- 1Department of Epidemiology, School of Public Health, Fudan University, Shanghai, China
- 2Key Laboratory of Public Health Safety, Ministry of Education, Fudan University, Shanghai, China
- 3Fudan University Taizhou Institute of Health Sciences, Taizhou, China
- 4State Key Laboratory of Genetic Engineering and Collaborative Innovation Center for Genetics and Development, School of Life Sciences, Fudan University, Shanghai, China
- 5Department of Chronic Diseases Prevention, Taizhou Center for Disease Control and Prevention, Jiangsu, China
- 6Human Phenome Institute, Fudan University, Shanghai, China
Background and Aim: Aberrant sleep parameters are associated with the risk of nonalcoholic fatty liver disease (NAFLD). However, existing information is inconsistent among studies and involves reverse causation. Therefore, we aimed to investigate the observational associations and causations between sleep traits and NAFLD.
Methods: We performed multivariable regression to assess observational associations of seven sleep traits (sleep duration, easiness of getting up in the morning, chronotype, nap during day, snoring, insomnia, and narcolepsy), and NAFLD in the UK Biobank (1,029 NAFLD). The Cox proportional hazards model was applied to derive hazard ratios and 95% confidence intervals (CIs). Furthermore, a bidirectional two-sample Mendelian randomization (MR) approach was used to explore the causal relationships between sleep traits and NAFLD.
Results: In the multivariable regression model adjusted for potential confounders, getting up in the morning not at all easy (HR, 1.51; 95% CI, 1.27–1.78) and usually insomnia (HR, 1.46; 95% CI, 1.21–1.75) were associated with the risk of NAFLD. Furthermore, the easiness of getting up in the morning and insomnia showed a dose–response association with NAFLD (Ptrend <0.05). MR analysis found consistent causal effects of NAFLD on easiness of getting up in the morning (OR, 0.995; 95% CI, 0.990–0.999; p = 0.033) and insomnia (OR, 1.006; 95% CI, 1.001–1.011; p = 0.024). These results were robust to weak instrument bias, pleiotropy, and heterogeneity.
Conclusions: Findings showed consistent evidence of observational analyses and MR analyses that trouble getting up in the morning and insomnia were associated with an increased risk of NAFLD. Bidirectional MR demonstrated causal effects of NAFLD on sleep traits.
Introduction
Nonalcoholic fatty liver disease (NAFLD) is the leading cause of chronic liver diseases worldwide (Younossi, 2017; Estes et al., 2018). The global prevalence of NAFLD is approximately 25% (Younossi et al., 2016) and is estimated to reach 56% by 2030 (Estes et al., 2018). The etiology of NAFLD involves multiple potential factors, the most common risk factors are overweight, type 2 diabetes mellitus (T2DM), high cholesterol, and metabolic syndrome (Liu et al., 2020). However, sleep-related problems have attracted less attention than other factors. Sleep-related problems involve a set of traits, including sleep duration, insomnia, nap during day, and easiness of getting up in the morning. Studies have shown that the traits are associated with various health effects (Richmond et al., 2019; Zheng et al., 2019; Cai et al., 2020; Park et al., 2020; Titova et al., 2020).
Sleep-related problems can disrupt the circadian clock and thereby the body metabolism (Sharma and Kavuru, 2010). Approximately 90% of patients with NAFLD have more than one feature of metabolic syndrome (Paschos and Paletas, 2009). Therefore, we hypothesized an association between sleep traits and NAFLD. However, there is limited information on the association between sleep traits and NAFLD. Previous study indicated that sleep disturbance was common in patients with NAFLD (Akram et al., 2021). In addition, obstructive sleep apnea, characterized by snoring and daytime naps, can influence the histological severity of NAFLD (Musso et al., 2013; Orr et al., 2020). Several prior studies have shown that both short and long sleep (Liu et al., 2016; Peng et al., 2017; Kim et al., 2018; Okamura et al., 2019), insomnia (Wijarnpreecha et al., 2017), daytime naps of more than 0.5 h (Peng et al., 2017) and snoring (Wang et al., 2020) were associated with an increased risk of NAFLD. However, most studies have focused on sleep duration (Peng et al., 2017; Kim et al., 2018; Okamura et al., 2019), while little has been reported on other sleep traits and NAFLD. Moreover, existing evidence on sleep traits and NAFLD was predominantly from cross-sectional studies with a limited number of participants and may be biased with obesity, type 2 diabetes mellitus (T2DM), and other potential confounders, while none of them implicated a clear causal effect of sleep traits on NAFLD.
The MR method using genetic variants as instrumental variables (IVs) for risk factors has been applied to examine the causal relationship between the risk factors and diseases. As the genotypes are robust and developed before exposure factors, MR is less susceptible to measurement errors, confounding, and reverse causation than conventional observational studies (Smith et al., 2007). In general, a rational MR design could provide more reliable evidence than observational studies under specific assumptions for IVs (Bowden et al., 2015; Zuccolo and Holmes, 2017), which can be used to guide clinical practice. This study conducted rigorous screening to ensure that the genetic variants met the central assumptions (Bowden et al., 2015). Furthermore, since many aspects of sleep are heritable and genes influence traits such as snoring and insomnia, genetic variants could empower the causal inference by reducing the potential confounding.
There is a growing interest in understanding whether different sleep traits could serve as etiological factors for NAFLD. Given the scarcity of information on the relationship between sleep traits and NAFLD, we investigated the association between seven sleep traits and NAFLD. We conducted an observational association analysis in the UK Biobank. To disentangle the causation between sleep traits and NAFLD, we combined external genome-wide association study (GWAS) summary data to conduct a bidirectional two-sample MR analysis.
Methods
Study Population
The individual-level analyses are based on data from the UK Biobank, which is a population-based prospective cohort comprising epidemiological and genetic data of 502,505 individuals aged between 37 and 73 years recruited across the United Kingdom between 2006 and 2010 (Sudlow et al., 2015). We included available information on seven self-reported sleep traits (sleep duration, chronotype, insomnia, nap during day, easiness of getting up in the morning, snoring, and narcolepsy) from 409,605 European ancestry individuals in the UK Biobank. Because sleep apnea is accompanied by snoring, trouble getting up in the morning, and interference with sleep duration, participants diagnosed with sleep apnea were excluded from this study. In addition, participants with missing covariates were excluded from the analysis. NAFLD was defined based on diagnosis codes from hospitalization records (data fields 41,270 and 41,271) following previous criteria (Bianco et al., 2020). Individuals with ICD-9 code 571.8 and ICD-10 code K76 but without hepatitis B or C infection or other specific liver diseases were characterized as NAFLD cases (Liu et al., 2020). Individuals with ICD-10 code G47.3 were characterized as apnea (Han et al., 2021).
