Cows. J. Dairy Sci. 103, 11574–11584. doi:10.3168/jds.2020-18615
Costilla, R., Kemper, K. E., Byrne, E. M., Porto-Neto, L. R., Carvalheiro, R., Purfield, D. C., et al. (2020). Genetic Control of Temperament Traits across Species: Association of Autism Spectrum Disorder Risk Genes with Cattle Temperament. Genet. Sel Evol. 52, 1–14. doi:10.1186/s12711-020-00569-z
Coutinho, M. A. d. S., Ramos, P. M., da Luz e Silva, S., Martello, L. S., Pereira, A. S. C., and Delgado, E. F. (2017). Divergent Temperaments Are Associated with Beef Tenderness and the Inhibitory Activity of Calpastatin. Meat Sci. 134, 61–67. doi:10.1016/j.meatsci.2017.06.017
Cross, A. J., Keel, B. N., Brown-Brandl, T. M., Cassady, J. P., and Rohrer, G. A. (2018). Genome-wide Association of Changes in Swine Feeding Behaviour Due to Heat Stress. Genet. Sel Evol. 50, 1–12. doi:10.1186/s12711-018-0382-1
Cundiff, L. V., Van Vleck, L. D., and Hohenboken, W. D. (2018). Guidelines for Uniform Beef Improvement Programs (Beef Improvement Federation) Guidelines. Available at: beefimprovement.org/index.php/Guidelines_for_Uniform_Beef_Improvement_Programs (Accessed September, 2021).
Cziszter, L. T., Gavojdian, D., Neamt, R., Neciu, F., Kusza, S., and Ilie, D.-E. (2016). Effects of Temperament on Production and Reproductive Performances in Simmental Dual-Purpose Cows. J. Vet. Behav. 15, 50–55. doi:10.1016/j.jveb.2016.08.070
Dechow, C. D., Sondericker, K. S., Enab, A. A., and Hardie, L. C. (2020). Genetic, Farm, and Lactation Effects on Behavior and Performance of US Holsteins in Automated Milking Systems. J. Dairy Sci. 103, 11503–11514. doi:10.3168/jds.2020-18786
Del Corvo, M., Bongiorni, S., Stefanon, B., Sgorlon, S., Valentini, A., Ajmone Marsan, P., et al. (2020). Genome-wide DNA Methylation and Gene Expression Profiles in Cows Subjected to Different Stress Level as Assessed by Cortisol in Milk. Genes 11, 850–919. doi:10.3390/genes11080850
Dennis, G., Sherman, B. T., Hosack, D. A., Yang, J., Gao, W., Lane, H. C., et al. (2003). DAVID: Database for Annotation, Visualization, and Integrated Discovery. Genome Biol. 4, P3. doi:10.1186/gb-2003-4-5-p3
Dickson, D. P., Barr, G. R., Johnson, L. P., and Wieckert, D. A. (1970). Social Dominance and Temperament of Holstein Cows. J. Dairy Sci. 53, 904–907. doi:10.3168/jds.S0022-0302(70)86316-0
Didychuk, A. L., Butcher, S. E., and Brow, D. A. (2018). The Life of U6 Small Nuclear RNA, from Cradle to Grave. RNA 24, 437–460. doi:10.1261/rna.065136.117
Dikmen, S., Cole, J. B., Null, D. J., and Hansen, P. J. (2012). Heritability of Rectal Temperature and Genetic Correlations with Production and Reproduction Traits in Dairy Cattle. J. Dairy Sci. 95, 3401–3405. doi:10.3168/jds.2011-4306
