- 1The First Clinical Medical College, School of Medicine, Nanchang University, Nanchang, China
- 2Department of Assisted Reproduction, The First Affiliated Hospital of Nanchang University, Nanchang, China
- 3Postgraduate Union Training Base of Jinzhou Medical University, Xiangyang No 1 People’s Hospital, Hubei University of Medicine, Xiangyang, China
- 4Key Laboratory of Zebrafish Modeling and Drug Screening for Human Diseases of Xiangyang City, Department of Obstetrics and Gynaecology, Xiangyang No. 1 People’s Hospital, Hubei University of Medicine, Xiangyang, China
Background: Cervical cancer (CC), the fourth most common cancer among women worldwide, has high morbidity and mortality. Necroptosis is a newly discovered form of cell death that plays an important role in cancer development, progression, and metastasis. However, the expression of necroptosis-related genes (NRGs) in CC and their relationship with CC prognosis remain unclear. Therefore, we screened the signature NRGs in CC and constructed a risk prognostic model.
Methods: We downloaded gene data and clinical information of patients with cervical squamous cell carcinoma and endocervical adenocarcinoma (CESC) from The Cancer Genome Atlas (TCGA) database. We performed functional enrichment analysis on the differentially expressed NRGs (DENRGs). We constructed prognostic models and evaluated them by Cox and LASSO regressions for DENRGs, and validated them using the International Cancer Genome Consortium (ICGC) dataset. We used the obtained risk score to classify patients into high- and low-risk groups. We employed the ESTIMATE and single sample gene set enrichment analysis (ssGSEA) algorithms to explore the relationship between the risk score and the clinical phenotype and the tumor immune microenvironment.
Results: With LASSO regression, we established a prognostic model of CC including 16 signature DENRGs (TMP3, CHMP4C, EEF1A1, FASN, TNF, S100A10, IL1A, H1.2, SLC25A5, GLTP, IFNG, H2AC13, TUBB4B, AKNA, TYK2, and H1.5). The risk score was associated with poor prognosis in CC. Survival was lower in the high-risk group than the low-risk group. The nomogram based on the risk score, T stage, and N stage showed good prognostic predictive power. We found significant differences in immune scores, immune infiltration analysis, and immune checkpoints between the high- and low-risk groups (p < 0.05).
Conclusion: We screened for DENRGs based on the TCGA database by using bioinformatics methods, and constructed prognostic models based on the signature DENRGs, which we confirmed as possibly having important biological functions in CC. Our study provides a new perspective on CC prognosis and immunity, and offers a series of new targets for future treatment.
1 Introduction
Cervical cancer (CC) is one of the most common cancers in women, and it is the fourth leading cause of cancer death (Vu et al., 2018). Globally, there are an estimated 530,000 new cases and 270,000 deaths each year. Today, preventive human papillomavirus (HPV) vaccination for CC is available worldwide, but more than a quarter of patients with CC still die each year due to a severe lack of medical supplies in many developing countries (Small et al., 2017). Despite the multidisciplinary approach of surgery combined with chemotherapy that has been applied to patients with CC, their prognosis remains unsatisfactory, making the search for an effective therapeutic target an urgent issue (Ghasemi et al., 2019).
Cell death is an important component in maintaining homeostasis in an organism, and resistance to cell death is usually the cause of tumor formation. Cell death can be divided into two types: necrosis and apoptosis. In recent years, a novel type of cell death has been identified that differs from necrosis and apoptosis, namely necroptosis, which is mechanistically and morphologically similar to apoptosis and necrosis (Gong et al., 2019). Necroptosis, a complementary mode of apoptotic failure, is a type of programmed cell death that is activated by caspase-independent signaling pathways, mainly by the receptor-interacting protein kinase 1 and 3 (RIPK1/RIPK3)/mixed lineage kinase domain-like protein (MLKL) complex (Quarato et al., 2016). Necroptosis is thought to play a key role in cancer progression and metastasis, and some studies have identified necroptosis-related genes (NRGs) as possible biomarkers of cancer prognosis (Gong et al., 2019). MLKL has recently been identified as a downstream component of RIPK3, a key factor in tumor necrosis factor (TNF)-induced necroptosis, and as a prognostic biomarker in CC (Ruan et al., 2015). Necroptosis also plays an important role in tumor immunology and cancer immunotherapy, where it is involved in triggering CD8+ T cell–driven antitumor immunity (Sprooten et al., 2020). RIPK3, a regulator of necroptosis in tumor cells, also serves as a novel predictive marker for cancer immunotherapy personalization (Smola, 2016). Fibroblasts in the tumor microenvironment (TME) induce a robust immune response through necroptosis and initiate transduction through nuclear factor κB (NF-κB) signaling (Yatim et al., 2015). Considering its important role in cancer biology and antitumor immunity, necroptosis has emerged as a new target for bypassing cell death resistance and modulating antitumor immunity and tumor therapy in oncological treatment. Induction of necroptosis by pharmacological intervention is emerging as a promising tool for multiple anti-apoptotic cancer cells. RETRA has been shown to play a role in CC treatment as a drug-induced necroptosis anticancer agent by selectively inducing necroptosis in CC cells through phosphorylation of the structural domains of RIPK1/RIPK3 and MLKL (Mohanty et al., 2022). However, to date, few studies have investigated the significance of NRGs in the prognosis and immunotherapy of CC.
In this study, we screened NRGs as prognostic biomarkers for CC using The Cancer Genome Atlas (TCGA) database and constructed an associated risk prediction model based on 16 signature DENRGs. We comprehensively analyzed the role of NRGs in CC and highlighted their prognostic and immunotherapeutic potential for CC. Analysis of immune infiltration, TME, immune checkpoints, mutations, and clinicopathological features revealed significant differences between the high- and low-risk CC groups. Our study provides accurate prognostic predictions and effective immunotherapy strategies for patients with CC.
2 Materials and methods
2.1 Data collection
We extracted transcriptome profiles, clinical characteristics, and tumor mutation data (simple nucleotide variation) of patients with cervical squamous cell carcinoma and endocervical adenocarcinoma (CESC) from the TCGA database (https://portal.gdc.cancer.gov/). We collected NRGs from the following Gene Ontology (GO) terms: necroptotic process (GO:0070266), execution phase of necroptosis (GO:0097528), necroptotic signaling pathway (GO:0097527), negative regulation of necroptotic process (GO:0060546), positive regulation of necroptotic process (GO:0060545), regulation of necroptotic process (GO:0060544), ripoptosome assembly involved in necroptotic process (GO:1901026), negative regulation of programmed necrotic cell death (GO:0062099), positive regulation of programmed necrotic cell death (GO:0062100), programmed necrotic cell death in response to starvation (GO:0097385), regulation of mitochondrial membrane permeability involved in programmed necrotic cell death (GO:1902445), and regulation of programmed necrotic cell death (GO:0062098). We identified 651 genes associated with necrotizing apoptosis via GeneCards. A published study proposed 159 genes (Zhang et al., 2022). After removing the duplicated genes involved in the above GO terms, we had a total of 749 NRGs for the downstream analysis.
2.2 Identification and functional analysis of differentially expressed NRGs in CC
We identified differentially expressed genes (DEGs) between 306 CC and three adjacent control samples by using the “DESeq2” R package with |log2FC| >1 and adjusted p-value <0.05 as criteria (Waardenberg and Field, 2019). We obtained differentially expressed NRGs (DENRGs) by overlapping DEGs with the 749 NRGs. We used the “ClusterProfiler” R package to screen significantly enriched GO terms and Kyoto Encyclopedia of Genes and Genomes (KEGG) pathways of DENRGs with the threshold of an adjusted p-value <0.05. Furthermore, we uploaded DENRGs to the STRING database (https://string-db.org/) to investigate their interactions.
2.3 Construction and verification of the risk score model in CC
According to the expressions of prognostic DENRGs and coefficients, we calculated the risk score of each patient in the training set with the following formula: ∑(coefficient × gene expression). According to the median value of the risk score, we divided patients in the training set into high- and low-risk groups. We analyzed the overall survival of high- and low-risk groups with Kaplan-Meier analysis. To evaluate the accuracy of risk score model, we plotted receiver operating characteristic (ROC) curves using “survivalROC” in R. We used the International Cancer Genome Consortium (ICGC) dataset as the validation group to verify the above results. Moreover, to test the reliability of the risk score model, we conducted similar analyses in the validation set.
