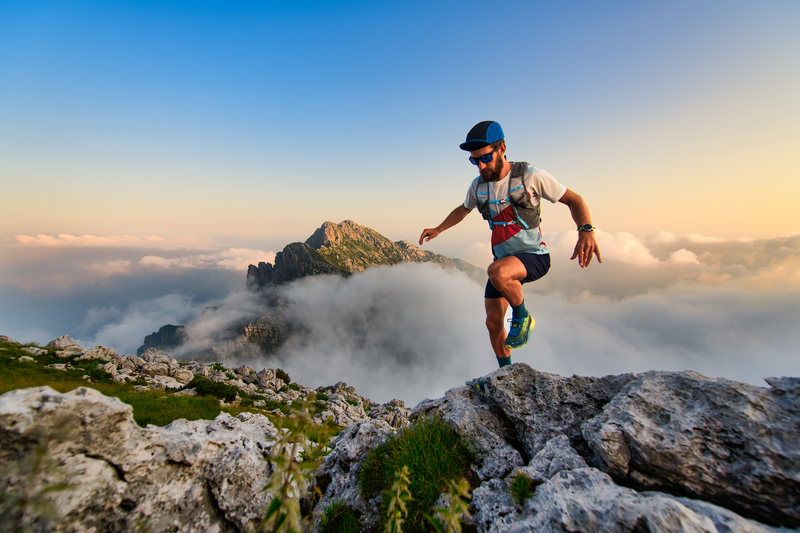
94% of researchers rate our articles as excellent or good
Learn more about the work of our research integrity team to safeguard the quality of each article we publish.
Find out more
MINI REVIEW article
Front. Genet. , 02 March 2023
Sec. Genomics of Plants and the Phytoecosystem
Volume 13 - 2022 | https://doi.org/10.3389/fgene.2022.1060304
This article is part of the Research Topic Advanced Genetics and Genomics and Climate Change Resilient Crops: Roots, Tubers, and Cereals View all 4 articles
Nitrogen (N) fertilizer is one of the major inputs for grain crops including barley and its usage is increasing globally. However, N use efficiency (NUE) is low in cereal crops, leading to higher production costs, unfulfilled grain yield potential and environmental hazards. N uptake is initiated from plant root tips but a very limited number of studies have been conducted on roots relevant to NUE specifically. In this review, we used barley, the fourth most important cereal crop, as the primary study plant to investigate this topic. We first highlighted the recent progress and study gaps in genetic analysis results, primarily, the genome-wide association study (GWAS) regarding both biological and statistical considerations. In addition, different factors contributing to NUE are discussed in terms of root morphological and anatomical traits, as well as physiological mechanisms such as N transporter activities and hormonal regulation.
N fertilizer is the primary resource to supply soil nutrients to conserve cereal crop production (Raun & Johnson, 1999; Sadras et al., 2016). N fertilization input is increasing globally while the global average for N recovery rate in cereal crops is only 33% (Raun & Johnson, 1999). Un-utilized N fertilizers are likely cause environmental hazards, such as eutrophication, soil acidification, and air pollution (Raun & Johnson, 1999; Tang et al., 2013; Skiba & Rees, 2014). NUE itself has barely been considered in most modern breeding programs and a continuous selection of high-yielding genotypes only under sufficient N environments has led to the reduced variation of NUE alleles in modern varieties (Yang et al., 2014; Garnett et al., 2015).
Barley (Hordeum vulgare) is an important cereal and ranks the fourth most grown crop worldwide (Arendt & Zannini, 2013). Barley is one of the most ancient cereal crops used by hunters and gatherers, and the history of its cultivation can be traced back to more than 20,000 years ago (Willcox, 2013; Pourkheirandish et al., 2015). In addition to its importance as a crop, the diploid nature of barley, the availability of multiple reference quality genomes, reference transcriptome, pangenome sequence, and its close genetic relationship with wheat, make it an ideal model for cereal crops (von Bothmer et al., 2003). Once a molecular mechanism relevant to the expression of a specific trait associated with NUE is discovered, it can be expected to offer opportunities for crop improvement both in barley and its close relatives.
