- Breast Plastic and Reconstructive Surgery Center, Plastic Surgery Hospital, Chinese Academy of Medical Sciences, Peking Union Medical College, Beijing, China
Regeneration and tumorigenesis are indicated as related processes, while regeneration leads to life and the outcome of tumorigenesis is death. Here, we show the upregulation of zfp281 (zinc finger 281) in our adipose de novo regeneration model through RNA-seq analysis. Then, we validated the upregulation of zfp281 in adipose regeneration via immunofluorescence. Following that, we found that ZNF281 (the human homolog of Zfp281) was upregulated in most types of cancer and related to worse prognosis in 10 tumors. We further investigated the role of ZNF281 in cervical squamous cell carcinoma and endocervical adenocarcinoma (CESC), pancreatic adenocarcinoma (PAAD), and stomach adenocarcinoma (STAD) and confirmed the high accuracy in the clinical diagnostic feature. Beyond that, based on these three types of cancers, we analyzed the ZNF281-related tumor immune infiltration and DNA methylation sites and finally built risk prediction models for future disease diagnosis. Taken together, our findings provide new insights into the dual role of ZNF281, and we found that it was a potential biomarker for regeneration and tumor prognosis.
1 Introduction
Regeneration is characterized by the process of restoring homeostasis when organs sense the signals of damage, and this renewal process is orchestrated by a complex network of gene regulation and cellular processes (Chargé and Rudnicki, 2004; Gurtner et al., 2008; Gray et al., 2018). Like wound healing, regeneration proceeds through several overlying statuses, including inflammation (Eming et al., 2017), tissue reconstruction (Ghuman et al., 2016), and remodeling (Kim et al., 2018). All along the regeneration process, specific signals induce cellular proliferation in a finite number of cells, and finally, termination signals are released to avoid dysregulated proliferation, which causes tumorigenesis. Interestingly, increasing evidence indicates that regeneration and tumorigenesis are regulated by the same molecular pathways, and they may be recognized as related processes (Beachy et al., 2004; Schäfer and Werner, 2008; Cernaro et al., 2012; Jung et al., 2021). Thus, it is intriguing and significant to understand the link between regeneration and tumorigenesis.
ZNF281 (zinc finger protein 281) plays a role in the regulation of embryonic stem cell (ESC) differentiation and is very important in maintaining cellular stemness (An integrated encyclopedia of DNA, 2012; Pieraccioli et al., 2018). Knock-out of ZNF281 induces multipotent stem cell differentiation to osteogenic lineage (Seo et al., 2013). Moreover, epithelial-to-mesenchymal transition (EMT) is activated by ZNF281 in colon cancer cells through the regulation of SNAI1 and other EMT-related gene expressions (Hahn et al., 2013). We identified transcription factor zfp281, the human homolog of which is ZNF281, upregulated in a mice adipose regeneration model through RNA-seq. However, the role of ZNF281 in the regeneration program and tumorigenesis is largely unveiled.
In this study, we present findings that implicate ZNF281 as a promising biomarker in regeneration and multiple cancers. We applied basic fibroblast growth factor (bFGF) via controlled release of decellularized cells to induce adipocyte regeneration in C57BL/6N mice, and this model has been proven efficient in inducing adipogenesis (Zhang et al., 2016). In addition to mRNA upregulation of zfp281, histological analysis also validated the higher expression of zfp281 in the regeneration group. Furthermore, for a more complex and systematic understanding of ZNF281 in tumors, the expression level of ZNF281 was also investigated in pan-cancer and an upregulated phenomenon in most tumors compared to their related normal tissues and adjacent tissues was observed. Next, ZNF281 expression was found in 10 types of human cancer related to worse prognosis considering overall survival (OS); however, in kidney renal papillary cell carcinoma (KIRP), higher ZNF281 expression was related to a better prognosis, and this may due to the complexity and heterogeneity in cancer. Then, in three types of cancer, cervical squamous cell carcinoma and endocervical adenocarcinoma (CESC), pancreatic adenocarcinoma (PAAD), and stomach adenocarcinoma (STAD), ZNF281 was confirmed as a good prognostic molecular marker considering the high accuracy in the clinical diagnostic feature. After that, we applied single-sample Gene Set Enrichment Analysis (ssGSEA) and found a positive relation between ZNF281 and T-cell activation in these three tumors. Then, we screened DNA methylation sites. The least absolute shrinkage and selection operator (LASS)) builds on linear regression by increasing the penalty term (lambda × absolute slope value) to reduce the overfitting of the model and improve the generalization ability of the model (Zhang et al., 2020). Statistical analysis using the LASSO technique allowed the prediction of cancer with high sensitivity and specificity (Zhang et al., 2017). Finally, we established risk prediction models based on ZNF281-related lncRNAs for future disease diagnosis using the LASSO technique. In conclusion, our data focused on the dual role of ZNF281 and showed that it was a potential biomarker for regeneration and might also be functional in the diagnosis and clinical prediction of multiple types of cancer.
2 Materials and methods
2.1 Preparation of decellularized adipose-derived matrix loaded with bFGF
The procedure of decellularizing adipose tissues was accomplished following our previous protocols (Tang et al., 2022). All protocols reported in this study complied with ethical regulations for work with human subjects, and the study protocol was approved by the Plastic Surgery Hospital Ethics Committee (No. ZX 201843). Briefly, human lipoaspirate from healthy women under liposuction was obtained at the Chinese Academy of Medical Sciences & Peking Union Medical College Plastic Surgery Hospital. The mixture was allowed to stand for 10–15 min; then, the upper oil and lower bloody fluids were removed. After several cycles of washing in distilled water, three freeze–thaw cycles (−80°C–37°C, 2 h each) were performed. Then, the samples were homogenized using the A11 Basic Analytical Mill (IKA, Germany) three times (28,000 rpm, 1 min each). Followed by centrifugation and removal of oil, the milky suspension was agitated in hypotonic (0.5 M NaCl, 4 h) and hypertonic solutions (1 M NaCl, 4 h). After overnight washing in distilled water, samples were soaked in 1% Triton X-100 solution, and the solution was changed three times a day for 48 h. The white floc-like precipitate was washed again in distilled water (30 min, three times), and then, 99.9% isopropanol was used for further lipid removal. After the final three repeated cycles of washing with distilled water (30 min each) and three times of agitation in 70% ethanol (30 min each) for disinfection, DAM was placed in phosphate-buffered saline (PBS) (HyClone) containing 1% penicillin–streptomycin (HyClone) at 4°C for storage.
In this study, we applied heparin cross-linking of DAM for the loading of bFGF following the previously described methods (Wissink et al., 2001; Zhang et al., 2016), the sustained releasing bFGF of which was <70% of the loaded bFGF over a period of 10 days. For loading bFGF, 250 mg wet weight DAM was mixed in 500 μL normal saline containing 2 μg/ml bFGF, and DAM was scissor-minced thoroughly. The bFGF dose has been reported as the optimal concentration for inducing adipose in situ regeneration (Hiraoka et al., 2006).
2.2 In vivo experiments
All animal model studies were approved in advance by the Animal Ethics Committee at the Chinese Academy of Medical Sciences & Peking Union Medical College and performed following the Animal Ethics Committee’s guidelines. Female eight-week-old C57BL/6 N mice were used for injecting the bFGF-loaded DAM mixture. As a control group, heparinized DAM mixed with PBS was used. The volume of suspension was 200 μL per site of injection (n ≥ 5 for each group). The animals were sacrificed at 1, 2, 3, and 12 weeks, and the samples were obtained for RNA-seq and histological analysis.
