- 1Division of Hematology/Oncology, Department of Pediatrics, The Seventh Affiliated Hospital of Sun Yat-Sen University, Shenzhen, China
- 2Department of Breast and Thyroid Surgery, Guangzhou Women and Children’s Medical Center, Guangzhou, China
Background: Tyrosyl phosphorylation is carried out by a group of enzymes known as non-receptor protein tyrosine phosphatases (PTPNs). In the current investigation, it is hoped to shed light on the relationships between the expression patterns of PTPN family members and the prognosis of acute myeloid leukemia (AML).
Methods: PTPN expression was examined using GEPIA and GEO databases. To investigate the connection between PTPN expression and survival in AML patients, we downloaded data from the Broad TCGA Firehose and Clinical Proteomic Tumor Analysis (CPTAC) of the Cancer Genome Atlas (TCGA). We used quantitative real-time PCR (qRT-PCR) to confirm that essential genes were performed in clinical samples and cell lines. We then used western blot to verify that the genes expressed in the above databases were positive in normal tissues, AML patient samples, and AML cell lines. Next, we investigated associations between genome-wide expression profiles and PTPN6 expression using the GEO datasets. We investigated the interactive exploration of multidimensional cancer genomics using the cBioPortal datasets. Using the DAVID database, a study of gene ontology enrichment was performed. The protein-protein interaction (PPI) network was created using the STRING portal, and the gene-gene interaction network was performed using GeneMANIA.
Results: Data from GEO and GEPIA revealed that most PTPN family members were linked to AML. Patients with leukemia have elevated levels of several PTPN members. All of the AML patients’ poor overall survival (OS, p < .05) was significantly linked with higher expression of PTPN1, PTPN6, and PTPN7. Additionally, clinical samples showed that the expression of PTPN 6, PTPN 7, PTPN 13, and PTPN 14 was higher than normal in AML patients (p = .0116, p = .0034, p = .0092, and p = .0057, respectively) and AML cell lines (p = .0004, p = .0035, p = .0357, and p = .0177, respectively). Western blotting results showed that the expression of PTPN6 in AML samples and AML cell lines was significantly higher than that in normal control samples.
Conclusion: Differentially expressed PTPN family members were found in AML. The prognosis of patients and PTPN gene expression were shown to be correlated. PTPN6 is one of these members and may be used as an AML diagnostic and prognostic marker.
Introduction
The most prevalent kind of acute leukemia in adults and the cause of the most significant number of leukemia-related fatalities each year in the United States is acute myeloid leukemia (AML), a heterogeneous hematologic malignancy characterized by the clonal growth of myeloid blasts in peripheral blood, bone marrow, and other organs (Tallman et al., 2019). In 2022, it is predicted that 20,050 people will be diagnosed with AML, and 11,540 people will pass away from the condition (Siegel et al., 2022). Despite improvements in AML treatment, such as the introduction of chemotherapy and other successful targeted medicines over the previous few decades, the 5-years relative survival rate increased from 6.2% in the mid- 1970s to 30% for those diagnosed from 2009 to 2015 (Lai et al., 2019). However, there are significant restrictions in the prognosis predicted by the existing biomarkers because of the clinical and molecular heterogeneity of AML (Patel et al., 2012). Therefore, it is vital to find reliable biomarkers that will allow for an earlier diagnosis and better, more specialized therapy of AML.
Complex phosphorylation and dephosphorylation networks are created when kinases and phosphatases, which carry out phosphorylation and dephosphorylation, are linked by their shared substrates or direct interactions. These networks are essential for controlling cellular functions (Li et al., 2013). Protein tyrosine phosphatases (PTPs) are a group of enzymes that catalyze the dephosphorylation of tyrosine residues (Chen et al., 2020). One hundred three genes encode PTPs, which are organized into four primary superfamily classes. The Human Genome Organization’s Nomenclature Committee has given each PTP member an official gene name (Ogino et al., 2007). There are 17 non-receptor PTPs in Class I of the most prominent family, known as PTPN, with a number, according to the literature (Alonso et al., 2004). More and more evidence points to the possibility that protein tyrosine kinases (PTKs) and protein tyrosine phosphatases (PTPNs) collaborate to control a wide range of cellular processes, including immune response, migration, metabolism, and proliferation and differentiation (Tonks, 2013; Yu and Zhang, 2018). The PTPN family numbers significantly influence various disorders, according to numerous research that has already been published. For instance, PTPN22 restricts T-cell receptor-induced proliferation. It hinders naive T-cell activation and effector cell responses in response to low-affinity antigens (Salmond et al., 2015), and PTPN12 expression is elevated in both stomach adenocarcinoma and cancer (Chen et al., 2020). Additionally, one study indicates that the deletion of PTPN2 may enhance the therapeutic effectiveness of CAR-T cells in the treatment of breast cancer (Wiede et al., 2020). The cell cycle, apoptosis, and metastasis are all heavily regulated by PTPN2, which is a significant predictor of the prognosis of pancreatic cancer (Kuang et al., 2022). The PTPN genes are generally a promising prognostic and therapeutic target for cancer therapy due to this evidence. However, the distinct roles of PTPN family genes in AML have yet to be understood entirely.
Therefore, discovering oncogenes or tumor suppressors mediated by PTPN as potential pathways for predicting biomarkers may offer novel therapeutic approaches for treating AML. The difficulty comes from the fact that most PTPN genes’ variances in transcriptional levels, prognostic values, molecular roles, and biological processes have not yet been thoroughly understood in the context of AML disease. In order to thoroughly investigate the association between PTPN subtypes and the pathogenesis and progression of AML, we combed through some widely used databases as part of this work to further our understanding of AML.
Materials and methods
Ethics statement
The Seventh Affiliated Hospital of Sun Yat-Sen University’s Academic Committee approved this study, which was carried out following the guidelines outlined in the Declaration of Helsinki. Each patient signed informed consent. Since all the datasets were taken from published works, it was verified that written informed consent had been obtained for every one of them.
Download and expression analysis of microarray data
The GEO database (http://www.ncbi.nlm.nih.gov/geo) is a public functional genomics data repository that downloads the GSE149237 microarray dataset (Jäger et al., 2021). This dataset was obtained by comparing five healthy HSPCs sequenced and compared to eight AML patient samples, and the study was conducted with GPL20301 Illumina HiSeq 4000 sequencing platform. Then, we performed a classification analysis on the mRNA expression values of the target genes. The filter conditions are p-value <0.05 and the absolute value of the difference (| log2 (Fold Change) |) > 1.
Download the data set GSE37642 and its corresponding platform file GPL96 (Affymetrix Human Genome U133A Array). The GSE37642 data set based on the GPL96 platform contains a total of 422 tissue samples (bone marrow mononuclear cells) of AML patients, and clinical information such as survival time, survival status, and whether PTPN6 mutations occur in the samples are extracted.
GEPIA dataset
GEPIA (Gene Expression Profiling Interactive Analysis) is a newly created interactive web server for evaluating the RNA sequencing expression data of 9,736 tumors and 8,587 normal samples from projects like the Genotype-Tissue Expression (GTEx) and the Cancer Genome Atlas (TCGA), using a regular processing pipeline. (http://gepia.cancer-pku.cn/). Customizable features offered by GEPIA include dimensionality reduction analysis, similar gene discovery, patient survival analysis, profiling based on cancer kinds or pathological stages, tumor or normal differential expression analysis, patient survival analysis, and similar gene detection (Tang et al., 2017).
LinkedOmics dataset
In the software ecosystem, LinkedOmics (http://www.linkedomics.orglogin.php) is a brand-new and unique tool for sharing data from extensive cancer omics initiatives. To reduce duplication of effort, which is concentrated on the detection and interpretation of attribute connections, preprocessed and normalized data from the Clinical Proteomic Tumor Analysis (CPTAC) data portal and the Broad TCGA Firehose are used, completing the work of the already-existing cancer data portals (Vasaikar et al., 2018).