Genome-wide association study (GWAS) is often referred as a “hypothesis-generating” analysis and could serve as a first step to gaining novel understanding of mechanisms of NUE by studying genetic variations of the entire genome instead of only targeting specified genes of interest (Ali et al., 2021; Özdemir et al., 2021). Although numerous above-ground crop traits have been determined to be closely correlated with NUE as well as identifying associated genes conferring high NUE in pre-breeding research (Yang et al., 2014; Han et al., 2016; Karunarathne et al., 2020a), a great study gap is present in relevant below-ground activities due to difficulties in phenotyping root traits under field conditions and dynamics in soil N status (Garnett et al., 2009; Plett et al., 2020). This mini-review will report the recent progress in identifying NUE-related genetic variants and phenotypic traits in barley as well as discuss the limitations and knowledge gap.
GWAS is a powerful tool to examine the contributions of genetic variants/single nucleotide polymorphisms (SNPs) to the traits of interests (Bush, 2019; Alqudah et al., 2020; Alomari et al., 2021). NUE is a polygenic trait which has not had its molecular mechanisms fully revealed, resulting in difficulties to target genes of interest in pre-breeding research. The rapid progress in high-throughput genomic technologies such as next-generation sequencing (NGS) has allowed more accurate reads for whole genome sequencing data, subsequently facilitating the precision of results generated from GWAS (Wu et al., 2017; Yin et al., 2019). Non-etheless, there is only one published research on examining NUE in barley to date (Karunarathne et al., 2020a). Most of the genes associated with NUE in barley were reported by quantitative trait loci (QTL) analysis without being well correlated with phenotypic traits. Although, only root length and dry weight have been examined for NUE in barley by GWAS (Supplementary Table S1), it has provided reliable and important genetic information to associate root traits and NUE. For example, HORVU3Hr1G095880 is the candidate gene associated with root dry weight and functionally annotated as NAC domain protein. The role of NAC domain protein on NUE has been well demonstrated by overexpression it in wheat and the improvement in several agronomic traits have been shown in both low and high N levels (He et al., 2015). Further investigation into identifying QTLs associated with various root traits would be valuable to discover novel mechanisms of NUE.
Several limitations of GWAS impede the implication of GWAS results for improving NUE. One of the major issues is that the identified variants are often unstable across experiments (Karunarathne et al., 2020b). There is a demand to increase statistical power of GWAS to generate replicable variants and the most direct approach is to incorporate a bigger sample size into the study. Another issue is that the detected variants can be false positives due to the complex population structures and cryptic relatedness (Wright et al., 2012; Yang et al., 2021). To date, fitting the population structure and/or kinship matrix as covariate to adjust the mixed linear model and assessing linkage disequilibrium (LD) block to project candidate genes are the most utilized methods to pinpoint the relevant variants (Kovi et al., 2015; Xu et al., 2017; Karunarathne et al., 2020a). Nonetheless, false negatives are caused at the same time when attempting to control those false positives.
Computing innovation has allowed a more powerful GWAS by increasing the statistical power as well as optimizing the detection of accurate variants. An early GWAS model, described as a univariate model, can only analyze a single phenotype for its association with variants (Fernandes et al., 2021). This model poses limitation of taking account of relatedness among phenotypic traits while most loci are pleiotropic. For instance, NUE is under the regulation of multiple genes, and each can affect several phenotypic traits associated with NUE so that a univariate GWAS could mask some information of the phenotypic and genetic relatedness. Subsequently, the multivariate model of GWAS was developed to improve the statistical power for detecting variants controlling multiple phenotypic traits and reducing false discoveries (Korte et al., 2012). Both models would also compromise partial true positives due to the confounding effect among the variants, population structure and kinship since they principally still utilize the same mixed linear model to adjust population structure and LD (Liu et al., 2016).
Although a few advancements have been achieved, the optimization of both statistical power and computational efficiency when controlling false discoveries was the primary burden. For instance, fixed and random model Circulating Probability Unification (FarmCPU) was developed to resolve the confounding issue owing to population structure and kinship by dissecting the model into fixed effect model and random effect model to separate population structure and kinship, then running the two models iteratively (Liu et al., 2016). Thereafter FarmCPU boosted the statistical power while the computing speed was the primary issue to be tackled. Bayesian-information and Linkage-disequilibrium Iteratively Nested Keyway (BLINK) is the new method built based on FarmCPU. BLINK utilizes Bayesian Information Criteria to substitute the computationally demanding random effect model into the fixed effect model and has been tested with its precision and efficiency with both simulated and real datasets (Huang et al., 2018). BLINK model has been employed in several research investigating plant performance such as rice, wheat and barley (Kumar et al., 2021; Zhong et al., 2021; Clare et al., 2022). Moreover, BLINK GWAS model has also be used to examine maize root performance in different N levels (Fu et al., 2022). Accordingly, BLINK would be the most updated GWAS model for ascertaining and providing context to the genetic-phenotypic relationship for plant underground performance in various N environments.
