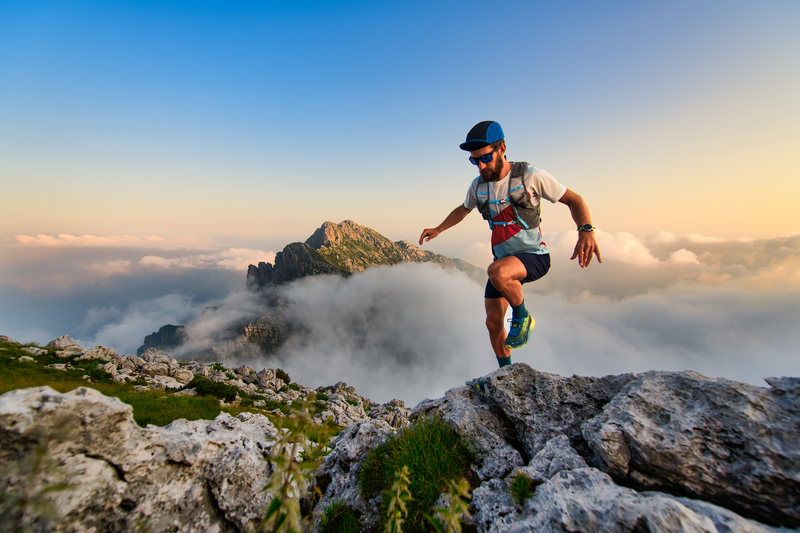
94% of researchers rate our articles as excellent or good
Learn more about the work of our research integrity team to safeguard the quality of each article we publish.
Find out more
REVIEW article
Front. Genet. , 02 June 2022
Sec. Livestock Genomics
Volume 13 - 2022 | https://doi.org/10.3389/fgene.2022.774113
This article is part of the Research Topic Omics Technologies in Livestock Improvement: From Selection to Breeding Decisions View all 30 articles
Conventional animal selection and breeding methods were based on the phenotypic performance of the animals. These methods have limitations, particularly for sex-limited traits and traits expressed later in the life cycle (e.g., carcass traits). Consequently, the genetic gain has been slow with high generation intervals. With the advent of high-throughput omics techniques and the availability of multi-omics technologies and sophisticated analytic packages, several promising tools and methods have been developed to estimate the actual genetic potential of the animals. It has now become possible to collect and access large and complex datasets comprising different genomics, transcriptomics, proteomics, metabolomics, and phonemics data as well as animal-level data (such as longevity, behavior, adaptation, etc.,), which provides new opportunities to better understand the mechanisms regulating animals’ actual performance. The cost of omics technology and expertise of several fields like biology, bioinformatics, statistics, and computational biology make these technology impediments to its use in some cases. The population size and accurate phenotypic data recordings are other significant constraints for appropriate selection and breeding strategies. Nevertheless, omics technologies can estimate more accurate breeding values (BVs) and increase the genetic gain by assisting the section of genetically superior, disease-free animals at an early stage of life for enhancing animal productivity and profitability. This manuscript provides an overview of various omics technologies and their limitations for animal genetic selection and breeding decisions.
Genetic selection and breeding are crucial tools for livestock improvement. They have resulted in genetically superior and disease-free animals with improved production and efficiency in various livestock species (Rexroad et al., 2019; Erasmus and van Marle-Köster 2021). In earlier days, the genetic selection of animals for breeding was primarily based on their phenotypic characteristics, such as production traits and breeding value (BV) estimation. Later, other economic traits, including reproduction and longevity traits, animal health, stress tolerance, disease resistance, animal welfare traits, etc., also became vital components of genetic improvement programs (Brito et al., 2020; Brito et al., 2021). Selective breeding of genetically superior animals ensured rapid genetic progress of production efficiency traits to the next generation. Many breeding techniques have thus, evolved to accrue the desired trait in genetically selected animals to meet the market demand for production and animal welfare (Plieschke et al., 2016).
Several selection indices have been developed for the genetic selection of animals for breeding. However, no single trait is ideal for these selection indices in all populations (Cole et al., 2021). Further, while animals for selective breeding can be identified based on phenotypic recordings, traits that are sex-limited, expressed at a later stage of life, difficult to measure, or have low heritability pose difficulties (Calus et al., 2013). The use of complex statistical models, advanced analytic tools, and new molecular methods may divulge newer traits and help identify animals for efficient genetic selection and breeding with greater accuracy (Stock et al., 2020).
The past three decades have seen tremendous advancements in molecular genetics that have provided a better genetic understanding of quantitative economic traits (Dekkers and Hospital 2002). A number of genes and gene combinations have been found to directly correlate with animal performance and production efficiency (Rexroad et al., 2019; Ruan et al., 2021). Many quantitative trait loci (QTL)—gene loci responsible for trait diversity—have been identified for various production and reproductive traits and used for selection and breeding decisions (Zhang et al., 2021; Al-Sharif et al., 2022). Several genetic markers have also been discovered for use in marker-assisted selection (MAS) of breeding stock (Ma et al., 2021; Raza et al., 2021). More recently, with advancements in high throughput omics technologies, genome selection is becoming widely accepted for the selection of animals for breeding (Tan et al., 2017; Yang et al., 2020). The application of omics tools in livestock improvement may provide a more accurate technology for animal selection and breeding and therefore has become a hot spot of research (Pedrosa et al., 2021; Ruan et al., 2021). This manuscript provides an overview of various omics tools and technologies for their application in livestock selection and improvement programs.
Omics technologies such as genomics, metagenomics, metabolomics, proteomics, transcriptomics, epigenomics, translatomics, etc., can allow rapid and effective detection of subtle phenotypic changes, dietary responses, and innate phenotypic propensities in animals (Mu et al., 2022; Wang et al., 2022). The utilization of omics tools in animal selection and breeding programs is thus, expected to provide an accurate estimation of BV for early selection, reduce generation interval and increase the rate of genetic gain (Figure 1). The word ‘omics’ originates from the suffix ‘-ome’, derived from a Greek word that means “whole”, “all” or “complete”. The suffix “-omics” is frequently used to refer to a field of study in life sciences that emphasizes large-scale high throughput data/information to understand life summed up in “omes” (Yadav, 2007). Several omics tools have been developed in the last two decades to collect and analyze high-throughput data on proteins (proteomics), mRNA transcripts (transcriptomics), gene sequences (genomics), microbial diversity (metagenomics), epigenetic regulation of gene expression (epigenomics), metabolic profile (metabolomics), lipid profile (lipidomics), etc., of a particular cell, tissue, organ or whole organism at a specific time point. The time (temporal) and space (spatial) level information from omics data can be integrated through robust bioinformatics and computational tools to the systems biology level (Odom et al., 2021; Velten et al., 2022). Network modeling of omics data can be used to study the mechanism, relationship, interaction, and function of cells, tissues, organs, and the whole organism at a molecular level in an unbiased manner (Aardema and MacGregor, 2003). More recently, multi-omics has emerged as high-dimensional biology (HBD) for simultaneous study of genetic variations in biological systems at the genes, transcripts, proteins, and metabolites level (Romero et al., 2006; Krassowski et al., 2020).
In the last two decades, a few landmark technological revolutions took place in omics technologies that revolutionized their application in genetic selection for animal breeding. In 2007, the first “high density” panel of bovine genetic markers was released commercially with a set of 54,001 single nucleotide polymorphisms (SNPs). Using such high-density SNP chips, genome-wide association studies (GWAS) demonstrated the link between SNPs and QTLs, such as coat color and presence or absence of horns (Matukumalli et al., 2009). In other studies, high-density SNP chips were shown to be useful in the genetic characterization of pig breeds for preserving their genomic variability (Muñoz et al., 2019). Whole-genome sequencing (WGS) by massively parallel sequencing was yet another breakthrough for detecting molecular signatures for the selection and breeding of animals (Elsik et al., 2009; Bovo et al., 2020). The Bovine Genome Sequencing and Assembly project (Elsik et al., 2009; The Bovine Genome Sequencing and Analysis Consortium et al., 2009; The Bovine HapMap Consortium, 2009) provided a landscape of genome sequence that subsequently led to a paradigm shift in QTL- and candidate gene-based approaches for genetic selection.
Molecular databases of NCBI (United States), EMBL (Europe), and DDBJ (Japan) provide vast information on nucleotide and protein sequences. These databases have been utilized in omics technology for understanding the genomic variability and molecular and physiological basis of economic traits (Wu et al., 2018; Ng et al., 2021). Unfortunately, however, very scant information is available on the precise regulatory networks through which these genes and proteins determine the phenotypic expression of economic traits. Further, a significant unexplained source of variation among phenotypes of various economic traits remains a matter of concern in livestock. Newer machine learning (ML) tools have been developed recently that can be exploited to analyze high throughput omics data, available in databases, for a greater understanding of gene regulatory networks (GRNs) and identification of functional genes by a systems biology approach (Guttula et al., 2020; Guttula et al., 2021; Ng et al., 2021).
Omics technologies can help identify functional SNPs and their prioritizing to increase the accuracy of genetic selection (Chang et al., 2019). They can also be used for selecting animals resistant to production diseases such as mastitis and thereby enhance their productivity (Russell et al., 2012; Bhattarai et al., 2017; Jaiswal et al., 2021). Further, population-level omics (e.g., population genomics) hold tremendous potential for classifying individuals based on allelic diversity and identifying genetically-related individuals (Lippert et al., 2017). Such strategies can help calculate homozygosity and inbreeding coefficients (Ghoreishifar et al., 2020; Sumreddee et al., 2021) for designing appropriate breeding programs to maintain genetic diversity and avoid inbreeding depression (Alemu et al., 2021; Bu et al., 2021). However, while many WGS databases and consortiums have been formed in humans (Zhang et al., 2018) (GenomeAsia 100K Consortium, 2019), no high-resolution database of population-level genetic variants is available for animals.
The genome is defined as the complete set of genetic material present in an organism. The term “genomics” was coined in 1986 by scientists who were naming a new journal (Kuska, 1998), and thus, the era of omics began. The major developments in genomics are the discovery of the genes and genetic codes, polymerase chain reaction (PCR), Genome sequencing by Sanger sequencing or Next Generation Sequencing (NGS), and genome editing tools such as Transcription activator-like effector nuclease (TALEN), Zinc-finer nuclease (ZNF), an Clustered regularly interspaced short palindromic repeats (CRISPR)/CRISPR-associated protein 9 (CRISPR/Cas9) technologies. The field of genomics started gaining popularity after the invention of PCR in the year 1985. Thereafter, MAS- and candidate gene-based approaches for selecting genetically superior animals became very popular and were found to be better than conventional phenotype-based selection and breeding (Williams 2005). Gene map construction was also used for genome sequencing. The gene map construction was initially based on the segregation of enzyme markers across panels of hybrid cell lines. However, with advancements in recombinant DNA (rDNA) technology, denser physical and genetic maps formed an important framework for genome sequencing (Riggs and Gill, 2009). During the early 2000s, most livestock genome sequencing was based on linkage maps using single markers and quantifying one or a few genes by real-time quantitative PCR (qPCR). Elsik and associates, in 2009, published the first bovine genome assembly (Elsik et al., 2009). Since then, rapid progress has been made in developing and using several whole genome-omics tools that have accelerated cattle genetics research (Reverter et al., 2013; Snelling et al., 2013).