Dodenhoff, J., Van Vleck, L. D., and Wilson, D. E. (1999). Comparison of Models to Estimate Genetic Effects of Weaning Weight of Angus Cattle. J. Anim. Sci. 77, 3176–3184. doi:10.2527/1999.77123176x
Dos Santos, F. C., Peixoto, M. G. C. D., Fonseca, P. A. d. S., Pires, M. d. F. Á., Ventura, R. V., Rosse, I. d. C., et al. (2017). Identification of Candidate Genes for Reactivity in Guzerat (Bos indicus) Cattle: A Genome-wide Association Study. PLoS ONE 12, e0169163–15. doi:10.1371/journal.pone.0169163
Dreher, C., Wellmann, R., Stratz, P., Schmid, M., Preuß, S., Hamann, H., et al. (2019). Genomic Analysis of Perinatal Sucking Reflex in German Brown Swiss Calves. J. Dairy Sci. 102, 6296–6305. doi:10.3168/jds.2019-16487
Durinck, S., Spellman, P. T., Birney, E., and Huber, W. (2009). Mapping Identifiers for the Integration of Genomic Datasets with the R/Bioconductor Package biomaRt. Nat. Protoc. 4, 1184–1191. doi:10.1038/jid.2014.371
Dvoriantchikova, G., Seemungal, R. J., and Ivanov, D. (2019). DNA Methylation Dynamics during the Differentiation of Retinal Progenitor Cells into Retinal Neurons Reveal a Role for the DNA Demethylation Pathway. Front. Mol. Neurosci. 12, 1–9. doi:10.3389/fnmol.2019.00182
Ede, T., Lecorps, B., von Keyserlingk, M. A. G., and Weary, D. M. (2019). Calf Aversion to Hot-Iron Disbudding. Sci. Rep. 9, 1–6. doi:10.1038/s41598-019-41798-7
Esmailizadeh, A. K., Bottema, C. D. K., Sellick, G. S., Verbyla, A. P., Morris, C. A., Cullen, N. G., et al. (2008). Effects of the Myostatin F94L Substitution on Beef Traits1. J. Anim. Sci. 86, 1038–1046. doi:10.2527/jas.2007-0589
Fortes, M. R. S., Lehnert, S. A., Bolormaa, S., Reich, C., Fordyce, G., Corbet, N. J., et al. (2012). Finding Genes for Economically Important Traits: Brahman Cattle Puberty. Anim. Prod. Sci. 52, 143–150. doi:10.1071/AN11165
Garza-Brenner, E., Sifuentes-Rincón, A. M., Randel, R. D., Paredes-Sánchez, F. A., Parra-Bracamonte, G. M., Arellano Vera, W., et al. (2017). Association of SNPs in Dopamine and Serotonin Pathway Genes and Their Interacting Genes with Temperament Traits in Charolais Cows. J. Appl. Genet. 58, 363–371. doi:10.1007/s13353-016-0383-0
Gauly, M., Mathiak, H., Hoffmann, K., Kraus, M., and Erhardt, G. (2001). Estimating Genetic Variability in Temperamental Traits in German Angus and Simmental Cattle. Appl. Anim. Behav. Sci. 74, 109–119. doi:10.1016/S0168-1591(01)00151-4
Geweke, J. (1991). Evaluating the Accuracy of Sampling-Based Approaches to the Calculation of Posterior Moments. Bayesian Statistics. Minneapolis, USA: Federal Reserve Bank of Minneapolis, Research Department, 169–193.