Meta-analysis of GWAS (Meta-GWAS) is another model worthen attention. It is to analyze the quantitative combination of summary statistics from independent experiments (Joukhadar and Daetwyler, 2022). Subsequently, it can increase sample size by analyzing multiple GWAS results, which boost statistical power (Lin and Zeng, 2010). Moreover, the algorithm of Meta-GWAS has been developed to effectively detect variants associated with multiple traits and environments (Bolormaa et al., 2014; Singh et al., 2021). NUE is affected by multiple traits and N availability is varied greatly from different environments due to its mobile nature. Therefore, meta-analysis can be useful for studying NUE and detecting more associated variants by analyzing different combination of associated traits and examining several N levels at the same time. Another advantage of meta-analysis is its capability to incorporate data from various genetic backgrounds (Tam et al., 2019). It could potentially bring extra benefits to increase the magnitude of association of the determined variants when significant association between variants and population structure presents (Tam et al., 2019). This provides insight to interpret the results with sound consideration of population structure and LD.
The accuracy of GWAS counts upon both high density markers and precise phenotyping. With the advancement in genome sequencing and availability of reference genome, the high-density genotyping is no longer a limit. Our understanding on the plant genome has been more advanced than the below-ground phenome in regards of barley NUE (Yang et al., 2014; Karunarathne et al., 2020a). Therefore, the information on the relationship between root traits and NUE could be ambiguous and lead to misinterpretation of data. This becomes an impediment for pre-breeding research of NUE even if molecular tools are well-developed.
Growing conditions have enormous impacts on root growth and development while there are different growth systems in pre-breeding research (Zhu et al., 2005; Liao et al., 2006; Karunarathne et al., 2020a). The results concluded from one growth system are not necessarily replicable in another one. For instance, a comparative study on maize has been conducted in three systems, which are paper roll, hydroponics, and vermiculite culture. The results demonstrated that only 13% of QTLs were repeatably identifiable across systems due to strong genetic-environmental effects (Liu et al., 2017). Moreover, this study also determined a relatively stronger correlation between root traits and N uptake efficiency in hydroponics and vermiculite systems compared to the paper roll system, suggesting they are the more suitable growth systems to examine NUE in maize (Liu et al., 2017).
The development of high-throughput phenotyping technologies is paramount for root phenotyping since the sample size for detecting QTLs is generally large and processing those samples in conventional ways can be laborious and time-consuming. The most common method is to collect root samples by washing them from soils and then scan clean samples using scanning software such as WinRhizo and RhizoVision (Kamoshita et al., 2019; Seethepalli et al., 2021). One of the difficulties during this process is efficiently getting the root out from fields. A method described by Teramoto et al. (2019) is to place a steel cylinder surrounding each plant then the whole plant can be pushed back vertically by a backhoe. It is demonstrated to greatly reduce labor work. Non-etheless, root washing processes are still challenging regarding labor intensity and sample integrity. The development of X-ray computed tomography (CT) permits the visualization of root in the soils so that root traits data could be collected non-destructively, which not only reduces the labor requirement but also guarantee the quality of data (Teramoto et al., 2020). This technology has been utilized in various crops, such as rice, wheat, and maize, for visualizing root systems in soils (Mairhofer et al., 2012; Teramoto et al., 2020). However, no barley NUE research so far has utilized this platform to examine root traits in soils.