The GWAS summary statistics of sleep traits were obtained from the UK Biobank and extracted from the MR Base platform, including sleep duration (n = 460,099), easiness of getting up in the morning (n = 461,658), nap during day (n = 337,074), and insomnia (n = 337,074). The GWAS summary statistics of NAFLD included 1,483 biopsied NAFLD cases and 17,781 controls and were downloaded from the GWAS Catalog (https://www.ebi.ac.uk/gwas/). The characteristics of the GWASs are listed in Supplementary Table S1. The study flowchart is showed in Supplementary Figure S1.
Participants enrolled in the UK Biobank signed the consent forms. This study was conducted under application number 58484.
Sleep Traits
Sleep traits were collected using a standardized touchscreen questionnaire in the UK Biobank (Bycroft et al., 2018). Sleep duration was assessed in the question “about how many hours do you get in every 24 h? (Please include naps).” Binary variables for short sleep duration (<7 h vs. 7–8 h) and long sleep duration (>8 h vs. 7–8 h) were also derived. Easiness of getting up in the morning was assessed in the question “On an average day, how easy do you find getting up in the morning?” with one of six possible answers: “Not at all easy,” “Not very easy,” “Fairly easy,” “Very easy,” “Do not Know,” and “Prefer not to answer.” Chronotype was assessed in the question “Do you consider yourself to be?” with one of the six possible answers: “Definitely a ‘morning’ person,” “More a ‘morning’ than ‘evening’ person,” “More of an ‘evening’ than a ‘morning’ person,” “Definitely an ‘evening’ person,” “Do not know” and “Prefer not to answer.” Nap during day was assessed in the question “Do you have a nap during day?” with one of the four possible answers: “Never/rarely,” “Sometimes,” “Usually,” and “Prefer not to answer.” Insomnia was assessed in the question “Do you have trouble falling asleep at night or do you wake up in the middle of the night?” with one of four possible answers “Never/rarely,” “Sometimes,” “Usually,” and “Prefer not to answer.” Snoring was assessed in the question “Does your partner or a close relative or friend complain about your snoring?” with one of four possible answers “Yes,” “No,” “Do not know,” and “Prefer not to answer.” Narcolepsy was assessed in the question “How likely are you to doze off or fall asleep during the daytime when you don’t mean to? (e.g., when working, reading, or driving)” with one of five possible answers “Never/rarely,” “Sometimes,” “Often,” “Prefer not to answer,” and “Do not know.”
Genetic Variants
Single nucleotide polymorphisms (SNPs) associated with insomnia and NAFLD identified in the published largest GWASs (Supplementary Tables S2, S3) were employed as IVs in the bidirectional MR analysis (Dashti et al., 2019; Jones et al., 2019; Lane et al., 2019; Anstee et al., 2020).
All of the SNPs that we employed as IVs were robustly and independently (the physical distance between each gene was greater than 10,000 KB and linkage disequilibrium (LD) R2 < 0.01) associated with sleep traits and NAFLD (p < 5 × 10–8) and had a minor allele frequency greater than 0.01 in the GWAS. A total of 57 and 12 target SNPs were utilized as the IVs for insomnia and NAFLD, respectively. If target SNPs were not available in the outcome GWAS summary statistics, we used the proxy SNPs that were in high LD (r2 > 0.8) with the SNPs of interest. The European samples from the 1,000 Genomes Project reference panel were adopted to estimate LD between the chosen SNPs. We then harmonized the direction of the effects between exposure and outcome associations.
Statistical Analysis
Observational Analysis
Potential confounders were identified by reviewing published articles (Kasturiratne et al., 2013; Mansour et al., 2019; Orr et al., 2020). Alanine aminotransferase (ALT), glutamic transaminase (AST), waist circumference, hip circumference, body mass index (BMI), T2DM, hypertension, high-density lipoprotein (HDL), low-density lipoprotein (LDL), triglyceride (TG), total cholesterol (TC), glucose, and smoking status were included. Continuous variables are presented as mean ± standard deviation, and categorical variables are reported as numbers and percentages. Chi-square test, Student’s t-test, and Fisher’s exact test were employed to estimate the differences as appropriate. A generalized additive model was applied to plot the association between sleep duration (<3 and >15 h was excluded) and the NAFLD odds.
A separate multivariable Cox proportional hazards model was used to estimate the association between the seven sleep traits and NAFLD and test the trend separately. In the Cox proportional hazards model, covariates were set in three ways: model 1 was adjusted for age and sex, and model 2 was adjusted for age, sex, BMI, T2DM, and hypertension. Model 3 was fully adjusted for 15 potential confounders identified in the literature. Considering the multiple testing, we used the false-positive rate (FDR) method to adjust the p-value in the multivariable regression analyses, and FDR-adjusted p-value under 0.05 was considered statistically significant in observational analyses.
Mendelian Randomization Analysis
We conducted a bidirectional two-sample MR analysis to assess whether sleep traits have causal effects on NAFLD, and conversely, if NAFLD has a causal effect on sleep traits. There are various methods to estimate the effect in a two-sample MR analysis, each of which has a different requirement for IVs (Bowden et al., 2015; Bowden et al., 2016a; Bowden et al., 2016b). The inverse variance weighted (IVW) method with multiplicative random effects is an efficient analysis method with valid IVs, and it accounts for heterogeneity in the variant-specific causal estimates (Lin et al., 2021). In this case, we adopted the IVW method as the main method to estimate the effect between sleep traits and NAFLD, and adopted the MR-Egger, weighted median, simple mode, and weighted mode methods as sensitivity analyses. We tested the MR assumption that the variants were strongly associated with sleep traits and NAFLD by calculating the mean F statistic across all IVs for insomnia and NAFLD. An F statistic greater than 10 is an acceptable threshold for considering a genetic variant to be strong enough for MR analyses (Burgess and Thompson, 2011; Bull et al., 2020).
Sensitivity Analysis
Cochran’s Q test was applied to estimate the heterogeneity of individual genetic variants in both the MR-Egger (Rees et al., 2017) and IVW (Burgess and Thompson, 2015) methods. Heterogeneity was considered absent if the p-value was greater than 0.05.