2.4 Construction of the nomogram to predict prognosis of CC
To determine independent prognostic factors for CC patients, we used clinical characteristics (age, sex, and TNM stage) and the risk score for univariate and multivariate Cox regression analysis. Then, we incorporated independent prognostic factors to construct the nomogram to predict the 1-, 3-, and 5-year survival of patients with CC. We plotted calibration curves to evaluate the performance of the nomogram.
2.5 Exploration of the mechanisms underlying necroptosis-related CC
To explore the potential mechanisms of prognostic DENRGs in regulating CC, we performed the following analyses. 1) We compared the risk score among different subgroups stratified by T stage (T1, T2, T3, T4), N stage (N0, N1), M stage (M0, M1), and grade (G1, G2, G3, G4) using the Wilcoxon or Kruskal–Wallis test to investigate the relationship between risk score and the progression of CC. 2) We downloaded GO and KEGG reference gene sets from the MSigDB database (https://www.gsea-msigdb.org/gsea/msigdb/) to perform gene set enrichment analysis (GSEA). We identified significantly enriched GO terms and KEGG pathways between the low- and high-risk score with an adjusted p-value <0.05. 3) We calculated the immune and stromal score of each patient with the ESTIMATE algorithm, and then determined the correlations between the risk score and immune/stromal scores. 4) We downloaded a 28 immune cell gene sets from The Cancer Imaging Archive (TCIA) database. We calculated the single sample GSEA (ssGSEA) scores for the different types of immune cells in each sample by using the “GSVA” package in R to compare the differences in immune infiltration levels between samples from the high- and low-risk groups (Cheng et al., 2021). We used the Wilcoxon test to analyze difference.
2.6 Analysis of the TME score, tumor mutation burden, and immune checkpoint molecules
1) We predicted the proportion of infiltrating stromal and immune cells in tumor tissue using the “estimate” package in R, based on ssGSEA. We generated the stromal score, the immune score, and the ESTIMATE score (Ke et al., 2021). 2) We downloaded mutation data from the TCGA database, selecting the TCGA-ESCA project, simple nucleotide variation as the data category, VarScan2 Variant Aggregation as the workflow type, and used masking. We used “maftools” in R to calculate the mutation load for each sample. We evaluated the mutations in the 16 genes used to establish the prognosis. 3) We extracted the expression of 47 immune checkpoint molecules from the training set expression matrix and compared their expression differences between the high- and low-risk groups using the Wilcoxon test. We screens 27 differentially expressed immune checkpoint–related genes between the high- and low-risk groups using an adjusted p-value <0.05 as the screening criterion.
2.7 Statistical analysis
We assessed the difference in overall survival between the high- and low-risk groups by the Kaplan-Meier method and log-rank test. We determined the predictive accuracy of the risk model by determining the area under the ROC curve (AUC). We used R version 4.0.0 for all analysis. We considered significant differences as p < 0.05 unless otherwise specified.
3 Results
3.1 One hundred eighty DENRGs are associated with CC
The study flow chart is presented in Figure 1. We identified 4,857 DEGs between the CC and control samples, including 2,998 upregulated and 1,859 downregulated genes (Figure 2A). We identified 749 NRGs from GeneCards and the literature; the list is shown in Table S1. The top 15 upregulated and top 15 downregulated genes are shown in a heatmap (Figure 2B); the logFC of the top 20 NRGs is shown in another heatmap (Supplementary Figure S1). A volcano plot of NRGs is presented in Figure S2. After overlapping DEGs with NRGs, we identified 180 genes as DENRGs (Figure 2C). The list of 180 DENRGs is shown in Table S2. In addition, we constructed a protein–protein interaction (PPI) network, in which we observed the interplay among most DENRGs (Figure 2D).
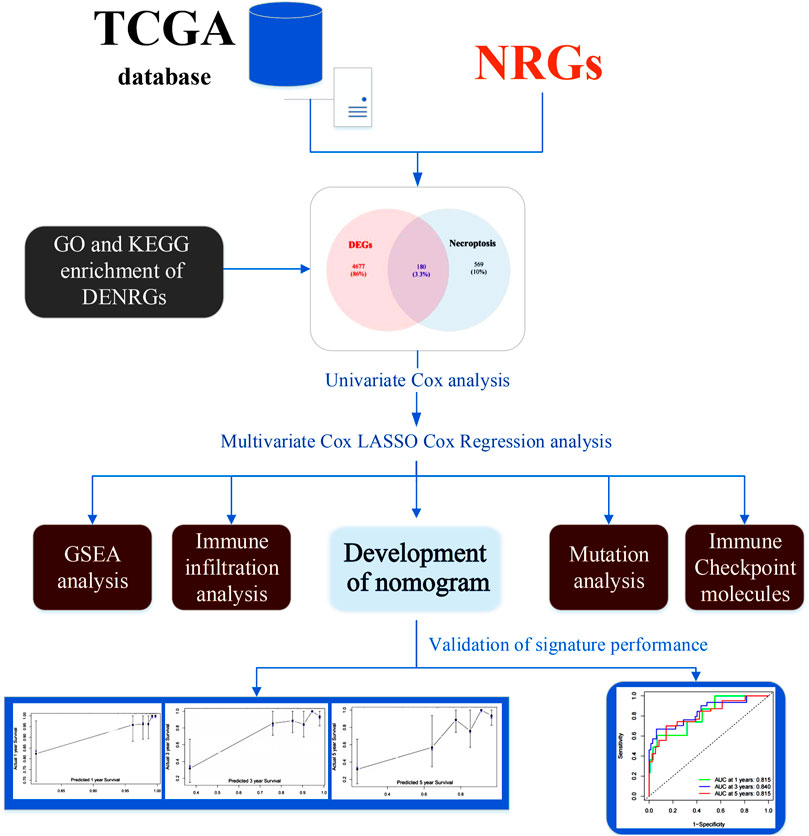
FIGURE 1. The flow chart of this study. Abbreviations: DENRGs, differentially expressed necroptosis-related genes; GO, Gene Ontology; GSEA, gene set enrichment analysis; KEGG, Kyoto Encyclopedia of Genes and Genomes; NRGs, necroptosis-related genes.
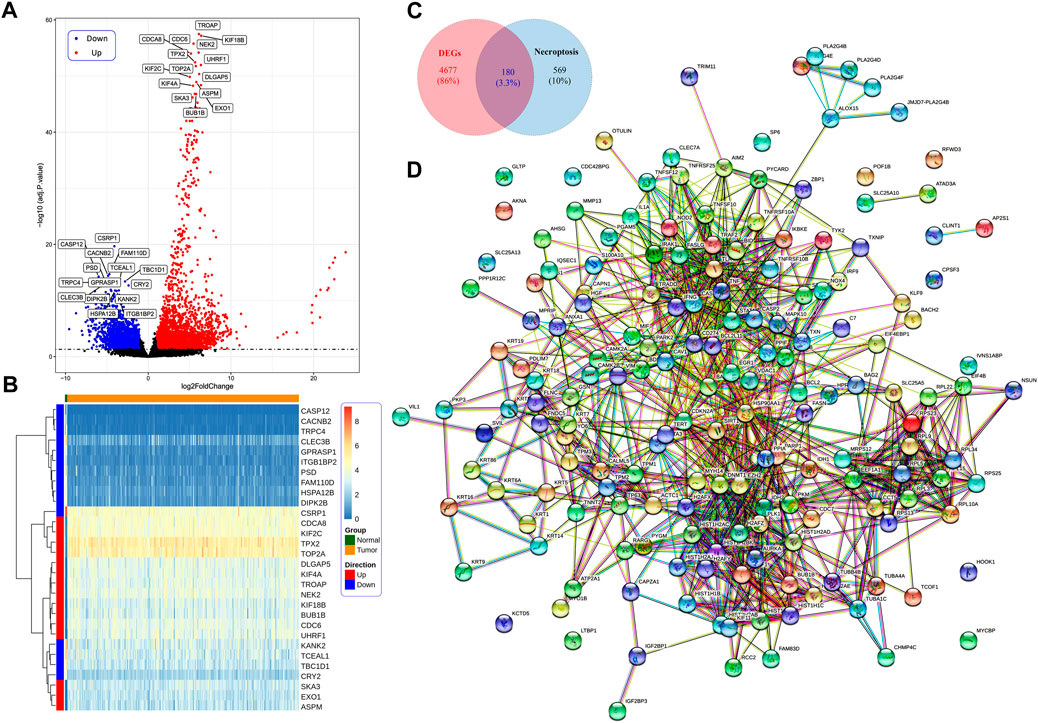
FIGURE 2. (A) Identification of differentially expressed necroptosis-related genes (DENRGs). Identification of differentially expressed genes (DEGs) in cervical cancer (CC) tumor tissue and normal control tissue based on a volcano plot. (B) The expression of the top 15 upregulated and downregulated genes in CC presented in a heatmap. (C) The expression of 180 DENRGs between the tumor and normal groups. (D) Protein–protein interaction network of the 180 DENRGs.