It is a widely accepted concept in cereals that larger root biomass can enhance N absorption (Anbessa & Juskiw, 2012). Root weight density refers to total root weight per unit of soil volume, which has been found to be significantly correlated with NUE of wheat (Liu et al., 2018). However, it does not always translate into better above-ground performance. First, the trade-off between above- and below-ground carbon (C) storage could limit the yield capacity when large amounts of energy are invested into root biomass. Several studies have supported this trade-off effect in maize and barley when N is insufficient (Gallais and Coque, 2005; Lynch, 2013; Tolley and Mohammadi, 2020). In contrast, research on wheat has indicated that larger root biomass is associated with higher yield and less leached N in high N conditions (Ehdaie & Waines, 2008; Tolley & Mohammadi, 2020). It implies that the yields are not being limited by the higher metabolic costs of roots since nutrient resources are sufficient to supply both above- and below-ground plant growth. To connect with above-ground performance, root:shoot (R:S) ratio is often used to explain the energy allocation. Research has suggested that R:S are altered by different N levels as the strategy to optimize the relative growth rate (Ågren & Franklin, 2003), but it has not been shown if R:S has a significantly linear association with NUE.
Root system architecture (RSA) is a collection of root features to describe the temporospatial distribution of roots in the soil. Intensive investigations have been done for the root length and it is positively related to N capture (Karunarathne et al., 2020a). With the same root length, it is desirable to obtain other RSA traits for optimizing the interception between root and soil while minimizing metabolic costs. One of the strategies could be targeting the specific root zones for nutrient uptake (Kitomi et al., 2018). The combination of the narrow root angle and deep roots, or the wide root angle and shallow roots have been suggested to enable reducing metabolic costs while maximizing soil nutrient exploitation in deeper and shallow soil profiles, respectively (Lynch, 2013). However, no significant correlation between root angle and barley grain yield has been determined so far, demanding more studies to map the correlation between root angle and NUE (Robinson et al., 2018). For a further step, increased lateral root number and root hairs, subsequently root surface area, have been found when plants are grown in N-deficient conditions (Marschner 1995; Gilroy & Jones, 2000). The debate has been around if such responses would contribute to N uptake when sufficient N is supplied to plants, specifically in the soil with a low leaching potential where N is abundant and the increase in root surface area is not required (Garnett et al., 2009). Meanwhile, it has also been reported that wheat with a higher level of root branching and lateral root number showed greater N uptake in sandy soils with an ample N level (Liao et al., 2006). Accordingly, the N availability and the leaching potential of soils are vital to be considered to conclude if increased root surface area is of importance for increasing N uptake.
Root cortical senescence (RCS) describes the death of root cortical cells, which can be promoted by severe N scarcity (Gillespie & Deacon, 1988). On the one hand, this could result in unhealthy plant tissues and restricted plant growth (Schneider et al., 2017a). On the other hand, RCS might demonstrate potential compensation for a plant’s survival in harsh environments. Initially, a reduction in nutrient partitioning, and efficiencies of radial water and nutrient transport in barley root have been determined to be correlated with RCS under N-deficient conditions (Schneider et al., 2017a). Simultaneously, barley root respiration also decreases, which can infer as a positive effect of RCS, saving the energy cost of roots (Schneider et al., 2017b). Also, RCS occurs in older tissues, located in the upper part of soil profile where nutrients might be depleted (Schneider et al., 2017a; Schneider et al., 2017b). Hence, it can be beneficial for young tissue growth when coming across nutrient-deficient situations since energy can be reinvested into effective plant parts by getting rid of older tissues (Schneider et al., 2017b).
Cereals have a fibrous root system that can be mainly dissected into two parts, seminal and nodal roots. Nevertheless, much less attention has been paid to nodal roots for their role in NUE until recently. A greater root diameter, meta-xylem area and more meta-xylem vessels have been examined in nodal roots compared to seminal roots in mature barley plants, which also contributes to a higher level of nitrate (NO3−) uptake (Liu B. et al., 2020). Hence, nodal roots may serve as a selection target in pre-breeding research for better barley NUE. Nevertheless, no similar analysis has been done on barley from the seedling stage. It might be since nodal roots have been considered more important for water uptake at lateral plant stages (Hochholdinger et al., 2018), and this concept has been applied to N uptake as well. Meanwhile, the work done by Schneider et al. (2020) showed barley nodal roots demonstrated equivalent importance of water uptake as seminal roots since the seedling stage. Given that N flux into plant roots with water via mass flow, this study enlightens the possibility that nodal roots might also be important in N uptake from an early stage.