2.3 mRNA-Seq assay
Total RNA extracts were acquired from implanted mouse tissues of bFGF-loaded DAM and DAM at 1 week using the MagBeads Total RNA Extraction Kit (Cat#T02-096) according to the manufacturer’s instructions, and RNA integrity was checked with the RNA integrity number (RIN) by using a bioanalyzer (Agilent Technologies, US). RNA purification was performed by using the RNAClean XP kit (Cat A63987, USA) and RNase-Free DNase Set (Cat#79254, QIAGEN, Germany). After the quality control of RNA, the sequencing libraries were constructed. After library inspection using the Qubit fluorometer and Agilent 4200, the libraries were sequenced with the Illumina NovaSeq 6,000 sequencing platform, and the PE150 mode was selected. All the data were analyzed in R 3.6.4, and the R package DESeq2 was used for differential expression significance analysis. For filtrating differential expressed genes, the absolute value of log2(fold change) was selected above 2, and the p value was less than 0.05.
2.4 Histological analysis and immunofluorescence staining
After 24–48 h fixation (4% formalin), the samples retrieved from the animals were dehydrated and embedded in paraffin (Leica) for routine sectioning. The sections underwent deparaffinization and rehydration, and then, antigen retrieval was processed in a microwave in citric acid (Solarbio). After washing with PBS, slides were blocked with 5% goat serum for half an hour at 37°C, and overnight incubation with the primary antibody at 4°C was performed. Secondary immunofluorescent-tagged antibodies were used to incubate slides for 1 hour at 37°C for signal amplification. The following antibodies were used for immunofluorescence: mouse monoclonal IgG2a κ ZNF281 antibody (1:100, Cat. No. sc-166933, Santa Cruz), rabbit polyclonal perilipin-1 antibody (1:100, Cat. No. ab3526, Abcam), CoraLite 488-conjugated goat anti-rabbit IgG (H + L) (1:100, Cat. No. SA00013-2, Proteintech), and CoraLite 594-conjugated goat anti-mouse IgG (H + L) (1:100, Cat.No. SA00013-3, Proteintech). The nuclei were stained with DAPI (Invitrogen). Single-channel and merge images were generated in Photoshop.
2.5 Analysis of ZNF281 expression in normal tissues and pan-cancer
The mRNA expression level of ZNF281 in normal tissues was analyzed in the Human Protein Atlas (HPA) online platform (https://www.proteinatlas.org/) (Sjöstedt et al., 2020; Karlsson et al., 2021). Based on the HPA RNA-seq data and scRNA-seq data, we displayed the tissue/cell distribution of ZNF281, especially in normal adipose tissues. The data of RNA-seq and related clinical information were acquired from The Cancer Genome Atlas (TCGA) and the Genotype-Tissue Expression (GTEx) database using USCS Xena (http://xena.ucsc.edu/) on 16 August 2022 (Vivian et al., 2017). The data of cancer involves adrenal cortical carcinoma (ACC), bladder urothelial carcinoma (BLCA), breast-invasive carcinoma (BRCA), CESC, cholangiocarcinoma (CHOL), colon adenocarcinoma (COAD), lymphoid neoplasm diffuse large B-cell lymphoma (DLBC), esophageal carcinoma (ESCA), glioblastoma multiforme (GBM), head and neck squamous cell carcinoma (HNSC), kidney chromophobe (KICH), kidney renal clear cell carcinoma (KIRC), KIRP, acute myeloid leukemia (LAML), brain lower grade glioma (LGG), liver hepatocellular carcinoma (LIHC), lung adenocarcinoma (LUAD), lung squamous cell carcinoma (LUSC), ovarian serous cystadenocarcinoma (OV), PAAD, pheochromocytoma and paraganglioma (PCPG), prostate adenocarcinoma (PRAD), rectum adenocarcinoma (READ), skin cutaneous melanoma (SKCM), STAD, testicular germ cell tumors (TGCT), thyroid carcinoma (THCA), thymoma (THYM), uterine corpus endometrial carcinoma (UCEC), and uterine carcinosarcoma (UCS). All the data were analyzed in R 3.6.4, and the visualization was completed with the R package ggplot2. The statistical method used was the Mann–Whitney U test, and when p < 0.05, the difference was considered to reach statistical significance.
2.6 Survival analysis
The survival curve (also known as the Kaplan–Meier curve) can describe the survival of each group of patients. Based on the median expression of ZNF281 or model risk scores, patients could be subdivided into the high-expression group and the low-expression group. The survminer R package was used for visualization, and the survival R package was used for the statistical analysis of survival data. OS was set as the survival outcome.
2.7 Receiver operating characteristic curve
The receiver operating characteristic (ROC) curve is a comprehensive index reflecting the sensitivity and specificity of continuous variables, and the composition method reflects the correlation between sensitivity and specificity. When the expression of a molecule is a trend to promote the occurrence of events, the area under the curve (AUC) of this molecule will be >0.5, and the closer the AUC approaches 1, the better the prediction performance. When the expression of a molecule is contrary to the trend of event occurrence, the molecule will be <0.5, and the closer the AUC is to 0, the more accurate the prediction performs. In brief, the point closest to the top left of the curve is the critical value with the highest sensitivity and specificity on the ROC curve. The larger the area under the curve, the higher the diagnostic accuracy. The R package pROC was used in the ROC analysis, and the ggplot2 R package was applied to visualize results (Robin et al., 2011). The expression level of ZNF281 was used as the input in ROC analysis in 30 types of cancer. To proceed with further analysis, we selected tumors for which ZNF281 might be recognized as a risk factor based on differential analysis, OS, ROC, and AUC; then, CESC, PAAD, and STAD were selected.
2.8 Single-sample Gene Set Enrichment Analysis
As an extension of Gene Set Enrichment Analysis (GSEA), single-sample Gene Set Enrichment Analysis calculates separate enrichment scores for each pairing of a sample and gene set. Gene markers for 24 immune cells were obtained from an article, and the classification and description of specific cells are shown in Bindea et al. (2013). Then, the procedures of ssGSEA were performed by the R package GSVA (version: 1.34.0) (Hänzelmann et al., 2013). Then, the relationship between the ssGSEA scores of 24 immune cells and ZNF281 expression in CESC, PAAD, and STAD was calculated by Spearman correlation analysis.
2.9 ZNF281-associated DNA methylation sites
The correlation between the beta value of methylation sites within 5000bp upstream and downstream of the transcription start site (TSS) and the expression level of ZNF281 was calculated. Spearman correlation analysis was included. The stat R package was used. DNA methylation sites with p value less than 0.05 and Spearman correlation coefficient greater than 0.3 were selected.
2.10 Screening ZNF281-related genes
In CESC, PAAD, and STAD, we investigated the correlation between other genes and ZNF281 expression via Spearman and Pearson correlation analyses. To find genes with statistical significance, P (Spearman) and p (Pearson) less than 0.05 were selected as the basic screening rule. Genes with correlation coefficient (Cor) more than 0.55 or less than −0.55 for both Spearman and Pearson analyses in CESC and STAD were screened. For PAAD, the absolute value of Cor (Spearman) was filtered over 0.7 and the absolute value of Cor (Pearson) was filtered over 0.6. This procedure was achieved via the stat R package.
2.11 Least absolute shrinkage and selection operator
The expression of ZNF281-related genes was selected as the input in LASSO. In the process of ten-fold cross validation, the seed number was set as 2021. The screening threshold of the model coefficients was selected as lambda. min. According to the selected genes by LASSO, we built the risk models in CESC, PAAD, and STAD. Also, the LASSO coefficients were used to calculate the risk scores with the expression of genes in models. The glmnet and survival R packages were used.
2.12 Time-dependent receiver operating characteristic curve
Time-dependent receiver operating characteristic (tdROC) curve analysis was mainly used to analyze the predictive efficacy of one continuous variable in predicting outcomes related to time. The tdROC was used to complete the analysis, and ggplot2 was used to visualize the results. Also, the tdROC results of the expression of ZNF281 at 1, 3, and 5 years were calculated.