Cell lines and cell culture
Four AML cells, HL-60, KG-1, THP-1, and MOLM-13, were purchased from American Type Culture Collection (Rockville, MD). HL-60 and KG-1 were cultured in IMDM medium (Invitrogen, Shanghai, China) supplemented with 20% fetal bovine serum (Biological Industries, Kibbutz Beit Haemek, Israel) and 100 units/ml penicillin and streptomycin. THP-1 and MOLM-13 were cultured in RPMI medium (Invitrogen, Shanghai, China) supplemented with 10% fetal bovine serum (Biological Industries, Kibbutz Beit Haemek, Israel) and 100 units/ml penicillin and streptomycin. Cells were incubated at 37°C in a humidified atmosphere of 95% air and 5% CO2, as described previously (Jin et al., 2015). The cells were confirmed to be mycoplasma-free routinely.
RNA extraction, reverse transcription and quantitative real-time polymerase chain reaction (qRT-PCR)
To verify the expression of crucial genes in clinical samples and cell lines, we further verified the expression level of essential genes in blood monocytes of four newly diagnosed patients with AML (confirmed by WHO-AML criteria, excluding AML-M3 cases. Not receive treatment was received) and four AML cell lines (HL-60, KG-1, THP-1, and MOLM-13) using qPCR. Peripheral blood monocytes from four anonymous healthy volunteers were used as control samples.
According to g Trizol reagent and the manufacturer’s instructions, total RNA was extracted from cultivated cells (Takara Bio, Kusatsu, Japan). The expression of the indicated genes was examined using SYBR Premix Ex Taq TM II and PCR detection equipment from Bio-Rad in Hercules, California, United States. A quick all-in-one RT-Kit was used to create the cDNA (ES Science Biotech). The internal control gene GAPDH’s transcript levels were used to standardize transcription levels. The supplementary table displays the order of the primers. Triplicate analyses of each RNA sample were carried out.
Reagents and antibodies
PTPN6 (Rabbit, 3759) and PTPN14 (Rabbit, 13,808) antibody was from Cell Signaling Technology (Beverly, MA). Antibodies against β-actin were from Sigma-Aldrich (Mouse, A5441, Shanghai, China). Antibodies against PTPN1(Goat, AF3954) was from Novus Biologicals (Littleton, CO). Antibodies against PTPN7(Goat, AF3954) was from Novus Biologicals (Littleton, CO). Antibodies against PTPN13 (Rabbit, PA5-72907) were from Thermo-Fisher Scientific (Shanghai, China). The fluorescent-conjugated secondary antibodies anti-mouse and anti-rabbit IgG were from LI-COR Biotechnology (Nebraska, United States).
For western blotting assays, whole cell lysates were prepared in RIPA buffer (1 × PBS, 1% NP-40, .5% sodium deoxycholate, .1% SDS) supplemented with 10 mmol/L β-glycerophosphate, 1 mmol/L sodium orthovanadate, 10 mmol/L NaF, 1 mmol/L phenylmethylsulfonyl fluoride, and 1 × Roche complete Mini protease inhibitor cocktail (Roche, Indianapolis, IN) (Jin et al., 2017). The cytosolic fractionations for cytochrome c detection were prepared with digitonin extraction buffer (10 mmol/L PIPES pH 6.8, .015% digitonin, 300 mmol/L sucrose, 100 mmol/L NaCl, 3 mmol/L MgCl2, 5 mmol/L EDTA, and 1 mmol/L phenylmethylsulfonyl fluoride) as described previously (Jin et al., 2017). Protein samples were separated by SDS-PAGE and transferred to nitrocellulose membranes, which were then incubated with the primary antibodies. After incubation with appropriate secondary antibodies, the membranes were scanned by the Odyssey infrared imaging system (LI-COR, Lincoln, Nebraska).
TCGA data and the cBioPortal
The cBioPortal (http://www.cbioportal.org/) for cancer genomics is an open-access and open-source platform developed for the interactive study of multidimensional cancer genomics datasets (Cerami et al., 2012). It supports and maintains data about non-synonymous mutations, DNA copy-numbers, mRNA and microRNA expression, protein-level and phosphoprotein levels, DNA methylation, and de-identified clinical data. We may compute mRNA expression z-scores (RNA Seq V2 RSEM), PTPN family gene correlations, and the frequency of gene modifications using the web tool cBioPortal.
GO and PPI analysis for the function and interaction of PTPN family
Enrichment analysis of gene ontology (GO) of PTPN genes was explored using the Database for Annotation, Visualization and Integrated Discovery (DAVID; v.6.8; https://david.ncifcrf.gov/home.jsp; accessed on 20 November 2019) (Chandrasekharan et al., 2013). The gene-gene interaction network was structured using the Gene Multiple Association Network Integration Algorithm (GeneMANIA; https://www.genemania.org/; accessed on 21 November 2019) (Warde-Farley et al., 2010) and the search tool for the Retrieval of Interacting Genes Database (STRING v.10.0; https://string-db.org/; accessed on 23 November 2019) was used to create a protein-protein interaction (PPI) network (Szklarczyk et al., 2017).
Statistical analysis
Utilizing the software packages R Studio (R version 4.0.2) and GraphPad Prism 8.3, statistical analysis and visualization were carried out (GraphPad Software, Inc., La Jolla, CA, United States). Single e-variable Cox proportional regression models and two-way ANOVA analysis were employed to examine the overall survival and mRNA expression datasets. An illustration of the prognosis was a Kaplan-Meier survival curve. We compared variations in central gene expression levels using two-way ANOVA analysis. Statistical significance was set at as < .05.
Results
Transcriptional level of PTPNs in patients with AML in the GEO database
The human genome contains PTPN genes, which have been found. We compared the transcriptional expression of the PTPN genes in tumorigenic and healthy control samples using the GSE149237 microarray dataset. GEO analysis revealed that the mRNA expression level of PTPN3, PTPN6, PTPN7, PTPN11, PTPN14, and PTPN18, was upregulated in AML patients compared with normal controls (p < .05, Figure 1). However, the expression levels of PTPN5, PTPN13, PTPN21, and PTPN22 were lower in AML patients (p < .05, Figure 1). While others, such as PTPN1, PTPN2, PTPN9, PTPN12, PTPN20, and PTPN23, showed no difference between AML and normal samples (p > .05, Supplementary Figure S1).
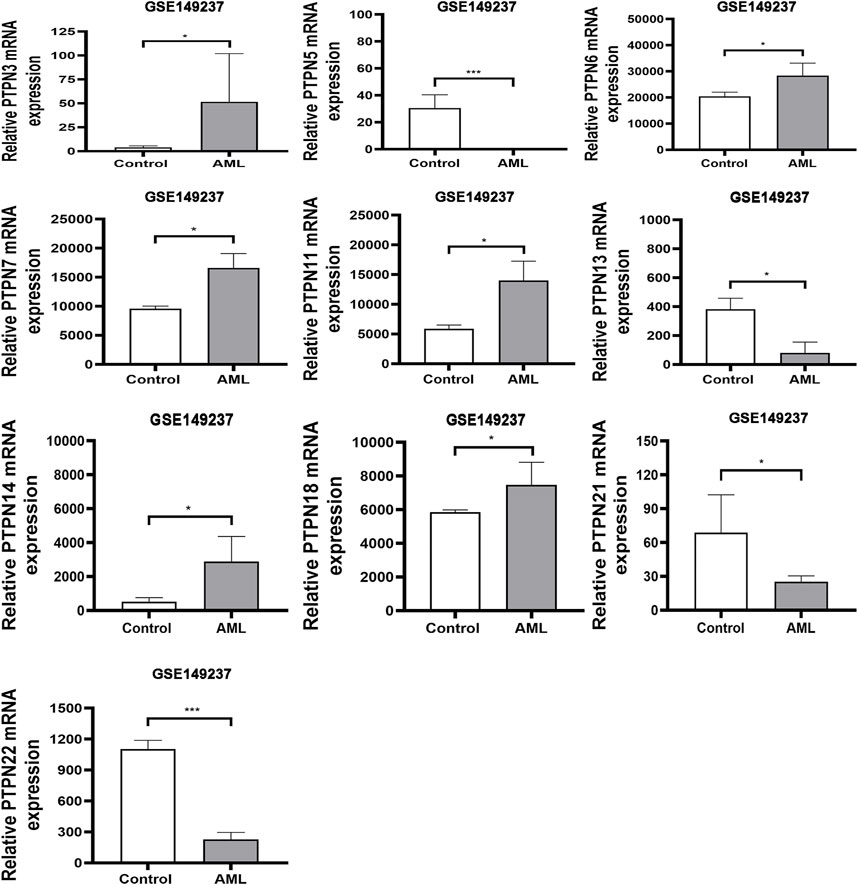
FIGURE 1. In the GSE149237 microarray dataset, there were differential transcript levels of the PTPN gene in AML and healthy control samples. The filter conditions are p-value <0.05 and the absolute value of the difference (| log2 (Fold Change) |) > 1.