Gianola, D., de los Campos, G., Toro, M. A., Naya, H., Schön, C.-C., and Sorensen, D. (2015). Do molecular Markers Inform about Pleiotropy? Genetics 201, 23–29. doi:10.1534/genetics.115.179978
Glenske, K., Prinzenberg, E.-M., Brandt, H., Gauly, M., and Erhardt, G. (2011). A Chromosome-wide QTL Study on BTA29 Affecting Temperament Traits in German Angus Beef Cattle and Mapping of DRD4. Animal 5, 195–197. doi:10.1017/S1751731110001801
Granholm, E. L., Panizzon, M. S., Elman, J. A., Jak, A. J., Hauger, R. L., Bondi, M. W., et al. (2017). Pupillary Responses as a Biomarker of Early Risk for Alzheimer's Disease. Jad 56, 1419–1428. doi:10.3233/JAD-161078
Greenwood, P. L. (2021). Review: An Overview of Beef Production from Pasture and Feedlot Globally, as Demand for Beef and the Need for Sustainable Practices Increase. Animal 15, 100295. doi:10.1016/j.animal.2021.100295
Haskell, M. J., Simm, G., and Turner, S. P. (2014). Genetic Selection for Temperament Traits in Dairy and Beef Cattle. Front. Genet. 5, 1–18. doi:10.3389/fgene.2014.00368
Hearnshaw, H., and Morris, C. (1984). Genetic and Environmental Effects on a Temperament Score in Beef Cattle. Aust. J. Agric. Res. 35, 723–733. doi:10.1071/AR9840723
Hedlund, L., and Løvlie, H. (2015). Personality and Production: Nervous Cows Produce Less Milk. J. Dairy Sci. 98, 5819–5828. doi:10.3168/jds.2014-8667
Heidelberger, P., and Welch, P. D. (1983). Simulation Run Length Control in the Presence of an Initial Transient. Operations Res. 31, 1109–1144. doi:10.1287/opre.31.6.1109
Hidalgo, J., Tsuruta, S., Lourenco, D., Masuda, Y., Huang, Y., Gray, K. A., et al. (2020). Changes in Genetic Parameters for Fitness and Growth Traits in Pigs under Genomic Selection. J. Anim. Sci. 98, 1–12. doi:10.1093/jas/skaa032
Hoppe, S., Brandt, H. R., König, S., Erhardt, G., and Gauly, M. (2010). Temperament Traits of Beef Calves Measured under Field Conditions and Their Relationships to Performance1. J. Anim. Sci. 88, 1982–1989. doi:10.2527/jas.2008-1557
Hu, Z.-L., Park, C. A., and Reecy, J. M. (2019). Building a Livestock Genetic and Genomic Information Knowledgebase through Integrative Developments of Animal QTLdb and CorrDB. Nucleic Acids Res. 47, D701–D710. doi:10.1093/nar/gky1084
Hulsman Hanna, L. L., Garrick, D. J., Gill, C. A., Herring, A. D., Riggs, P. K., Miller, R. K., et al. (2014). Genome-wide Association Study of Temperament and Tenderness Using Different Bayesian Approaches in a Nellore-Angus Crossbred Population. Livestock Sci. 161, 17–27. doi:10.1016/j.livsci.2013.12.012
Ibeagha-Awemu, E. M., and Zhao, X. (2015). Epigenetic marks: Regulators of Livestock Phenotypes and Conceivable Sources of Missing Variation in Livestock Improvement Programs. Front. Genet. 6, 1–17. doi:10.3389/fgene.2015.00302
Johnson, T., Keehan, M., Harland, C., Lopdell, T., Spelman, R. J., Davis, S. R., et al. (2019). Short Communication: Identification of the Pseudoautosomal Region in the Hereford Bovine Reference Genome Assembly ARS-UCD1.2. J. Dairy Sci. 102, 3254–3258. doi:10.3168/jds.2018-15638
Kanehisa, M., and Goto, S. (2000). KEGG: Kyoto Encyclopedia of Genes and Genomes. Nucleic Acids Res. 28, 27–30. doi:10.1093/nar/28.1.27