3 Results
3.1 New adipose formation and concomitant zfp281 expression upregulated at an early stage
The differential gene expression of RNA-seq is displayed in Supplementary Figure S1A, and a significant fold difference in zfp281 is shown between the two groups. Compared to the control group (Figures 1D–F), samples derived from bFGF-loaded DAM (Figures 1A–C) showed a significant adipose regeneration phenomenon. Moreover, zfp281 expression in the early stage (1 week) was validated higher in the bFGF-loaded DAM group (Figures 1G–L).
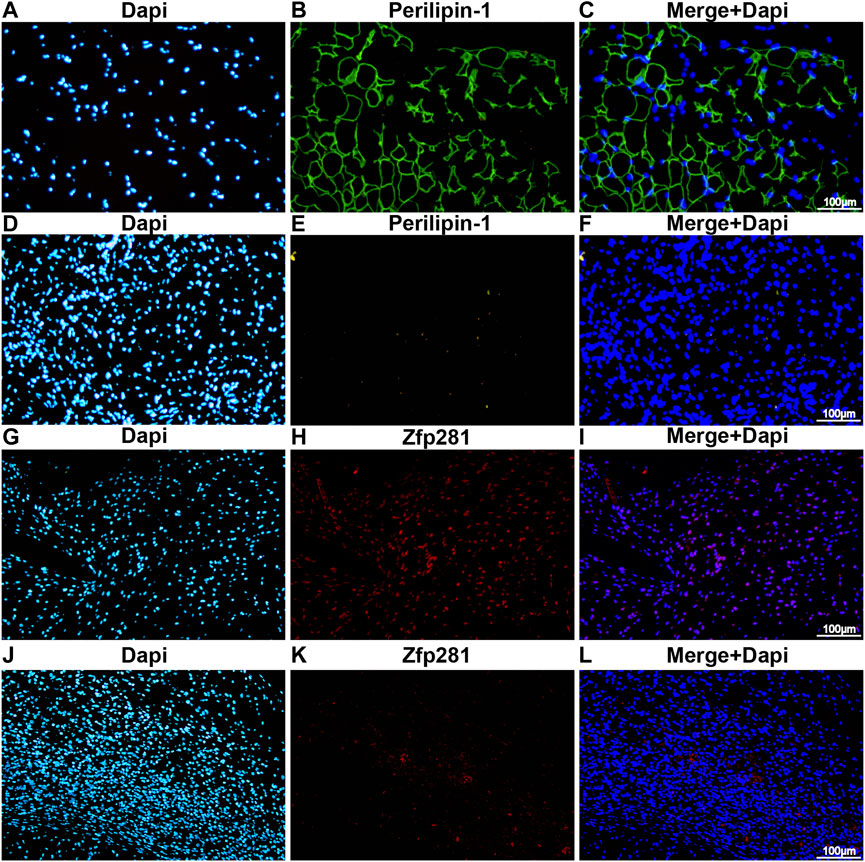
FIGURE 1. Zfp281 is upregulated at an early stage in adipose de novo regeneration tissues. (A–F) Immunofluorescence staining of perilipin-1 in bFGF-loaded DAM (A–C) and DAM-injected mouse tissuees (D–F) at 12 weeks; (G–L) immunofluorescence staining of zfp281 in bFGF-loaded DAM (G–I) and DAM-injected mouse tissues (J–L) at 1 week. Perilippin-1 is labeled in green. Zfp281 is Labeled in red. Nuclei are labeled with DAPI in blue.
3.2 Gene expression in normal tissues and pan-cancer
As shown in Figures 2A, B, we found ZNF281 was highly expressed in the bone marrow, followed by the liver and white matter. Moreover, the cell type that expressed the highest level of ZNF281 is hepatocytes. In adipose tissues (Supplementary Figure S2B), a cluster of macrophages express the highest amount of ZNF281 mRNA. We compared ZNF281 expression levels across 30 types of tumors and relevant normal tissues. In most types of cancers, ZNF281 was expressed significantly higher in tumors (Figures 2C, D). However, ZNF281 was less expressed in three types of tumors compared to corresponding normal tissues, including ACC, KICH, and THCA. By integrating data from the GTEx database, we compared the ZNF281 expression levels in cancer and their normal adjacent tissues, and ZNF281 was significantly expressed higher in 11 cancer types, including BLCA, BRCA, CHOL, COAD, ESCA, HNSC, KIRC, LIHC, LUAD, PRAD, and STAD. Meanwhile, in KICH and THCA, ZNF281 was downregulated in cancer.
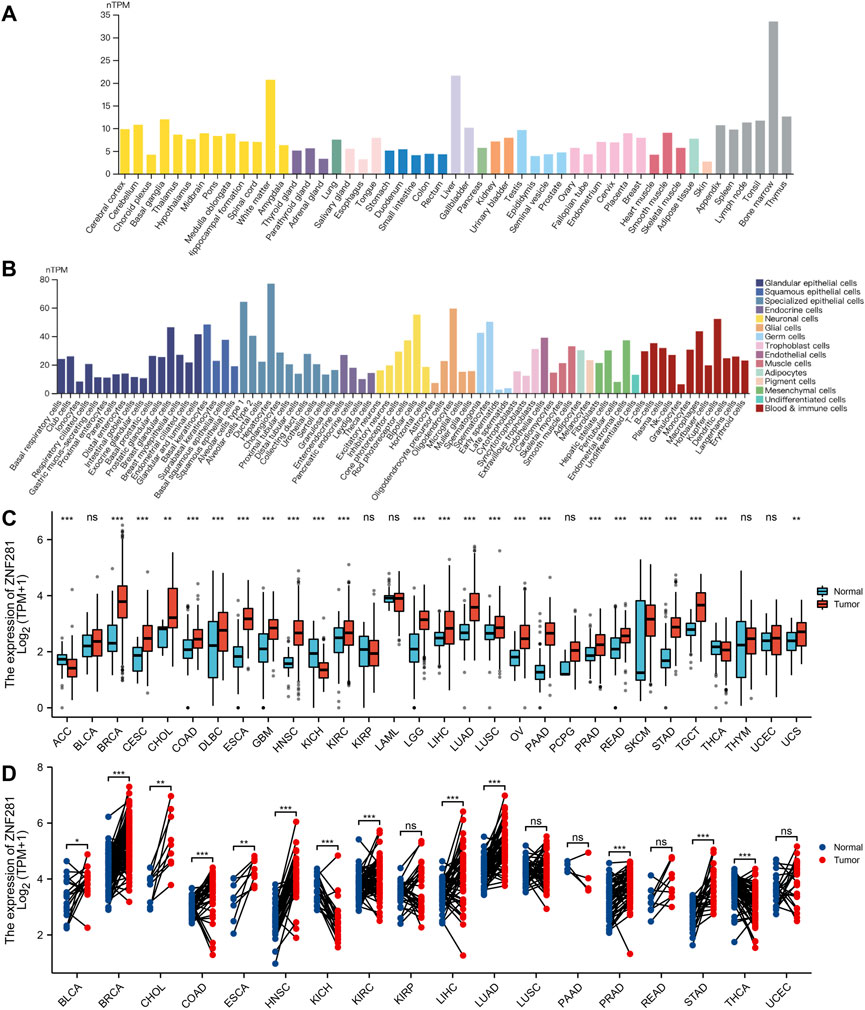
FIGURE 2. Expression levels of ZNF281 were higher in tumors and normal tissues. (A) ZNF281 expression in normal tissues, (B) ZNF281 expression in single-cell types, (C) ZNF281 expression was upregulated in most types of cancers than normal tissues, combining the data of TCGA and the GTEx database, and (D) ZNF281 expression in TCGA tumors and adjacent normal tissues (*p < 0.05, **p < 0.01, and ***p < 0.001).