The MR-Egger intercept test was used to estimate the directional pleiotropy. In addition, we considered the absence of pleiotropic effects if the intercept was not significantly different from zero (p > 0.05) (Zhang et al., 2020). We also applied the MR-Pleiotropy Residual Sum and Outlier (MR-PRESSO) global test (Verbanck et al., 2018) to evaluate horizontal pleiotropy and identify outlier variants.
The leave-one-out analysis by MR-Egger and IVW was performed to test whether the overall results were driven from an individual SNP by removing single SNPs. In addition, the IVW and MR-Egger methods were used to perform a sensitivity analysis of SNPs. Therefore, the MR-Egger estimate is unbiased, provided that the genetic instrument is not dependent on pleiotropic effects.
All statistical analyses were performed using R version 3.6.0 (R Development Core Team, Vienna, Austria). We mainly used the Mendelian Randomization (Yavorska and Burgess, 2017), Two Sample MR (Hemani et al., 2018), and the MR-PRESSO (Verbanck et al., 2018) packages.
Results
Baseline Characteristics of Participants
A total of 1,029 NAFLD cases and 272,063 healthy participants were included in the final analyses. The baseline characteristics of the study population are shown in Table1. Briefly, the NAFLD cases were older (mean age, 57.97 vs. 56.80 years) and had a higher mean BMI (31.22 vs. 27.32) than the non-NAFLD cases. In addition, the NAFLD cases showed a higher value of ALT, AST, waist circumference, hip circumference, BMI, HDL, LDL, TG, TC, glucose, and HbA1c than the healthy controls and presented a higher prevalence of current smoking, T2DM, and primary hypertension than non-NAFLD individuals.
Observational Analysis
The multivariable regression model adjusted for age and sex showed a significant association between the seven sleep traits and NAFLD (Figure 1; Table 2). After full adjustment, NAFLD was significantly associated with long sleep (HR, 1.22; 95% CI, 1.01–1.48), getting up in the morning not very easy (HR, 1.33; 95% CI, 1.02–1.74) and not at all easy (HR, 1.51; 95% CI, 1.27–1.78), usually nap during day (HR, 1.28; 95% CI, 1.02–1.61), usually insomnia (HR, 1.24; 95% CI, 1.04–1.48), and sometimes insomnia (HR, 1.46; 95% CI, 1.21–1.75) (Table 2). After the FDR was adjusted, NAFLD was significantly associated with getting up in morning not at all easy and usually insomnia (FDR adjusted p < 0.007). Furthermore, the easiness of getting up in the morning and insomnia showed a dose–response association with NAFLD (Ptrend <0.05). The general additive model plotted a U-shaped association between sleep duration and the probability of prevalent NAFLD (Figure 2).
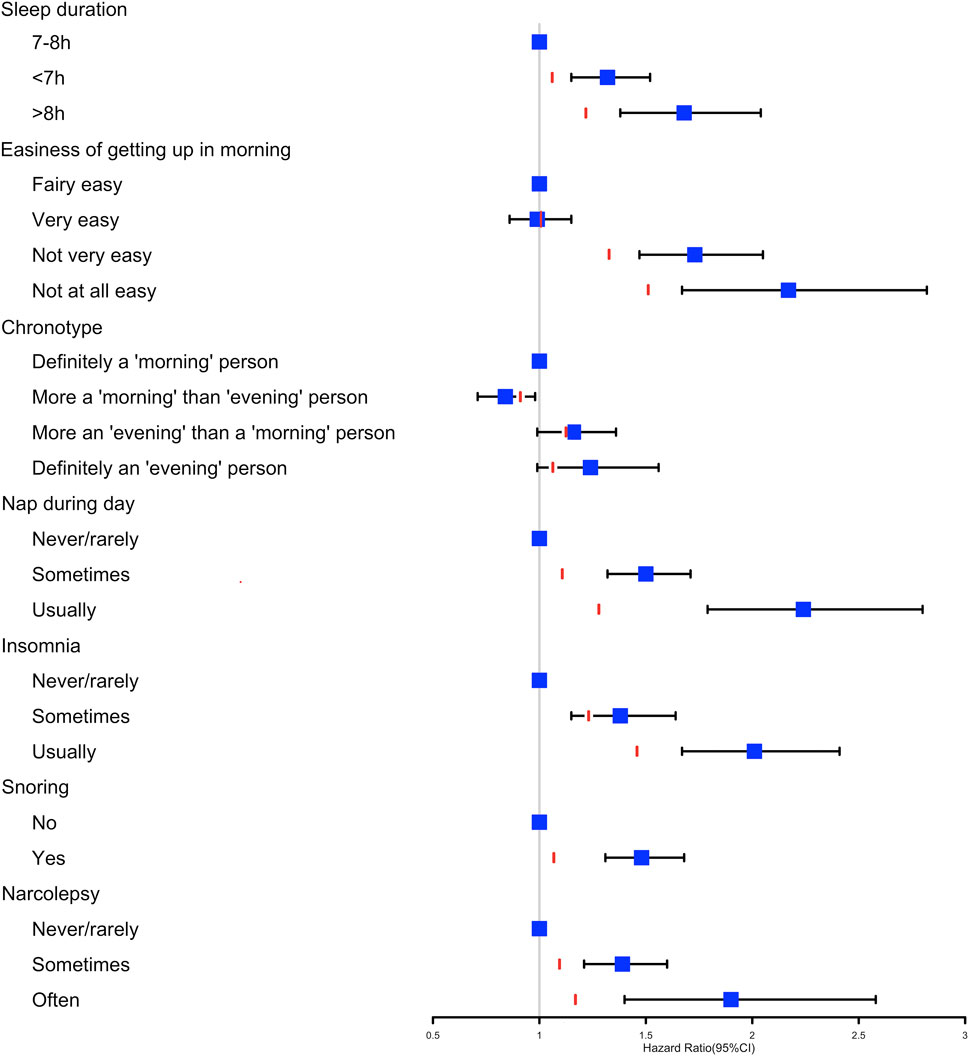
FIGURE 1. Cox proportional hazards model adjusted by age and sex analysis used to determine the association between the seven sleep traits and nonalcoholic fatty liver disease. The red line represents the HR estimates of the fully adjusted model 3 for each sleep variable.