Karamfilov, S. (2022). Study on the Temperament of Cows of the Aberdeen Angus Cattle Breed. Czech J. Anim. Sci. 67, 8–14. doi:10.17221/88/2021-cjas
Kasimanickam, V., Abdel Aziz, R., Williams, H., and Kasimanickam, R. (2018). Predictors of Beef Calf Temperament at Weaning and its Impact on Temperament at Breeding and Reproductive Performance. Reprod. Dom Anim. 53, 484–494. doi:10.1111/rda.13135
Kilgour, R. (1975). The Open-Field Test as an Assessment of the Temperament of Dairy Cows. Anim. Behav. 23, 615–624. doi:10.1016/0003-3472(75)90139-6
King, D. A., Schuehle Pfeiffer, C. E., Randel, R. D., Welsh, T. H., Oliphint, R. A., Baird, B. E., et al. (2006). Influence of Animal Temperament and Stress Responsiveness on the Carcass Quality and Beef Tenderness of Feedlot Cattle. Meat Sci. 74, 546–556. doi:10.1016/j.meatsci.2006.05.004
Kleinridders, A., Cai, W., Cappellucci, L., Ghazarian, A., Collins, W. R., Vienberg, S. G., et al. (2015). Insulin Resistance in Brain Alters Dopamine Turnover and Causes Behavioral Disorders. Proc. Natl. Acad. Sci. USA 112, 3463–3468. doi:10.1073/pnas.1500877112
Legarra, A., and Reverter, A. (2018). Semi-parametric Estimates of Population Accuracy and Bias of Predictions of Breeding Values and Future Phenotypes Using the LR Method. Genet. Sel Evol. 50, 1–18. doi:10.1186/s12711-018-0426-6
Lenth, R. V. (2016). Least-Squares Means: TheRPackagelsmeans. J. Stat. Soft. 69. doi:10.18637/jss.v069.i01
Littlejohn, B. P., Price, D. M., Neuendorff, D. A., Carroll, J. A., Vann, R. C., Riggs, P. K., et al. (2020). Influence of Prenatal Transportation Stress-Induced Differential DNA Methylation on the Physiological Control of Behavior and Stress Response in Suckling Brahman Bull Calves. J. Anim. Sci. 98, 1–18. doi:10.1093/jas/skz368
Lu, D., Sargolzaei, M., Kelly, M., Li, C., Vander Voort, G., Wang, Z., et al. (2012). Linkage Disequilibrium in Angus, Charolais, and Crossbred Beef Cattle. Front. Gene 3, 1–10. doi:10.3389/fgene.2012.00152
Lyrae Silva, N. D. M., Lam, M. P., Soares, C. N., Munoz, D. P., Milev, R., and De Felice, F. G. (2019). Insulin Resistance as a Shared Pathogenic Mechanism between Depression and Type 2 Diabetes. Front. Psychiatry 10. doi:10.3389/fpsyt.2019.00057
Macleod, I. M., Bowman, P. J., Chamberlain, A. J., Vander Jagt, C. J., Daetwyler, H. D., Hayes, B. J., et al. (2019). “Genomic Prediction and Candidate Gene Discovery for Dairy Cattle Temperament Using Sequence Data and Functional Biology,” in Abstract retrieved from the 23rd Association for the Advancement of Animal Breeding and Genetics, Armidale, NSW, October 27–November 1, 2019, 416–419.
McBride, W. D., and Mathews, K. (2011). The Diverse Structure and Organization of U.S. Beef Cow-Calf Farms. Available at: www.ers.usda.gov/webdocs/publications/44530/7611_eib73.pdf?v=0 (Accessed September, 2021).
Medrano, J. F. (2017). “The New Bovine Reference Assembly and its Value for Genomic Research,” in Abstract retrieved from the 21st Association for the Advancement of Animal Breeding and Genetics, Queensland, Australia, July 2–5, 2017, 161–166.