3.3 Higher expression of ZNF281 in a variety of tumors suggests a poorer prognosis
According to the results of survival analyses (Figure 3), patients with ZNF281 expression above the median value had worse OS in ACC (p = 0.01, HR = 3.01), CESC (p < 0.001, HR = 2.45), KIRP (p = 0.001, HR = 2.66), LUSC (p = 0.021, HR = 1.38), MESO (p = 0.003, HR = 2.17), PAAD (p = 0.034, HR = 1.76), SKCM (p = 0.01, HR = 1.43), STAD (p = 0.008, HR = 1.58), THCA (p = 0.037, HR = 2.86), and UCEC (p = 0.013, HR = 1.94). However, patients with lower ZNF281 expression levels based on the median value showed the worst prognosis in KIRC (p = 0.03, HR = 0.72). However, in the other types of cancer, ZNF281 was not related to prognosis, and the results are shown in Supplementary Figure S2.
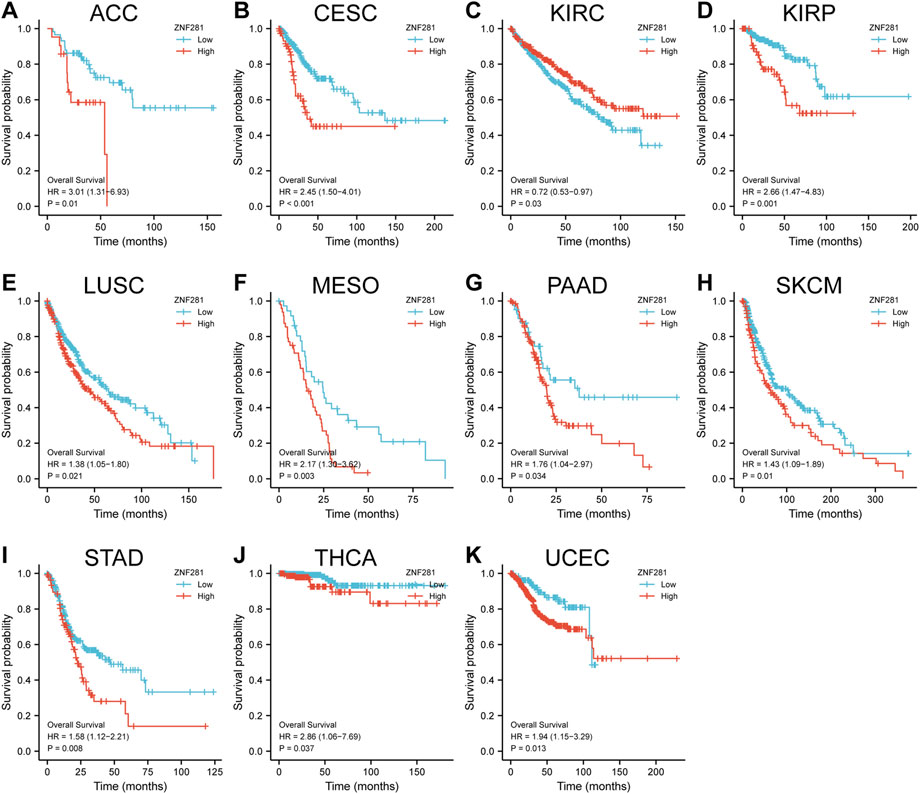
FIGURE 3. Correlations between the ZNF281 expression and prognosis (OS) of patients in different cancers. (A) ACC, (B) CESC, (C) KIRC, (D) KIRP, (E) LUSC, (F) MESO, (G) PAAD, (H) SKCM, (I) STAD, (J) THCA, and (K) UCEC.
3.4 ZNF281 has good diagnostic efficacy in CESC, STAD, and PAAD
According to ROC analysis, ZNF281 showed a good diagnostic ability in differentiating tumors from benign tissues in CESC (Figure 4A, AUC = 0.794), STAD (Figure 4B, AUC = 0.946), and PAAD (Figure 4C, AUC = 0.917). The ROC analysis in the other types of tumors is shown in Supplementary Figure S3.
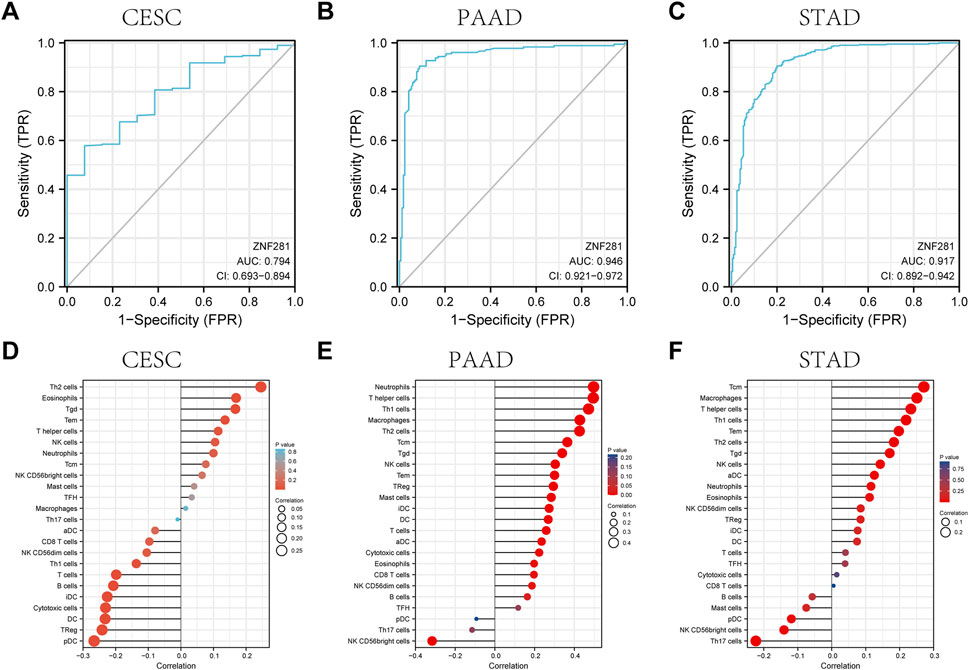
FIGURE 4. ROC curve and the relationship with immune cell infiltration for ZNF281 in CESC, PAAD, and STAD. (A) ROC curve for ZNF281 in CESC, (B) ROC curve for ZNF281 in PAAD, and (C) ROC curve for ZNF281 in STAD. (D) Immune infiltration analysis for ZNF281 in CESC, (E) immune infiltration analysis for ZNF281 in PAAD, and (F) immune infiltration analysis for ZNF281 in STAD.
3.5 ZNF281 is mainly related to the activation of the T-cell family
As shown in Figure 4D, ZNF281 was positively correlated to the levels of Th2 cells, eosinophils, T gamma delta cells, T effector memory cells, and T helper cells in CESC. Also, according to Figure 4E, more expressions of ZNF281 indicated more levels of neutrophils, T helper cells, Th1 cells, macrophages, and Th2 cells in PAAD. In STAD (Figure 4F), the levels of ZNF281 were associated with the content of T central memory cells, macrophages, T helper cells, Th1 cells, and T effector memory cells.
3.6 Two DNA methylation sites in the TSS region of ZNF281 may regulate its transcription activity in PAAD
Methylation is mainly carried out through DNA methyltransferase to add methyl groups to DNA and to affect the DNA transcription process without changing the DNA sequence. Based on the screening conditions of p < 0.05 and Spearman correlation coefficient >0.3, two DNA methylation sites (cg03559467: correlation coefficient = −0.303, p < 0.001 and cg25841477: correlation coefficient = −0.334, p < 0.001) might be related to the transcription of ZNF281 in PAAD (Figures 5E, G). However, the transcriptional regulation of ZNF281 did not seem to be related to DNA methylation in STAD and CESC (Figure 5).