The mRNA levels of PTPNs in AML samples and normal samples in the GEPIA
Database
In order to compare the mRNA expression of PTPN factors in leukemia and normal samples, we used the GEPIA (Gene Expression Profiling Interactive Analysis) dataset (http://gepia.cancer-pku.cn/). The findings showed that PTPN6, PTPN18, and PTPN22 had higher expression levels in leukemia than in normal blood samples, while PTPN1 had a lower expression level in the former than the latter (Figure 2). However, there was no discernible difference in the expression levels of PTPN2, PTPN3, PTPN4, PTPN5, PTPN7, PTPN9, PTPN 11, PTPN12, PTPN13, PTPN14, PTPN20, PTPN21, and PTPN23 (Supplementary Figure S2).
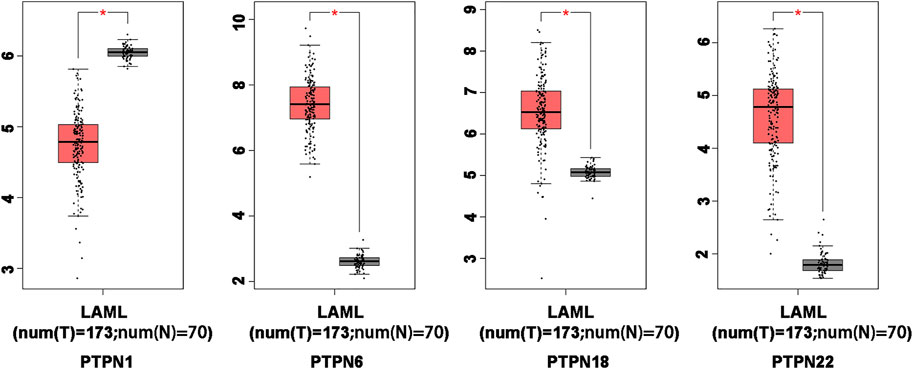
FIGURE 2. Differentially expressed PTPN genes in AML and healthy control samples in the GEPIA database. The filter conditions are p-value <0.05 and the absolute value of the difference (| log2 (Fold Change) |) > 1.
Relationship between the mRNA level of PTPNs and the prognosis of patients with leukemia
The role of PTPN genes in AML patients’ survival was the subject of our further research. We conducted a prognostic analysis of PTPN genes in patients with AML using the LinkedOmics database. The results of the study showed that there was a significant correlation between poor overall survival (OS, p < .05, Figure 3) and increased expression levels of PTPN1, PTPN6, and PTPN7 in all AML patients, but was associated with low expression of PTPN13 and PTPN14 (OS, p < .05, Figure 3). However, there were no significantly different in the expression levels of PTPN2, PTPN3, PTPN4, PTPN5, PTPN9, PTPN11, PTPN12, PTPN18, PTPN20, PTPN21, PTPN22, and PTPN23 (Supplementary Figure S3). PTPN1, PTPN6, and PTPN7 overexpression may indicate a poor prognosis for AML.
Clinical samples were used to verify the mRNA and protein levels of PTPNs in AML and normal samples
We next carried out qRT-PCR experiments utilizing clinical samples and cell lines to confirm further the expression level of PTPNs mRNA in AML. According to the findings, the expression of PTPN 6, PTPN 7, PTPN 13, and PTPN 14 was higher in AML patients (p = .0116, p = .0034, p = .0092, and p = .0057, respectively) and AML cell lines (p = .0004, p = .0035, p = .0357, and p = .0177, respectively) than in normal individuals. The expression level of PTPN1 was, however, lower in AML patients (p = .0094) and AML cell lines (p = .0013) (Figure 4A). However, there were no significantly different in the expression levels of PTPN2, PTPN3, PTPN4, PTPN5, PTPN9, PTPN11, PTPN12, PTPN18, PTPN20, PTPN21, PTPN22, and PTPN23 (Supplementary Figure S4A).
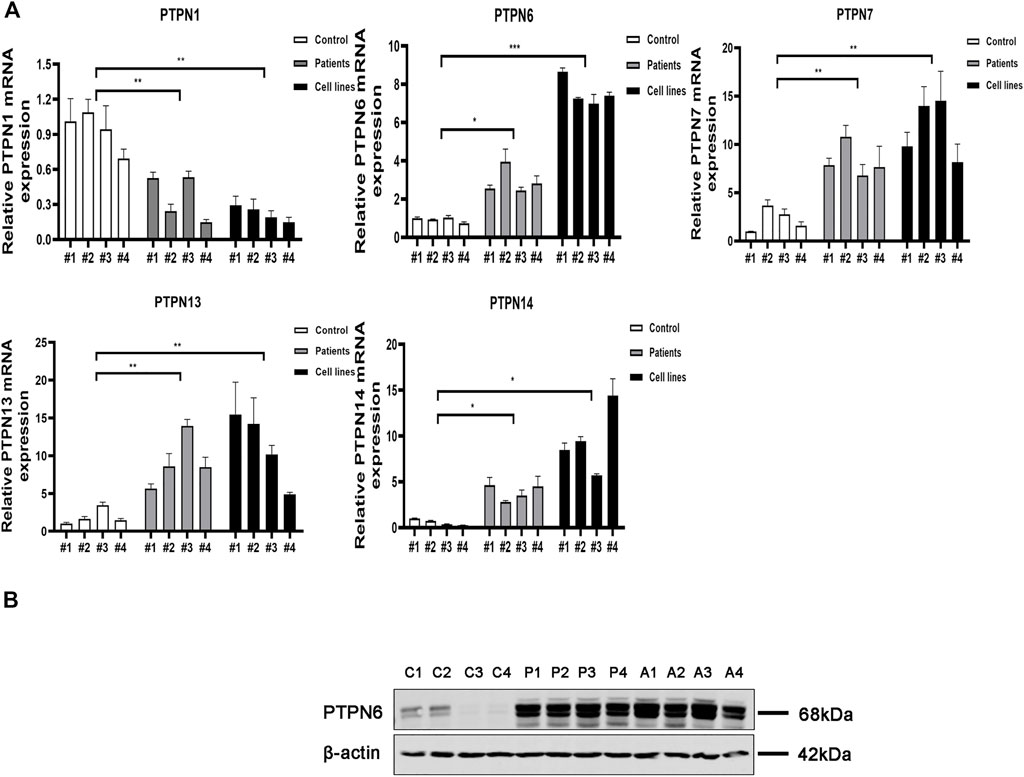
FIGURE 4. Relative mRNA and protein expression of the PTPN gene in AML samples and AML cell lines and normal controls were detected by qRT-PCR and western blotting. (A) Relative mRNA expression of PTPN gene in AML samples and AML cell lines and normal healthy controls detected by qRT-PCR. (B) Protein expression levels of PTPN6 in AML samples and AML cell lines and normal healthy controls detected by western blotting. Note: C1-C4 represent 4 normal controls, P1-P4 control 4 AML clinical samples, A1-A4 represent 4 AML cell lines including HL-60, KG-1, THP-1, and MOLM-13.
According to the results of GEO, GEPIA, LinkedOmics, and qRT-PCR, only PTPN6 expression was increased in AML patients in all major databases and qRT-PCR, and the results were consistent with each other. Next, we explored the protein expression of PTPN 1, PTPN6, PTPN 7, PTPN 13, and PTPN 14 in normal controls, clinical AML patients, and AML cell lines. The results of western blotting assays showed that the expression of PTPN6 in AML patient samples and AML cell lines was significantly higher than that in normal controls (Figure 4B). However, the expression levels of PTPN1, PTPN7, PTPN13 and PTPN14 were not different among AML patient samples, AML cell lines and normal controls (Supplementary Figure S4B).