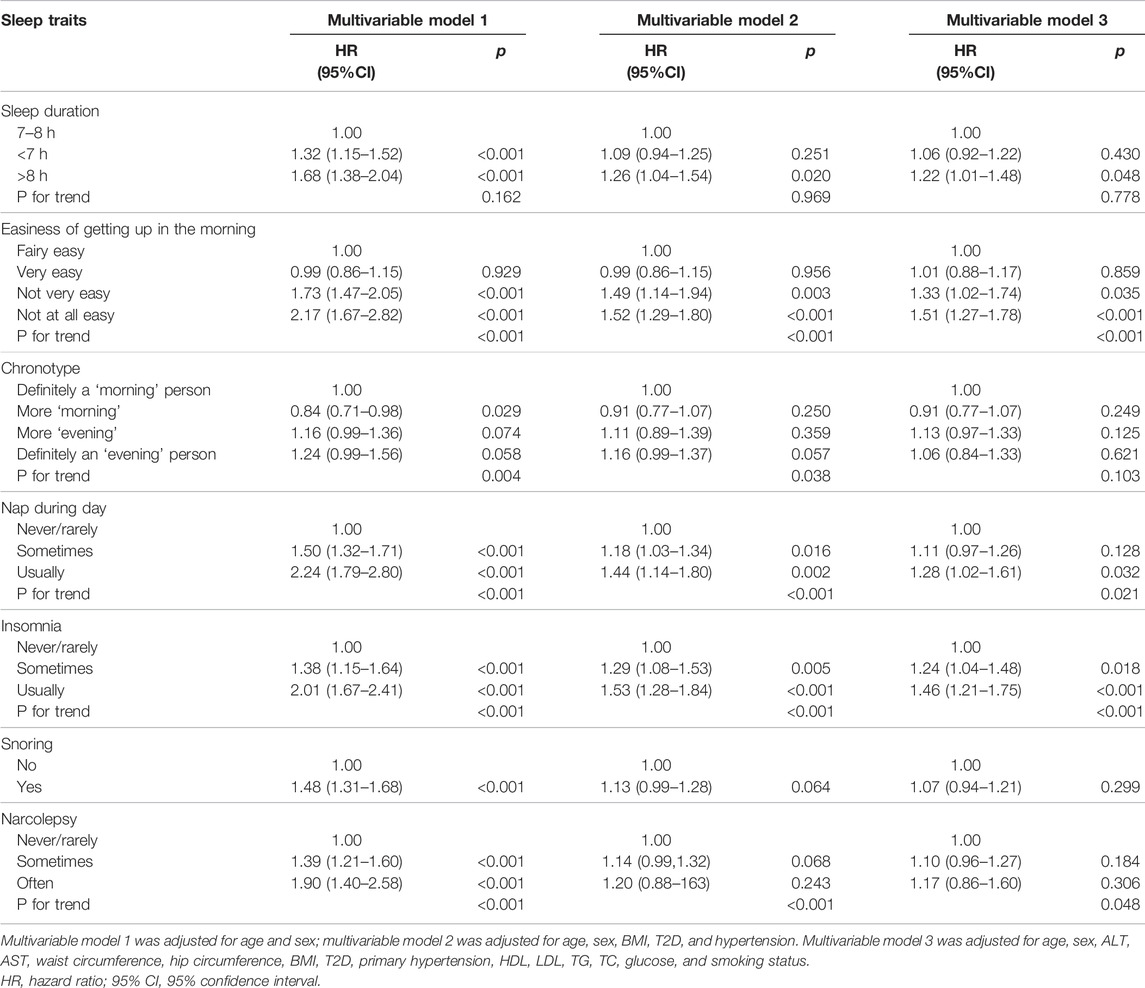
TABLE 2. Multivariable Cox proportional hazards model analysis for risk of nonalcoholic fatty liver disease and sleep traits (7 × 3 different models).
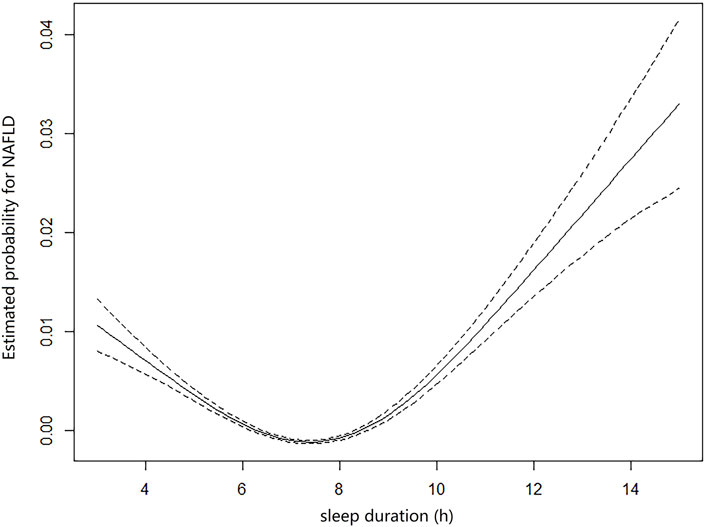
FIGURE 2. General additive model plotting the U-shaped association between self-reported sleep duration and probabilities of prevalent NAFLD (sleep duration <3 h and >15 h were excluded).
Mendelian Randomization Analysis
After harmonization of the SNP effects, 47 and 9 SNPs were employed as IVs for insomnia and NAFLD, respectively, in the bi-directional MR analysis. The MR-PRESSO outlier test identified no outlier SNPs The F statistics of insomnia and NAFLD were 31.802 and 55.156, respectively, indicating that the none of our IVs was subject to weak instrument bias, as each F statistic was >10 (Supplementary Table S4) (Burgess and Thompson, 2011; Bull et al., 2020).
In the MR analyses of sleep traits on NAFLD, no genetic evidence of causal effects of insomnia on NAFLD was identified (Table 3). In the MR analyses of NAFLD on sleep traits, NAFLD had a causal effect on easiness of getting up in the morning (IVW; OR, 0.995; 95% CI, 0.990–0.999; p = 0.033) and insomnia (IVW; OR, 1.006; 95% CI, 1.001–1.011; p = 0.024) (Table 3). Cochran’s Q test and MR-Egger intercept revealed no pleiotropic effect or horizontal heterogeneity that existed in the bi-directional MR analyses (Supplementary Table S4). The results of the leave-one-out analysis suggested that no individual SNP was responsible for driving the observed associations (Supplementary Figures S2–4).