The concept of “Genetical Genomics”, which integrates structural and functional genomic data, has evolved with the development of microarray technology for gene expression analysis, which divulged marker genotypes across whole genome. The field of Genetical Genomics has expanded with the availability of high throughput tools for genomic analysis such as high-density (HD) genotyping-chips (Illumina, San Diego, CA), WGS, genotyping by sequencing, and RNA-sequencing (RNAseq) to measure the gene expression in the entire transcriptome (Wickramasinghe et al., 2014). Several SNP chips of 60 K for pig and chicken, 50 K for sheep, and 77 K for cattle have also been developed (Suravajhala et al., 2016). The GWAS studies have become very popular among different livestock species focusing on production and health traits (Shamra et al., 2015). For example, GWAS on female reproduction traits in tropically adapted beef cattle (Hawken et al., 2012), feed efficiency traits in pigs (Do et al., 2013 and 2014), body weight in broilers (Wang et al., 2014), and obesity and metabolic diseases using the pig as a model (Kogelman et al., 2014) have been conducted. The genomic selection is particulary advantagious as it can be used for selecting animals for breeding at an early stage of life without having reference to their own breeding or production records. Studies have shown that genomic selection improved the genetic gain as much as 60–120% in dairy cattle by decreasing genetic interval by 2 years, although the extent of added genetic gain was lower in other livestock species (Table 1). The term “systems genetics” or “systems genomics” was also proposed by Kadarmideen (2014). This branch has a wide range of approaches ranging from relating the individual’s omics levels data to their functional annotation and analysis of signaling pathways by integrating different multi-omic levels data to phenotypes.
TABLE 1. Impact of genomic selectiona.
Transcriptomic methods can be used to compare a biological response to different conditions or treatments or to assess physiological responses to external stimuli (Brannan et al., 2014). Whole transcriptome sequencing is the most widely used method for studying RNA functions, exploring and analyzing the gene structure and function, and revealing intrinsic links between gene expression and life phenomena (Shi and Zhang, 2019). To date, extensive research has been carried out in different livestock species using high-throughput RNAseq technology that has replaced the earlier used Maxam and Gilbert chemical degradation sequencing method. The NGS technologies generate sequence data by producing millions of short DNA fragments in parallel. The template is broken into many smaller fragments by sheering, which are then ligated to adapters to create cDNA libraries by the bridge (e.g., Illumina sequencing) or emulsion (e.g., pyrosequencing) PCR. The clones of cDNA fragments of each library are then sequenced to obtain short reads; the length and number of the reads vary with the specific technology but generally range between 30 and 300 bases, which is shorter than those obtained from Sanger sequencing (Ghaffari et al., 2013). NGS has led to the characterization and quantification of many omics, including genomics (DNA sequencing), transcriptomics (RNA and cDNA sequencing), and epigenomics (ChiP-seq and DNA methylation analysis). More recently, third-generation sequencing methods involving single-molecule real-time (SMRT) sequencing have emerged (Sahoo et al., 2021a; Sahoo et al., 2021b). These newer SMRT sequencing methods do not require PCR amplification of templates and hence are devoid of PCR biases. Moreover, the SMRT sequencing approaches generally produce longer reads for better genome assembly and identification of indels (Athanasopoulou et al., 2021). However, SMRT methods such as NanoporeTM and PacBioTM sequencing also offer versatility in terms of rapid time and the transportability of the equipment. Newer techniques such as tunneling currents DNA sequencing, sequencing with mass spectrometry, microscopy-based sequencing, etc., are under development.
The RNAseq has made a revolutionary impact on transcriptome analysis (Mortazavi et al., 2008). RNAseq has major advantages such as a large dynamic range and sensitivity, precise, unbiased quantification of transcripts, and comprehensive coverage of all expressed sequences in a given tissue sample. The direct RNAseq is vital for functional studies to capture the dynamic RNA population under different environmental conditions (Athanasopoulou et al., 2021). It has revolutionized gene annotation, which was hitherto very difficult with genome sequencing. The RNAseq also finds application in analyzing molecular features such as alternate isoforms, splice variants, fusion transcripts, RNA editing, etc. (Li and Wang 2021). Combination of genome sequencing with RNAseq can be utilized to interpret mutations on regulatory regions of genes, which do not produce an obvious effect on the protein sequence (Cohen et al., 2020).
Today, very accurate and efficient sequencing platforms are available, which can distinguish closely related transcripts from each other (Marguerat and Braga-Neto, 2015). Therefore, RNAseq has become very popular for the identification and quantification of splice variants, fused transcripts, and mutants. In RNAseq technology, messenger RNAs are first randomly fragmented into small pieces by sheering and converted to a library of complementary DNA (cDNA) fragments. These cDNA fragments are then amplified and sequenced in parallel and mapped to a given region of the target genome. PCR-free cDNA sequencing and direct RNAseq without first-strand cDNA synthesis have also become possible with SMRT technology such as NanoporeTM sequencing. In expression quantification, a count, which is determined by the number of reads mapping to each gene (FPKM or TPM; fragments per kilobase of transcripts per million mapped reads or transcripts per million), is a discrete measure of the corresponding gene expression level (Ghaffariet al., 2013, Li et al., 2012) (Figure 2). The differentially expressed genes (DEGs) between two samples can be obtained by transcript compilation with gene annotation file followed by gene identification and differential expression analysis based on FPKM or TPM values (Alessandrì et al., 2019). Functional analysis of DEGs by bioinformatics tools revealed that the immune and inflammatory responses were the most impacted pathways between purebred and crossbred cattle populations (Moridi et al., 2019). Such type of RNAseq-based transcriptomic studies on animals of high- and low-genetic merit may be helpful for the selection and breeding of elite animals in the future to enhance health, productivity, and profitability.
FIGURE 2. A software pipeline and computational resources used for analysis of RNAseq data. Each type of RNAseq has distinct requirements and challenges but there is a common workflow/pipeline.
Canovas et al. (2014) integrated the RNAseq data with GWAS and bovine transcriptional factors in multiple tissues from pre- and post-pubertal cattle and constructed co-expression GRNs, which revealed genes and their complex interactions during puberty in cattle. Therefore, early selection of individuals based on multi-omics data from early sexual maturity may help increase the genetic gain by reducing generation intervals. In another study, the resistance or susceptibility of Creole goats to gastrointestinal nematodes was studied by RNAseq (Aboshady et al., 2021). Aboshady et al. (2021) reported that the T-cell receptor signaling pathway was one of the top significant pathways that distinguish the resistant from the susceptible genotype, with 78% of the genes involved in this pathway showing genomic variants in Creole goats. This shows another important example of applying omics for selecting disease-resistant animals.
Wilkins and Williams first described proteomics in the mid-1990s (Speicher, 2004). Proteomics allows analysis of all proteins, including their isoforms, in a particular cell, tissue, or organ at a specific time in a single experiment. Advance proteomic tools can also provide information on various protein isoforms, their quantification, and protein-protein interaction. However, the application of proteomics in livestock research has been limited in the past due to its high cost and lack of optimized protocols for various cell types in different species (Baykalir et al., 2018). Nevertheless, with advancements in new analytical methods and computational tools for the analysis of proteomic data, reports on proteomics studies in animal science are increasing for understanding the animal health status and production and reproduction efficiency (Zhao et al., 2021; Kaya et al., 2022; Ye et al., 2022).
Proteomics techniques range from one-dimensional (ID) gel electrophoresis, two-dimensional (2D) gel electrophoresis, Chromatography (liquid and gas) methods to sophisticated mass spectrometry (MALDI-MS, ESI-MS, LC-MS/MS, MALDI-TOF MS, etc.,), which measures mass-to-charge (m:z ratio) of ionized peptides to identify proteins (Gupta et al., 2009a). In a typical MS experiment, the proteins are isolated from target cells/tissue/organ/biological fluid, separated by 1D or 2D gel electrophoresis or liquid chromatography, and digested by a sequence-specific protease such as trypsin (Figure 3). The trypsin digests are then purified by affinity chromatography or biochemical fractionation and ionized by electronspray ion (ESI), matrix-assisted laser desorption ionization (MALDI), or surface-enhanced laser desorption ionization (SELDI) before being pushed into the mass spectrometer to measure the m:z ratio. The m:z ratio can be measured in quadrupole (Q), ion trap (e.g., quadrupole ion trap or QIT and linear ion trap or LIT), time-of-flight (TOF), quadrupole mass filters (QMF), ion cyclotron resonance (ICR), high-resolution orbitraps, or a hybrid of mass spectrometers. The MS spectra are then matched with protein databases to identify proteins using a variety of algorithms that usually come in-built with the MS machines (e.g., SpectraMillTM) (Gupta et al., 2009b). Several methods have also been developed for relative or absolute quantification (AQUA) of proteins and identification of post-translational modifications by modified MS such as selected reaction monitoring (SRM), isotope labeling of amino acids in cell culture (SILAC), isotope-coded affinity tags (ICAT), isobaric mass tagging (iTRAQ), etc.
The number of proteomics studies associated with reproductive problems has been increased dramatically in the last decade (Peddinti et al., 2011). The research applications in proteomics range from early growth and development to postmortem events important for meat quality (Yarmush and Jayaraman, 2002; Bendixen, 2005). One of the major areas of interest in proteomics is finding out robust protein biomarkers that could be useful in disease surveillance, monitoring the health and wellbeing of animals, elucidating disease mechanisms, and assessing pharmacologic response to therapeutics (Oskoueian et al., 2016) (Muhanguzi et al., 2022). Proteomics can also be applied for different animal products post-harvest like meat, milk, cheese, etc., to identify genetic variants with desirable traits for selection and breeding (Almeida et al., 2015).
Proteomics has also enabled the identification of candidate protein markers of fertility for molecular breeding. The LC-MS/MS analysis of pig sperm revealed eight fertility-related proteins over-represented in Tibetan pigs having heritable adaptation to hypoxic environments (Zhao et al., 2021). In another study, analysis of seminal plasma proteins by LC-MS/MS found a consistent correlation of 1,343 proteins with fertility (Willforss et al., 2021). Thus, identifying fertility markers by proteomics can help identify fertile bulls to reduce non-return rates (NRR) and increase productivity. Proteomic tools can also be harnessed to identify superior genetic variants to dietary response and muscle growth for selective breeding. By a newly described transcriptome-assisted label-free shotgun proteomics method, Mullins et al. (2021) identified 24 differentially abundant proteins in liver tissues from cattle that were fed ad libitum or restricted diet. Identifying protein markers by proteomics could help the selection of genetic variants for compensatory growth upon undernutrition, which may accelerate genetic gain and increase profitability (Mullins et al., 2021).