Associations between genome-wide expression profiles and PTPN6 expression
In order to further study the biological role of PTPN6 in leukemogenesis, the gene expression profile related to PTPN6 was obtained based on the analysis of GSE dataset 37,642. As a result, 128 upregulated genes and 390 downregulated genes were identified as being significantly associated with the expression of PTPN6 (fdr-adjusted p < .05 and FC > 1.5 or FC < 1/1.5, Figure 5A). In addition, we also presented these differentially expressed genes as a heat map (Figure 5B). The upregulated genes include: 1) Genes related to leukemia (such as HHEX, NET1), tumor-promoting factors (such as CDK6, HOX family genes), tyrosine kinase genes (c-KIT, GRB10); 2) Prognosis-related genes (such as WT1, CXXC5, MSI2, etc.); 3) CD34 (a marker of hematopoietic progenitor cells); 4) AML drug resistance-related genes (such as IGFBP2 and ABCC1). Downregulated genes include: 1) Immune system activators, such as CD86; 2) blood tumor suppressors ID2 and KLF4; 3) CEBPB, CEBPB is a BCR/ABL negative regulator gene, which can inhibit the proliferation of BCR/ABL-positive cells, and promote cell differentiation.
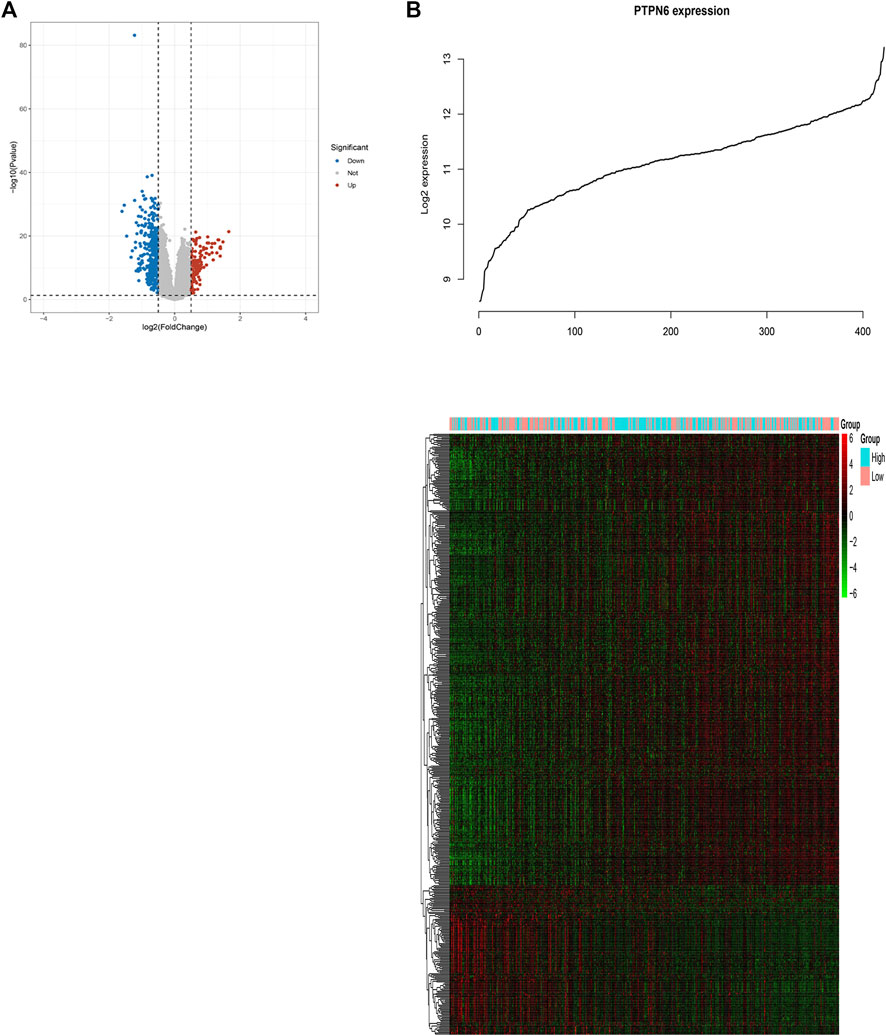
FIGURE 5. Genome-wide genes associated with PTPN6 expression. (A) Volcano plot of differential gene profiles between PTPN6 high and PTPN6 low. (B) Expression heatmap of PTPN6-associated genes. The top curve shows PTPN6’s expression distribution of 156 CN-AML samples.
Genetic alterations and correlations of PTPN genes in AML
We gathered details on genetic changes to the PTPN genes and determined any gene-to-gene correlations using the online resource cBioPortal and the “TCGA, Firehose Legacy” database for AML. In 68/163 (42%) patient samples with AML, PTPN genes were changed (Figure 6A). Mutation, deep deletion, mRNA overexpression, mRNA down-expression, and numerous alterations were among the several genetic alterations. The percentage of genetic alterations in PTPN family members for leukemia varied from .6% to 9% for individual genes based on the TCGA, Firehose Legacy dataset (PTPN1, 6%; PTPN2, 5%; PTPN3, 1.2%; PTPN4, 3%; PTPN5, 4%; PTPN6, 5%; PTPN7, 3%; PTPN9, 3%; PTPN11, 9%; PTPN12, 6%; PTPN13, 4%; PTPN14, 6%; PTPN18, 5%; PTPN20, .6%; PTPN21, 5%; PTPN22, 4%; PTPN23, 1.8%; Figure 6A). In addition, cBioPortal was used to investigate the expression of PTPN genes in AML [using mRNA sequencing (RNA-seq) version V2 RSEM], and the relationships between certain PTPN genes (including Pearson’s correlation) were calculated. Except for PTPN1 and PTPN22, the findings showed a significant positive correlation between any two PTPN family gene members (Pearson = .00952, p = .905) (Figure 6B).
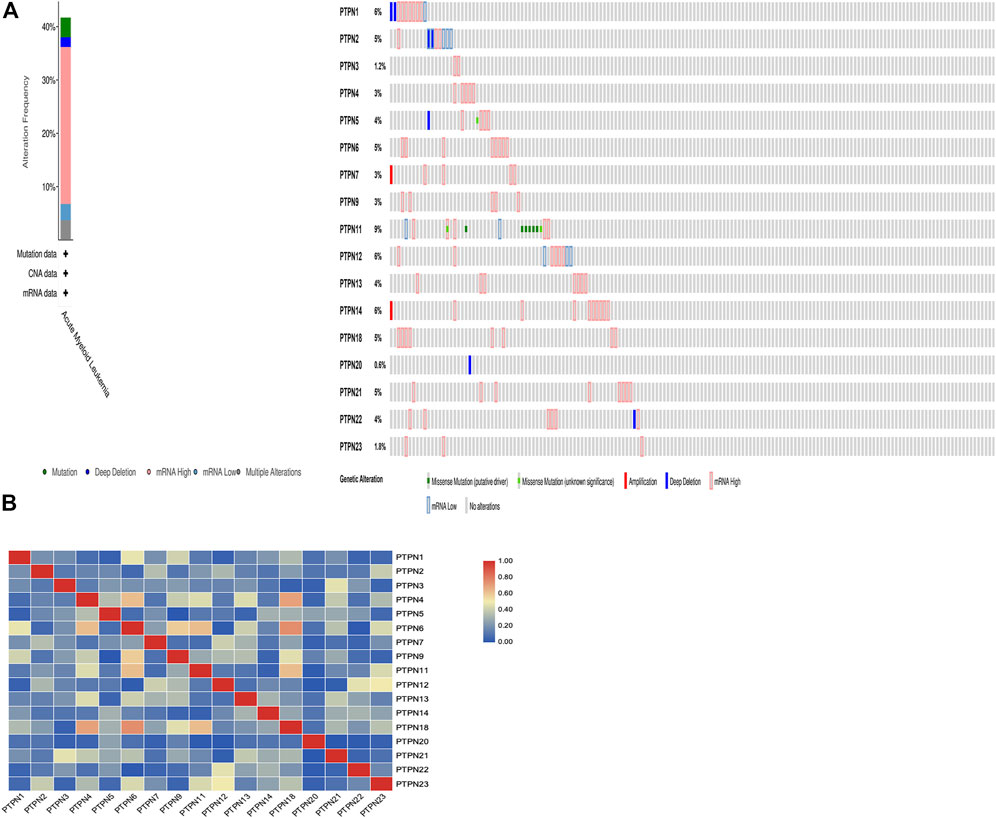
FIGURE 6. Genetic alteration and correlations of PTPN genes in AML. (A) Genetic alteration of PTPN gene in AML. (B) Genetic correlations of PTPN genes in AML.