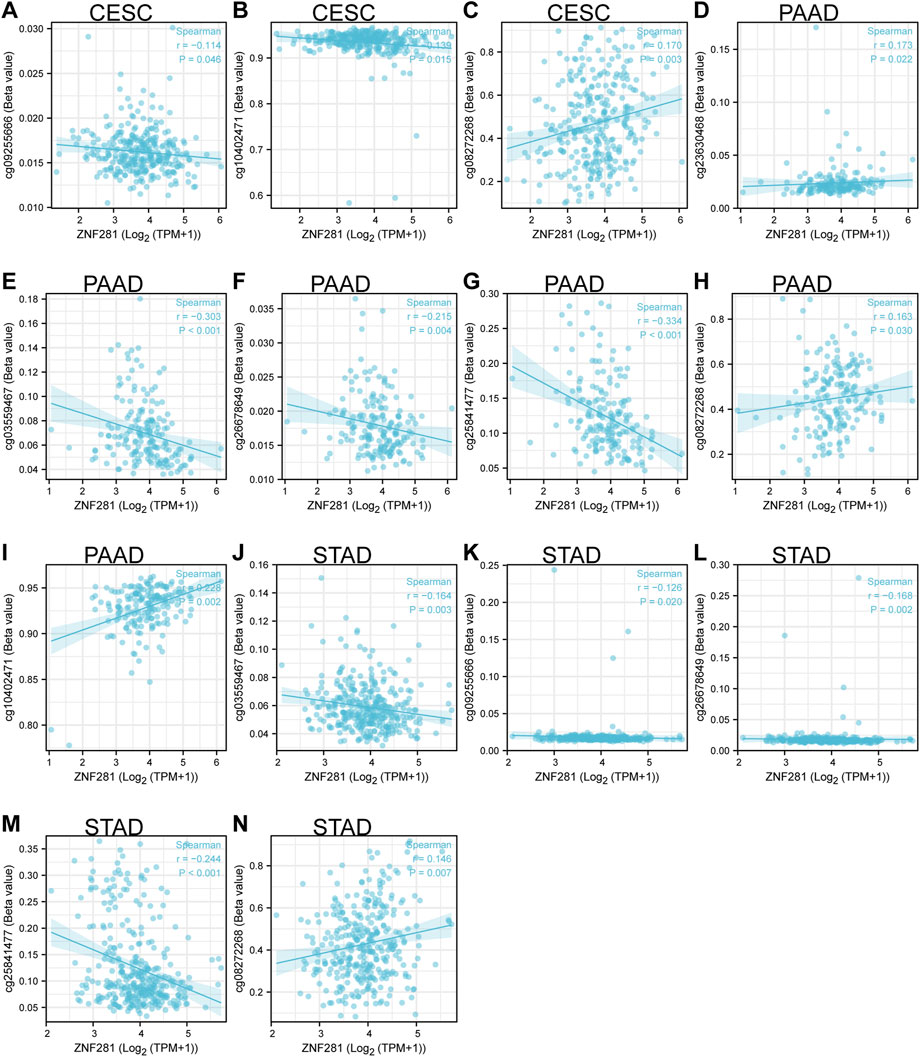
FIGURE 5. ZNF281-associated DNA methylation sites in CESC, PAAD, and STAD. (A–C) Methylation sites in the TSS region of ZNF281 in CESC, (D–I) methylation sites in the TSS region of ZNF281 in CESC, and (J–N) methylation sites in the TSS region of ZNF281 in CESC.
3.7 Three lncRNA prognostic models based on ZNF281-correlated genes were constructed in CESC, PAAD, and STAD
We screened 129 lncRNA coding genes in CESC (Supplementary Table S1), 63 lncRNA coding genes in PAAD (Supplementary Table S2), and 41 lncRNA coding genes in STAD (Supplementary Table S3) associated with the ZNF281 level. The expression of these selected lncRNA coding genes was used as the input to train the prognostic models in CESC, PAAD, and STAD. As shown in Figures 6A–C, each point in the figure represented the mean value of likelihood deviation corresponding to each lambda in the process of cross validation, and the error line represents the corresponding error situation. In general, a smaller likelihood deviation value corresponds to a better model, which corresponds to the lambda. min value. According to the lambda. min value, genes in the final models were selected and their coefficients are shown in Figures 6D–F. The risk score can be calculated as follows: CESC: risk score = BOLA3-AS1 × 0.048 + AC139887.2 × 0.024 + NADK2-AS1 × 0.081 + NKILA × 0.130 + LINC01719 × 0.417 + AC022784.5 × 0.068 + AP001094.2 × 0.103 + AL365436.2 × 0.083; PAAD: risk score = AC099850.3 × 0.139 + AP003119.3 × 0.133 + AC112721.2 × 0.129 + AC073046.1 × 0.032—HCG18 × 0.073; and STAD: risk score = ERICD × 0.573 + AC105036.3 × 0.272 + ZNF8-ERVK3-1 × 0.226 + NKILA × 0.166 + PTOV1-AS1×0.132 + AC005332.6 × 0.124 + AP000759.1 × 0.114 + STARD4-AS1 × 0.070 + LINC00205 × 0.019—AC092171.2 × 0.013—AC107068.1 × 0.276—AL355574 × 0.288—LINC00630 × 0.367. The higher risk score is related to a worse prognosis. As shown in Figures 6G–I, patients with higher risk scores presented worse OS in CESC (p < 0.001, HR = 2.79), PAAD (p < 0.001, HR = 2.23), and STAD (p < 0.001, HR = 2.19). Furthermore, these three lncRNA prognostic models for CESC, PAAD, and STAD exhibited good predictive ability for PFI at 1, 3, and 5 years (Figures 6J–L).
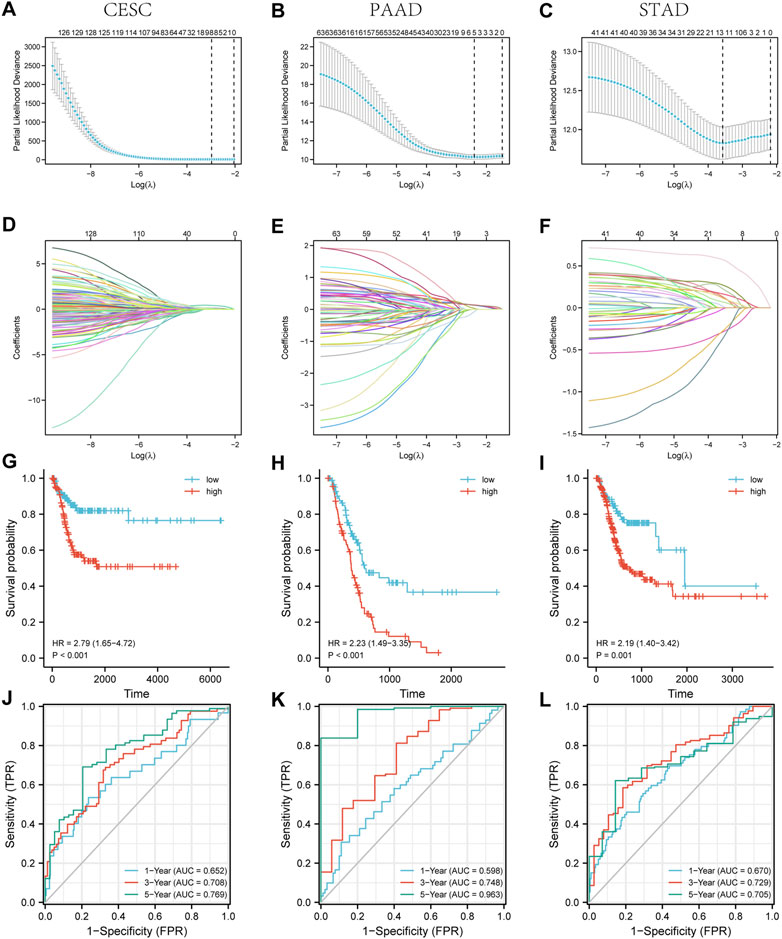
FIGURE 6. Three lncRNA prognostic models associated with ZNF281 showed good accuracy in predicting the prognosis of patients in CESC, PAAD, and STAD. (A–C) Cross validation based on C-index to determine the best choice of genes in the prediction models. (D–F) Genes in the different choices of models and their corresponding coefficients based on different lambda values. (G–I) According to prediction models, the relationship between the survival outcome and risk levels of patients. (J–L) The tdROC curve of 1, 3, and 5 years verified the efficacy of prediction models. (A,D,G,J) CESC, (B,E,H,K) PAAD, and (C,F,I,L) STAD.