3.2 Functional enrichment of DENRGs
Functional analysis showed that DENRGs are mainly enriched in GO terms and KEGG pathways relevant to necroptosis and apoptosis. In biological processes, DENRGs are mainly enriched in cell death and related receptor signaling pathways. In cellular components, DENRGs are mainly enriched in DNA damage repair related pathways. In molecular functions, DENRGs are enriched in pattern recognition receptor activity pathways (Figures 3A–C). Moreover, KEGG analysis revealed that these DENRGs are mainly enriched in necroptosis, influenza A, and apoptosis (Figure 3D).
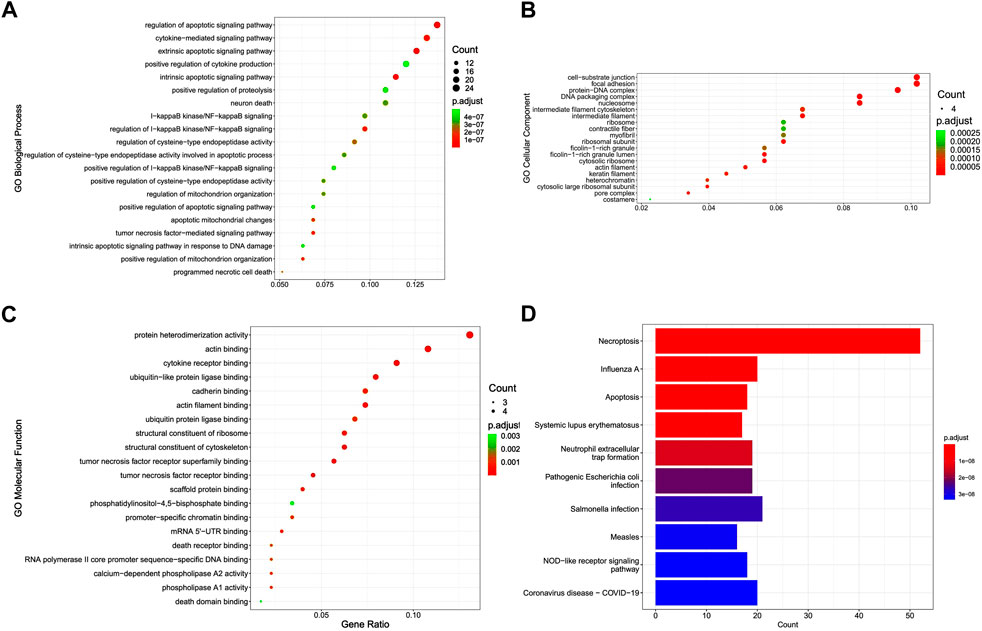
FIGURE 3. Gene Ontology (GO) and Kyoto Encyclopedia of Genes and Genomes (KEGG) enrichment analysis of differentially expressed necroptosis-related genes (DENRGs). GO enrichment of DENRGs for (A) biological processes, (B) cellular components, and (C) molecular functions. (D) KEGG enrichment of DENRGs.
3.3 Construction and validation of DENRG-related prognostic signature
We evaluated the prognostic value of 16 DENRGs in CC by univariate Cox and LASSO regressions. By univariate Cox regression, we found 26 DENRGs (TPM3, CHMP4C, EEF1A1, FASN, TNF, MY O 1B, S100A10, IL1A, H2BC12, ALOX15, H2AC8, H1.2, EZH2, GSN, SLC25A5, FASLG, GLTP, IFNG, H2AC13, TRAF2, TUBB4B, AKNA, BCL2, TYK2, H2AC16, and H1.5) to be significantly related with the survival of patients with CC (Figure 4A). To obtain a more robust prognostic signature, we input those 26 DENRGs into the LASSO algorithm. We narrowed the prognostic signature down to 16 DENRGs (TPM3, CHMP4C, EEF1A1, FASN, TNF, S100A10, IL1A, H1.2, SLC25A5, GLTP, IFNG, H2AC13, TUBB4B, AKNA, TYK2, and H1.5) (Figures 4B,C). Then, we calculated the DENRG-related prognostic as: [the expression of TPM3 × 0.102505 + expression of CHMP4C × 0.189606 + expression of EEF1A1 × 0.033772 + expression of FASN × 0.192809 + expression of TNF × 0.203929 + expression of S100A10 × 0.007325 + expression of IL1A × 0.103649 + expression of H1.2 × (−0.10396) + expression of SLC25A5 × (−0.11957) + expression of GLTP × (−0.38244) + expression of IFNG × (−0.19587) + expression of H2AC13 × (−0.10861) + expression of TUBB4B × (−0.31664) + expression of AKNA × (−0.27736) + expression of TYK2 × (−0.27591) + expression of H1.5 × (−0.34445)]. These 16 prognostic DENRGs were expressed abnormally in the CC samples compared with the normal samples (Figure 4D).
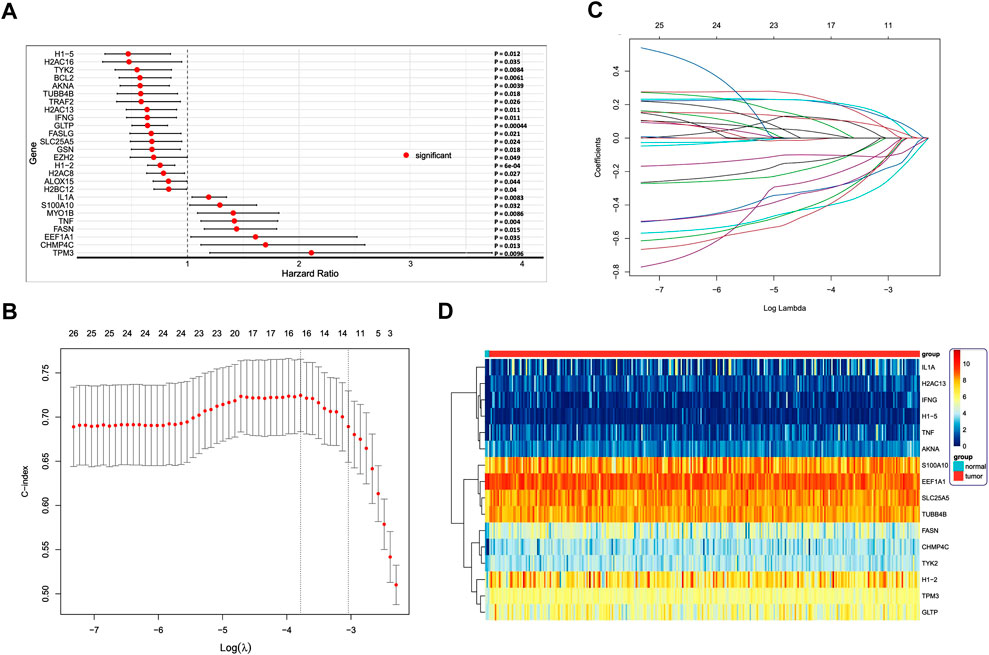
FIGURE 4. (A) Cox regression analysis to identify differentially expressed necroptosis-related genes (DENRGs) related to biochemical recurrence-free survival. Univariate Cox regression identified 26 DENRGs significantly associated with prognosis (p < 0.05). (B) Screening for genes that can be used independently for prognostic risk prediction using the best LASSO model parameter λ. (C) Variable number change. (D) Heatmap of the expression of the 16 DENRGs in the high- and low-risk groups.