Function and interaction of PTPN family genes
The three main categories of GO analysis were molecular function groups, cellular component groups, and biological process groups. Figure 7A displays the top five enriched categories for each group as determined by the analysis results. GO analysis revealed that most PTPN proteins were associated with the cytoplasm. Protein dephosphorylation and protein tyrosine phosphatase activity were the main targets of PTPN genes’ actions. The KEGG pathway enriched 11 genes using the David online platform. JAK-STAT signaling pathway, Adherens junction, PD-L1 expression, and PD-1 checkpoint pathway in cancer, as well as insulin resistance and natural killer cell-mediated cytotoxicity, were the principal pathways associated with the eleven genes (Figure 7B). To further understand the relationships between colocalization, shared protein domains, co-expression, prediction, and pathways, interaction analysis of PTPN genes at the gene level was carried out using GeneMANIA (Figure 7C). The STRING protein-protein interaction network analysis revealed that the connections between the members of the PTPN gene family were complex (Figure 7D).
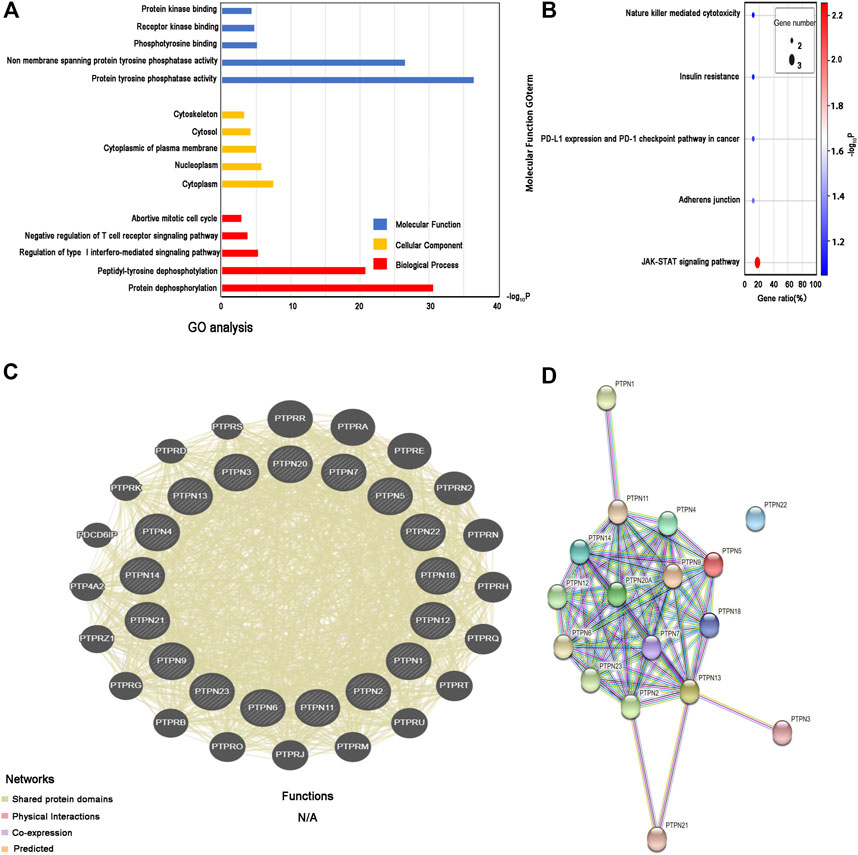
FIGURE 7. Enrichment and correlation analysis among PTPN family genes. (A) GO analysis of PTPN family genes. The top five enriched categories for Molecular Function, Cellular Component, and Biological Process were showed. (B) KEGG pathway analysis of PTPN family genes, top five KEGG pathway category were showed. (C) Gene–gene interaction network among PTPN gene family members. (D) Protein–protein interaction network among PTPN gene family members.
Discussion
According to the Global Burden of Disease study, acute myeloid leukemia (AML) is the deadliest form of leukemia in the world, with 147,000 fatalities attributed to it in 2019 (Fitzmaurice et al., 2019; GBD 2019 Diseases and Injuries Collaborators, 2020) AML is linked to 40% of leukemia-related mortality in the United States and around the world, but only about 30% of leukemia occurrences (Siegel et al., 2021). AML incidence has increased more during the previous 10 years than chronic lymphocytic leukemia (CLL) (34% vs 25%), acute lymphoblastic leukemia (ALL) (22%), and chronic myeloid leukemia (13%), in part due to population expansion and aging as well as an increase in the standardized incidence rate (Cao et al., 2021). Therefore, it is noteworthy and crucial for AML that new biological therapeutic targets are found.
Together with protein-tyrosine kinases, PTPN regulates tyrosine phosphorylation and dephosphorylation in cellular signal transmission. They are essential members of the protein tyrosine phosphatases family (Tonks, 2006). Numerous studies have examined the relationships between specific PTPN family members and various neoplasms (Chen et al., 2020; Mitra and Ayyannan, 2020). To date, no report has provided an overview of the connections between PTPN family genes and human leukemia. In this study, PTPN gene expression patterns were illustrated for the first time, and it was also shown how the PTPN family of genes and AML diagnosis and prognosis are related. This information gave genes clearer understanding of the clinical value of all the PTPN genes in human leukemia.