4 Discussion
We hypothesized that ZNF281 (zfp281 in Mus musculus) would be a potential biomarker in some forms of regeneration and tumorigenesis, which share similar molecular pathways. We first detected the upregulation of zfp281 in de novo adipogenesis through RNA-seq and then validated the histological expression in the early stage of the adipose regeneration model. Through literature review, we found that ZNF281 was related to the tumor development and progression mechanism, such as EMT (Hahn et al., 2013; Pierdomenico et al., 2018; Sadłecki et al., 2019; Xue et al., 2019). Considering the heterogeneity and complexity of tumors, we analyzed the ZNF281 expression in pan-cancer and related normal tissues using RNA-seq data from TCGA and GTEx and found the upregulation of ZNF281 in most types of cancer. Moreover, the ZNF281 expression level was related to worse prognosis in 10 types of cancer. However, in KIRC, higher expression of ZNF281 was expected to have survival benefits given the OS analysis. Further investigation indicated ZNF281 had good prognostic value in CESC, PAAD, and STAD. Also, certain types of T-cell activation were positively correlated to the ZNF281 expression. In addition, we explored two DNA methylation sites in the TSS region of ZNF281 that may regulate its transcription activity in PAAD and could be targeted when treating PAAD. Finally, we established clinical prognostic models based on ZNF281-related lncRNA in CESC, PAAD, and STAD.
ZNF281 has also shown its role in regeneration regulation. Zhou et al. (2017) found ZNF281 enhances cardiac reprogramming and upregulation of ZNF281 significantly activated genes related to myogenesis, muscle contraction, and heart processes. As a strong activator in cardiac reprogramming, ZNF281 promotes cardiac regeneration by working on GATA4, which represents an important cardiogenic transcription factor (Lalit et al., 2017; Stone et al., 2019), and works against inflammatory reactions, which inhibits cardiac reprogramming. Here, we hypothesized three possible explanations for elevated zfp281 at the early stages of adipose regeneration. First, that might be the result of environmental response to regeneration signals, which promotes cell proliferation and migration. A mesenchymal-like program as negative feedback is exerted by the environment to keep homeostasis, and then, the expression of zfp281 might be upregulated (Kciuk et al., 2022; Nobre et al., 2022). Second, ZNF281 might promote regeneration by activating EMT-related pathways (Hahn et al., 2013). EMT is divided into three different subtypes: type-1 EMT, type-2 EMT, and type-3 EMT. Type-2 EMT is correlated to the wound healing process, tissue regeneration, and organ fibrosis (Oh et al., 2018; Aharonov et al., 2020; Klatt Shaw et al., 2021; Marconi et al., 2021). Activation of EMT has been linked to the acquisition of both normal and neoplastic stem cells, which might lead to regeneration and carcinogenesis, respectively (Lambert and Weinberg, 2021). Finally, ZNF281 has been recognized as a direct participant in DNA damage response and repair (DRR), and the upregulation of DDR-related molecules reduces tumor immunogenicity and then causes neoplastic cells to escape from the immune system (Nicolai et al., 2020a). A similar mechanism may also exist in regenerating cells.
ZNF281 inhibits the differentiation of neurons, and higher expression was proven related to a worse prognosis in neuroblastoma (Pieraccioli et al., 2018). Moreover, ZNF281 is mainly expressed in poorly differentiated cells and tissues, and accompanying differentiating process, the expression level of ZNF281 was downregulated. Nicolai et al. (2020b) proposed that ZNF281 directly inhibits muscle differentiation promoted by microRNA-1. Furthermore, they validated the higher expression of ZNF281 in certain types of soft-tissue sarcoma, such as rhabdomyosarcoma and leiomyosarcoma tumors. In our research, we found that ZNF281 was expressed higher in most tumors than in the corresponding normal tissues. Moreover, reduced survival expectations were observed in high ZNF281 cancer. These findings can also be explained by EMT and DDR mechanisms, which are related to metastasis and immune escape of tumors. Beyond that, we validated the good prognostic value of ZNF281 in CESC, STAD, and PAAD and finally established risk prediction models based on ZNF281-related molecules for future clinical diagnosis and treatment.
Sharing common molecular pathways, more and more evidence indicates that regeneration and carcinogenesis are related processes, although accompanying diverse outcomes are life and death (Ewerbeck et al., 1985; Sarig and Tzahor, 2017). In this context, the activity of ZNF281 might be recognized as a potential biomarker for regeneration and cancer. However, there are still some limitations. First, the risk model does not apply an external database to verify its effectiveness. Second, extensive further work is required to understand the mechanism that how ZNF281 is regulated in the specific regeneration-promoted environment and how ZNF281, in turn, regulates regeneration. Further interactions between regeneration and tumorigenesis should also be uncovered in future scientific research. Moreover, in this study, we utilized shallow machine learning to build the risk model other than deep learning, which is more flexible.
Data availability statement
The datasets presented in this study can be found in online repositories. The names of the repository/repositories and accession number(s) can be found in the article/Supplementary Material.
Ethics statement
The studies involving human participants were reviewed and approved by the Plastic Surgery Hospital Ethics Committee. The patients/participants provided their written informed consent to participate in this study. The animal study was reviewed and approved by the Plastic Surgery Hospital Ethics Committee.
Author contributions
JL and SF conceptualized and designed the study. XH performed the experimental studies and analyzed the data in consultation with SF. XH wrote the manuscript with editorial feedback provided by JL and SF.
Funding
This work was supported by the CAMS Innovation Fund for Medical Sciences (2021-2M-1-052) and the Key projects of medical school development of Shijingshan district (20078).
Conflict of interest
The authors declare that the research was conducted in the absence of any commercial or financial relationships that could be construed as a potential conflict of interest.
Publisher’s note
All claims expressed in this article are solely those of the authors and do not necessarily represent those of their affiliated organizations, or those of the publisher, the editors, and the reviewers. Any product that may be evaluated in this article, or claim that may be made by its manufacturer, is not guaranteed or endorsed by the publisher.