An emerging area in the application of omics tools is the interrogation of the metabolome. Metabolomics is a comprehensive, qualitative and quantitative study of all the small molecules in an organism (Kalaiselvi et al., 2019; (Lippa et al., 2022). Metabolomic tools are being increasingly used to generate an unbiased global profile of metabolites in samples (i.e., untargeted analysis) or to quantify with high sensitivity a small panel of metabolites (targeted analysis) (Riggs et al., 2017; Evans et al., 2020). In dairy cattle, many potential biomarkers of milk yield and quality have been detected by studying the metabolome of different body fluids (Sun et al., 2015). One advantage of profiling metabolites is exploring the impact of metabolism on systemic health by monitoring the production and further metabolism of compounds present in the diet, digesta, and plasma (Seidel et al., 2014). It can also be used to evaluate feed conversion efficiency, metabolic response of animals to environmental conditions, and estimation of production efficiency and carcass quality traits (Jorge-Smeding et al., 2021; Martin et al., 2021; Artegoitia et al., 2022). Studies have also shown that the heritability of water-soluble compounds such as free amino acids, nucleotides, and sugars in beef was less than 0.30 and varied with animal age (Sakuma et al., 2016). However, these water-soluble compounds were negatively correlated with carcass weight and beef marbling standard at the genetic level. Thus, metabolomics may help identify animals with high carcass quality for breeding. In another study, metabolic profiling of muscle by GC-MS and LC-MS could distinguish grass- and grain-fed cattle with 100% predictive accuracy (Carrillo et al., 2016). These results suggest that metabolic signatures could be a good indicator of animals’ feeding habits and carcass quality and, therefore, could be utilized to select animals with desired traits (Figure 4).
Metabolomic selection is an emerging breeding technology based on nuclear magnetic resonance (NMR) or LC-MS metabolomics (Evans et al., 2020; Lippa et al., 2022). The NMR spectra of biological samples can be analyzed for chemical shifts, peak intensities, and coupling patterns to identify and quantify various metabolites and generate NMR fingerprints of the sample (Figure 4). Metabolomic studies on muscle and fat from cattle, pigs, and poultry have shown tissue- and species-specific differences in metabolites with specific compounds detected in each species (Ueda et al., 2018). The GC-MS could also distinguish between cattle breeds (Ueda et al., 2018). Thus, comparing NMR spectra from different animals such as those from low- and high-performing animals may help identify NMR fingerprints in high-performing animals. Such NMR fingerprints can then be used for the genetic selection of animals for breeding purposes (Figure 4). Metabolomic analysis of muscle from Nellore cattle having high- or low-growth traits revealed that high growth animals had a distinct metabolic profile with a higher concentration of specific metabolites affecting protein and fatty acid metabolism (Cansolo et al., 2020) that can be harnessed for selection of animals for growth.
High-resolution MS (HRMS) can detect metabolites at nano-to the pico-molar concentration of metabolome and, therefore, can provide a better landscape of metabolites than NMR (Goldansaz et al., 2017). The MS is usually combined with separation techniques such as capillary, liquid, or gas chromatography, depending on the polarity and lipophilicity of the metabolites of interest. The separated molecules are ionized by ESI, electron ionization (EI), chemical ionization (CI), or atmospheric pressure CI (APCI) and evaluated for m:z ratio in the mass spectrometer based on TOF, Fourier transformation ion cyclotron resonance (FT-ICR) or orbitrap to obtain structural information for identifying the metabolites. Several databases such as METLIN, Human Metabolome Database (HMDB), and MassBank are available that can be used to match the MS spectra of metabolites for their identification. A number of statistical and bioinformatic tools can then be applied to discover molecular pathways involved in the generation of critical metabolites. Software such as MetaboAnalyst and Kyoto Encyclopedia of Genes and Genomes (KEGG) can be used for multivariate analysis and visualization of metabolic pathways. The correlation analysis between animal performance parameters and metabolic profiles may help identify key metabolic markers of animals’ performance for genetic selection. Metabolomics has also been used in GWAS for metabolite-featured phenotyping and animal breeding (Fontansesi, 2016).
The metabolomic tools can also be combined with molecular breeding tools such as WGS and high-density SNP chips to increase the accuracy of genetic selection and livestock breeding (Wang and Kadarmideen 2020) (Ehret et al., 2015). Given that genomic prediction to predict breeding values based on phenotypic, pedigree, and genomic data is insufficient to describe the genetic potential of animals, incorporating the whole-metabolomic data in the genomic prediction equation may play a crucial role in increasing the genetic gain by increasing the accuracy of selection. The latter is further substantiated by the fact that metabolites represent cells’ ultimate physiological response and thereby represent a link between genotype and phenotype (Wang and Kadarmideen 2020). GWAS-based studies, using SNP chips and LC-MS metabolomics, the identified mechanism underlying the genetic variation in pigs for feed efficiency (Banerjee et al., 2020; Wang and Kadarmideen 2020). Integrating high-density SNP data and metabolite information with predictive value was also found to help improve the accuracy of genetic selection in cattle (Ehret et al., 2015). The power of metabolomics is that it non-invasively detects subtle phenotypic changes, innate phenotypic propensities, and dietary responses in livestock research, breeding, and assessment through new varieties of bio-samples such as semen, amniotic fluid, saliva, and urine.
Wu et al. (2021) found that small metabolite profiling of pig feces by LC-MS metabolomics correlated with their feed efficiency and can be used as a reference for selecting animals with high feed conversion efficiency and responsiveness to new feed additives (Wu et al., 2021). Given that fecal metabolome are reflections of intestinal microbiota, cellular metabolism and digestion/absorption of nutrients in the gut (Zierer et al., 2018; Malheiros et al., 2021), the metabolites present in the feces could indicate their feed conversion efficiency (Wu et al., 2021). Fecal metabolome was also shown to change as a function of stress in beef cattle (Valerio et al., 2020). Thus, metabolic profiling of fecal matter may be used to identify animals with “metabolic fingerprints” that are known to exist in animals of high feed conversion efficiency or tolerance to stress. Such animal can then be selected for breeding purposes. Metabolomics has also been used to study the effect of genetic selection on indirect genetic effects (IGE) in breeding programs (Dervishi et al., 2021). Future metabolomics research may be integrated with multi-omics experiments using various analytical platforms/techniques (e.g., ICP-MS, MSI, and fluxomics) by using more sensitive platforms, such as ESI-MS, to get more accurate information.
Metagenomics is the collection and analysis of genetic material (genomes) from a mixed community of organisms. Metagenomics is an area of considerable research interest, particularly in ruminant animals to study microbial communities in rumen and milk. In metagenomics, genomic sequencing tools are used to identify the complex structure of the rumen microbiota and their changes in response to diet in concert with the host ruminant genome. These rumen microbiotas may influence a range of phenotypes in the host, including feed efficiency, the inflammatory state in the digestive tract, and volume of methane production in the rumen (Morgan et al., 2014; Ritchie et al., 2015). Metagenomics is also the best way to reveal modern species’ phylogenetic and evolutionary relationships with the natives and ancestors of livestock and poultry (Sahu et al., 2017). Other important applications of metagenomics in livestock improvement are to identify the disease-resistant strains for drug of choice and information generation for genotype and environmental interactions for better control over management (Sahu et al., 2017).
A typical metagenomic experiment involves isolation of genomic DNA from microbial population and amplicon sequencing of 16 rRNA hypervariable V3-V4 region of bacteria and/or WGS by NGS (e.g., IlluminaTM sequencing) or the third-generation sequencing [e.g., Oxford Nanopore TechnologyTM (ONT) and PacBioTM] (Figures 5, 6). The DNA reads obtained from WGS data are assembled computationally to obtain larger DNA sequences and identify the operational taxonomic units (OUTs) of the microbes. Statistical tools can then be utilized to estimate richness (number of taxonomic groups) and evenness (distribution of abundances of the groups) of various microbial populations by computing alpha and beta-diversities. A number of tools such as Mothur, QIIME2 (Quantitative Insights Into Microbial Ecology), DADA2 (Divisive Amplicon Denoising Algorithm), Usearch etc. are available for amplicon sequencing of 16 rDNA in bacteria. A typical bioinformatics pipeline and relavent tools for analysis of amplicon sequencing is shown in Figure 5. On the other hand, while WGS allows high analysis of entire community of microbs, including viruses and fungi, they are relatively expensive, time consuming and computationally demanding. A bioinformatic pipeline and tools for WGS analysis of WGS is shown in Figure 6. The details of various metagenomic pipelines for amplicon sequencing and WGS can be seen elsewhere (Florian et al., 2019).
FIGURE 5. A software pipeline for analysis of amplicon sequencing of bacteria. Each type of experiment has distinct requirements and challenges but there is a common workflow/pipeline.
FIGURE 6. A software pipeline for analysis of whole genome metagenomic sequencing data. Each type of experiment has distinct requirements and challenges but there is a common workflow/pipeline.
Metagenomic studies have been used extensively in cattle, pigs, and horses to understand the importance of the microbiome in the gut and mammary microbiome and their relation to feeding efficiency, immunity, and mastitis (Chen C. et al., 2021; Gomez et al., 2021). Metagenomics has shown that gut microbiota can affect feed intake, feed conversion ratio, and production traits such as daily weight gain and back-fat thickness in pigs (Aliakbari et al., 2021; Jiang et al., 2021; Tiezzi et al., 2021). It can also relieve immune stress and help maintain homeostasis in the intestine (Sun et al., 2021). Similarly, parasitic infestations of tapeworm in horses were also found to alter the colonic microbiome (Slater et al., 2021). Such changes in gut microbiota showed implications in individual animals’ performance and metabolic health. An improved understanding of gut microbiota by metagenomics can, therefore, help to genetic selection of animals for better animal health and productivity. The meganomic profile of fecal matter or oral swab may be used to identify animals with “microbial fingerprints” that are known to exist in animals of high genetic merit and can subsequently be used for breeding purposes. Thus, metagenomics may offer a non-invasive means of animal selection and breeding. Metagenomic studies have also revealed that the milk microbiome varies with health status (e.g., mastitis, endometritis, bacteremia, etc.,), age, parity, lactation duration, and feed composition (Bach et al., 2021). Consequently, characterization of milk microbiomes may help identify novel “microbial fingerprints” for healthy mammary glands and genetic selection of healthy dairy animals (Andrews et al., 2019).
Epigenomics is another branch of omics technology that deals with studying epigenetic changes in a cell. Epigenetic changes regulate gene expression without changing the actual DNA sequence. Epigenetic modifications can be altered by external or internal environmental factors such as diet, exercise, drugs, and chemicals and can change gene expression and define specific phenotypes. Mapping epigenomics components in many cell types helped identify millions of putative regulatory elements (Zentner and Henikoff, 2015). A whole-genomic bisulfate sequencing identified breed-specific hypomethylated regions that were associated with male fertility (Chen S. et al., 2021). Epigenomic biomarkers of male fertility were also identified in the genome-wide DNA methylation map of pig testis (Wang and Kadarmideen 2019). However, studies on global-level epigenomics for genetic selection and breeding of animals are very limited.