We investigated the level of PTPN gene expression in AML using GEO database and the online databases GEPIA, Next, we used the LinkedOmics database to explore the relationship between the PTPN family’s expression and AML patients’ survival. Then we validated our findings using qRT-PCR. As a result of the high levels of expression of numerous PTPN family members in human AML discovered in our study, PTPNs may 1 day be used as an AML diagnostic biomarker. However, there is much debate about the expression of the PTPN family in AML and normal samples. The expression level of PTPN1 in AML mice is significantly lower than in normal mice, and myeloid-specific deficiency of PTPN1 can promote the development of AML (Le Sommer et al., 2018). In the GSE149237 microarray, there was no difference in the expression of PTPN1 in AML and normal samples. In contrast to the GEPIA database and RT-PCR, which revealed the opposite findings. This suggests that the expression and role of PTPN1 in AML are still controversial, and further studies are needed to explore and clarify. PTPN2 is frequently absent in adult and pediatric T-ALL patients, but the expression level of PTPN1 does not correlate with patient prognosis (Alcantara et al., 2019). Similar to our findings, PTPN2 expression did not differ between AML and normal samples and was not associated with patient prognosis. PTPN3 is an oncoprotein with a poor prognosis and can promote cell invasion and proliferation in biliary cancer (Wang et al., 2018). PTPN4 is highly expressed in AML and can guide the classification of AML (Kabir et al., 2013). PTPN5 act as a tumor suppressor in breast cancer (Palaniappan et al., 2018), but previous studies have not found differences in the expression of PTPN5 between AML and normal samples. Our findings demonstrated that, PTPN3, PTPN4, and PTPN5 were not differentially expressed in AML and normal blood, and there was no correlation between their expression levels and patient survival. Several transmembrane receptors’ intracellular signaling is modulated by PTPN6 (SHP-1). When SHP-1 expression and activity are reduced, JAK kinase activity is raised, which causes aberrant cell proliferation (Wu et al., 2003). Activation of PTPN6 (SHP-1) recruits CAMK1 to mediate self-renewal of AML (Hao et al., 2021). Compared to samples from AML patients and AML cell lines, the expression of PTPN6 was significantly lower in our study’s normal samples (whether in GEO and GEPIA databases or RT-PCR and western blotting results). Further evidence that PTPN6 can be employed as a marker for the diagnosis and prognosis of AML comes from the substantial correlation between the high expression of PTPN6 and the poor survival of AML patients. Cytoplasmic protein tyrosine phosphatase PTPN7, sometimes referred to as hematopoietic PTP, was first cloned from human T-cells. Since PTPN7 dephosphorylated ERK, T-cell activation was decreased in T cells derived from PTPN7-KO mice, as seen by the hyperphosphorylation of ERK on those cells (Inamdar et al., 2019). Our results from GEO and RT-PCR showed that PTPN7 expression was lower in normal samples than in AML patients. However, the GEPIA results showed no statistically significant difference between the two. In contrast, the cytoplasmic PTP PTPN9 is broadly distributed in the tissues of the brain, leukocytes, endocrine cells, and other cells (Wang et al., 2019). PTPN11 is involved in many signal transduction functions necessary for normal hematopoiesis, and mutations in PTPN11 can mediate the development of AML and are associated with poor prognosis (Alfayez et al., 2021). PTPN12 is highly expressed in AML samples (Arora et al., 2012). Our results showed that in the GSE149237 microarray dataset, the expression of PTPN11 was higher in AML than in normal controls, but in the GEPIA database, there was no difference between the two. The expression of PTPN9 and PTPN12 did not differ between AML patients and normal controls, neither in the GEO nor the GEPIA database. Transcription levels of PTPN13 are abnormally elevated in myeloid malignancies (Mundle et al., 1999). PTPN14 is now considered a tumor suppressor, but its expression and role in AML have not been studied and reported (Au et al., 2010). Our results showed the opposite. In the analysis of the GEO database, PTPN13 was higher in AML than normal controls, while PTPN14 was the utter opposite. In contrast, GEPIA results showed PTPN13 and PTPN14 expression in AML and normal controls with no difference. RT-PCR results showed that the expression of PTPN13 and PTPN14 was higher in AML and AML cell lines than in the control group. Only brain tissues express PTPN20 (Xia et al., 2018), and overexpression of PTPN21 promotes the proliferation of ALL cells by activating the mitogen-activated protein kinase (MAPK) signaling pathway (Wang et al., 2020). In our study, PTPN20 expression did not differ between AML and normal samples. However, the expression results of PTPN21 in AML and normal samples in the GSE149237 microarray dataset and GEPIA database were inconsistent. PTPN23 is required for AML cell survival (Zhang et al., 2017). However, our results showed that normal samples had higher expression levels of PTPN23 than AML samples. Furthermore, the expression of PTPN23 in AML patients was not associated with the prognosis of the patients. Combined with the GSE149237 microarray dataset, GEPIA database, LinkedOmics database, RT-PCR, and western blotting results. PTPN6 can be used as a marker for the diagnosis and prognosis of AML. However, further research and testing are needed to determine its association with AML risk.
Significant genomic genes of genetic alterations include changing the genetic code, inducing gene disruptions, and producing phenotypic differences (Egger et al., 2004; Zhou et al., 2016; Wu and Xu, 2020). An abnormal expression and PTPN dysfunction in AML can result from altered PTPN genes’ chromosomal structure. In our study, 68/163 (42%) of the patient samples with AML had PTPN gene alterations, which included mutations, deep deletions, mRNA overexpression, mRNA down-expression, and multiple alterations. PTPN11 mutations are frequently associated with acute myelomonocytic/monocytic leukemia subtypes, and PTPN11 is associated with lower rates of complete remission and shorter overall survival (Alfayez et al., 2021). Our study shows that PTPN11 has the highest probability of mutation, followed by PTPN1, PTPN12, PTPN12, and PTPN6. These results suggest that high genetic alterations in the PTPN gene are related to the development of AML.
GO analysis in this study showed that the PTPN protein was primarily related with the cytoplasm. The primary functions of PTPN genes, which have been extensively documented in various publications, are protein tyrosine phosphatase activity and dephosphorylation. PTPN11 contains two N-terminal Src homology 2 (SH2) domains, a protein tyrosine phosphatase (PTP) catalytic domain, and a COOH terminus. PTPN11 further contributes to the transformation of AML by encoding a ubiquitously expressed cytoplasmic phosphatase SHP2, which mediates cellular responses to hormones and cytokines (Stasik et al., 2021). Additionally, the outcomes of interaction network analysis at the gene and protein levels further suggested extensive interactions between PTPN members and other genes. However, few studies have examined interactions between PTPN members in AML. According to one investigation, the dephosphorylation of IRF3 at Y245 is mediated by a protein that both PTPN1 and PTPN2 target (Xia et al., 2019). By dephosphorylating protein tyrosine kinases unique to lymphocytes, PTPN2 and PTPN22 have been found to affect T-cell receptor signaling (Cloutier and Veillette, 1999; Wiede et al., 2011). Multiple studies showed that PTPN5 and PTPN7 might bind to and inactivate the mitogen-activated protein kinases Erk2 and P38, which could negatively influence cell proliferation and differentiation (Francis et al., 2011; Francis et al., 2013; Francis et al., 2014). It has been noted that diffuse large B-cell lymphomas are prevented from progressing by the hypermethylation of the PTPN6 and PTPN13 promoters (Wang et al., 2016). In our study, the prognosis of patients with AML was related to PTPN6, PTPN7, PTPN13, and PTPN13. Therefore, PTPN members have the potential to play the role of numerous diseases, including AML, via consortium processes.
Our results demonstrate the expression status and prognostic value of PTPN members in AML. The results showed the differential expression of some PTPN members and their correlation with the prognosis of AML. These members’ samples from healthy people had significantly lower levels of PTPN6 expression than samples from AML patients. Furthermore, patients with AML had significantly worse survival rates when their PTPN6 expression was higher. PTPN6 may be employed as a diagnostic and prognostic marker for AML, according to our findings. To further develop the therapeutic applicability of PTPNs, further well-designed studies are required to explain the importance of our findings.
Data availability statement
The datasets presented in this study can be found in online repositories. The names of the repository/repositories and accession number(s) can be found in the article/Supplementary Material.
Ethics statement
The studies involving human participants were reviewed and approved by Academic Committee of Seventh Affiliated Hospital of Sun Yat-Sen University. The patients/participants provided their written informed consent to participate in this study.
Author contributions
CC designed the paper. YL and CC are responsible for the conceptualization of the paper. CC performed the formal analysis. YL and CC wrote and corrected the manuscript. All authors contributed to the article and approved the submitted version.
Acknowledgments
We thank Sanming Project of Medicine in Shenzhen (No. SZSM202011004) to support the design of the study, Shenzhen Healthcare Research Project (Grant No. SZLY2018001), and Shenzhen Science and Technology Innovation Commission (JCYJ20180307150419435 and JCYJ20210324123004011) to support the manuscript preparation and publication.
Conflict of interest
The authors declare that the research was conducted in the absence of any commercial or financial relationships that could be construed as a potential conflict of interest.
Publisher’s note
All claims expressed in this article are solely those of the authors and do not necessarily represent those of their affiliated organizations, or those of the publisher, the editors and the reviewers. Any product that may be evaluated in this article, or claim that may be made by its manufacturer, is not guaranteed or endorsed by the publisher.