Supplementary material
The Supplementary Material for this article can be found online at: https://www.frontiersin.org/articles/10.3389/fgene.2022.1082654/full#supplementary-material
References
Aharonov, A., Shakked, A., Umansky, K. B., Savidor, A., Genzelinakh, A., Kain, D., et al. (2020). ERBB2 drives YAP activation and EMT-like processes during cardiac regeneration. Nat. Cell Biol. 22 (11), 1346–1356. Epub 2020/10/14PubMed PMID: 33046882. doi:10.1038/s41556-020-00588-4
An integrated encyclopedia of DNA (2012). An integrated encyclopedia of DNA elements in the human genome. Nature 489 (7414), 57–74. doi:10.1038/nature11247
Beachy, P. A., Karhadkar, S. S., and Berman, D. M. (2004). Tissue repair and stem cell renewal in carcinogenesis. Nature 432 (7015), 324–331. Epub 2004/11/19PubMed PMID: 15549094. doi:10.1038/nature03100
Bindea, G., Mlecnik, B., Tosolini, M., Kirilovsky, A., Waldner, M., Obenauf, A. C., et al. (2013). Spatiotemporal dynamics of intratumoral immune cells reveal the immune landscape in human cancer. Immunity 39 (4), 782–795. Epub 2013/10/22PubMed PMID: 24138885. doi:10.1016/j.immuni.2013.10.003
Cernaro, V., Lacquaniti, A., Donato, V., Fazio, M. R., Buemi, A., and Buemi, M. (2012). Fibrosis, regeneration and cancer: What is the link? Nephrol. Dial. Transpl. 27 (1), 21–27. Epub 2011/11/22PubMed PMID: 22102616. doi:10.1093/ndt/gfr567
Chargé, S. B., and Rudnicki, M. A. (2004). Cellular and molecular regulation of muscle regeneration. Physiol. Rev. 84 (1), 209–238. Epub 2004/01/13PubMed PMID: 14715915. doi:10.1152/physrev.00019.2003
Eming, S. A., Wynn, T. A., and Martin, P. (2017). Inflammation and metabolism in tissue repair and regeneration. Science 356 (6342), 1026–1030. Epub 2017/06/10PubMed PMID: 28596335. doi:10.1126/science.aam7928
Ewerbeck, V., Bolkenius, M., Braun, A., and Brandeis, W. E. (1985). Bone tumors and tumor-like changes in the neonatal period and in infancy. Z Orthop. Ihre Grenzgeb 123 (6), 918–928. Epub 1985/11/01PubMed PMID: 3832678. doi:10.1055/s-2008-1044780
Ghuman, H., Massensini, A. R., Donnelly, J., Kim, S. M., Medberry, C. J., Badylak, S. F., et al. (2016). ECM hydrogel for the treatment of stroke: Characterization of the host cell infiltrate. Biomaterials 91, 166–181. Epub 2016/04/01PubMed PMID: 27031811; PubMed Central PMCID: PMCPMC4893791. doi:10.1016/j.biomaterials.2016.03.014
Gray, N., Le Bot, N., and Heemels, M. T. (2018). Regeneration. Nature 557 (7705), 321. Epub 2018/05/17PubMed PMID: 29765125. doi:10.1038/d41586-018-05155-4
Gurtner, G. C., Werner, S., Barrandon, Y., and Longaker, M. T. (2008). Wound repair and regeneration. Nature 453 (7193), 314–321. Epub 2008/05/16PubMed PMID: 18480812. doi:10.1038/nature07039
Hahn, S., Jackstadt, R., Siemens, H., Hünten, S., and Hermeking, H. (2013). SNAIL and miR-34a feed-forward regulation of ZNF281/ZBP99 promotes epithelial-mesenchymal transition. Embo J. 32 (23), 3079–3095. Epub 2013/11/05PubMed PMID: 24185900; PubMed Central PMCID: PMCPMC3844956. doi:10.1038/emboj.2013.236
Hänzelmann, S., Castelo, R., and Guinney, J. (2013). Gsva: Gene set variation analysis for microarray and RNA-seq data. BMC Bioinforma. 14 (1), 7. doi:10.1186/1471-2105-14-7
Hiraoka, Y., Yamashiro, H., Yasuda, K., Kimura, Y., Inamoto, T., and Tabata, Y. (2006). In situ regeneration of adipose tissue in rat fat pad by combining a collagen scaffold with gelatin microspheres containing basic fibroblast growth factor. Tissue Eng. 12 (6), 1475–1487. Epub 2006/07/19PubMed PMID: 16846345. doi:10.1089/ten.2006.12.1475
Jung, Y. S., Stratton, S. A., Lee, S. H., Kim, M. J., Jun, S., Zhang, J., et al. (2021). TMEM9-v-ATPase activates wnt/β-catenin signaling via APC lysosomal degradation for liver regeneration and tumorigenesis. Hepatology 73 (2), 776–794. Epub 2020/05/08PubMed PMID: 32380568; PubMed Central PMCID: PMCPMC7647947. doi:10.1002/hep.31305
Karlsson, M., Zhang, C., Méar, L., Zhong, W., Digre, A., Katona, B., et al. (2021). A single-cell type transcriptomics map of human tissues. Sci. Adv. 7 (31), eabh2169. Epub 2021/07/30PubMed PMID: 34321199; PubMed Central PMCID: PMCPMC8318366. doi:10.1126/sciadv.abh2169
Kciuk, M., Gielecińska, A., Kołat, D., Kałuzińska, Ż., and Kontek, R. (2022). Cancer-associated transcription factors in DNA damage response. Biochim. Biophys. Acta Rev. Cancer 1877 (4), 188757. Epub 2022/07/06PubMed PMID: 35781034. doi:10.1016/j.bbcan.2022.188757
Kim, G. H., Uriel, N., and Burkhoff, D. (2018). Reverse remodelling and myocardial recovery in heart failure. Nat. Rev. Cardiol. 15 (2), 83–96. Epub 2017/09/22PubMed PMID: 28933783. doi:10.1038/nrcardio.2017.139
Klatt Shaw, D., Saraswathy, V. M., Zhou, L., McAdow, A. R., Burris, B., Butka, E., et al. (2021). Localized EMT reprograms glial progenitors to promote spinal cord repair. Dev. Cell 56 (5), 613–626.e7. e7. Epub 2021/02/21PubMed PMID: 33609461; PubMed Central PMCID: PMCPMC8044706. doi:10.1016/j.devcel.2021.01.017
Lalit, P. A., Rodriguez, A. M., Downs, K. M., and Kamp, T. J. (2017). Generation of multipotent induced cardiac progenitor cells from mouse fibroblasts and potency testing in ex vivo mouse embryos. Nat. Protoc. 12 (5), 1029–1054. Epub 2017/04/21PubMed PMID: 28426026; PubMed Central PMCID: PMCPMC5693216. doi:10.1038/nprot.2017.021
Lambert, A. W., and Weinberg, R. A. (2021). Linking EMT programmes to normal and neoplastic epithelial stem cells. Nat. Rev. Cancer 21 (5), 325–338. Epub 2021/02/07PubMed PMID: 33547455. doi:10.1038/s41568-021-00332-6
Marconi, G. D., Fonticoli, L., Rajan, T. S., Pierdomenico, S. D., Trubiani, O., Pizzicannella, J., et al. (2021). Epithelial-mesenchymal transition (EMT): The type-2 EMT in wound healing, tissue regeneration and organ fibrosis. Cells 10 (7), 1587. Epub 2021/07/03PubMed PMID: 34201858; PubMed Central PMCID: PMCPMC8307661. doi:10.3390/cells10071587
Nicolai, S., Mahen, R., Raschellà, G., Marini, A., Pieraccioli, M., Malewicz, M., et al. (2020). ZNF281 is recruited on DNA breaks to facilitate DNA repair by non-homologous end joining. Oncogene 39 (4), 754–766. Epub 2019/10/02PubMed PMID: 31570788; PubMed Central PMCID: PMCPMC6976523. doi:10.1038/s41388-019-1028-7
Nicolai, S., Pieraccioli, M., Smirnov, A., Pitolli, C., Anemona, L., Mauriello, A., et al. (2020). ZNF281/Zfp281 is a target of miR-1 and counteracts muscle differentiation. Mol. Oncol. 14 (2), 294–308. Epub 2019/11/30PubMed PMID: 31782884; PubMed Central PMCID: PMCPMC6998661. doi:10.1002/1878-0261.12605
Nobre, A. R., Dalla, E., Yang, J., Huang, X., Wullkopf, L., Risson, E., et al. (2022). ZFP281 drives a mesenchymal-like dormancy program in early disseminated breast cancer cells that prevents metastatic outgrowth in the lung. Nat. Cancer 3, 1165–1180. Epub 2022/09/02PubMed PMID: 36050483. doi:10.1038/s43018-022-00424-8