Meyer, K. (1994). Estimates of Direct and Maternal Correlations Among Growth Traits in Australian Beef Cattle. Livestock Prod. Sci. 38, 91–105. doi:10.1016/0301-6226(94)90053-1
Michenet, A., Saintilan, R., Venot, E., and Phocas, F. (2016). Insights into the Genetic Variation of Maternal Behavior and Suckling Performance of continental Beef Cows. Genet. Sel Evol. 48, 1–12. doi:10.1186/s12711-016-0223-z
Misztal, I., Legarra, A., and Aguilar, I. (2009). Computing Procedures for Genetic Evaluation Including Phenotypic, Full Pedigree, and Genomic Information. J. Dairy Sci. 92, 4648–4655. doi:10.3168/jds.2009-2064
Misztal, I., Tsuruta, S., Aguilar, I., Legarra, A., VanRaden, P. M., and Lawlor, T. J. (2013). Methods to Approximate Reliabilities in Single-step Genomic Evaluation. J. Dairy Sci. 96, 647–654. doi:10.3168/jds.2012-5656
Musante, L., and Ropers, H. H. (2014). Genetics of Recessive Cognitive Disorders. Trends Genet. 30, 32–39. doi:10.1016/j.tig.2013.09.008
Neja, W., Sawa, A., Jankowska, M., Bogucki, M., and Krężel-Czopek, S. (2015). Effect of the Temperament of Dairy Cows on Lifetime Production Efficiency. Arch. Anim. Breed. 58, 193–197. doi:10.5194/aab-58-193-2015
Northcutt, S., and Bowman, B. (2007). By the Numbers: Docility Genetic Evaluation Research. Available at: www.angus.org/Nce/Documents/ByThenumbersDocility.pdf (Accessed September, 2021).
Oh, D. Y., Talukdar, S., Bae, E. J., Imamura, T., Morinaga, H., Fan, W., et al. (2010). GPR120 Is an omega-3 Fatty Acid Receptor Mediating Potent Anti-inflammatory and Insulin-Sensitizing Effects. Cell 142, 687–698. doi:10.1016/j.cell.2010.07.041
Oh, D. Y., and Walenta, E. (2014). Omega-3 Fatty Acids and FFAR4. Front. Endocrinol. 5, 2–6. doi:10.3389/fendo.2014.00115
Oliveira, H. R., Brito, L. F., Miller, S. P., and Schenkel, F. S. (2020). Using Random Regression Models to Genetically Evaluate Functional Longevity Traits in North American Angus Cattle. Animals 10, 2410–2430. doi:10.3390/ani10122410
Ozeri-Rotstain, A., Shachaf, I., Farah, R., and Horowitz-Kraus, T. (2020). Relationship between Eye-Movement Patterns, Cognitive Load, and reading Ability in Children with reading Difficulties. J. Psycholinguist Res. 49, 491–507. doi:10.1007/s10936-020-09705-8
Petherick, J. C., Holroyd, R. G., Doogan, V. J., and Venus, B. K. (2002). Productivity, Carcass and Meat Quality of Lot-Fed Bos indicus Cross Steers Grouped According to Temperament. Aust. J. Exp. Agric. 42, 389–398. doi:10.1071/EA01084
Phocas, F., Boivin, X., Sapa, J., Trillat, G., Boissy, A., and Le Neindre, P. (2006). Genetic Correlations between Temperament and Breeding Traits in Limousin Heifers. Anim. Sci. 82, 805–811. doi:10.1017/ASC200696
R Core Team (2019). R: A Language and Environment for Statistical Computing. Vienna, Austria: R Foundation for Statistical Computing.
Reay, W. R., and Cairns, M. J. (2020). The Role of the Retinoids in Schizophrenia: Genomic and Clinical Perspectives. Mol. Psychiatry 25, 706–718. doi:10.1038/s41380-019-0566-2
Reinhardt, V., Reinhardt, C., and Reinhardt, A. (1987). Evaluating Sex Differences in Aggressiveness in Cattle, Bison and Rhesus Monkeys. Behav 102, 58–66. Available at: brill.com/view/journals/beh/102/1-2/article-p58_4.xml?casa_token=_Lc1kNXVu_8AAAAA:itN_-I1NB9w7VZiB40fpS0uZ_oCkfajHcIuM1sBBSm9Nur9jV2BeVX1Q-rvl0XpQkIKQh7CTyA. doi:10.1163/156853986x00045 Accessed February 14, 2022).