Supplementary material
The Supplementary Material for this article can be found online at: https://www.frontiersin.org/articles/10.3389/fgene.2022.1087938/full#supplementary-material
References
Alcantara, M., Simonin, M., Lhermitte, L., Touzart, A., Dourthe, M. E., Latiri, M., et al. (2019). Clinical and biological features of PTPN2-deleted adult and pediatric T-cell acute lymphoblastic leukemia. Blood Adv. 3 (13), 1981–1988. doi:10.1182/bloodadvances.2018028993
Alfayez, M., Issa, G. C., Patel, K. P., Wang, F., Wang, X., Short, N. J., et al. (2021). The Clinical impact of PTPN11 mutations in adults with acute myeloid leukemia. Leukemia 35 (3), 691–700. doi:10.1038/s41375-020-0920-z
Alonso, A., Sasin, J., Bottini, N., Friedberg, I., Friedberg, I., Osterman, A., et al. (2004). Protein tyrosine phosphatases in the human genome. Cell 117 (6), 699–711. doi:10.1016/j.cell.2004.05.018
Arora, D., Köthe, S., van den Eijnden, M., Hooft van Huijsduijnen, R., Heidel, F., Fischer, T., et al. (2012). Expression of protein-tyrosine phosphatases in acute myeloid leukemia cells: FLT3 ITD sustains high levels of DUSP6 expression. Cell Commun. Signal 10 (1), 19. doi:10.1186/1478-811X-10-19
Au, A. C., Hernandez, P. A., Lieber, E., Nadroo, A. M., Shen, Y. M., Kelley, K. A., et al. (2010). Protein tyrosine phosphatase PTPN14 is a regulator of lymphatic function and choanal development in humans. Am. J. Hum. Genet. 87 (3), 436–444. doi:10.1016/j.ajhg.2010.08.008
Cao, W., Chen, H. D., Yu, Y. W., Li, N., and Chen, W. Q. (2021). Changing profiles of cancer burden worldwide and in China: A secondary analysis of the global cancer statistics 2020. Chin. Med. J. Engl. 134 (7), 783–791. doi:10.1097/CM9.0000000000001474
Cerami, E., Gao, J., Dogrusoz, U., Gross, B. E., Sumer, S. O., Aksoy, B. A., et al. (2012). The cBio cancer genomics portal: An open platform for exploring multidimensional cancer genomics data. Cancer Discov. 2 (5), 401–404. doi:10.1158/2159-8290.CD-12-0095
Chandrasekharan, U. M., Dechert, L., Davidson, U. I., Waitkus, M., Mavrakis, L., Lyons, K., et al. (2013). Release of nonmuscle myosin II from the cytosolic domain of tumor necrosis factor receptor 2 is required for target gene expression. Sci. Signal 6 (284), ra60. doi:10.1126/scisignal.2003743
Chen, J., Zhao, X., Yuan, Y., and Jing, J. J. (2020). The expression patterns and the diagnostic/prognostic roles of PTPN family members in digestive tract cancers. Cancer Cell Int. 20, 238. doi:10.1186/s12935-020-01315-7
Cloutier, J. F., and Veillette, A. (1999). Cooperative inhibition of T-cell antigen receptor signaling by a complex between a kinase and a phosphatase. J. Exp. Med. 189 (1), 111–121. doi:10.1084/jem.189.1.111
Egger, G., Liang, G., Aparicio, A., and Jones, P. A. (2004). Epigenetics in human disease and prospects for epigenetic therapy. Nature 429 (6990), 457–463. doi:10.1038/nature02625
Fitzmaurice, C., Abate, D., Abbasi, N., Abbastabar, H., Abd-Allah, F., Abdel-Rahman, O., et al. (2019). Global, regional, and national cancer incidence, mortality, years of life lost, years lived with disability, and disability-adjusted life-years for 29 cancer groups, 1990 to 2017: A systematic analysis for the global burden of disease study. JAMA Oncol. 5 (12), 1749–1768. doi:10.1001/jamaoncol.2019.2996
Francis, D. M., Koveal, D., Tortajada, A., Page, R., and Peti, W. (2014). Interaction of kinase-interaction-motif protein tyrosine phosphatases with the mitogen-activated protein kinase ERK2. PLoS One 9 (3), e91934. doi:10.1371/journal.pone.0091934
Francis, D. M., Różycki, B., Koveal, D., Hummer, G., Page, R., and Peti, W. (2011). Structural basis of p38α regulation by hematopoietic tyrosine phosphatase. Nat. Chem. Biol. 7 (12), 916–924. doi:10.1038/nchembio.707
Francis, D. M., Kumar, G. S., Koveal, D., Tortajada, A., Page, R., and Peti, W. (2013). The differential regulation of p38α by the neuronal kinase interaction motif protein tyrosine phosphatases, a detailed molecular study. Structure 21 (9), 1612–1623. doi:10.1016/j.str.2013.07.003
GBD 2019 Diseases and Injuries Collaborators (2020). Global burden of 369 diseases and injuries in 204 countries and territories, 1990-2019: A systematic analysis for the global burden of disease study 2019. Lancet 396, 1204–1222. doi:10.1016/S0140-6736(20)30925-9
Hao, F., Wang, C., Sholy, C., Cao, M., and Kang, X. (2021). Strategy for leukemia treatment targeting SHP-1, 2 and SHIP. Front. Cell Dev. Biol. 9, 730400. doi:10.3389/fcell.2021.730400
Inamdar, V. V., Reddy, H., Dangelmaier, C., Kostyak, J. C., and Kunapuli, S. P. (2019). The protein tyrosine phosphatase PTPN7 is a negative regulator of ERK activation and thromboxane generation in platelets. J. Biol. Chem. 294 (33), 12547–12554. doi:10.1074/jbc.RA119.007735
Jäger, P., Geyh, S., Twarock, S., Cadeddu, R. P., Rabes, P., Koch, A., et al. (2021). Acute myeloid leukemia-induced functional inhibition of healthy CD34+ hematopoietic stem and progenitor cells. Stem Cells 39 (9), 1270–1284. doi:10.1002/stem.3387
Jin, Y., Cao, Q., Chen, C., Du, X., Jin, B., and Pan, J. (2015). Tenovin-6-mediated inhibition of SIRT1/2 induces apoptosis in acute lymphoblastic leukemia (ALL) cells and eliminates ALL stem/progenitor cells. BMC Cancer 15, 226. doi:10.1186/s12885-015-1282-1
Jin, B., Wang, C., Li, J., Du, X., Ding, K., and Pan, J. (2017). Anthelmintic niclosamide disrupts the interplay of p65 and FOXM1/β-catenin and eradicates leukemia stem cells in chronic myelogenous leukemia. Clin. Cancer Res. 23 (3), 789–803. doi:10.1158/1078-0432.CCR-16-0226
Kabir, N. N., Rönnstrand, L., and Kazi, J. U. (2013). Deregulation of protein phosphatase expression in acute myeloid leukemia. Med. Oncol. 30 (2), 517. doi:10.1007/s12032-013-0517-8
Kuang, W., Wang, X., Ding, J., Li, J., Ji, M., Chen, W., et al. (2022). PTPN2, A key predictor of prognosis for pancreatic adenocarcinoma, significantly regulates cell cycles, apoptosis, and metastasis. Front. Immunol. 13, 805311. doi:10.3389/fimmu.2022.805311
Lai, C., Doucette, K., and Norsworthy, K. (2019). Recent drug approvals for acute myeloid leukemia. J. Hematol. Oncol. 12 (1), 100. doi:10.1186/s13045-019-0774-x
Le Sommer, S., Morrice, N., Pesaresi, M., Thompson, D., Vickers, M. A., Murray, G. I., et al. (2018). Deficiency in protein tyrosine phosphatase PTP1B shortens lifespan and leads to development of acute leukemia. Cancer Res. 78 (1), 75–87. doi:10.1158/0008-5472.CAN-17-0946
Li, X., Wilmanns, M., Thornton, J., and Köhn, M. (2013). Elucidating human phosphatase-substrate networks. Sci. Signal 6 (275), rs10. doi:10.1126/scisignal.2003203
Mitra, R., and Ayyannan, S. R. (2020). Small-molecule inhibitors of Shp2 phosphatase as potential chemotherapeutic agents for glioblastoma: A minireview. ChemMedChem 16, 777–787. doi:10.1002/cmdc.202000706
Mundle, S. D., Mativi, B. Y., Bagai, K., Feldman, G., Cheema, P., Gautam, U., et al. (1999). Spontaneous down-regulation of Fas-associated phosphatase-1 may contribute to excessive apoptosis in myelodysplastic marrows. Int. J. Hematol. 70 (2), 83–90.