Omics technologies generate a voluminous amount of complex data in gigabyte to terabyte range (hence, called Big Data) that are difficult to handle by traditional data management tools (Angerer et al., 2017). Expertise from different biological fields, skilled and knowledgeable bioinformaticians, statisticians, and computer scientists are required to analyze and interpret these data (Riggs et al., 2017). The tremendous high-dimensional data resulting from a large number of experimental variables (e.g., physiological state, age, sex, parity, nutritional status, experimental design, etc.) and simultaneous evaluation of multiple genes/proteins/metabolites/transcripts, etc. requires implementation of complex statistical techniques and models to avoid spurious results and misinterpretation of research data. Various open-source and commercial bioinformatics softwares are now available in online and offline modes in R packages of Bioconductor, EMBOSS, Galaxy, Staden, Biophython, Bioconda, Linux, etc., for various omics data analysis (Table 2).
TABLE 2. Overview of some free bioinformatics software for integrating information across several omics techniques.
Data handling is a vital component of analyzing raw data from omics experiments for their correct biological interpretation. Data handling must address issues related to data filtering, imputation, transformation, normalization, quality control, and scaling (Li et al., 2022). Several algorithms and pipelines are now available for the analysis of various omics data, including transcriptomics (Figure 3), proteomics (Figure 4), and metagenomics (Figure 4). However, using one pipeline or tool may yield different results from other pipelines. One approach to avoid this problem is to use multiple well-documented analysis pipelines for each step in the pipeline (Misra et al., 2019). The detailed discussion on various omics and multi-omics pipelines is beyond the manuscript’s scope. There are excellent reviews available on transcriptomic (Wadapurkar et al., 2021), proteomic (Halder et al., 2021), metagenomic (Yang et al., 2021), and metabolomic (Du et al., 2022) pipelines and their integration for multi-omics (Subramanian et al., 2020; Reel et al., 2021), which can be referred. GitHub (https://github.com/danielecook/Awesome-Bioinformatics) and Biostars (https://www.biostars.org/) are also good sources of various updates on omics-related softwares and data analysis, respectively.
Applications of omics technology to explore the full potential of livestock face many practical challenges. Some of those challenges are as follows:
Data recording and handling of raw data is a big challenge for breeders. To avoid errors and bias in data processing and analysis, suitable cutoffs (e.g., microbial relative abundance, gene expression threshold, metabolite similarity, differential expression cutoff, enriched function cutoff, significant impact value of pathways), data preprocessing options (e.g., data baseline filtering and calibration, peak alignment, deconvolution analysis, peak identification), data normalization, data transformation, and data scaling methods should be carefully considered and addressed within each study. Database construction is an important way for data storage and data maintenance. One such database is ASlive, which has been designed for livestock to capture alternative splicing events in heterogeneous samples from a wide range of tissues, cell types, and biological conditions (Liu et al., 2020). More such databases will accelerate the study and applications of omics technology for animal improvement.
Most organized animal farms maintain the performance records of various economic traits, including production traits, reproduction traits, and growth traits. However, organism-wide phenotypic data of animals during different growth phases, various physiological or production stages, in response to dietary changes or upon their selective breeding (i.e., phenome-level data) are challenging to maintain and are generally missing (Pérez-Enciso and Steibel 2021). Accurate phenomics data on adaptability, fitness, body conformation, disease resistance/susceptibility, production performance, reproduction, and growth characteristics will help better estimate accurate BV and selection of genetically superior animals (Juárez et al., 2021; Pérez-Enciso and Steibel 2021). Particular emphasis should be given for multi-omics with other “big data”; for example, those detected by advanced management technologies (e.g., using remote sensors communicating with the Internet of Things to measure physiological and behavioral data, which can be applied to monitor estrus, lameness, or rumination) to have complete data set (Sun et al., 2019). The systematic collection of large data sets from different biological layers will help generate a more holistic understanding of the biological factors affecting the performances of the animals.
Omics technology generates enormous amounts of data at the genome, transcriptome, proteome, or metabolome levels. Proper handling of omics data and advanced knowledge of statistics and bioinformatics are prerequisites for the adequate utilization of omics technology. One should have good knowledge in the above-mentioned fields and computer knowledge to interpret the data generated through omics technology. Lack of good expertise may mislead for selection of suitable animals. There are many challenges associated with proper data recording, processing, quality control, normalization, and genetic prediction (Yamada et al., 2021). Breeders, biological scientists, veterinarians, statisticians, and computer scientists should be trained to overcome these problems. All should collaborate to interpret and adequately utilize omics data, including phenomics data for animal improvement.
The goal of animal production is to achieve increased productivity to fulfill human demand while enhancing the health and wellbeing of animals. Population growth, climate change, resource depletion, human health and nutrition, and sustainability are all issues with which the world is grappling. Different new breeding technologies and molecular technologies such as genomic selection, WGS, and gene editing contribute tremendously to the selection and breeding of livestock species for sustainable improvement in productivity and profitability. Omics technologies such as genomics, proteomics, transcriptomics, metagenomics, and metabolomics offer powerful analytical tools that can be combined with molecular breeding for the accurate selection of animals for improved productivity. Genomics, in particular, can make conventional breeding and advanced breeding techniques more efficient and precisely targeted by increasing consistency and predictability. Integration of multi-layers of omics technology, including phenomics, into the breeding models, will be helpful for proper selection and breeding for animal improvement in the near future.
DC, NS, SK, and MG conceptualized the idea, collected literature, and drafted the manuscript; SS, SL, NS, and MG reviewed, edited, and finalized the manuscript.
This study was supported by the Department of Biotechnology, Government of India (No. BT/PR26321/SPD/9/1307/2017), the National Research Foundation of Korea grant funded by Korean Government (MSIT) (No. 2020R1A2C2004128) and DBT, Government of India (No. BT/PR40124/BTIS/137/14/2021).
The authors declare that the research was conducted in the absence of any commercial or financial relationships that could be construed as a potential conflict of interest.
All claims expressed in this article are solely those of the authors and do not necessarily represent those of their affiliated organizations, or those of the publisher, the editors and the reviewers. Any product that may be evaluated in this article, or claim that may be made by its manufacturer, is not guaranteed or endorsed by the publisher.
Aardema, M. J., and MacGregor, J. T. (2003). Toxicology and Genetic Toxicology in the New Era of "Toxicogenomics": Impact of "-omics" Technologies. Mutat. Res. 499, 171–193. doi:10.1007/978-4-431-66999-9_22
Aboshady, H. M., Mandonnet, N., Johansson, A. M., Jonas, E., and Bambou, J. C. (2021). Genomic Variants from RNA-Seq for Goats Resistant or Susceptible to Gastrointestinal Nematode Infection. PloS One 16 (3), e0248405. doi:10.1371/journal.pone.0248405
Al-Sharif, M., Radwan, H., Hendam, B., and Ateya, A. (2022). DNA Polymorphisms of FGFBP1, Leptin, κ-casein, and αs1-casein Genes and Their Association with Reproductive Performance in Dromedary She-Camels. Theriogenology 178, 18–29. doi:10.1016/j.theriogenology.2021.11.001
Alemu, S. W., Kadri, N. K., Harland, C., Faux, P., Charlier, C., Caballero, A., et al. (2021). An Evaluation of Inbreeding Measures Using a Whole-Genome Sequenced Cattle Pedigree. Heredity 126 (3), 410–423. doi:10.1038/s41437-020-00383-9
Alessandrì, L., Arigoni, M., and Calogero, R. (2019). Differential Expression Analysis in Single-Cell Transcriptomics. Methods Mol. Biol. Clift. NJ) 1979, 425–432.
Aliakbari, A., Zemb, O., Billon, Y., Barilly, C., Ahn, I., Riquet, J., et al. (2021). Genetic Relationships between Feed Efficiency and Gut Microbiome in Pig Lines Selected for Residual Feed Intake. J. animal Breed. Genet. 138 (4), 491–507. doi:10.1111/jbg.12539
Almeida, A. M., Bassols, A., Bendixen, E., Bhide, M., Ceciliani, F., Cristobal, S., et al. (2015). Animal Board Invited Review: Advances in Proteomics for Animal and Food Sciences. Animal 9 (1), 1–17. doi:10.1017/s1751731114002602
Andrews, T., Neher, D. A., Weicht, T. R., and Barlow, J. W. (2019). Mammary Microbiome of Lactating Organic Dairy Cows Varies by Time, Tissue Site, and Infection Status. PloS one 14 (11), e0225001. doi:10.1371/journal.pone.0225001
Angerer, P., Simon, L., Tritschler, S., Wolf, F. A., Fischer, D., and Theis, F. J. (2017). Single Cells Make Big Data: New Challenges and Opportunities in Transcriptomics. Curr. Opin. Syst. Biol. 4, 85–91. doi:10.1016/j.coisb.2017.07.004
Argelaguet, R., Velten, B., Arnol, D., Dietrich, S., Zenz, T., Marioni, J. C., et al. (2018). Multi-Omics Factor Analysis—A Framework for Unsupervised Integration of Multi‐omics Data Sets. Mol. Syst. Biol. 14 (6), 8124. doi:10.15252/msb.20178124
Artegoitia, V. M., Newman, J. W., Foote, A. P., Shackelford, S. D., King, D. A., Wheeler, T. L., et al. (2022). Non-invasive Metabolomics Biomarkers of Production Efficiency and Beef Carcass Quality Traits. Sci. Rep. 12 (1), 231. doi:10.1038/s41598-021-04049-2
Aten, J. E., Fuller, T. F., Lusis, A. J., and Horvath, S. (2008). Using Genetic Markers to Orient the Edges in Quantitative Trait Networks: the NEO Software. BMC Syst. Biol. 2 (1), 1–21. doi:10.1186/1752-0509-2-34
Athanasopoulou, K., Boti, M. A., Adamopoulos, P. G., Skourou, P. C., and Scorilas, A. (2021). Third-Generation Sequencing: The Spearhead towards the Radical Transformation of Modern Genomics. Life (Basel, Switz. 12 (1). doi:10.3390/life12010030
Bach, A., Joulie, I., Chevaux, E., Elcoso, G., and Ragués, J. (2021). Milk Performance and Rumen Microbiome of Dairy Cows as Affected by the Inclusion of Corn Silage or Corn Shredlage in a Total Mixed Ration. Animal Int. J. animal Biosci. 15 (1), 100014. doi:10.1016/j.animal.2020.100014
Banerjee, P., Carmelo, V. A. O., and Kadarmideen, H. N. (2020). Genome-Wide Epistatic Interaction Networks Affecting Feed Efficiency in Duroc and Landrace Pigs. Front. Genet. 11, 121. doi:10.3389/fgene.2020.00121
Baykalir, Y., Baykalir, B. G., and Simsek, U. G. (2018). “Application of Some Proteome Analysis Techniques in Animal Reproduction.” in New Insights into Theriogenology. Editor Payan-Carreira, R. (London, UK: IntechOpen), 63–76. doi:10.5772/intechopen.80521
Bendixen, E. (2005). The Use of Proteomics in Meat Science. Meat Sci. 71, 138–149. doi:10.1016/j.meatsci.2005.03.013
Bhattarai, D., Chen, X., Ur Rehman, Z., Hao, X., Ullah, F., Dad, R., et al. (2017). Association of MAP4K4 Gene Single Nucleotide Polymorphism with Mastitis and Milk Traits in Chinese Holstein Cattle. J. dairy Res. 84 (1), 76–79. doi:10.1017/s0022029916000832
Bovo, S., Ribani, A., Muñoz, M., Alves, E., Araujo, J. P., Bozzi, R., et al. (2020). Whole-genome Sequencing of European Autochthonous and Commercial Pig Breeds Allows the Detection of Signatures of Selection for Adaptation of Genetic Resources to Different Breeding and Production Systems. Genet. Sel. Evol. GSE 52 (1), 33. doi:10.1186/s12711-020-00553-7
Brito, L. F., Bedere, N., Douhard, F., Oliveira, H. R., Arnal, M., Peñagaricano, F., et al. (2021). Review: Genetic Selection of High-Yielding Dairy Cattle toward Sustainable Farming Systems in a Rapidly Changing World. Animal Int. J. animal Biosci. 15 (Suppl. 1), 100292. doi:10.1016/j.animal.2021.100292
Brito, L. F., Oliveira, H. R., McConn, B. R., Schinckel, A. P., Arrazola, A., Marchant-Forde, J. N., et al. (2020). Large-Scale Phenotyping of Livestock Welfare in Commercial Production Systems: A New Frontier in Animal Breeding. Front. Genet. 11, 793. doi:10.3389/fgene.2020.00793
Bu, D., Wang, X., and Tang, H. (2021). Haplotype-based Membership Inference from Summary Genomic Data. Bioinforma. Oxf. Engl. 37 (Suppl. l_1), i161–i168. doi:10.1093/bioinformatics/btab305
Calus, M. P. L., De Haas, Y., Pszczola, M., and Veerkamp, R. F. (2013). Predicted Accuracy of and Response to Genomic Selection for New Traits in Dairy Cattle. Animal 7 (2), 183–191. doi:10.1017/s1751731112001450
Cánovas, A. (2016). Looking Ahead: Applying New Genomic Technologies to Accelerate Genetic Improvement in Beef Cattle. Ceiba 54 (1), 41–49.