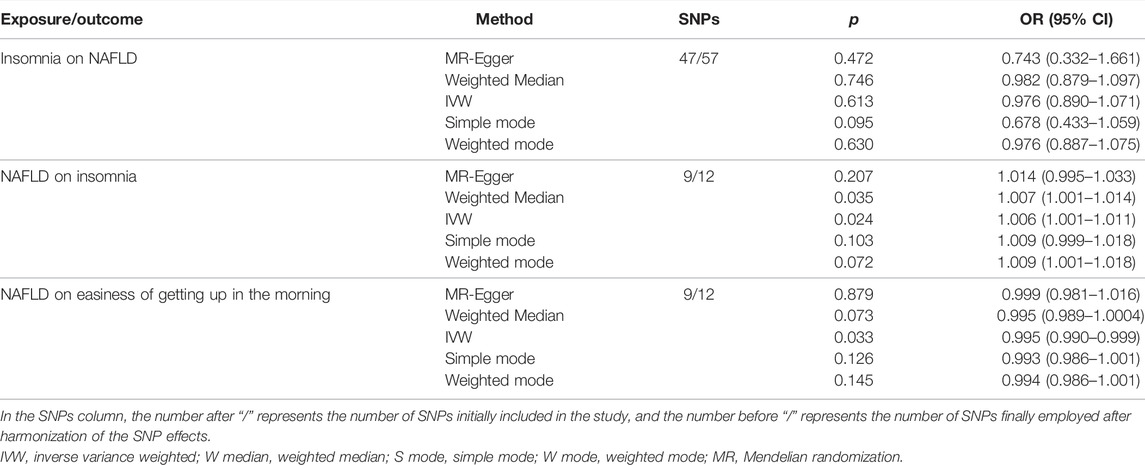
TABLE 3. Association between genetically predicted insomnia on nonalcoholic fatty liver and genetically predicted nonalcoholic fatty liver on insomnia and easiness of getting up in the morning used as instrument variables.
Discussion
This study included seven sleep traits in the UK Biobank, covering almost all sleep phenotypes and comprehensively evaluated the observational associations between seven sleep traits and NAFLD. Meanwhile, we used bidirectional two-sample MR to estimate the bidirectional causal effects between sleep traits (ease of getting up in the morning and insomnia) and NAFLD, providing more reliable causal evidence.
Previous studies suggested that short sleep and long sleep durations increased the risk of NAFLD (Liu et al., 2016; Peng et al., 2017; Kim et al., 2018; Okamura et al., 2019), while sleeping 6–8 h per day lowered the risk of liver steatosis (Mikolasevic et al., 2020). However, the effects were inconsistent across studies (Miyake et al., 2015; Liu et al., 2016; Shen et al., 2016). This may be because previous studies are biased with potential confounding and limited sample size. Our study adopted individual-level data from the UK Biobank with a large sample size and strict data quality control, with findings that 7–8 h of sleep was associated with the lowest risk of NAFLD.
The present study used multivariable regression approach to adjust different covariates, which could provide independent associations. Findings of the observational analyses showed that trouble getting up in the morning and usually insomnia were significantly associated with an increased risk of NAFLD. Moreover, the observational associations were consistent with the causal effects of the MR analyses. The MR analyses highlight the importance of genetically predicted NAFLD on insomnia and easiness of getting up in the morning, which provides a possible target for further mechanism research. People who suffer from insomnia often have trouble getting up in the morning (Danielsson et al., 2019). However, trouble getting up in the morning received much less attention and a lack of drug development than insomnia, which might be an important direction for future research.
Our analysis also identified some clinically significant sleep traits, including narcolepsy, snoring, chronotype, napping during day, and narcolepsy. The regression model adjusted for age and sex showed that evening preference, snoring, long sleep, nap during day, and narcolepsy individuals had a higher risk of NAFLD, and the correlation disappeared after adjusting for the 15 potential confounders. However, given the results of previous studies and the correlation between the sleep traits (Peng et al., 2017; Alshaer et al., 2019; Wang et al., 2020), evening preference, snoring, long sleep, nap during day, and narcolepsy remain clinically important for NAFLD. A previous study showed that a longer daytime napping duration was associated with NAFLD in a dose-dependent manner (Peng et al., 2017). Our findings corroborate that daytime napping is associated with NAFLD risk, but because napping during day increases sleep duration, it is not clear whether daytime naps directly affect NAFLD or affect sleep duration. Unfortunately, the duration of daytime naps was not available in the UK Biobank, precluding further analyses.
Many previous reports have employed MR studies to determine the causal relationship between sleep-related problems and various diseases, such as cardiovascular (Zheng et al., 2019) and several types of cancer (Richmond et al., 2019; Titova et al., 2020). However, the existing evidence on sleep traits and NAFLD are predominantly from cross-sectional studies involving reverse causality (Musso et al., 2013; Liu et al., 2016; Peng et al., 2017; Wijarnpreecha et al., 2017; Kim et al., 2018; Okamura et al., 2019; Wang et al., 2020), and no MR study has assessed the causal relationship between sleep traits and NAFLD, regardless of increasing sleep-related diseases. Our study used a bi-directional MR approach to examine the causal relationships between NAFLD and sleep traits filling the blanks that current evidence of sleep traits and NAFLD involve reverse causation (Okamura et al., 2019).
There are some strengths and limitations to our study. The major strength of this work is the application of bidirectional MR to explore the causation between sleep traits and NAFLD, which could avoid reverse causation and potential false associations caused by unmeasured confounders. Second, all the IVs we used are from the largest published GWAS, and none of our IVs has been subject to weak instrument bias (F statistic >10). Meanwhile, we performed multiple methods to examine pleiotropy and heterogeneity to ensure that the IVs met the core assumptions. Third, we implemented a variety of sensitivity analyses to reduce potential bias and winner’s curse (Munafò et al., 2018; Hughes et al., 2019) and applied multiple methods to estimate the causal effect as cross-validation. One limitation is that multivariable regression might be with overfitting problems. But our study with a large sample size could, to some extent, avoid overfitting. Another potential limitation is that the sleep traits used in this study are self-reported. Therefore, participants may have misunderstood the questions. Insomnia symptoms were not necessarily associated with clinical insomnia (Richmond et al., 2019).
In conclusion, our findings demonstrate that trouble getting up in the morning and insomnia are associated with an increased risk of NAFLD. The MR analysis revealed that the associations were causal of NAFLD on easiness of getting up in the morning and insomnia. Our study has significant implications for understanding the causal relationship between sleep traits and NAFLD; more research on their pathways and interactions are highly warranted.