Oh, S. H., Swiderska-Syn, M., Jewell, M. L., Premont, R. T., and Diehl, A. M. (2018). Liver regeneration requires Yap1-TGFβ-dependent epithelial-mesenchymal transition in hepatocytes. J. Hepatol. 69 (2), 359–367. Epub 2018/05/15PubMed PMID: 29758331; PubMed Central PMCID: PMCPMC6349217. doi:10.1016/j.jhep.2018.05.008
Pieraccioli, M., Nicolai, S., Pitolli, C., Agostini, M., Antonov, A., Malewicz, M., et al. (2018). ZNF281 inhibits neuronal differentiation and is a prognostic marker for neuroblastoma. Proc. Natl. Acad. Sci. U. S. A. 115 (28), 7356–7361. Epub 2018/06/27PubMed PMID: 29941555; PubMed Central PMCID: PMCPMC6048510. doi:10.1073/pnas.1801435115
Pierdomenico, M., Palone, F., Cesi, V., Vitali, R., Mancuso, A. B., Cucchiara, S., et al. (2018). Transcription factor ZNF281: A novel player in intestinal inflammation and fibrosis. Front. Immunol. 9, 2907. Epub 2019/01/09PubMed PMID: 30619271; PubMed Central PMCID: PMCPMC6297801. doi:10.3389/fimmu.2018.02907
Robin, X., Turck, N., Hainard, A., Tiberti, N., Lisacek, F., Sanchez, J. C., et al. (2011). pROC: an open-source package for R and S+ to analyze and compare ROC curves. BMC Bioinforma. 12, 77. Epub 2011/03/19PubMed PMID: 21414208; PubMed Central PMCID: PMCPMC3068975. doi:10.1186/1471-2105-12-77
Sadłecki, P., Grabiec, M., Grzanka, D., Jóźwicki, J., Antosik, P., and Walentowicz-Sadłecka, M. (2019). Expression of zinc finger transcription factors (ZNF143 and ZNF281) in serous borderline ovarian tumors and low-grade ovarian cancers. J. Ovarian Res. 12 (1), 23. Epub 2019/03/20PubMed PMID: 30885238; PubMed Central PMCID: PMCPMC6423742. doi:10.1186/s13048-019-0501-9
Sarig, R., and Tzahor, E. (2017). The cancer paradigms of mammalian regeneration: Can mammals regenerate as amphibians? Carcinogenesis 38 (4), 359–366. Epub 2017/03/24PubMed PMID: 28334384. doi:10.1093/carcin/bgw103
Schäfer, M., and Werner, S. (2008). Cancer as an overhealing wound: An old hypothesis revisited. Nat. Rev. Mol. Cell Biol. 9 (8), 628–638. Epub 2008/07/17PubMed PMID: 18628784. doi:10.1038/nrm2455
Seo, K. W., Roh, K. H., Bhandari, D. R., Park, S. B., Lee, S. K., and Kang, K. S. (2013). ZNF281 knockdown induced osteogenic differentiation of human multipotent stem cells in vivo and in vitro. Cell Transpl. 22 (1), 29–40. Epub 2012/09/12PubMed PMID: 22963690. doi:10.3727/096368912x654948
Sjöstedt, E., Zhong, W., Fagerberg, L., Karlsson, M., Mitsios, N., Adori, C., et al. (2020). An atlas of the protein-coding genes in the human, pig, and mouse brain. Science 367 (6482), eaay5947. Epub 2020/03/07PubMed PMID: 32139519. doi:10.1126/science.aay5947
Stone, N. R., Gifford, C. A., Thomas, R., Pratt, K. J. B., Samse-Knapp, K., Mohamed, T. M. A., et al. (2019). Context-specific transcription factor functions regulate epigenomic and transcriptional dynamics during cardiac reprogramming. Cell Stem Cell 25 (1), 87–102. e9. Epub 2019/07/05PubMed PMID: 31271750; PubMed Central PMCID: PMCPMC6632093. doi:10.1016/j.stem.2019.06.012
Tang, W., Qi, J., Wang, Q., Qu, Y., Fu, S., and Luan, J. (2022). Investigating the adipogenic effects of different tissue-derived decellularized matrices. Front. Bioeng. Biotechnol. 10, 872897. Epub 2022/05/03PubMed PMID: 35497363; PubMed Central PMCID: PMCPMC9046558. doi:10.3389/fbioe.2022.872897
Vivian, J., Rao, A. A., Nothaft, F. A., Ketchum, C., Armstrong, J., Novak, A., et al. (2017). Toil enables reproducible, open source, big biomedical data analyses. Nat. Biotechnol. 35 (4), 314–316. Epub 2017/04/12PubMed PMID: 28398314; PubMed Central PMCID: PMCPMC5546205. doi:10.1038/nbt.3772
Wissink, M. J., Beernink, R., Pieper, J. S., Poot, A. A., Engbers, G. H., Beugeling, T., et al. (2001). Binding and release of basic fibroblast growth factor from heparinized collagen matrices. Biomaterials 22 (16), 2291–2299. Epub 2001/07/18PubMed PMID: 11456069. doi:10.1016/s0142-9612(00)00418-x
Xue, Y. B., Ding, M. Q., Xue, L., and Luo, J. H. (2019). CircAGFG1 sponges miR-203 to promote EMT and metastasis of non-small-cell lung cancer by upregulating ZNF281 expression. Thorac. Cancer 10 (8), 1692–1701. Epub 2019/06/28PubMed PMID: 31243884; PubMed Central PMCID: PMCPMC6669801. doi:10.1111/1759-7714.13131
Zhang, E., Hou, X., Hou, B., Zhang, M., and Song, Y. (2020). A risk prediction model of DNA methylation improves prognosis evaluation and indicates gene targets in prostate cancer. Epigenomics 12 (4), 333–352. Epub 2020/02/07PubMed PMID: 32027524. doi:10.2217/epi-2019-0349
Zhang, J., Rector, J., Lin, J. Q., Young, J. H., Sans, M., Katta, N., et al. (2017). Nondestructive tissue analysis for ex vivo and in vivo cancer diagnosis using a handheld mass spectrometry system. Sci. Transl. Med. 9 (406), eaan3968. Epub 2017/09/08PubMed PMID: 28878011; PubMed Central PMCID: PMCPMC5830136. doi:10.1126/scitranslmed.aan3968
Zhang, S., Lu, Q., Cao, T., and Toh, W. S. (2016). Adipose tissue and extracellular matrix development by injectable decellularized adipose matrix loaded with basic fibroblast growth factor. Plast. Reconstr. Surg. 137 (4), 1171–1180. Epub 2016/03/29PubMed PMID: 27018672. doi:10.1097/prs.0000000000002019
Zhou, H., Morales, M. G., Hashimoto, H., Dickson, M. E., Song, K., Ye, W., et al. (2017). ZNF281 enhances cardiac reprogramming by modulating cardiac and inflammatory gene expression. Genes Dev. 31 (17), 1770–1783. Epub 2017/10/07PubMed PMID: 28982760; PubMed Central PMCID: PMCPMC5666675. doi:10.1101/gad.305482.117
Keywords: ZNF281, regeneration, tumorigenesis, biomarker, pan-cancer
Citation: Hou X, Luan J and Fu S (2023) Multi-functional gene ZNF281 identified as a molecular biomarker in soft tissue regeneration and pan-cancer progression. Front. Genet. 13:1082654. doi: 10.3389/fgene.2022.1082654
Received: 28 October 2022; Accepted: 15 December 2022;
Published: 05 January 2023.
Edited by:
Jiao Hu, Xiangya Hospital, Central South University, ChinaReviewed by:
Jian Kang, Northeastern University, ChinaZhixiang Yu, Fourth Military Medical University, China
Xiaokang Zhang, Beijing Tiantan Hospital, Capital Medical University, China
Copyright © 2023 Hou, Luan and Fu. This is an open-access article distributed under the terms of the Creative Commons Attribution License (CC BY). The use, distribution or reproduction in other forums is permitted, provided the original author(s) and the copyright owner(s) are credited and that the original publication in this journal is cited, in accordance with accepted academic practice. No use, distribution or reproduction is permitted which does not comply with these terms.
*Correspondence: Jie Luan, luanjieplastic@126.com; Su Fu, doctorsufu@163.com
†These authors have contributed equally to this work and share last authorship