Cánovas, A., Reverter, A., DeAtley, K. L., Ashley, R. L., Colgrave, M. L., Fortes, M. R., et al. (2014). Multi-tissue Omics Analyses Reveal Molecular Regulatory Networks for Puberty in Composite Beef Cattle. PloS One 9 (7), 102551. doi:10.1371/journal.pone.0102551
Cansolo, N. R. B., Silva, J. D., Buarque, V. L. M., Higuera-Padilla, A., Barbosa, L., Zawadzki, A., et al. (2020). Selection for Growth and Precocity Alters Muscle Metabolism in Nellore Cattle. Metabolites 10 (2). doi:10.3390/metabo10020058
Carrillo, J. A., He, Y., Li, Y., Liu, J., Erdman, R. A., Sonstegard, T. S., et al. (2016). Integrated Metabolomic and Transcriptome Analyses Reveal Finishing Forage Affects Metabolic Pathways Related to Beef Quality and Animal Welfare. Sci. Rep. 6, 25948. doi:10.1038/srep25948
Chang, L. Y., Toghiani, S., Aggrey, S. E., and Rekaya, R. (2019). Increasing Accuracy of Genomic Selection in Presence of High Density Marker Panels through the Prioritization of Relevant Polymorphisms. BMC Genet. 20 (1), 21. doi:10.1186/s12863-019-0720-5
Chen, C., Zhou, Y., Fu, H., Xiong, X., Fang, S., Jiang, H., et al. (2021a). Expanded Catalog of Microbial Genes and Metagenome-Assembled Genomes from the Pig Gut Microbiome. Nat. Commun. 12 (1), 1106. doi:10.1038/s41467-021-21295-0
Chen, S., Liu, S., Mi, S., Li, W., Zhang, S., Ding, X., et al. (2021b). Comparative Analyses of Sperm DNA Methylomes Among Three Commercial Pig Breeds Reveal Vital Hypomethylated Regions Associated with Spermatogenesis and Embryonic Development. Front. Genet. 12, 740036. doi:10.3389/fgene.2021.740036
Cohen, D., Hondelink, L. M., Solleveld-Westerink, N., Uljee, S. M., Ruano, D., Cleton-Jansen, A. M., et al. (2020). Optimizing Mutation and Fusion Detection in NSCLC by Sequential DNA and RNA Sequencing. J. Thorac. Oncol. official Publ. Int. Assoc. Study Lung Cancer 15 (6), 1000–1014. doi:10.1016/j.jtho.2020.01.019
Cole, J. B., Dürr, J. W., and Nicolazzi, E. L. (2021). Invited Review: The Future of Selection Decisions and Breeding Programs: What Are We Breeding for, and Who Decides? J. dairy Sci. 104 (5), 5111–5124. doi:10.3168/jds.2020-19777
Dekkers, J. C., and Hospital, F. (2002). The Use of Molecular Genetics in the Improvement of Agricultural Populations. Nat. Rev. Genet. 3 (1), 22–32. doi:10.1038/nrg701
Dekkers, J. C. M., Zhao, H. H., Habier, D., and Fernando, R. L. (2009). Opportunities for Genomic Selection with Redesign of Breeding Programs. J. Animal Sci. 87 (Suppl. E), 275.
Dervishi, E., Reimert, I., van der Zande, L. E., Mathur, P., Knol, E. F., and Plastow, G. S. (2021). Relationship between Indirect Genetic Effects for Growth, Environmental Enrichment, Coping Style and Sex with the Serum Metabolome Profile of Pigs. Sci. Rep. 11 (1), 23377. doi:10.1038/s41598-021-02814-x
Do, D. N., Strathe, A. B., Ostersen, T., Pant, S. D., and Kadarmideen, H. N. (2014). Genome-wide Association and Pathway Analysis of Feed Efficiency in Pigs Reveal Candidategenes and Pathways for Residual Feed Intake. Front. Genet. 5, 307. doi:10.3389/fgene.2014.00307
Do, D. N., Strathe, A. B., Ostersen, T., Jensen, J., Mark, T., and Kadarmideen, H. N. (2013). Genome-wide Association Study Reveals Genetic Architecture of Eating Behavior in Pigs and its Implications for Humans Obesity by Comparative Mapping. PloSOne 8 (8), 71509. doi:10.1371/journal.pone.0071509
Doublet, A. C., Croiseau, P., Fritz, S., Michenet, A., Hozé, C., Danchin-Burge, C., et al. (2019). The Impact of Genomic Selection on Genetic Diversity and Genetic Gain in Three French Dairy Cattle Breeds. Genet. Sel. Evol. 51 (1), 1–13. doi:10.1186/s12711-019-0495-1
Druet, T., Macleod, I. M., and Hayes, B. J. (2014). Toward Genomic Prediction from Whole-Genome Sequence Data: Impact of Sequencing Design on Genotype Imputation and Accuracy of Predictions. Heredity 112 (1), 39–47. doi:10.1038/hdy.2013.13
Du, X., Aristizabal-Henao, J. J., Garrett, T. J., Brochhausen, M., Hogan, W. R., and Lemas, D. J. (2022). A Checklist for Reproducible Computational Analysis in Clinical Metabolomics Research. Metabolites 12 (1). doi:10.3390/metabo12010087
Ehret, A., Hochstuhl, D., Krattenmacher, N., Tetens, J., Klein, M. S., Gronwald, W., et al. (2015). Use of Genomic and Metabolic Information as Well as Milk Performance Records for Prediction of Subclinical Ketosis Risk via Artificial Neural Networks. J. Dairy Sci. 98 (1), 322–329. doi:10.3168/jds.2014-8602
Elsik, C. G., Tellam, R. L., Worley, K. C., Gibbs, R. A., Muzny, D. M., Weinstock, G. M., et al. (2009). Bovine Genome Sequencing and Analysis Consortium. The Genome Sequence of Taurine Cattle: a Window to Ruminant Biology and Evolution. Science 324, 522–528. doi:10.1126/science.1169588
Erasmus, L. M., and van Marle-Köster, E. (2021). Moving towards Sustainable Breeding Objectives and Cow Welfare in Dairy Production: a South African Perspective. Trop. animal health Prod. 53 (5), 470. doi:10.1007/s11250-021-02914-w
Evans, A. M., O'Donovan, C., Playdon, M., Beecher, C., Beger, R. D., Bowden, J. A., et al. (2020). Dissemination and Analysis of the Quality Assurance (QA) and Quality Control (QC) Practices of LC-MS Based Untargeted Metabolomics Practitioners. Metabolomics 16 (10), 113. doi:10.1007/s11306-020-01728-5
Florian, P. B., Lu, J., and Salzberg, S. L. (2019). A Review of Methods and Databases for Metagenomic Classification and Assembly. Briefings Bioinforma. 20 (4), 1125–1136.
Fontanesi, L. (2016). Metabolomics and Livestock Genomics: Insights into a Phenotyping Frontier and its Applications in Animal Breeding. Anim. Front. 6, 73–79. doi:10.2527/af.2016-0011
Fukunaga, T., and Iwasaki, W. (2020). Logicome Profiler: Exhaustive Detection of Statistically Significant Logic Relationships from Comparative Omics Data. PloS One 15 (5), 0232106. doi:10.1371/journal.pone.0232106
Ghaffari, N., Yousefi, M. R., Johnson, C. D., Ivanov, I., and Dougherty, E. R. (2013). Modeling the Next Generation Sequencing Sample Processing Pipeline for the Purposes of Classification. BMC Bioinforma. 14 (1), 1–14. doi:10.1186/1471-2105-14-307
Ghoreishifar, S. M., Moradi-Shahrbabak, H., Fallahi, M. H., Jalil Sarghale, A., Moradi-Shahrbabak, M., Abdollahi-Arpanahi, R., et al. (2020). Genomic Measures of Inbreeding Coefficients and Genome-wide Scan for Runs of Homozygosity Islands in Iranian River Buffalo, Bubalus Bubalis. BMC Genet. 21 (1), 16. doi:10.1186/s12863-020-0824-y
Goldansaz, S. A., Guo, A. C., Sajed, T., Steele, M. A., Plastow, G. S., and Wishart, D. S. (2017). Livestock Metabolomics and the Livestock Metabolome: A Systematic Review. PLoS One 12 (5), e0177675. doi:10.1371/journal.pone.0177675
Gomez, A., Sharma, A. K., Grev, A., Sheaffer, C., and Martinson, K. (2021). The Horse Gut Microbiome Responds in a Highly Individualized Manner to Forage Lignification. J. equine veterinary Sci. 96, 103306. doi:10.1016/j.jevs.2020.103306
Gupta, M. K., Jang, J. M., Jung, J. W., Uhm, S. J., Kim, K. P., and Lee, H. T. (2009a). Proteomic Analysis of Parthenogenetic and In Vitro Fertilized Porcine Embryos. Proteomics 9 (10), 2846–2860. doi:10.1002/pmic.200800700
Gupta, M. K., Jung, J. W., Uhm, S. J., Lee, H., Lee, H. T., and Kim, K. P. (2009b). Combining Selected Reaction Monitoring with Discovery Proteomics in Limited Biological Samples. Proteomics 9 (21), 4834–4836. doi:10.1002/pmic.200900310
Guttula, P. K., Monteiro, P. T., and Gupta, M. K. (2020). A Boolean Logical Model for Reprogramming of Testes-Derived Male Germline Stem Cells into Germline Pluripotent Stem Cells. Comput. methods programs Biomed. 192, 105473. doi:10.1016/j.cmpb.2020.105473
Guttula, P. K., Monteiro, P. T., and Gupta, M. K. (2021). Prediction and Boolean Logical Modelling of Synergistic microRNA Regulatory Networks during Reprogramming of Male Germline Pluripotent Stem Cells. Bio Syst. 207, 104453. doi:10.1016/j.biosystems.2021.104453
Halder, A., Verma, A., Biswas, D., and Srivastava, S. (2021). Recent Advances in Mass-Spectrometry Based Proteomics Software, Tools and Databases. Drug Discov. today Technol. 39, 69–79. doi:10.1016/j.ddtec.2021.06.007
Hawken, R. J., Zhang, Y. D., Fortes, M. R. S., Collis, E., Barris, W. C., Corbet, N. J., et al. (2012). Genome-wide Association Studies of Female Reproduction in Tropically Adapted Beef Cattle. J. Animal Sci. 90 (5), 1398–1410. doi:10.2527/jas.2011-4410
Hernandez-Ferrer, C., Ruiz-Arenas, C., Beltran-Gomila, A., and González, J. R. (2017). MultiDataSet: an R Package for Encapsulating Multiple Data Sets with Application to Omic Data Integration. BMC Bioinforma. 18 (1), 1–7. doi:10.1186/s12859-016-1455-1
Ibtisham, F., Zhang, L., Xiao, M., An, L., Ramzan, M. B., Nawab, A., et al. (2017). Genomic Selection and its Application in Animal Breeding. Thai J. Veterinary Med. 47 (3), 301.