According to the median risk score, we divided the patients with CC in the TCGA training set into high- and low-risk groups (Figure 5A). As the risk score increased, we observed more dead patients (Figure 5B). The high-risk group had worse survival compared with the low-risk group (Figure 5C). The AUC of the ROC curves were 0.792, 0.818, and 0.855 for 1-, 3-, and 5-year survival, respectively (Figure 5D), suggesting that the risk score model had good performance in predicting the prognosis of patients with CC. Furthermore, we tested the risk score model in the ICGC validation set and obtained similar results (Figures 5E–G). The AUC of ROC curves in the validation set were 0.767, 0.788 and 0.828 for 1-, 3-, and 5-year survival (Figure 5H), further demonstrating the reliability of the DENRG-related prognostic signature in predicting the survival of patients with CC.
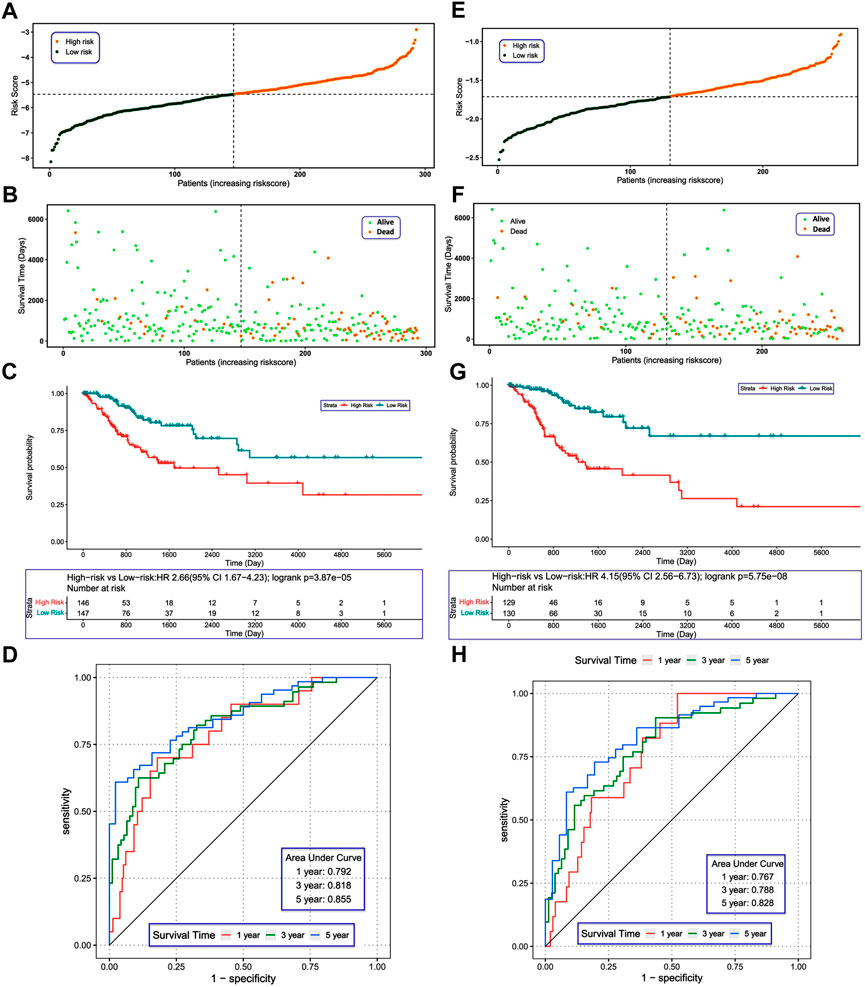
FIGURE 5. (A) Construction and evaluation of a risk model. Distribution of risk scores in the training set. (B) Survival status of patients in the training set. (C) Kaplan–Meier plot of the training set (p < 0.05). (D) Receiver operating characteristic curves showing the 1-, 3-, and 5-year predictive efficiency of the risk score. (E) Distribution of risk scores in the validation set. (F) Survival status of patients in the validation set. (G) Kaplan–Meier plot of the validation set (p < 0.05). (H) The area under the receiver operating characteristic curves in the validation set for 1-, 3-, and 5-year survival.
3.4 The development of a DENRG-related nomogram
We performed univariate and multivariate analyses with independent prognostic factors in CC, including the risk score, T stage, N stage (Figures S3, 6A). We established the nomogram based on prognostic factors to predict the 1-, 3-, and 5-year survival of patients with CC (Figure 6B). Calibration curves showed that the predicted overall survival was close to the actual overall survival (Figures 6C–E). The AUC values for 1-, 3-, and 5-year survival of the nomogram model were 0.815, 0.840, and 0.815, respectively, indicating the good performance of the nomogram (Figure 6F).
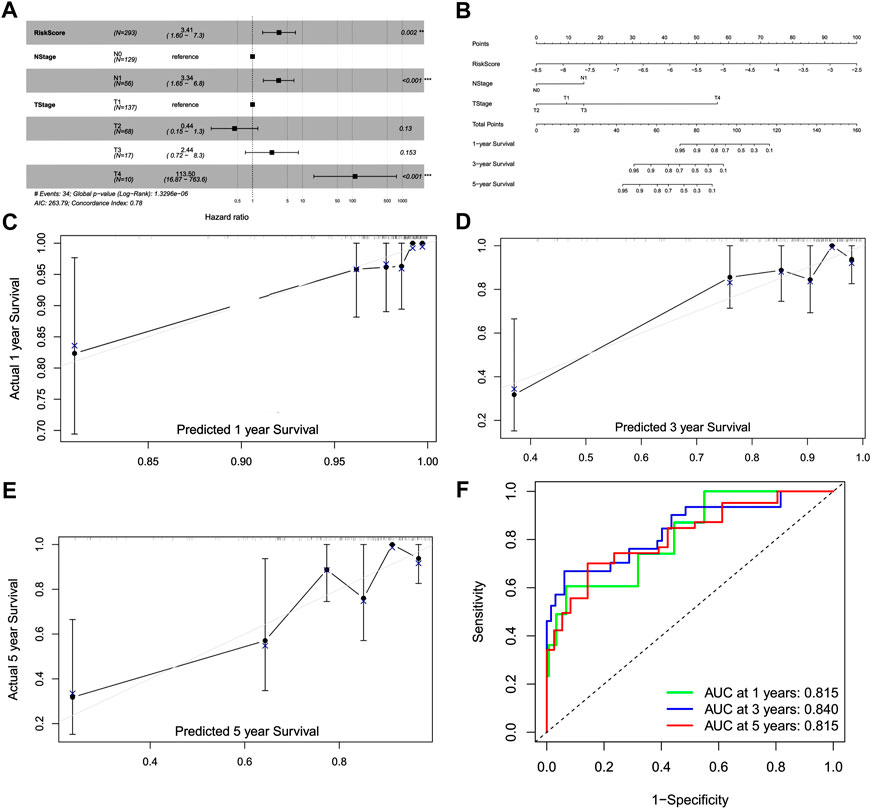
FIGURE 6. Construction of a prognostic model and nomogram. (A) Forest plot of the multivariate Cox model. (B) Nomogram for the prognostic model. (C) Calibration curve for 1-year survival. (D) Calibration curve for 3-year survival. (E) Calibration curve for 5-year survival. (F) Receiver operating characteristic curve for 1-, 3-, and 5-year survival.
3.5 Risk score and tumor stage
To further evaluate the utility of prognostic DENRGs, we compared the risk scores among different groups divided by T stage, N stage, M stage, and grade. Interestingly, we noticed that the risk score increased with the progression of CC, and patients with T4 CC had the highest risk scores (p < 0.05). However, the risk score was not significantly changed in other stages and grades (p > 0.05) (Figures 7A–D). These results indicate that prognostic DENRGs play important roles in the T stage metastasis and malignancy degree of CC, which may further affect the survival of patients with CC.