N molecules pass through the plasma membrane of root cells, initiating the N uptake process, mediated by several N transporters (Tsay & Hsu, 2010; Hove et al., 2015; Han et al., 2016; Figure 1). Nitrate (NO3−) is widely considered as the most common form absorbed by crops since nitrification is continuously taking place in the soil under the effect of soil bacteria, converting ammonium (NH4+) into NO3−. Therefore, most research has focused on NO3−. After NO3− is taken up by roots, it is reduced into nitrite (NO2−) by NO3− reductase (NR), then further reduced into NH4+ by NO2− reductase (NiR). Subsequently, N in the NH4+ form will undergo several enzymatic reactions and be converted into organic forms, predominantly amino acids (Tsay & Hsu, 2010). To illustrate, glutamate and glutamine are synthesized in the plastid, which are the two primary sources of the amine group for other amino acids (Tsay & Hsu, 2010; Han et al., 2016). This process is primarily regulated by two enzymes, which are glutamine synthetase (GS) and glutamate synthetase (GOGAT) (Tsay & Hsu, 2010; Han et al., 2016). Moreover, it has been determined that NO3− has an important role in regulating lateral root growth (Zhang & Forde, 2000; Liu B. et al., 2020).
FIGURE 1. N transport and assimilation in the barley plant at a cellular level. In the transport process, different forms of N including nitrate, ammonium and urea are transported via different classes of proteins in different concentrations. In the assimilation process, the conversion of nitrate and urea into ammonium is happened first, followed by GS-GOGAT cycle to convert ammonium into glutamine (Gln) and glutamate (Glu). Created with BioRender.com.
NH4+ has often been overlooked due to its lower availability in soils and adverse effects on several domesticated plants including barley (Britto & Kronzucker, 2002; Liu et al., 2013; Chen et al., 2020; Naz et al., 2021). Interestingly, wheat cultivars, Yitpi and Wyalkatchem, have been determined to prefer NH4+ over NO3− (O’Sullivan et al., 2016). Moreover, NH4+ can become more significant in certain environments including low N conditions, and soil environments unfavored by nitrification. Initially, crops will preferentially absorb NH4+ in nutrient-depleted soils, since unlike NO3− it does not need to undergo energy-dependent transformation once inside the root (Boudsocq et al., 2012). Further, soil acidification and insufficient soil organic matter could inhibit bacterial processes, such as nitrification. Subsequently, NH4+ would become more abundant since less NH4+ would be converted into NO3−. Accordingly, selecting barley genotypes for better use of NH4+ could improve its NUE performance in field conditions featured by N deficiency, acidity, and low organic matter contents. Nevertheless, differing from NO3− uptake, a high accumulation of NH4+ is toxic to barley. Therefore, a more effective use of NH4+ additionally requires plants to have improved detoxifying mechanisms. It has been shown in barley that NH4+ toxicity is caused by a great level of NH4+ efflux across the cell membrane which consumes a huge amount of metabolic energy (Britto et al., 2001). Hence, more efficient utilization of NH4+ into amino acids via GS-GOGAT cycle mentioned above could potentially work as a detoxifying process by reducing NH4+ accumulation and efflux while also improving overall N utilization into nutrients.
Urea is an organic form of N and is intensively used as N fertilizer in agricultural fields. Urea is hydrolyzed into NH3 and ammonium carbamate (H2NCOONH4), and this process is catalyzed by urease (Figure 1). Although only a limited number of studies have yielded the role of urea itself on plant NUE since the major opinion is that urea uptake by plants is not significant in the past, today’s studies have established several classes of proteins capable to absorb and transport urea (Witte, 2011). For instance, urea transporter (DUR3) and aquaporins (AQP) have been found to act in active and passive urea transport of several plants such as Arabidopsis, maize and barley (Liu et al., 2003; Zanin et al., 2014; Hove et al., 2015). It might offer crops the opportunity to absorb more N with more efficient urea transport while also reducing urea losses as NH3 since more urea uptake occurs before being converted into gases.