Jaiswal, S., Jagannadham, J., Kumari, J., Iquebal, M. A., Gurjar, A. K. S., Nayan, V., et al. (2021). Genome Wide Prediction, Mapping and Development of Genomic Resources of Mastitis Associated Genes in Water Buffalo. Front. veterinary Sci. 8, 593871. doi:10.3389/fvets.2021.593871
Jiang, H., Fang, S., Yang, H., and Chen, C. (2021). Identification of the Relationship between the Gut Microbiome and Feed Efficiency in a Commercial Pig Cohort. J. Anim. Sci. 99 (3). doi:10.1093/jas/skab045
Jorge-Smeding, E., Bonnet, M., Renand, G., Taussat, S., Graulet, B., Ortigues-Marty, I., et al. (2021). Common and Diet-specific Metabolic Pathways Underlying Residual Feed Intake in Fattening Charolais Yearling Bulls. Sci. Rep. 11 (1), 24346. doi:10.1038/s41598-021-03678-x
Juárez, M., Lam, S., Bohrer, B. M., Dugan, M. E. R., Vahmani, P., Aalhus, J., et al. (2021). Enhancing the Nutritional Value of Red Meat through Genetic and Feeding Strategies. Foods (Basel, Switz. 10 (4).
Kadarmideen, H. N. (2014). Genomics to Systems Biology in Animal and Veterinary Sciences: Progress, Lessons and Opportunities. Livest. Sci. 166, 232–248. doi:10.1016/j.livsci.2014.04.028
Kalaiselvi, G., Tirumurugaan, K. G., Vijayarani, K., Dhinakar Raj, G., Baranidharan, G. R., and Bobade, S. (2019). Livestock Metabolomics-An Overview. Int. J. Curr. Res. 11 (3), 1972–1980.
Kasper, C., Ribeiro, D., Almeida, A. M. D., Larzul, C., Liaubet, L., and Murani, E. (2020). Omics Application in Animal Science—A Special Emphasis on Stress Response and Damaging Behaviour in Pigs. Genes. 11 (8), 920. doi:10.3390/genes11080920
Kaya, A., Dogan, S., Vargovic, P., Kutchy, N. A., Ross, P., Topper, E., et al. (2022). Sperm Proteins ODF2 and PAWP as Markers of Fertility in Breeding Bulls. Cell. tissue Res. 387 (1), 159–171. doi:10.1007/s00441-021-03529-1
Kogelman, L. J., Pant, S. D., Fredholm, M., and Kadarmideen, H. N. (2014). Systems Genetics of Obesity in an F2 Pig Model by Genome-wide Association, Genetic Network, and Pathway Analyses. Front. Genet. 5, 214. doi:10.3389/fgene.2014.00214
Krassowski, M., Das, V., Sahu, S. K., and Misra, B. B. (2020). State of the Field in Multi-Omics Research: From Computational Needs to Data Mining and Sharing. Front. Genet. 11, 610798. doi:10.3389/fgene.2020.610798
Kuska, B. (1998). Beer, Bethesda, and Biology: How “Genomics” Came into Being. J. Natl. Cancer Inst. 90, 93. doi:10.1093/jnci/90.2.93
Langfelder, P., and Horvath, S. (2008). WGCNA: an R Package for Weighted Correlation Network Analysis. BMC Bioinforma. 9 (1), 1–13. doi:10.1186/1471-2105-9-559
Li, C., Gao, Z., Su, B., Xu, G., and Lin, X. (2022). Data Analysis Methods for Defining Biomarkers from Omics Data. Anal. Bioanal. Chem. 414 (1), 235–250. doi:10.1007/s00216-021-03813-7
Li, J., Witten, D. M., Johnstone, I. M., and Tibshirani, R. (2012). Normalization, Testing, and False Discovery Rate Estimation for RNA-Sequencing Data. Biostatistics 13 (3), 523–538. doi:10.1093/biostatistics/kxr031
Li, X., and Wang, C. Y. (2021). From Bulk, Single-Cell to Spatial RNA Sequencing. Int. J. oral Sci. 13 (1), 36. doi:10.1038/s41368-021-00146-0
Lillehammer, M., Meuwissen, T. H. E., and Sonesson, A. K. (2011). Genomic Selection for Maternal Traits in Pigs. J. animal Sci. 89 (12), 3908–3916. doi:10.2527/jas.2011-4044
Lippa, K. A., Aristizabal-Henao, J. J., Beger, R. D., Bowden, J. A., Broeckling, C., Beecher, C., et al. (2022). Reference Materials for MS-based Untargeted Metabolomics and Lipidomics: a Review by the Metabolomics Quality Assurance and Quality Control Consortium (mQACC). Metabolomics 18 (4), 24. doi:10.1007/s11306-021-01848-6
Lippert, C., Sabatini, R., Maher, M. C., Kang, E. Y., Lee, S., Arikan, O., et al. (2017). Identification of Individuals by Trait Prediction Using Whole-Genome Sequencing Data. Proc. Natl. Acad. Sci. U. S. A. 114 (38), 10166–10171. doi:10.1073/pnas.1711125114
Liu, J., Tan, S., Huang, S., and Huang, W. (2020). ASlive: a Database for Alternative Splicing Atlas in Livestock Animals. BMC Genomics 21, 97. doi:10.1186/s12864-020-6472-9
Ma, B., Khan, R., Raza, S. H. A., Gao, Z., Hou, S., Ullah, F., et al. (2021). Determination of the Relationship between Class IV Sirtuin Genes and Growth Traits in Chinese Black Tibetan Sheep. Anim. Biotechnol. 2021, 1–7. doi:10.1080/10495398.2021.2016434
Malheiros, J. M., Correia, B. S. B., Ceribeli, C., Cardoso, D. R., Colnago, L. A., Junior, S. B., et al. (2021). Comparative Untargeted Metabolome Analysis of Ruminal Fluid and Feces of Nelore Steers (Bos indicus). Sci. Rep. 11 (1), 12752. doi:10.1038/s41598-021-92179-y
Martin, M. J., Pralle, R. S., Bernstein, I. R., VandeHaar, M. J., Weigel, K. A., Zhou, Z., et al. (2021). Circulating Metabolites Indicate Differences in High and Low Residual Feed Intake Holstein Dairy Cows. Metabolites 11 (12). doi:10.3390/metabo11120868
Matukumalli, L. K., Lawley, C. T., Schnabel, R. D., Taylor, J. F., Allan, M. F., Heaton, M. P., et al. (2009). Development and Characterization of a High Density SNP Genotyping Assay for Cattle. PloS One 4 (4), 5350. doi:10.1371/journal.pone.0005350
Misra, B. B., Langefeld, C., Olivier, M., and Cox, L. A. (2019). Integrated Omics: Tools, Advances and Future Approaches. J. Mol. Endocrinol. 62, R21–R45. doi:10.1530/jme-18-0055
Morgan, J. L., Ritchie, L. E., Crucian, B. E., Theriot, C., Wu, H., Sams, C., et al. (2014). Increased Dietary Iron and Radiation in Rats Promote Oxidative Stress, Induce Localized and Systemic Immune System Responses, and Alter Colon Mucosal Environment. FASEB J. 28 (3), 1486–1498. doi:10.1096/fj.13-239418
Moridi, M., Moghaddam, S. H. H., Mirhoseini, S. Z., and Bionaz, M. (2019). Transcriptome Analysis Showed Differences of Two Purebred Cattle and Their Crossbreds. Italian J. Animal Sci. 18 (1), 70–79. doi:10.1080/1828051x.2018.1482800
Mortazavi, A., Williams, B. A., McCue, K., Schaeffer, L., and Wold, B. (2008). Mapping and Quantifying Mammalian Transcriptomes by RNA-Seq. Nat. methods 5 (7), 621–628. doi:10.1038/nmeth.1226
Mu, Y., Qi, W., Zhang, T., Zhang, J., and Mao, S. (2022). Multi-omics Analysis Revealed Coordinated Responses of Rumen Microbiome and Epithelium to High-Grain-Induced Subacute Rumen Acidosis in Lactating Dairy Cows. mSystems 7 (1), e0149021. doi:10.1128/msystems.01490-21
Muhanguzi, D., Ndekezi, C., Nkamwesiga, J., Kalayou, S., Ochwo, S., Vuyani, M., et al. (2022). Anti-Tick Vaccines: Current Advances and Future Prospects. Methods Mol. Biol. Clift. NJ) 2411, 253–267. doi:10.1007/978-1-0716-1888-2_15
Mullins, Y., Keogh, K., Blackshields, G., Kenny, D. A., Kelly, A. K., and Waters, S. M. (2021). Transcriptome Assisted Label Free Proteomics of Hepatic Tissue in Response to Both Dietary Restriction and Compensatory Growth in Cattle. J. proteomics 232, 104048. doi:10.1016/j.jprot.2020.104048
Muñoz, M., Bozzi, R., García-Casco, J., Núñez, Y., Ribani, A., Franci, O., et al. (2019). Genomic Diversity, Linkage Disequilibrium and Selection Signatures in European Local Pig Breeds Assessed with a High Density SNP Chip. Sci. Rep. 9 (1), 13546.