Riley, D. G., Gill, C. A., Boldt, C. R., Funkhouser, R. R., Herring, A. D., Riggs, P. K., et al. (2016). Crossbred Bos indicus Steer Temperament as Yearlings and Whole Genome Association of Steer Temperament as Yearlings and Calf Temperament post-weaning12. J. Anim. Sci. 94, 1408–1414. doi:10.2527/jas.2015-0041
Rolfe, K. M., Snelling, W. M., Nielsen, M. K., Freetly, H. C., Ferrell, C. L., and Jenkins, T. G. (2011). Genetic and Phenotypic Parameter Estimates for Feed Intake and Other Traits in Growing Beef Cattle, and Opportunities for Selection123. J. Anim. Sci. 89, 3452–3459. doi:10.2527/jas.2011-3961
Rosen, B. D., Bickhart, D. M., Schnabel, R. D., Koren, S., Elsik, C. G., Zimin, A., et al. (2018). “Modernizing the Bovine Reference Genome Assembly,” in 11th World Congress of Genetics Applied to Livestock Production, Auckland, New Zealand, February 11–16, 2018, 6–11.
Sant'Anna, A. C., Paranhos da Costa, M. J. R., Baldi, F., Rueda, P. M., and Albuquerque, L. G. (2012). Genetic Associations between Flight Speed and Growth Traits in Nellore Cattle1. J. Anim. Sci. 90, 3427–3432. doi:10.2527/jas.2011-5044
Sant’anna, A. C., Valente, T. D. S., Magalhães, A. F. B., Espigolan, R., Ceballos, M. C., De Albuquerque, L. G., et al. (2019). Relationships between Temperament, Meat Quality, and Carcass Traits in Nellore Cattle1. J. Anim. Sci. 97, 4721–4731. doi:10.1093/jas/skz324
Santos, B., Archer, J. A., Martin-Collado, D., Quinton, C. D., Crowley, J., Amer, P. R., et al. (2019). “Industry Consultation Survey for the American Angus $Value Indexes Review,” in 23rd Conference of the Association for the Advancement of Animal Breeding and Genetics, Armidale, NSW, October 27–November 1, 2019.
Sato, S. (1981). Factors Associated with Temperament of Beef Cattle. Nihon Chikusan Gakkaiho 52, 595–605. doi:10.2508/chikusan.52.595
Smith, B. J. (2007). boa: AnRPackage for MCMC Output Convergence Assessment and Posterior Inference. J. Stat. Soft. 21. doi:10.18637/jss.v021.i11
Song, Y., Biernacka, J. M., and Winham, S. J. (2021). Testing and Estimation of X-Chromosome SNP Effects: Impact of Model Assumptions. Genet. Epidemiol. 45 (6), 577–592. doi:10.1002/gepi.22393
Strandén, I., and Garrick, D. J. (2009). Technical Note: Derivation of Equivalent Computing Algorithms for Genomic Predictions and Reliabilities of Animal merit. J. Dairy Sci. 92, 2971–2975. doi:10.3168/jds.2008-1929
Takahashi, A., Flanigan, M. E., McEwen, B. S., and Russo, S. J. (2018). Aggression, Social Stress, and the Immune System in Humans and Animal Models. Front. Behav. Neurosci. 12. doi:10.3389/fnbeh.2018.00056
Terenina, E., Babigumira, B. M., Le Mignon, G., Bazovkina, D., Rousseau, S., Salin, F., et al. (2013). Association Study of Molecular Polymorphisms in Candidate Genes Related to Stress Responses with Production and Meat Quality Traits in Pigs. Domest. Anim. Endocrinol. 44, 81–97. doi:10.1016/j.domaniend.2012.09.004
Tsuruta, S., and Misztal, I. (2006). “THRGIBBSF90 for Estimation of Variance Components with Threshold and Linear Models,” in Abstract retrieved from 8th World Congress on Genetics Applied to Livestock Production, Belo Horizonte, Brazil, August 13–18, 2006.