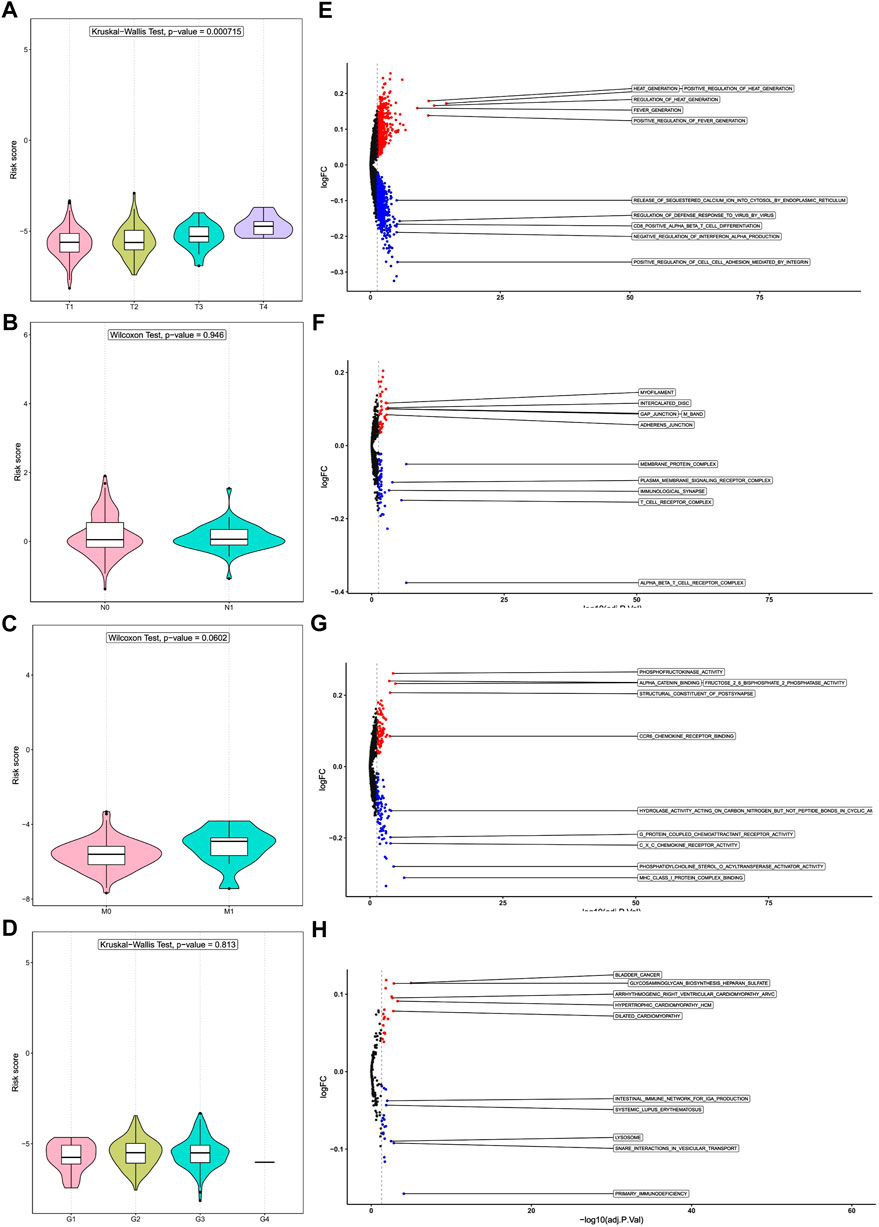
FIGURE 7. Risk score and tumor stages and gene set enrichment analysis (GSEA). Difference in risk score among (A) T stages, (B) N stages, (C) M stages, and (D) grades. Differences in pathway enrichment in tumors of patients in the high- and low-risk groups: (E) Gene Ontology (GO) analysis of biological processes, (F) GO analysis of cell components, and (G) GO analysis of molecular functions. (H) Kyoto Encyclopedia of Genes and Genomes (KEGG) enrichment analysis.
3.6 Gene set variation analysis between high- and low-risk groups
We performed GSVA to unveil the potential molecular mechanisms and to identify biological processes. For GO biological process, cellular component, and molecular function analysis, the pathways enriched in the high-risk group include cell junctions, phosphorylation, and other related pathways; the pathways enriched in the low-risk group include those related to viral defense, T-cell differentiation, cell adhesion, cytokine receptors, and protein coupling (Figures 7E–G). Among the KEGG pathways, the high-risk group was significantly enriched in pathways related to glycosaminoglycan biosynthesis, while the low-risk group was significantly enriched in pathways related to immunity and lysosomes (Figure 7H). These results suggest that prognostic DENRGs may regulate the development and progression of CC via cell proliferation.
3.7 Differences in immune-related outcomes between the high- and low-risk groups
Increasing evidence has demonstrated the important role of the immune microenvironment in the outcome of CC. Thus, we explored whether NRGs could modulate the immune microenvironment of patients with CC. We found that the risk score was not correlated with stromal score (p > 0.05, Figure 8A). In addition, although the risk score was significantly correlated with immune score, it was weak (p < 0.01, r = -0.303, Figure 8B). The TME score analysis showed that the immune score was significantly higher in the low-risk group than in the high-risk group (p < 0.01, Figure 8C). Furthermore, we compared the immune infiltration between patients in the low- and high-risk groups and observed that the infiltration levels of activated B-cell, activated CD4+ T-cell, activated CD8+ T-cell, activated dendritic cells, central memory CD4+ T-cell, effector memory CD4+ T-cell, immature B-cell, immature dendritic cells, natural killer T-cell, and natural killer T-cell, were different (Figure 8D). Figure 8E shows the mutation status of the 16 genes used to build the prognostic model.
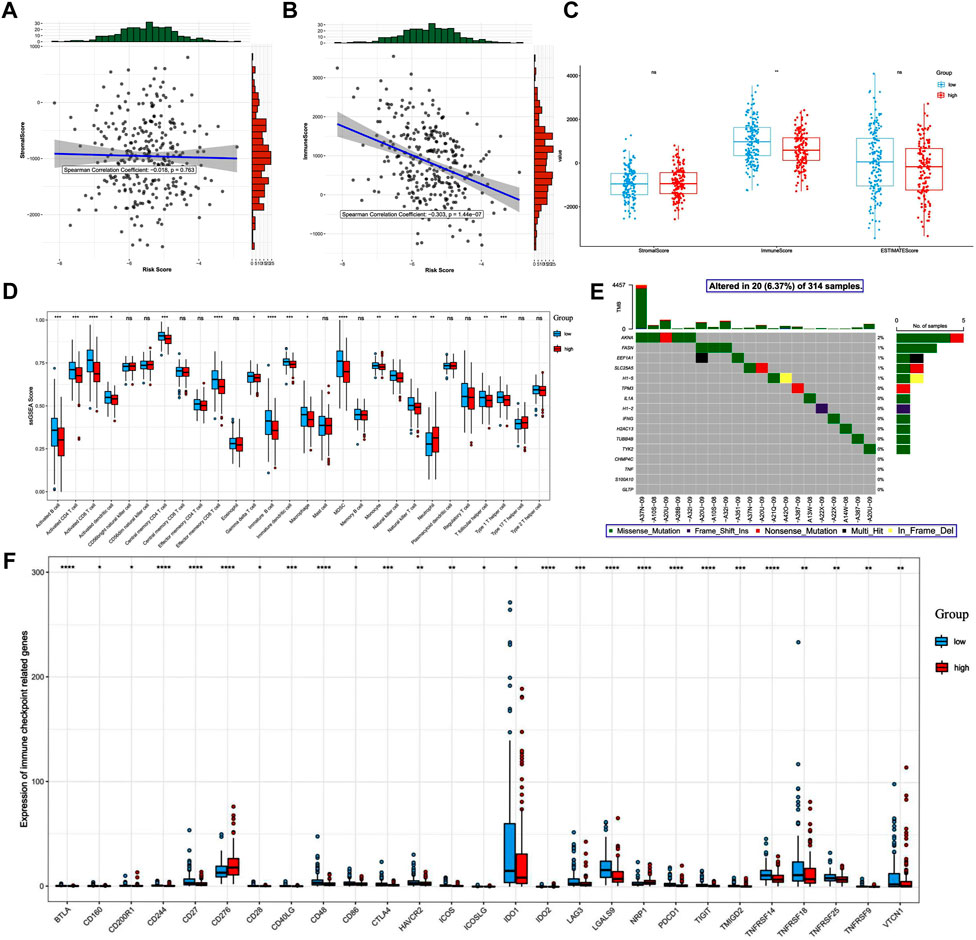
FIGURE 8. Differences in immune-related outcomes between the high-risk and low-risk groups. Risk and ESTIMATE scores: (A) correlation between the risk score and the stromal score. (B) Correlation between the risk score and the immune score. (C) Differences in tumor microenvironment scores between the high- and low-risk groups. (D) Boxplot of differences in the degree of immune cell infiltration. (E) Mutation map of prognosis-related genes in patients with cervical cancer. (F) Differential expression of immune checkpoint molecules.