Ng, B., Casazza, W., Kim, N. H., Wang, C., Farhadi, F., Tasaki, S., et al. (2021). Cascading Epigenomic Analysis for Identifying Disease Genes from the Regulatory Landscape of GWAS Variants. PLoS Genet. 17 (11), e1009918. doi:10.1371/journal.pgen.1009918
Odom, G. J., Colaprico, A., Silva, T. C., Chen, X. S., and Wang, L. (2021). PathwayMultiomics: An R Package for Efficient Integrative Analysis of Multi-Omics Datasets with Matched or Un-matched Samples. Front. Genet. 12, 783713. doi:10.3389/fgene.2021.783713
Oskoueian, E., Eckersall, P. D., Bencurova, E., and Dandekar, T. (2016). “Application of Proteomic Biomarkers in Livestock Disease Management,” in Agricultural Proteomics- Volume 2. Editor G. H. Salekdeh (Phillippines: Springer), 299–310. doi:10.1007/978-3-319-43278-6_14
Peddinti, D., Memili, E., and Burgess, S. C. (2011). 13 Proteomics in Animal Reproduction and Breeding. Methods Animal Proteomics 369.
Pedrosa, V. B., Schenkel, F. S., Chen, S. Y., Oliveira, H. R., Casey, T. M., Melka, M. G., et al. (2021). Genomewide Association Analyses of Lactation Persistency and Milk Production Traits in Holstein Cattle Based on Imputed Whole-Genome Sequence Data. Genes. 12 (11). doi:10.3390/genes12111830
Peng, C., Wang, J., Asante, I., Louie, S., Jin, R., Chatzi, L., et al. (2020). A Latent Unknown Clustering Integrating Multi-Omics Data (LUCID) with Phenotypic Traits. Bioinformatics 36 (3), 842–850. doi:10.1093/bioinformatics/btz667
Pérez-Enciso, M., and Steibel, J. P. (2021). Phenomes: the Current Frontier in Animal Breeding. Genet. Sel. Evol. GSE 53 (1), 22.
Perez-Riverol, Y., Bai, M., da Veiga Leprevost, F., Squizzato, S., Park, Y. M., Haug, K., et al. (2017). Discovering and Linking Public Omics Data Sets Using the Omics Discovery Index. Nat. Biotechnol. 35 (5), 406–409. doi:10.1038/nbt.3790
Pimentel, E. C. G., and König, S. (2012). Genomic Selection for the Improvement of Meat Quality in Beef. J. Animal Sci. 90 (10), 3418–3426. doi:10.2527/jas.2011-5005
Plieschke, L., Edel, C., Pimentel, E. C., Emmerling, R., Bennewitz, J., and Götz, K. U. (2016). Systematic Genotyping of Groups of Cows to Improve Genomic Estimated Breeding Values of Selection Candidates. Genet. Sel. Evol. 48 (1), 1–11. doi:10.1186/s12711-016-0250-9
Pryce, J. E., and Daetwyler, H. D. (2011). Designing Dairy Cattle Breeding Schemes under Genomic Selection: a Review of International Research. Animal Prod. Sci. 52 (3), 107–114.
Raza, S. H. A., Khan, R., Pant, S. D., Shah, M. A., Quan, G., Feng, L., et al. (2021). Genetic Variation in the OPN Gene Affects Milk Composition in Chinese Holstein Cows. Anim. Biotechnol. 2021, 1–7. doi:10.1080/10495398.2021.2001343
Reel, P. S., Reel, S., Pearson, E., Trucco, E., and Jefferson, E. (2021). Using Machine Learning Approaches for Multi-Omics Data Analysis: A Review. Biotechnol. Adv. 49, 107739. doi:10.1016/j.biotechadv.2021.107739
Reverter, A., and Fortes, M. R. S. (2013). Breeding and Genetics Symposium: Building Single Nucleotide Polymorphism-Derived Gene Regulatory Networks: Towards Functional Genome-wide Association Studies. J. Animal Sci. 91 (2), 530–536. doi:10.2527/jas.2012-5780
Rexroad, C., Vallet, J., Matukumalli, L. K., Reecy, J., Bickhart, D., Blackburn, H., et al. (2019). Genome to Phenome: Improving Animal Health, Production, and Well-Being - A New USDA Blueprint for Animal Genome Research 2018-2027. Front. Genet. 10, 327. doi:10.3389/fgene.2019.00327
Riggs, P. K., and Gill, C. A. (2009). “Molecular Mapping and Marker Assisted Breeding for Meat Quality,” in Applied Muscle Biology and Meat Science. Editor M. Du (Boca Raton: CRC Press), 288–310.
Riggs, P. K., Tedeschi, L. O., Turner, N. D., Braga-Neto, U., and Jayaraman, A. (2017). The Role of “Omics” Technologies for Livestock Sustainability. Arch. Prod. Anim. 25 (3-4).
Ritchie, L. E., Sturino, J. M., Carroll, R. J., Rooney, L. W., Azcarate-Peril, M. A., and Turner, N. D. (2015). Polyphenol-rich Sorghum Brans Alter Colon Microbiota and Impact Species Diversity and Species Richness after Multiple Bouts of Dextran Sodium Sulfate-Induced Colitis. FEMS Microbiol. Ecol. 91 (3). doi:10.1093/femsec/fiv008
Rohart, F., Gautier, B., Singh, A., and Lê Cao, K. A. (2017). mixOmics: An R Package for ‘omics Feature Selection and Multiple Data Integration. PLoS Comput. Biol. 13 (11), 1005752. doi:10.1371/journal.pcbi.1005752
Romero, R., Espinoza, J., Gotsch, F., Kusanovic, J. P., Friel, L. A., Erez, O., et al. (2006). The Use of High-Dimensional Biology (Genomics, Transcriptomics, Proteomics, and Metabolomics) to Understand the Preterm Parturition Syndrome. BJOG Int. J. obstetrics Gynaecol. 113 (Suppl. 3), 118–135. doi:10.1111/j.1471-0528.2006.01150.x
Ruan, D., Zhuang, Z., Ding, R., Qiu, Y., Zhou, S., Wu, J., et al. (2021). Weighted Single-step GWAS Identified Candidate Genes Associated with Growth Traits in a Duroc Pig Population. Genes. 12 (1). doi:10.3390/genes12010117
Russell, C. D., Widdison, S., Leigh, J. A., and Coffey, T. J. (2012). Identification of Single Nucleotide Polymorphisms in the Bovine Toll-like Receptor 1 Gene and Association with Health Traits in Cattle. Veterinary Res. 43 (1), 17. doi:10.1186/1297-9716-43-17
Sahoo, B., Choudhary, R. K., Sharma, P., Choudhary, S., and Gupta, M. K. (2021a). Significance and Relevance of Spermatozoal RNAs to Male Fertility in Livestock. Front. Genet. 12, 768196. doi:10.3389/fgene.2021.768196
Sahoo, B., Guttula, P. K., and Gupta, M. K. (2021b). Comparison of Spermatozoal RNA Extraction Methods in Goats. Anal. Biochem. 614, 114059. doi:10.1016/j.ab.2020.114059
Sahu, A., Nayak, N., Sahu, R., and Kumar, J. (2017). Application of Metagenomics in Livestock Improvement. Int. J. Livest. Res. 7, 30–38. doi:10.5455/ijlr.20170423033727
Sakuma, H., Saito, K., Kohira, K., Ohhashi, F., Shoji, N., and Uemoto, Y. (2016). Estimates of Genetic Parameters for Chemical Traits of Meat Quality in Japanese Black Cattle. Anim. Sci. J. 88 (2), 203–212. doi:10.1111/asj.12622
Seidel, D., Martínez, I., Taddeo, S., Joseph, M., Carroll, R., Haub, M., et al. (2014). A Polyphenol‐rich Sorghum Cereal Alters Colon Microbiota and Plasma Metabolites in Overweight Subjects (270.7). FASEB J. 28, 270–277. doi:10.1096/fasebj.28.1_supplement.270.7
Shang, L., Smith, J. A., and Zhou, X. (2020). Leveraging Gene Co-expression Patterns to Infer Trait-Relevant Tissues in Genome-wide Association Studies. PLoS Genet. 16 (4), 1008734. doi:10.1371/journal.pgen.1008734
Shannon, P., Markiel, A., Ozier, O., Baliga, N. S., Wang, J. T., Ramage, D., et al. (2003). Cytoscape: a Software Environment for Integrated Models of Biomolecular Interaction Networks. Genome Res. 13 (11), 2498–2504. doi:10.1101/gr.1239303
Sharma, A., Lee, J. S., Dang, C. G., Sudrajad, P., Kim, H. C., Yeon, S. H., et al. (2015). Stories and Challenges of Genome Wide Association Studies in Livestock—A Review. Asian-Australasian J. Animal Sci. 28 (10), 1371. doi:10.5713/ajas.14.0715
Shi, T. P., and Zhang, L. (2019). Application of Whole Transcriptomics in Animal Husbandry. Yi Chuan= Hered. 41 (3), 193–205. doi:10.16288/j.yczz.18-218
Shumbusho, F., Raoul, J., Astruc, J. M., Palhière, I., and Elsen, J. M. (2013). Potential Benefits of Genomic Selection on Genetic Gain of Small Ruminant Breeding Programs. J. Animal Sci. 91 (8), 3644–3657. doi:10.2527/jas.2012-6205
Singh, A., Shannon, C. P., Gautier, B., Rohart, F., Vacher, M., Tebbutt, S. J., et al. (2019). DIABLO: Anintegrative Approach for Identifying Key Molecular Drivers from Multi-Omics Assays. Bioinformatics 35, 3055–3062. doi:10.1093/bioinformatics/bty1054
Sitzenstock, F., Ytournel, F., Sharifi, A. R., Cavero Täubert, D. H., Preisinger, R., and Simianer, H. (2013). Efficiency of Genomic Selection in an Established Commercial Layer Breeding Program. Genet. Sel. Evol. 45, 1–11. doi:10.1186/1297-9686-45-29
Snelling, W. M., Cushman, R. A., Keele, J. W., Maltecca, C., Thomas, M. G., Fortes, M. R. S., et al. (2013). Breeding and Genetics Symposium: Networks and Pathways to Guide Genomic Selection. J. Animal Sci. 91 (2), 537–552. doi:10.2527/jas.2012-5784
Speicher, D. W. (2004). “Overview of Proteome Analysis,” in Proteome Analysis (Phillippines: Elsevier), 1–18. doi:10.1016/b978-044451024-2/50018-7
Stock, J., Bennewitz, J., Hinrichs, D., and Wellmann, R. (2020). A Review of Genomic Models for the Analysis of Livestock Crossbred Data. Front. Genet. 11, 568. doi:10.3389/fgene.2020.00568
Subramanian, I., Verma, S., Kumar, S., Jere, A., and Anamika, K. (2020). Multi-omics Data Integration, Interpretation, and its Application. Bioinforma. Biol. insights 14, 1177932219899051. doi:10.1177/1177932219899051
Sumreddee, P., Hay, E. H., Toghiani, S., Roberts, A., Aggrey, S. E., and Rekaya, R. (2021). Grid Search Approach to Discriminate between Old and Recent Inbreeding Using Phenotypic, Pedigree and Genomic Information. BMC genomics 22 (1), 538. doi:10.1186/s12864-021-07872-z