Data Availability Statement
The original contributions presented in the study are included in the article/Supplementary Material, and further inquiries can be directed to the corresponding authors.
Author Contributions
HF, XC, and TZ conceived of the study design. HF, ZL, XZ (3rd author), and HY performed the statistical analysis. HF and ZL wrote the manuscript. All authors provided critical revisions of the draft and approved the submitted draft. All authors gave final approval of the version to be published and have contributed to the manuscript. TZ had the final responsibility for the decision to submit for publication. The corresponding author attests that all listed authors meet authorship criteria and that no others meeting the criteria have been omitted.
Funding
This work was supported by the National Science and Technology Fundamental Resources Investigation Program of China (2019FY101103); National Natural Science Foundation of China (grant numbers: 81772170, 91846302, 82073637, and 82003548); the National Key Research and Development Program of China (grant numbers: 2017YFC0907000, 2017YFC0907500, 2017YFC0211700, and 2019YFC1315804); key basic research grants from the Science and Technology Commission of Shanghai Municipality (grant number: 16JC1400500); the Shanghai Municipal Science and Technology Major Project (No. 2017SHZDZX01); Three-Year Action Plan for Strengthening Public Health System in Shanghai (grant number: GWV-10.2-YQ32); Innovation Grant from Science and Technology Commission of Shanghai Municipality, China (grant number: 20ZR1405600); and Local Innovative and Research Teams Project of Guangdong Pearl River Talents Program (2017BT01S131).
Conflict of Interest
The authors declare that the research was conducted in the absence of any commercial or financial relationships that could be construed as a potential conflict of interest.
Publisher’s Note
All claims expressed in this article are solely those of the authors and do not necessarily represent those of their affiliated organizations, or those of the publisher, the editors, and the reviewers. Any product that may be evaluated in this article, or claim that may be made by its manufacturer, is not guaranteed or endorsed by the publisher.
Acknowledgments
We sincerely appreciate the great work of the UK Biobank collaborators.
Supplementary Material
The Supplementary Material for this article can be found online at: https://www.frontiersin.org/articles/10.3389/fgene.2022.792558/full#supplementary-material
References
Akram, S. T., Ewy, M. W., and Said, A. (2021). Sleep Disruption in Nonalcoholic Fatty Liver Disease: What Is the Role of Lifestyle and Diet? Eur. J. Gastroenterol. Hepatol. 33 (1S), e308–e312. doi:10.1097/meg.0000000000002049
Alshaer, H., Hummel, R., Mendelson, M., Marshal, T., and Bradley, T. D. (2019). Objective Relationship between Sleep Apnea and Frequency of Snoring Assessed by Machine Learning. J. Clin. Sleep Med. 15, 463–470. doi:10.5664/jcsm.7676
Anstee, Q. M., Darlay, R., Cockell, S., Meroni, M., Govaere, O., Tiniakos, D., et al. (2020). Genome-wide Association Study of Non-alcoholic Fatty Liver and Steatohepatitis in a Histologically Characterised Cohort☆. J. Hepatol. 73, 505–515. doi:10.1016/j.jhep.2020.04.003
Bianco, C., Jamialahmadi, O., Pelusi, S., Baselli, G., Dongiovanni, P., Zanoni, I., et al. (2020). Non-invasive Stratification of Hepatocellular Carcinoma Risk in Non-alcoholic Fatty Liver Using Polygenic Risk Scores. J. Hepatol. 74 (4), 775–782. doi:10.1016/j.jhep.2020.11.024
Bowden, J., Del Greco M, F., Minelli, C., Davey Smith, G., Sheehan, N. A., and Thompson, J. R. (2016). Assessing the Suitability of Summary Data for Two-Sample Mendelian Randomization Analyses Using MR-Egger Regression: the Role of the I2 Statistic. Int. J. Epidemiol. 45, 1961–1974. doi:10.1093/ije/dyw220
Bowden, J., Davey Smith, G., and Burgess, S. (2015). Mendelian Randomization with Invalid Instruments: Effect Estimation and Bias Detection through Egger Regression. Int. J. Epidemiol. 44, 512–525. doi:10.1093/ije/dyv080
Bowden, J., Davey Smith, G., Haycock, P. C., and Burgess, S. (2016). Consistent Estimation in Mendelian Randomization with Some Invalid Instruments Using a Weighted Median Estimator. Genet. Epidemiol. 40, 304–314. doi:10.1002/gepi.21965
Bull, C. J., Bell, J. A., Murphy, N., Sanderson, E., Davey Smith, G., Timpson, N. J., et al. (2020). Adiposity, Metabolites, and Colorectal Cancer Risk: Mendelian Randomization Study. BMC Med. 18, 396. doi:10.1186/s12916-020-01855-9
Burgess, S., and Thompson, S. G. (2011). Avoiding Bias from Weak Instruments in Mendelian Randomization Studies. Int. J. Epidemiol. 40, 755–764. doi:10.1093/ije/dyr036
Burgess, S., and Thompson, S. G. (2015). Multivariable Mendelian Randomization: the Use of Pleiotropic Genetic Variants to Estimate Causal Effects. Am. J. Epidemiol. 181, 251–260. doi:10.1093/aje/kwu283
Bycroft, C., Freeman, C., Petkova, D., Band, G., Elliott, L. T., Sharp, K., et al. (2018). The UK Biobank Resource with Deep Phenotyping and Genomic Data. Nature 562, 203–209. doi:10.1038/s41586-018-0579-z
Cai, H., Liang, J., Liu, Z., Fang, L., Zheng, J., Xu, J., et al. (2020). Causal Effects of Sleep Traits on Ischemic Stroke and its Subtypes: A Mendelian Randomization Study. Nss Vol. 12, 783–790. doi:10.2147/nss.s265946