4 Discussion
The occurrence and development of CC is a complex process regulated by multiple factors, and the incidence of CC has been trending younger in recent years (Torre et al., 2015; Small et al., 2017). Necroptosis is a newly discovered mode of programmed cell death and often used as an alternative when apoptosis induction is compromised. Necroptosis is involved in tumor proliferation and metastasis and is closely associated with the TME and tumor immunity (Su et al., 2015). In recent years, it has been shown that necroptosis enhances anti-tumor immunity in cancer therapy and could become an effective cancer treatment (Wu et al., 2022). Therefore, we developed an NRG characterization model to predict the prognosis of patients with CC, and we used the obtained risk score to group them into high- and low-risk groups for the analysis of clinical indicators, the ESTIMATE score, the tumor mutational load, immune checkpoint molecules, and immune infiltrating cells.
Both apoptosis and necroptosis are forms of programmed cell death, which is a natural barrier limiting the survival and propagation of malignant cells. The molecular mechanisms regulating necroptosis are closely related to the signaling cascades that control apoptosis and necrosis (Schmidt et al., 2015). MLKL is a prognostic biomarker for cervical squamous cell carcinoma and has recently been identified as a key RIPK3 downstream component of TNF-induced necroptosis (Ruan et al., 2015). Through the use of CC models, PolyIC-driven immunogenicity has been shown to be dependent on the necroptosis regulator RIPK3 in tumor cells, suggesting that RIPK3 could serve as a novel predictive marker for the personalization of cancer immunotherapy (Smola, 2016). Considering the above criteria, NRGs may be involved in necroptosis, necrosis, apoptosis, tumor immunity, and their interactions. Consistently, based on our GO, KEGG, and GSEA analyses, these DENRGs are involved in necroptosis, necrosis, apoptosis, and immune- and tumor-related pathways.
We identified 16 DENRGs, and they have been reported that are associated with necroptosis. TPM3, CHMP4C, EEF1A1, FASN, TNF, S100A10, and IL1A represent a high-risk score and poor prognosis, suggesting that these genes may be associated with the tumor process in patients with CC and appear to be pro-oncogenes. In contrast, H1.2, SLC25A5, GLTP, IFNG, H2AC13, TUBB4B, AKNA, TYK2, and H1.5 are abundantly expressed in the low-risk group, suggesting that these genes may be oncogenes for CC. CHMP4C is highly expressed in CC tissues and cell lines and plays a role as a pro-oncogene in them (Lin et al., 2020). The EEF1A protein blocks apoptosis and facilitates viral replication and interacts with the E7 protein of human papillomavirus (HPV) 38 to participate in events related to incidence of CC formation-related events (Ghittoni et al., 2010). FASN plays a key role in tumor lipid metabolism and is associated with the tumor-associated phosphoinositide 3-kinase (PI3K)/AKT signaling pathway. Its overexpression is often associated with tumor progression and poor prognosis (Cao et al., 2017). FASN may be a potential therapeutic target for CC, and a FASN inhibitor (orlistat) reduces apoptosis triggered by CC cells (C-33A, ME-180, HeLa, and SiHa) in a time-dependent manner (Nascimento et al., 2022). S100A10 may have anti-apoptotic effects in cancer cells, interacting with Bad and impeding its pro-apoptotic activity (Bharadwaj et al., 2021). The S100A10 subunit promotes L2-mediated human papillomavirus infection, which is associated with the development of CESC (Taylor et al., 2018). Elevated expression of IL1A, a pleiotropic pro-inflammatory cytokine, is associated with poorer prognosis in CC through multiple complex mechanisms involving cell proliferation, apoptosis, angiogenesis, and the inflammatory microenvironment (Song et al., 2016). There is growing evidence that H1-2 has important functions in multiple cellular processes including apoptosis, autophagy, the cell cycle, and gene transcription. H1-2 acts as a signaling molecule to initiate apoptosis, and its deletion may lead to resistance to apoptosis in mice and tumor cells (Lai et al., 2021). Both SLC25A5 and GLTP are associated with good prognosis in CC (Qu et al., 2021). Colon cancer cells overexpressing GLTP (HT-29) exhibit RIPK-3-mediated MLKL phosphorylation, increased intracellular Ca2+, levels and induce cell death through necroptosis (Mishra et al., 2019). The CC-associated oncoprotein HPV E6 can downregulate AKNA and lead to cancer progression (Wang et al., 2020). In contrast, AKNA contributes to dysregulation of the cancer immune system and can serve as a genetic factor and biomarker of susceptibility to CC (Ramírez-González et al., 2021). This is consistent with our results that TYK2 is a protective gene in the prognostic model of necroptosis in CC (Ding et al., 2020). Both TNF and IFGN are triggers of necroptosis and can synergistically induce RIPK-dependent necroptosis (Hojo et al., 2019). MLKL was initially identified as a key mediator of TNF-induced necroptosis and can be used to assess the prognosis of patients with cervical squamous cell carcinoma, and TNF is also important for immune and cellular homeostasis in mammals (Ruan et al., 2015). Its role as a major regulator to balance cell survival, apoptosis, and necroptosis has been studied extensively in various cell types and tissues (Blaser et al., 2016). IFNG is an immune response gene and some of its single nucleotide polymorphisms (SNPs) are associated with cervical carcinogenesis and plays a decisive prognostic role in squamous cervical cancer (Chen et al., 2020). However, there are few reports on the role of TPM3, H2AC13, TUBB4B, and H1.5 in CC, which will be an important direction for our future research.
Resistance to apoptosis is one of the characteristics of tumors, and therefore induction of cell death mechanisms other than apoptosis is emerging as a new cancer treatment strategy. Necroptosis mediates cancer-related immune responses by promoting interactions between cancer and immune cells through the release of damage-associated molecular patterns (DAMPs), cytokines, or chemokines in the TME (Sprooten et al., 2020). The TME plays an important role in the course of tumorigenesis, progression, and prognosis of CC. In this study, we found that risk scores were negatively correlated with immune scores, and different risk score groups showed different TME infiltration characteristics. In low-risk group, the abundance of CD8+ T-cell, CD4+ T-cell, and NK cells were increased. These immune cells have been widely reported as effector cells in the TME, and have a positive immune response to cancer cells (Litwin et al., 2021). Furthermore, tumor cells undergo necroptosis, which activates CD8+ T-cell to eliminate cancer cells and thus induce an anti-tumor immune response. Necroptosis-associated genes (EEF1A1, IFNG) can activate CD8+ T-cell (Guan et al., 2022). DAMP from necrotic tumor cells can induce strong anti-tumor CD8+ T-cell expression (Yatim et al., 2015). According to our results, it indicates that the lower the risk score, the better the immunity and prognosis of patients. In high-risk group, neutrophil was increased. Tumors can increase tumor cell proliferation by inducing the conversion of neutrophils into tumor-associated neutrophils and releasing inflammatory mediators (Demkow, 2021). Therefore, the patients with high risk score might have more severe inflammatory reaction, tumor proliferation and worse prognosis. Overall, we could roughly predict immunity of patients according to their risk score.