Tulloh, N. M. (1961). Behaviour of Cattle in Yards. II. A Study of Temperament. Anim. Behav. 9, 25–30. doi:10.1016/0003-3472(61)90046-X
USDA–APHIS, United States Department of Agriculture-Animal and Plant Health Inspection Service (2017). Beef Cow-Calf Management Practices in the United States. Available at: www.ers.usda.gov/publications/pub-details/?pubid=44532 (Accessed September, 2021).
Valente, T. S., Sant’Anna, A. C., Baldi, F., Albuquerque, L. G., and da Costa, M. J. R. P. (2015). Genetic Association between Temperament and Sexual Precocity Indicator Traits in Nellore Cattle. J. Appl. Genet. 56, 349–354. doi:10.1007/s13353-014-0259-0
Vallée Marcotte, B., Cormier, H., Rudkowska, I., Lemieux, S., Couture, P., and Vohl, M.-C. (2017). Polymorphisms in FFAR4 (GPR120) Gene Modulate Insulin Levels and Sensitivity after Fish Oil Supplementation. Jpm 7, 15. doi:10.3390/jpm7040015
VanRaden, P. M. (2008). Efficient Methods to Compute Genomic Predictions. J. Dairy Sci. 91, 4414–4423. doi:10.3168/jds.2007-0980
Wang, H., Misztal, I., Aguilar, I., Legarra, A., Fernando, R. L., Vitezica, Z., et al. (2014). Genome-wide Association Mapping Including Phenotypes from Relatives without Genotypes in a Single-step (ssGWAS) for 6-week Body Weight in Broiler Chickens. Front. Genet. 5, 1–10. doi:10.3389/fgene.2014.00134
Wang, H., Misztal, I., Aguilar, I., Legarra, A., and Muir, W. M. (2012). Genome-wide Association Mapping Including Phenotypes from Relatives without Genotypes. Genet. Res. 94, 73–83. doi:10.1017/S0016672312000274
Wang, M., Lai, X., Yu, H., Wang, J., Chen, Z.-Q., Lan, X.-Y., et al. (2010). Two Novel Single Nucleotide Polymorphisms (SNPs) and 4-bp Deletion Mutation of RBP4 Gene in Chinese Cattle. J. Genet. 89, 233–236. doi:10.1007/s12041-010-0031-0
Weikard, R., Hadlich, F., and Kuehn, C. (2013). Identification of Novel Transcripts and Noncoding RNAs in Bovine Skin by Deep Next Generation Sequencing. BMC Genomics 14, 789. doi:10.1186/1471-2164-14-789
Willham, R. L. (2000). Problems in Estimating Maternal Effects. Livestock Prod. Sci. 7, 405–418. doi:10.1016/0301-6226(80)90080-9
Keywords: behavior, genome-wide association study, livestock, long noncoding RNA genes, xlinked trait, weighted single-step GBLUP
Citation: Alvarenga AB, Oliveira HR, Miller SP, Silva FF and Brito LF (2022) Genetic Modeling and Genomic Analyses of Yearling Temperament in American Angus Cattle and Its Relationship With Productive Efficiency and Resilience Traits. Front. Genet. 13:794625. doi: 10.3389/fgene.2022.794625
Received: 13 October 2021; Accepted: 25 February 2022;
Published: 04 April 2022.
Edited by:
Fernando Baldi, São Paulo State University, BrazilReviewed by:
Just Jensen, Aarhus University, DenmarkLudmilla Costa Brunes, Brazilian Agricultural Research Corporation (EMBRAPA), Brazil
Angelica Pereira, University of São Paulo, Brazil
Copyright © 2022 Alvarenga, Oliveira, Miller, Silva and Brito. This is an open-access article distributed under the terms of the Creative Commons Attribution License (CC BY). The use, distribution or reproduction in other forums is permitted, provided the original author(s) and the copyright owner(s) are credited and that the original publication in this journal is cited, in accordance with accepted academic practice. No use, distribution or reproduction is permitted which does not comply with these terms.
*Correspondence: Luiz F. Brito, YnJpdG9sQHB1cmR1ZS5lZHU=