Danielsson, K., Sakarya, A., and Jansson-Fröjmark, M. (2019). The Reduced Morningness-Eveningness Questionnaire: Psychometric Properties and Related Factors in a Young Swedish Population. Chronobiology Int. 36, 530–540. doi:10.1080/07420528.2018.1564322
Dashti, H. S., Jones, S. E., Wood, A. R., Lane, J. M., van Hees, V. T., Wang, H., et al. (2019). Genome-wide Association Study Identifies Genetic Loci for Self-Reported Habitual Sleep Duration Supported by Accelerometer-Derived Estimates. Nat. Commun. 10, 1100. doi:10.1038/s41467-019-08917-4
Estes, C., Anstee, Q. M., Arias-Loste, M. T., Bantel, H., Bellentani, S., Caballeria, J., et al. (2018). Modeling NAFLD Disease burden in China, France, Germany, Italy, Japan, Spain, United Kingdom, and United States for the Period 2016-2030. J. Hepatol. 69, 896–904. doi:10.1016/j.jhep.2018.05.036
Han, X., Lee, S. S.-Y., Ingold, N., McArdle, N., Khawaja, A. P., MacGregor, S., et al. (2021). Associations of Sleep Apnoea with Glaucoma and Age-Related Macular Degeneration: an Analysis in the United Kingdom Biobank and the Canadian Longitudinal Study on Aging. BMC Med. 19, 104. doi:10.1186/s12916-021-01973-y
Hemani, G., Zheng, J., Elsworth, B., Wade, K. H., Haberland, V., Baird, D., et al. (2018). The MR-Base Platform Supports Systematic Causal Inference across the Human Phenome. eLife 7. doi:10.7554/eLife.34408
Hughes, R. A., Davies, N. M., Davey Smith, G., and Tilling, K. (2019). Selection Bias when Estimating Average Treatment Effects Using One-Sample Instrumental Variable Analysis. Epidemiology 30, 350–357. doi:10.1097/ede.0000000000000972
Jones, S. E., Lane, J. M., Wood, A. R., van Hees, V. T., Tyrrell, J., Beaumont, R. N., et al. (2019). Genome-wide Association Analyses of Chronotype in 697,828 Individuals Provides Insights into Circadian Rhythms. Nat. Commun. 10, 343. doi:10.1038/s41467-018-08259-7
Kasturiratne, A., Weerasinghe, S., Dassanayake, A. S., Rajindrajith, S., de Silva, A. P., Kato, N., et al. (2013). Influence of Non-alcoholic Fatty Liver Disease on the Development of Diabetes Mellitus. J. Gastroenterol. Hepatol. 28, 142–147. doi:10.1111/j.1440-1746.2012.07264.x
Kim, D., Kim, H. J., Kushida, C. A., Heo, N.-Y., Ahmed, A., and Kim, W. R. (2018). Short Sleep Duration Is Associated with Abnormal Serum Aminotransferase Activities and Nonalcoholic Fatty Liver Disease. Clin. Gastroenterol. Hepatol. 16, 588–590. doi:10.1016/j.cgh.2017.08.049
Lane, J. M., Jones, S. E., Jones, S. E., Dashti, H. S., Wood, A. R., Aragam, K. G., et al. (2019). Biological and Clinical Insights from Genetics of Insomnia Symptoms. Nat. Genet. 51, 387–393. doi:10.1038/s41588-019-0361-7
Lin, Z., Deng, Y., and Pan, W. (2021). Combining the Strengths of Inverse-Variance Weighting and Egger Regression in Mendelian Randomization Using a Mixture of Regressions Model. Plos Genet. 17, e1009922. doi:10.1371/journal.pgen.1009922
Liu, C., Zhong, R., Lou, J., Pan, A., Tang, Y., Chang, J., et al. (2016). Nighttime Sleep Duration and Risk of Nonalcoholic Fatty Liver Disease: the Dongfeng-Tongji Prospective Study. Ann. Med. 48, 468–476. doi:10.1080/07853890.2016.1193787
Liu, Z., Zhang, Y., Graham, S., Wang, X., Cai, D., Huang, M., et al. (2020). Causal Relationships between NAFLD, T2D and Obesity Have Implications for Disease Subphenotyping. J. Hepatol. 73, 263–276. doi:10.1016/j.jhep.2020.03.006
Mansour, A., Mohajeri-Tehrani, M. R., Samadi, M., Gerami, H., Qorbani, M., Bellissimo, N., et al. (2019). Risk Factors for Non-alcoholic Fatty Liver Disease-Associated Hepatic Fibrosis in Type 2 Diabetes Patients. Acta Diabetol. 56, 1199–1207. doi:10.1007/s00592-019-01374-x
Mikolasevic, I., Domislovic, V., Filipec Kanizaj, T., Radic-Kristo, D., Krznaric, Z., Milovanovic, T., et al. (2020). Relationship between Coffee Consumption, Sleep Duration and Smoking Status with Elastographic Parameters of Liver Steatosis and Fibrosis; Controlled Attenuation Parameter and Liver Stiffness Measurements. Int. J. Clin. Pract. 75 (3), e13770. doi:10.1111/ijcp.13770
Miyake, T., Kumagi, T., Furukawa, S., Hirooka, M., Kawasaki, K., Koizumi, M., et al. (2015). Short Sleep Duration Reduces the Risk of Nonalcoholic Fatty Liver Disease Onset in Men: a Community-Based Longitudinal Cohort Study. J. Gastroenterol. 50, 583–589. doi:10.1007/s00535-014-0989-0
Munafò, M. R., Tilling, K., Taylor, A. E., Evans, D. M., and Davey Smith, G. (2018). Collider Scope: when Selection Bias Can Substantially Influence Observed Associations. Int. J. Epidemiol. 47, 226–235. doi:10.1093/ije/dyx206
Musso, G., Cassader, M., Olivetti, C., Rosina, F., Carbone, G., and Gambino, R. (2013). Association of Obstructive Sleep Apnoea with the Presence and Severity of Non-alcoholic Fatty Liver Disease. A Systematic Review and Meta-Analysis. Obes. Rev. 14, 417–431. doi:10.1111/obr.12020
Okamura, T., Hashimoto, Y., Hamaguchi, M., Obora, A., Kojima, T., and Fukui, M. (2019). Short Sleep Duration Is a Risk of Incident Nonalcoholic Fatty Liver Disease: a Population-Based Longitudinal Study. Jgld 28, 73–81. doi:10.15403/jgld.2014.1121.281.alc
Orr, W. C., Fass, R., Sundaram, S. S., and Scheimann, A. O. (2020). The Effect of Sleep on Gastrointestinal Functioning in Common Digestive Diseases. Lancet Gastroenterol. Hepatol. 5, 616–624. doi:10.1016/s2468-1253(19)30412-1
Park, S., Lee, S., and Kim, Y. (2020). Short or Long Sleep Duration and CKD: A Mendelian Randomization Study. J. Am. Soc. Nephrol. : JASN. doi:10.1681/asn.2020050666
Paschos, P., and Paletas, K. (2009). Non Alcoholic Fatty Liver Disease and Metabolic Syndrome. Hippokratia 13, 9–19.