Immune checkpoints present an effective immunosuppressive mechanism in cancer, providing more effective treatment options to improve cancer survival. Immune checkpoint inhibitor (ICI) therapy is considered an effective treatment for CC (Mauricio et al., 2021). In our results, most immune checkpoint molecules—including BTLA, CD27, CD28, CD86, CTLA4, ICOS, ID O 1, TIGIT, TNFRSF14, TNFRSF18, TNFRSF25, TNFRSF9, and VTCN1—are highly expressed in the low-risk group. CTLA-4, CD28, BTLA, TIGIT, and ICOS belong to the immunoglobulin-associated receptor family and are responsible for various aspects of T-cell immune regulation. CTLA-4 is an immune checkpoint protein receptor that downregulates the immune system. CTLA-4 has been identified as a prognostic marker in CC, and CTLA-4 inhibitors CTLA-4 inhibitors in combination with radiation/chemotherapy may improve outcomes for patients with CC (Odiase et al., 2021). Blocking CTLA-4 allows the body to overcome HPV-driven immunosuppression associated with CC (Callahan and Wolchok, 2013). In addition to conventional ICIs targeting CTLA4, PD-1, and PD-L1, novel ICIs including agonists targeting BTLA, TIGIT, and the co-stimulatory receptor ICOS are increasingly being used in clinical therapy. IDO1 induces immunosuppression of T-cell by depleting l-tryptophan and kynurenine in the local TME, suppressing effector T-cell and over-activating regulatory T-cell (Jung et al., 2019). Blockade of IDO1 contributes to shrinkage of CC (Blocking IDO1 Helps Shrink Bladder, Cervical Tumors, 2018). In addition, members of the tumor necrosis factor receptor superfamily (TNFRSF) are present on T-cell and play a key role in T-cell development, survival, immune activation, and the anti-tumor immune response (Schaer et al., 2014). Combined with our results, patients in the high-risk group may be less sensitive to ICIs, and thus ICI treatment may be more effective in the low-risk group. Taken together, these immune checkpoint molecules may be explored as meaningful targets for CC, and the combination strategy of ICIs with radiation/chemotherapy offers a new direction for the future treatment of CC and may help to overcome resistance to radiation/chemotherapy and immunotherapy alone.
In conclusion, we have identified 16 NRGs that are significantly associated with CC prognosis. Our findings provide possible explanations for the different prognostic assessments of patients with CC and offer prospects for future studies on necroptosis as a therapeutic target for CC and exploration of new immunotherapeutic approaches.
Data availability statement
The datasets presented in this study can be found in online repositories. The names of the repository/repositories and accession number(s) can be found in the article/Supplementary Material.
Author contributions
XX wrote the main manuscript text, prepared figures and tables, conceived and designed the experiments. YT analyzed the data, prepared figures and approved the final draft. XJ reviewed the manuscript.
Funding
This article was funded by the National Natural Science Foundation of China (No. 81760285) (NO. 31160246) and the National Natural Science Foundation of China (No. 31160246).
Conflict of interest
The authors declare that the research was conducted in the absence of any commercial or financial relationships that could be construed as a potential conflict of interest.
Publisher’s note
All claims expressed in this article are solely those of the authors and do not necessarily represent those of their affiliated organizations, or those of the publisher, the editors and the reviewers. Any product that may be evaluated in this article, or claim that may be made by its manufacturer, is not guaranteed or endorsed by the publisher.
Supplementary material
The Supplementary Material for this article can be found online at: https://www.frontiersin.org/articles/10.3389/fgene.2022.1061107/full#supplementary-material
Abbreviations
AUC, area under the curve; CESC, Cervical squamous cell carcinoma and endocervical adenocarcinoma; DAMPs, Damage-associated molecular patterns; DENRGs, Differentially expressed necroptosis-related genes; GO, Gene Ontology; GSEA, Gene set enrichment analysis; GSVA, Gene set variation analysis; HPV, Human papillomavirus; ICIs, Immune checkpoint inhibitors; KEGG, Kyoto Encyclopedia of Genes and Genomes; NRGs, Necroptosis-related genes; PPI, Protein–protein interaction; ROC, receiver operating characteristic; SNPs, Single nucleotide polymorphisms; TCGA, The Cancer Genome Atlas; TNFRSF, Tumor necrosis factor receptor superfamily.
References
Bharadwaj, A. G., Holloway, R. W., Miller, V. A., and Waisman, D. M. (2021). Plasmin and plasminogen system in the tumor microenvironment: Implications for cancer diagnosis, prognosis, and therapy. Cancers (Basel) 13 (8), 1838. doi:10.3390/cancers13081838
Blaser, H., Dostert, C., Mak, T. W., and Brenner, D. (2016). TNF and ROS crosstalk in inflammation. Trends Cell Biol. 26 (4), 249–261. doi:10.1016/j.tcb.2015.12.002
Blocking IDO1 Helps Shrink Bladder, Cervical Tumors (2018). Blocking Ido1 helps Shrink bladder, cervical tumors. Cancer Discov. 8 (1), Of3. doi:10.1158/2159-8290.cd-nb2017-167
Callahan, M. K., and Wolchok, J. D. (2013). At the bedside: CTLA-4- and PD-1-blocking antibodies in cancer immunotherapy. J. Leukoc. Biol. 94 (1), 41–53. doi:10.1189/jlb.1212631
Cao, D., Song, X., Che, L., Li, X., Pilo, M. G., Vidili, G., et al. (2017). Both de novo synthetized and exogenous fatty acids support the growth of hepatocellular carcinoma cells. Liver Int. 37 (1), 80–89. doi:10.1111/liv.13183
Chen, H., Deng, Q., Wang, W., Tao, H., and Gao, Y. (2020). Identification of an autophagy-related gene signature for survival prediction in patients with cervical cancer. J. Ovarian Res. 13 (1), 131. doi:10.1186/s13048-020-00730-8
Cheng, L., Yuan, M., Li, S., Lian, Z., Chen, J., Lin, W., et al. (2021). Identification of an IFN-β-associated gene signature for the prediction of overall survival among glioblastoma patients. Ann. Transl. Med. 9 (11), 925. doi:10.21037/atm-21-1986
Demkow, U. (2021). Neutrophil extracellular traps (NETs) in cancer invasion, evasion and metastasis. Cancers (Basel) 13 (17), 4495. doi:10.3390/cancers13174495
Ding, H., Fan, G. L., Yi, Y. X., Zhang, W., Xiong, X. X., and Mahgoub, O. K. (2020). Prognostic implications of immune-related genes' (IRGs) signature models in cervical cancer and endometrial cancer. Front. Genet. 11, 725. doi:10.3389/fgene.2020.00725
Ghasemi, F., Shafiee, M., Banikazemi, Z., Pourhanifeh, M. H., Khanbabaei, H., Shamshirian, A., et al. (2019). Curcumin inhibits NF-kB and Wnt/β-catenin pathways in cervical cancer cells. Pathol. Res. Pract. 215 (10), 152556. doi:10.1016/j.prp.2019.152556
Ghittoni, R., Accardi, R., Hasan, U., Gheit, T., Sylla, B., and Tommasino, M. (2010). The biological properties of E6 and E7 oncoproteins from human papillomaviruses. Virus Genes 40 (1), 1–13. doi:10.1007/s11262-009-0412-8
Gong, Y., Fan, Z., Luo, G., Yang, C., Huang, Q., Fan, K., et al. (2019). The role of necroptosis in cancer biology and therapy. Mol. Cancer 18 (1), 100. doi:10.1186/s12943-019-1029-8
Guan, X., Polesso, F., Wang, C., Sehrawat, A., Hawkins, R. M., Murray, S. E., et al. (2022). Androgen receptor activity in T cells limits checkpoint blockade efficacy. Nature 606 (7915), 791–796. doi:10.1038/s41586-022-04522-6
Hojo, T., Piotrowska-Tomala, K. K., Jonczyk, A. W., Lukasik, K., Jankowska, K., Okuda, K., et al. (2019). Receptor interacting protein kinases-dependent necroptosis as a new, potent mechanism for elimination of the endothelial cells during luteolysis in cow. Theriogenology 128, 193–200. doi:10.1016/j.theriogenology.2019.01.035