Sun, H. Z., Plastow, G., and Guan, L. L. (2019). Invited Review: Advances and Challenges in Application of Feedomics to Improve Dairy Cow Production and Health. J. Dairy Sci. 102 (7), 5853–5870. doi:10.3168/jds.2018-16126
Sun, H. Z., Wang, D. M., Wang, B., Wang, J. K., Liu, H. Y., Guan, L. L., et al. (2015). Metabolomics of Four Biofluids from Dairy Cows: Potential Biomarkers for Milk Production and Quality. J. Proteome Res. 14 (2), 1287–1298. doi:10.1021/pr501305g
Sun, X., Cui, Y., Su, Y., Gao, Z., Diao, X., Li, J., et al. (2021). Dietary Fiber Ameliorates Lipopolysaccharide-Induced Intestinal Barrier Function Damage in Piglets by Modulation of Intestinal Microbiome. mSystems 6 (2). doi:10.1128/msystems.01374-20
Suravajhala, P., Kogelman, L. J., and Kadarmideen, H. N. (2016). Multi-omic Data Integration and Analysis Using Systems Genomics Approaches: Methods and Applications in Animal Production, Health and Welfare. Genet. Sel. Evol. 48 (1), 1–14. doi:10.1186/s12711-016-0217-x
Tan, C., Bian, C., Yang, D., Li, N., Wu, Z. F., and Hu, X. X. (2017). Application of Genomic Selection in Farm Animal Breeding. Yi Chuan 39 (11), 1033–1045. doi:10.16288/j.yczz.17-286
Tenenhaus, A., Philippe, C., Guillemot, V., Le Cao, K. A., Grill, J., and Frouin, V. (2014). Variable Selection for Generalized Canonical Correlation Analysis. Biostatistics 15 (3), 569–583. doi:10.1093/biostatistics/kxu001
The Bovine HapMap Consortium (2009). Genome-Wide Survey of SNP Variation Uncovers the Genetic Structure of Cattle Breeds. Science 324, 528–532. doi:10.1126/science.1167936
The Bovine Genome Sequencing and Analysis Consortium Elsik, C. G., Tellam, R. L., and Worley, K. C. (2009). The Genome Sequence of Taurine Cattle: A Window to Ruminant Biology and Evolution. Science 324, 522–528. doi:10.1126/science.1169588
Tiezzi, F., Fix, J., Schwab, C., Shull, C., and Maltecca, C. (2021). Gut Microbiome Mediates Host Genomic Effects on Phenotypes: a Case Study with Fat Deposition in Pigs. Comput. Struct. Biotechnol. J. 19, 530–544. doi:10.1016/j.csbj.2020.12.038
Ueda, S., Iwamoto, E., Kato, Y., Shinohara, M., Shirai, Y., and Yamanoue, M. (2018). Comparative Metabolomics of Japanese Black Cattle Beef and Other Meats Using Gas Chromatography-Mass Spectrometry. Biosci. Biotechnol. Biochem. 83 (1), 137–147. doi:10.1080/09168451.2018.1528139
Valerio, A., Casadei, L., Giuliani, A., and Valerio, M. (2020). Fecal Metabolomics as a Novel Noninvasive Method for Short-Term Stress Monitoring in Beef Cattle. J. Proteome Res. 19 (2), 845–853. doi:10.1021/acs.jproteome.9b00655
Velten, B., Braunger, J. M., Argelaguet, R., Arnol, D., Wirbel, J., Bredikhin, D., et al. (2022). Identifying Temporal and Spatial Patterns of Variation from Multimodal Data Using MEFISTO. Nat. methods 19, 179. doi:10.1038/s41592-021-01343-9
Wadapurkar, R. M., Sivaram, A., and Vyas, R. (2021). Computational Studies Reveal Co-occurrence of Two Mutations in IL7R Gene of High-Grade Serous Carcinoma Patients. J. Biomol. Struct. Dyn. 2021, 1–15. doi:10.1080/07391102.2021.1987326
Wang, H., Misztal, I., Aguilar, I., Legarra, A., Fernando, R. L., and Vitezica, Z. (2014). Genome-wide Association Mapping Including Phenotypes from Relativeswithout Genotypes in a Single-step (ssGWAS) for 6-week Bodyweight in Broiler Chickens. Front. Genet. 5, 134. doi:10.3389/fgene.2014.00134
Wang, X., and Kadarmideen, H. N. (2019). An Epigenome-wide DNA Methylation Map of Testis in Pigs for Study of Complex Traits. Front. Genet. 10, 405. doi:10.3389/fgene.2019.00405
Wang, X., and Kadarmideen, H. N. (2020). Metabolite Genome-wide Association Study (mGWAS) and Gene-Metabolite Interaction Network Analysis Reveal Potential Biomarkers for Feed Efficiency in Pigs. Metabolites 10 (5). doi:10.3390/metabo10050201
Wang, Y., Li, J., Lu, D., Meng, Q., Song, N., Zhou, H., et al. (2022). Integrated Proteome and Phosphoproteome Analysis of Interscapular Brown Adipose and Subcutaneous White Adipose Tissues upon High Fat Diet Feeding in Mouse. J. proteomics 255, 104500. doi:10.1016/j.jprot.2022.104500
Wickramasinghe, S., Cánovas, A., Rincon, G., and Medrano, J. F. (2014). Review: RNA-Seq Applications in Livestock. Livest. Rev. 166, 206–216. doi:10.1016/j.livsci.2014.06.015
Willforss, J., Morrell, J. M., Resjö, S., Hallap, T., Padrik, P., Siino, V., et al. (2021). Stable Bull Fertility Protein Markers in Seminal Plasma. J. proteomics 236, 104135. doi:10.1016/j.jprot.2021.104135
Williams, J. L. (2005). The Use of Marker-Assisted Selection in Animal Breeding and Biotechnology. Revue Sci. Tech. Int. Office Epizootics) 24 (1), 379–391. doi:10.20506/rst.24.1.1571
Wu, J., Ye, Y., Quan, J., Ding, R., Wang, X., Zhuang, Z., et al. (2021). Using Nontargeted LC-MS Metabolomics to Identify the Association of Biomarkers in Pig Feces with Feed Efficiency. Porc. health Manag. 7 (1), 39. doi:10.1186/s40813-021-00219-w
Wu, Y., Zeng, J., Zhang, F., Zhu, Z., Qi, T., Zheng, Z., et al. (2018). Integrative Analysis of Omics Summary Data Reveals Putative Mechanisms Underlying Complex Traits. Nat. Commun. 9 (1), 918. doi:10.1038/s41467-018-03371-0
Yadav, S. P. (2007). The Wholeness in Suffix-Omics,-Omes, and the Word Om. J. Biomol. Tech. 18 (5), 277.
Yamada, R., Okada, D., Wang, J., Basak, T., and Koyama, S. (2021). Interpretation of Omics Data Analyses. J. Hum. Genet. 66 (1), 93–102. doi:10.1038/s10038-020-0763-5
Yang, A. Q., Chen, B., Ran, M. L., Yang, G. M., and Zeng, C. (2020). The Application of Genomic Selection in Pig Cross Breeding. Yi chuan = Hered. 42 (2), 145–152. doi:10.16288/j.yczz.19-253
Yang, C., Chowdhury, D., Zhang, Z., Cheung, W. K., Lu, A., Bian, Z., et al. (2021). A Review of Computational Tools for Generating Metagenome-Assembled Genomes from Metagenomic Sequencing Data. Comput. Struct. Biotechnol. J. 19, 6301–6314. doi:10.1016/j.csbj.2021.11.028
Yarmush, M. L., and Jayaraman, A. (2002). Advances in Proteomic Technologies. Annu. Rev. Biomed. Eng. 4 (1), 349–373. doi:10.1146/annurev.bioeng.4.020702.153443
Ye, J., Yan, X., Qin, P., Gong, X., Li, H., Liu, Y., et al. (2022). Proteomic Analysis of Hypothalamus in Prepubertal and Pubertal Female Goat. J. proteomics 251, 104411. doi:10.1016/j.jprot.2021.104411
Zhang, B., and Horvath, S. (2005). A General Framework for Weighted Gene Co-expression Network Analysis. Stat. Appl. Genet. Mol. Biol. 4 (1), 4. doi:10.2202/1544-6115.1128
Zhang, C., Gao, Y., Liu, J., Xue, Z., Lu, Y., Deng, L., et al. (2018). PGG.Population: a Database for Understanding the Genomic Diversity and Genetic Ancestry of Human Populations. Nucleic acids Res. 46 (D1), D984–d993. doi:10.1093/nar/gkx1032
Zhang, S., Gao, X., Jiang, Y., Shen, Y., Xie, H., Pan, P., et al. (2021). Population Validation of Reproductive Gene Mutation Loci and Association with the Litter Size in Nubian Goat. Arch. Anim. Breed. 64 (2), 375–386. doi:10.5194/aab-64-375-2021
Zhao, Y., Wang, Y., Guo, F., Lu, B., Sun, J., Wang, J., et al. (2021). iTRAQ-based Proteomic Analysis of Sperm Reveals Candidate Proteins that Affect the Quality of Spermatozoa from Boars on Plateaus. Proteome Sci. 19 (1), 9. doi:10.1186/s12953-021-00177-9
Keywords: omics, selection, animal improvement, phenomics, data analysis
Citation: Chakraborty D, Sharma N, Kour S, Sodhi SS, Gupta MK, Lee SJ and Son YO (2022) Applications of Omics Technology for Livestock Selection and Improvement. Front. Genet. 13:774113. doi: 10.3389/fgene.2022.774113
Received: 11 September 2021; Accepted: 16 May 2022;
Published: 02 June 2022.
Edited by:
Sunday O. Peters, Berry College, United StatesReviewed by:
Suxu Tan, Michigan State University, United StatesCopyright © 2022 Chakraborty, Sharma, Kour, Sodhi, Gupta, Lee and Son. This is an open-access article distributed under the terms of the Creative Commons Attribution License (CC BY). The use, distribution or reproduction in other forums is permitted, provided the original author(s) and the copyright owner(s) are credited and that the original publication in this journal is cited, in accordance with accepted academic practice. No use, distribution or reproduction is permitted which does not comply with these terms.
*Correspondence: Neelesh Sharma, ZHJuZWVsZXNoX3NoYXJtYUB5YWhvby5jby5pbg==; Young Ok Son, c291bmFnaUBqZWp1bnUuYWMua3I=
Disclaimer: All claims expressed in this article are solely those of the authors and do not necessarily represent those of their affiliated organizations, or those of the publisher, the editors and the reviewers. Any product that may be evaluated in this article or claim that may be made by its manufacturer is not guaranteed or endorsed by the publisher.
Research integrity at Frontiers
Learn more about the work of our research integrity team to safeguard the quality of each article we publish.