Peng, K., Lin, L., Wang, Z., Ding, L., Huang, Y., Wang, P., et al. (2017). Short Sleep Duration and Longer Daytime Napping Are Associated with Non-alcoholic Fatty Liver Disease in Chinese Adults. J. Diabetes 9, 827–836. doi:10.1111/1753-0407.12489
Rees, J. M. B., Wood, A. M., and Burgess, S. (2017). Extending the MR-Egger Method for Multivariable Mendelian Randomization to Correct for Both Measured and Unmeasured Pleiotropy. Stat. Med. 36, 4705–4718. doi:10.1002/sim.7492
Richmond, R. C., Anderson, E. L., Dashti, H. S., Jones, S. E., Lane, J. M., Strand, L. B., et al. (2019). Investigating Causal Relations between Sleep Traits and Risk of Breast Cancer in Women: Mendelian Randomisation Study. Bmj 365, l2327. doi:10.1136/bmj.l2327
Sharma, S., and Kavuru, M. (2010). Sleep and Metabolism: an Overview. Int. J. Endocrinol. 2010, 1–12. doi:10.1155/2010/270832
Shen, N., Wang, P., and Yan, W. (2016). Sleep Duration and the Risk of Fatty Liver Disease: A Systematic Review and Meta-Analysis. Sci. Rep. 6, 31956. doi:10.1038/srep31956
Smith, G. D., Lawlor, D. A., Harbord, R., Timpson, N., Day, I., and Ebrahim, S. (2007). Clustered Environments and Randomized Genes: a Fundamental Distinction between Conventional and Genetic Epidemiology. Plos Med. 4, e352. doi:10.1371/journal.pmed.0040352
Sudlow, C., Gallacher, J., Allen, N., Beral, V., Burton, P., Danesh, J., et al. (2015). UK Biobank: an Open Access Resource for Identifying the Causes of a Wide Range of Complex Diseases of Middle and Old Age. Plos Med. 12, e1001779. doi:10.1371/journal.pmed.1001779
Titova, O. E., Michaëlsson, K., Vithayathil, M., Mason, A. M., Kar, S., Burgess, S., et al. (2020). Sleep Duration and Risk of Overall and 22 Site-specific Cancers: A Mendelian Randomization Study. Int. J. Cancer 148 (4), 914–920. doi:10.1002/ijc.33286
Verbanck, M., Chen, C.-Y., Neale, B., and Do, R. (2018). Detection of Widespread Horizontal Pleiotropy in Causal Relationships Inferred from Mendelian Randomization between Complex Traits and Diseases. Nat. Genet. 50, 693–698. doi:10.1038/s41588-018-0099-7
Wang, H., Gao, Q., He, S., Bao, Y., Sun, H., Meng, L., et al. (2020). Self-reported Snoring Is Associated with Nonalcoholic Fatty Liver Disease. Sci. Rep. 10, 9267. doi:10.1038/s41598-020-66208-1
Wijarnpreecha, K., Thongprayoon, C., Panjawatanan, P., and Ungprasert, P. (2017). Insomnia and Risk of Nonalcoholic Fatty Liver Disease: A Systematic Review and Meta-Analysis. J. Postgrad. Med. 63, 226–231. doi:10.4103/jpgm.jpgm_140_17
Yavorska, O. O., and Burgess, S. (2017). MendelianRandomization: an R Package for Performing Mendelian Randomization Analyses Using Summarized Data. Int. J. Epidemiol. 46, 1734–1739. doi:10.1093/ije/dyx034
Younossi, Z. M., Koenig, A. B., Abdelatif, D., Fazel, Y., Henry, L., and Wymer, M. (2016). Global Epidemiology of Nonalcoholic Fatty Liver Disease-Meta-Analytic Assessment of Prevalence, Incidence, and Outcomes. Hepatology 64, 73–84. doi:10.1002/hep.28431
Younossi, Z. M. (2017). Long-Term Outcomes of Nonalcoholic Fatty Liver Disease: From Nonalcoholic Steatohepatitis to Nonalcoholic Steatofibrosis. Clin. Gastroenterol. Hepatol. 15, 1144–1147. doi:10.1016/j.cgh.2017.05.029
Zhang, Y., Liu, Z., Choudhury, T., Cornelis, M. C., and Liu, W. (2020). Habitual Coffee Intake and Risk for Nonalcoholic Fatty Liver Disease: a Two-Sample Mendelian Randomization Study. Eur. J. Nutr. doi:10.1007/s00394-020-02369-z
Zheng, B., Yu, C., Lv, J., Guo, Y., Bian, Z., Zhou, M., et al. (2019). Insomnia Symptoms and Risk of Cardiovascular Diseases Among 0.5 Million Adults. Neurology 93, e2110–e2120. doi:10.1212/wnl.0000000000008581
Keywords: Mendelian randomization, nonalcoholic fatty liver disease, sleep duration, insomnia, easiness of getting up in the morning
Citation: Fan H, Liu Z, Zhang X, Yuan H, Zhao X, Zhao R, Shi T, Wu S, Xu Y, Suo C, Chen X and Zhang T (2022) Investigating the Association Between Seven Sleep Traits and Nonalcoholic Fatty Liver Disease: Observational and Mendelian Randomization Study. Front. Genet. 13:792558. doi: 10.3389/fgene.2022.792558
Received: 10 October 2021; Accepted: 28 March 2022;
Published: 17 May 2022.
Edited by:
Chien-Chang Lee, National Taiwan University Hospital, TaiwanCopyright © 2022 Fan, Liu, Zhang, Yuan, Zhao, Zhao, Shi, Wu, Xu, Suo, Chen and Zhang. This is an open-access article distributed under the terms of the Creative Commons Attribution License (CC BY). The use, distribution or reproduction in other forums is permitted, provided the original author(s) and the copyright owner(s) are credited and that the original publication in this journal is cited, in accordance with accepted academic practice. No use, distribution or reproduction is permitted which does not comply with these terms.
*Correspondence: Xingdong Chen, eGluZ2RvbmdjaGVuQGZ1ZGFuLmVkdS5jbg==; Tiejun Zhang, dGp6aGFuZ0BzaG11LmVkdS5jbg==