Jung, K. H., LoRusso, P., Burris, H., Gordon, M., Bang, Y. J., Hellmann, M. D., et al. (2019). Phase I study of the indoleamine 2, 3-dioxygenase 1 (Ido1) inhibitor navoximod (GDC-0919) administered with PD-L1 inhibitor (atezolizumab) in advanced solid tumors. Clin. Cancer Res. 25 (11), 3220–3228. doi:10.1158/1078-0432.ccr-18-2740
Ke, Z. B., Wu, Y. P., Huang, P., Hou, J., Chen, Y. H., Dong, R. N., et al. (2021). Identification of novel genes in testicular cancer microenvironment based on ESTIMATE algorithm-derived immune scores. J. Cell. Physiol. 236 (1), 706–713. doi:10.1002/jcp.29898
Lai, S., Jia, J., Cao, X., Zhou, P. K., and Gao, S. (2021). Molecular and cellular functions of the linker histone H1.2. Front. Cell Dev. Biol. 9, 773195. doi:10.3389/fcell.2021.773195
Lin, S. L., Wang, M., Cao, Q. Q., and Li, Q. (2020). Chromatin modified protein 4C (CHMP4C) facilitates the malignant development of cervical cancer cells. FEBS Open Bio 10 (7), 1295–1303. doi:10.1002/2211-5463.12880
Litwin, T. R., Irvin, S. R., Chornock, R. L., Sahasrabuddhe, V. V., Stanley, M., and Wentzensen, N. (2021). Infiltrating T-cell markers in cervical carcinogenesis: a systematic review and meta-analysis. Br. J. Cancer 124 (4), 831–841. doi:10.1038/s41416-020-01184-x
Mauricio, D., Zeybek, B., Tymon-Rosario, J., Harold, J., and Santin, A. D. (2021). Immunotherapy in cervical cancer. Curr. Oncol. Rep. 23 (6), 61. doi:10.1007/s11912-021-01052-8
Mishra, S. K., Stephenson, D. J., Chalfant, C. E., and Brown, R. E. (2019). Upregulation of human glycolipid transfer protein (GLTP) induces necroptosis in colon carcinoma cells. Biochim. Biophys. Acta. Mol. Cell Biol. Lipids 1864 (2), 158–167. doi:10.1016/j.bbalip.2018.11.002
Mohanty, S., Yadav, P., Lakshminarayanan, H., Sharma, P., Vivekanandhan, A., and Karunagaran, D. (2022). RETRA induces necroptosis in cervical cancer cells through RIPK1, RIPK3, MLKL and increased ROS production. Eur. J. Pharmacol. 920, 174840. doi:10.1016/j.ejphar.2022.174840
Nascimento, J., Mariot, C., Vianna, D. R. B., Kliemann, L. M., Chaves, P. S., Loda, M., et al. (2022). Fatty acid synthase as a potential new therapeutic target for cervical cancer. An. Acad. Bras. Cienc. 94 (2), e20210670. doi:10.1590/0001-3765202220210670
Odiase, O., Noah-Vermillion, L., Simone, B. A., and Aridgides, P. D. (2021). The incorporation of immunotherapy and targeted therapy into chemoradiation for cervical cancer: A focused review. Front. Oncol. 11, 663749. doi:10.3389/fonc.2021.663749
Qu, X., Shi, Z., Guo, J., Guo, C., Qiu, J., and Hua, K. (2021). Identification of a novel six-gene signature with potential prognostic and therapeutic value in cervical cancer. Cancer Med. 10 (19), 6881–6896. doi:10.1002/cam4.4054
Quarato, G., Guy, C. S., Grace, C. R., Llambi, F., Nourse, A., Rodriguez, D. A., et al. (2016). Sequential engagement of distinct MLKL phosphatidylinositol-binding sites executes necroptosis. Mol. Cell 61 (4), 589–601. doi:10.1016/j.molcel.2016.01.011
Ramírez-González, A., Manzo-Merino, J., Contreras-Ochoa, C. O., Bahena-Román, M., Aguilar-Villaseñor, J. M., Lagunas-Martínez, A., et al. (2021). Functional role of AKNA: A scoping review. Biomolecules 11 (11), 1709. doi:10.3390/biom11111709
Ruan, J., Mei, L., Zhu, Q., Shi, G., and Wang, H. (2015). Mixed lineage kinase domain-like protein is a prognostic biomarker for cervical squamous cell cancer. Int. J. Clin. Exp. Pathol. 8 (11), 15035–15038.
Schaer, D. A., Hirschhorn-Cymerman, D., and Wolchok, J. D. (2014). Targeting tumor-necrosis factor receptor pathways for tumor immunotherapy. J. Immunother. Cancer 2, 7. doi:10.1186/2051-1426-2-7
Schmidt, S. V., Seibert, S., Walch-Rückheim, B., Vicinus, B., Kamionka, E. M., Pahne-Zeppenfeld, J., et al. (2015). RIPK3 expression in cervical cancer cells is required for PolyIC-induced necroptosis, IL-1α release, and efficient paracrine dendritic cell activation. Oncotarget 6 (11), 8635–8647. doi:10.18632/oncotarget.3249
Small, W., Bacon, M. A., Bajaj, A., Chuang, L. T., Fisher, B. J., Harkenrider, M. M., et al. (2017). Cervical cancer: A global health crisis. Cancer 123 (13), 2404–2412. doi:10.1002/cncr.30667
Smola, S. (2016). RIPK3-a predictive marker for personalized immunotherapy? Oncoimmunology 5 (4), e1075695. doi:10.1080/2162402x.2015.1075695
Song, Z., Lin, Y., Ye, X., Feng, C., Lu, Y., Yang, G., et al. (2016). Expression of IL-1α and IL-6 is associated with progression and prognosis of human cervical cancer. Med. Sci. Monit. 22, 4475–4481. doi:10.12659/msm.898569
Sprooten, J., De Wijngaert, P., Vanmeerbeerk, I., Martin, S., Vangheluwe, P., Schlenner, S., et al. (2020). Necroptosis in immuno-oncology and cancer immunotherapy. Cells 9 (8), 1823. doi:10.3390/cells9081823
Su, Z., Yang, Z., Xu, Y., Chen, Y., and Yu, Q. (2015). Apoptosis, autophagy, necroptosis, and cancer metastasis. Mol. Cancer 14, 48. doi:10.1186/s12943-015-0321-5
Taylor, J. R., Fernandez, D. J., Thornton, S. M., Skeate, J. G., Lühen, K. P., Da Silva, D. M., et al. (2018). Heterotetrameric annexin A2/S100A10 (A2t) is essential for oncogenic human papillomavirus trafficking and capsid disassembly, and protects virions from lysosomal degradation. Sci. Rep. 8 (1), 11642. doi:10.1038/s41598-018-30051-2
Torre, L. A., Bray, F., Siegel, R. L., Ferlay, J., Lortet-Tieulent, J., and Jemal, A. (2015). Global cancer statistics, 2012. Ca. Cancer J. Clin. 65 (2), 87–108. doi:10.3322/caac.21262
Vu, M., Yu, J., Awolude, O. A., and Chuang, L. (2018). Cervical cancer worldwide. Curr. Probl. Cancer 42 (5), 457–465. doi:10.1016/j.currproblcancer.2018.06.003
Waardenberg, A. J., and Field, M. A. (2019). consensusDE: an R package for assessing consensus of multiple RNA-seq algorithms with RUV correction. PeerJ 7, e8206. doi:10.7717/peerj.8206
Wang, G., Sun, D., Li, W., and Xin, Y. (2020). AKNA is a potential prognostic biomarker in gastric cancer and function as a tumor suppressor by modulating EMT-related pathways. Biomed. Res. Int. 2020, 6726759. doi:10.1155/2020/6726759
Wu, Z., Huang, X., Cai, M., Huang, P., and Guan, Z. (2022). Novel necroptosis-related gene signature for predicting the prognosis of pancreatic adenocarcinoma. Aging (Albany NY) 14 (2), 869–891. doi:10.18632/aging.203846
Yatim, N., Jusforgues-Saklani, H., Orozco, S., Schulz, O., Barreira da Silva, R., Reis e Sousa, C., et al. (2015). RIPK1 and NF-κB signaling in dying cells determines cross-priming of CD8⁺ T cells. Science 350 (6258), 328–334. doi:10.1126/science.aad0395
Keywords: cervical cancer, necroptosis, prognosis, immune microenvironment, TCGA
Citation: Xing X, Tian Y and Jin X (2023) Immune infiltration and a necroptosis-related gene signature for predicting the prognosis of patients with cervical cancer. Front. Genet. 13:1061107. doi: 10.3389/fgene.2022.1061107
Received: 04 October 2022; Accepted: 01 December 2022;
Published: 06 January 2023.
Edited by:
Wei Xu, Shanghai Changzheng Hospital, ChinaCopyright © 2023 Xing, Tian and Jin. This is an open-access article distributed under the terms of the Creative Commons Attribution License (CC BY). The use, distribution or reproduction in other forums is permitted, provided the original author(s) and the copyright owner(s) are credited and that the original publication in this journal is cited, in accordance with accepted academic practice. No use, distribution or reproduction is permitted which does not comply with these terms.
*Correspondence: Xuan Jin, amlubGFvc2hpMDJAMTYzLmNvbQ==
†These authors have contributed equally to this work