Ogino, S., Gulley, M. L., den Dunnen, J. T., and Wilson, R. B. (2007). Standard mutation nomenclature in molecular diagnostics: Practical and educational challenges. J. Mol. Diagn 9 (1), 1–6. doi:10.2353/jmoldx.2007.060081
Palaniappan, M., Edwards, D., Creighton, C. J., Medina, D., and Conneely, O. M. (2018). Reprogramming of the estrogen responsive transcriptome contributes to tamoxifen-dependent protection against tumorigenesis in the p53 null mammary epithelial cells. PLoS One 13 (3), e0194913. doi:10.1371/journal.pone.0194913
Patel, J. P., Gönen, M., Figueroa, M. E., Fernandez, H., Sun, Z., Racevskis, J., et al. (2012). Prognostic relevance of integrated genetic profiling in acute myeloid leukemia. N. Engl. J. Med. 366 (12), 1079–1089. doi:10.1056/NEJMoa1112304
Salmond, R. J., Brownlie, R. J., and Zamoyska, R. (2015). Multifunctional roles of the autoimmune disease-associated tyrosine phosphatase PTPN22 in regulating T cell homeostasis. Cell Cycle 14 (5), 705–711. doi:10.1080/15384101.2015.1007018
Siegel, R. L., Miller, K. D., Fuchs, H. E., and Jemal, A. (2021). Cancer statistics, 2017. CA Cancer J. Clin. 71 (1), 7–30. doi:10.3322/caac.21387
Siegel, R. L., Miller, K. D., Fuchs, H. E., and Jemal, A. (2022). Cancer statistics, 2016. CA Cancer J. Clin. 72 (1), 7–30. doi:10.3322/caac.21332
Stasik, S., Eckardt, J. N., Kramer, M., Röllig, C., Krämer, A., Scholl, S., et al. (2021). Impact of PTPN11 mutations on clinical outcome analyzed in 1529 patients with acute myeloid leukemia. Blood Adv. 5 (17), 3279–3289. doi:10.1182/bloodadvances.2021004631
Szklarczyk, D., Morris, J. H., Cook, H., Kuhn, M., Wyder, S., Simonovic, M., et al. (2017). The STRING database in 2017: Quality-controlled protein-protein association networks, made broadly accessible. Nucleic Acids Res. 45 (D1), D362–D368. doi:10.1093/nar/gkw937
Tallman, M. S., Wang, E. S., Altman, J. K., Appelbaum, F. R., Bhatt, V. R., Bixby, D., et al. (2019). Acute myeloid leukemia, version 3.2019, NCCN clinical practice guidelines in oncology. J. Natl. Compr. Canc Netw. 17 (6), 721–749. doi:10.6004/jnccn.2019.0028
Tang, Z., Li, C., Kang, B., Gao, G., Li, C., and Zhang, Z. (2017). Gepia: A web server for cancer and normal gene expression profiling and interactive analyses. Nucleic Acids Res. 45 (W1), W98–W102. doi:10.1093/nar/gkx247
Tonks, N. K. (2006). Protein tyrosine phosphatases: From genes, to function, to disease. Nat. Rev. Mol. Cell Biol. 7 (11), 833–846. doi:10.1038/nrm2039
Tonks, N. K. (2013). Protein tyrosine phosphatases--from housekeeping enzymes to master regulators of signal transduction. Febs J. 280 (2), 346–378. doi:10.1111/febs.12077
Vasaikar, S. V., Straub, P., Wang, J., and Zhang, B. (2018). LinkedOmics: Analyzing multi-omics data within and across 32 cancer types. Nucleic Acids Res. 46 (D1), D956–D63. doi:10.1093/nar/gkx1090
Wang, W., Wang, J., Li, Z., Zhu, M., Zhang, Z., Wang, Y., et al. (2016). Promoter hypermethylation of PTPL1, PTPN6, DAPK, p16 and 5-azacitidine inhibits growth in DLBCL. Oncol. Rep. 35 (1), 139–146. doi:10.3892/or.2015.4347
Wang, Y., Su, Y., Ji, Z., and Lv, Z. (2018). High expression of PTPN3 predicts progression and unfavorable prognosis of glioblastoma. Med. Sci. Monit. 24, 7556–7562. doi:10.12659/MSM.911531
Wang, D., Cheng, Z., Zhao, M., Jiao, C., Meng, Q., Pan, H., et al. (2019). PTPN9 induces cell apoptosis by mitigating the activation of Stat3 and acts as a tumor suppressor in colorectal cancer. Cancer Manag. Res. 11, 1309–1319. doi:10.2147/CMAR.S187001
Wang, H., Zhu, N., Ye, X., Wang, L., Wang, B., Shan, W., et al. (2020). PTPN21-CDSlong isoform inhibits the response of acute lymphoblastic leukemia cells to NK-mediated lysis via the KIR/HLA-I axis. J. Cell Biochem. 121 (5-6), 3298–3312. doi:10.1002/jcb.29601
Warde-Farley, D., Donaldson, S. L., Comes, O., Zuberi, K., Badrawi, R., Chao, P., et al. (2010). The GeneMANIA prediction server: Biological network integration for gene prioritization and predicting gene function. Nucleic Acids Res. 38, W214–W220. doi:10.1093/nar/gkq537
Wiede, F., Shields, B. J., Chew, S. H., Kyparissoudis, K., van Vliet, C., Galic, S., et al. (2011). T cell protein tyrosine phosphatase attenuates T cell signaling to maintain tolerance in mice. J. Clin. Invest. 121 (12), 4758–4774. doi:10.1172/JCI59492
Wiede, F., Lu, K. H., Du, X., Liang, S., Hochheiser, K., Dodd, G. T., et al. (2020). PTPN2 phosphatase deletion in T cells promotes anti-tumour immunity and CAR T-cell efficacy in solid tumours. Embo J. 39 (2), e103637. doi:10.15252/embj.2019103637
Wu, C., Sun, M., Liu, L., and Zhou, G. W. (2003). The function of the protein tyrosine phosphatase SHP-1 in cancer. Gene 306, 1–12. doi:10.1016/s0378-1119(03)00400-1
Wu, Y., and Xu, Y. (2020). Integrated bioinformatics analysis of expression and gene regulation network of COL12A1 in colorectal cancer. Cancer Med. 9 (13), 4743–4755. doi:10.1002/cam4.2899
Xia, C. Q., Han, K., Qi, Y., Zhang, Y., and Yu, D. J. (2018). A self-training subspace clustering Algorithm under low-rank representation for cancer classification on gene expression data. IEEE/ACM Trans. Comput. Biol. Bioinform. 15 (4), 1315–1324. doi:10.1109/TCBB.2017.2712607
Xia, T., Yi, X. M., Wu, X., Shang, J., and Shu, H. B. (2019). PTPN1/2-mediated dephosphorylation of MITA/STING promotes its 20S proteasomal degradation and attenuates innate antiviral response. Proc. Natl. Acad. Sci. U. S. A. 116 (40), 20063–20069. doi:10.1073/pnas.1906431116
Yu, Z. H., and Zhang, Z. Y. (2018). Regulatory mechanisms and novel therapeutic targeting strategies for protein tyrosine phosphatases. Chem. Rev. 118 (3), 1069–1091. doi:10.1021/acs.chemrev.7b00105
Zhang, S., Fan, G., Hao, Y., Hammell, M., Wilkinson, J. E., and Tonks, N. K. (2017). Suppression of protein tyrosine phosphatase N23 predisposes to breast tumorigenesis via activation of FYN kinase. Genes Dev. 31 (19), 1939–1957. doi:10.1101/gad.304261.117
Keywords: PTPNs, AML, bioinformatics, prognosis, biomarker, expression level
Citation: Liu Y, Zhang J, Du Z, Huang J, Cheng Y, Yi W, Li T, Yang J and Chen C (2023) Comprehensive analysis of PTPN family expression and prognosis in acute myeloid leukemia. Front. Genet. 13:1087938. doi: 10.3389/fgene.2022.1087938
Received: 02 November 2022; Accepted: 23 December 2022;
Published: 09 January 2023.
Edited by:
Jiao Hu, Central South University, ChinaReviewed by:
Anna M. Eiring, Texas Tech University Health Sciences Center El Paso, United StatesJingfeng Zhou, Shenzhen University, China
Copyright © 2023 Liu, Zhang, Du, Huang, Cheng, Yi, Li, Yang and Chen. This is an open-access article distributed under the terms of the Creative Commons Attribution License (CC BY). The use, distribution or reproduction in other forums is permitted, provided the original author(s) and the copyright owner(s) are credited and that the original publication in this journal is cited, in accordance with accepted academic practice. No use, distribution or reproduction is permitted which does not comply with these terms.
*Correspondence: Chun Chen, Y2hlbmNodW5AbWFpbC5zeXN1LmVkdS5jbg==