Cytokinin (CK) is a plant hormone responsible for plant cell division, which has been suggested as a key substance communicating N availability with plants and coordinating the metabolism from roots to shoots (Lu et al., 1990). The responses of barley relevant to NUE in accordance with CK varied between different plant organs (Figure 2). Two main enzymes, namely phosphate-isopentenyl transferase (IPT) and cytokinin oxidase (CXK) are responsible for CK synthesis and degradation, respectively (del Mar Rubio-Wilhelmi et al., 2011; Ramireddy et al., 2018). Through transgenic approaches, the overexpression of IPT has been done in wheat, engendering a higher CK content with delayed leaf senescence and NR activity, which are positively associated with N remobilization and utilization process, respectively (Sýkorová et al., 2008; Masclaux-Daubresse et al., 2010). However, no significant increase in grain yield was observed (Sýkorová et al., 2008). Meanwhile, CXK has been overexpressed in barley, leading to the breakdown of CK (Holubová et al., 2018). It leads to a larger root system without a significant yield sacrifice (Holubová et al., 2018). Interestingly, at the same time, the thousand-grain weight was shown to be reduced while increases in tiller and grain numbers and the higher accumulation of nutrients were recorded (Holubová et al., 2018). Accordingly, grain productivity is under sophisticated control via CK as a signal between roots and above-ground plant, and more investigation into the molecular level would merit future research on barley NUE.
FIGURE 2. The regulatory role of cytokinin (CK) in the barley plant. The degradation and biosynthesis of CK is majorly controlled by two enzymes, CK oxidase and phosphate-isopentenyl transferase (IPT). The increase in CK level will lead to a series changes of above-ground plant performance associated with N metabolism. The upwards arrows and downwards arrows indicate the increase and decrease in the corresponding traits, respectively. Created with BioRender.com.
In conclusion, it must be acknowledged that there is limited amount of research has examined NUE in barley, especially considering the below-ground performance. First, several classes of candidate NUE genes have been identified in barley while only a few have been proved to be associated with root traits such as NAC domain protein gene. Moreover, most identified root-related QTLs are not consistent across experiments due to statistical limitations, and biological knowledge gaps including phenotyping platforms and the understanding of relevant phenotypes. Given the advances in plant genomic areas such as whole-genome sequencing technologies and the development of meta-analysis algorithm of GWAS, a large gap still exists in the association between plant phenotypes and N levels. At morphological and anatomical levels, future studies should not be limited to root biomass and length but pay more attention to RSA traits and RCS. Moreover, more research into nodal roots is also highly demanded. Regarding physiological aspects, different N forms are absorbed via different transporters. Most NUE research focuses on investigating the effects of NO3− transporters. Nevertheless, a more effective uptake of NH4+ and urea can be potentially desirable for barley plants adapting to a wider range of environments and reducing N losses from the system. Lastly, hormonal regulation is a sophisticated mechanism to be examined for communicating roots with above-ground plant responses.
CH performed the literature search and drafted the manuscript. CB, DM, and MP provided guidance on the literature search and commented on the manuscript writing. All authors revised the paper and approved the final version to be published.
We acknowledge the staff members from InterGrain company for providing the expertise assistance of breeding knowledge and field experience. CH received Graduate Research Scholarship from the University of Melbourne.
Author DM is employed by InterGrain.
The remaining authors declare that the research was conducted in the absence of any commercial or financial relationships that could be construed as a potential conflict of interest.
All claims expressed in this article are solely those of the authors and do not necessarily represent those of their affiliated organizations, or those of the publisher, the editors and the reviewers. Any product that may be evaluated in this article, or claim that may be made by its manufacturer, is not guaranteed or endorsed by the publisher.
The Supplementary Material for this article can be found online at: https://www.frontiersin.org/articles/10.3389/fgene.2022.1060304/full#supplementary-material
Ågren, G. I., and Franklin, O. (2003). Root : Shoot ratios, optimization and nitrogen productivity. Ann. Bot. 92 (6), 795–800. doi:10.1093/AOB/MCG203
Ali, F., Nadeem, M. A., Habyarimana, E., Altaf, M. T., Barut, M., Kurt, C., et al. (2021). Identification of genetic basis associated with agronomic traits in a global safflower panel using genome-wide association study. Turkish J. Agric. For. 45 (6), 834–849. doi:10.3906/tar-2105-55
Alomari, D. Z., Alqudah, A. M., Pillen, K., von Wirén, N., and Röder, M. S. (2021). Toward identification of a putative candidate gene for nutrient mineral accumulation in wheat grains for human nutrition purposes. J. Exp. Bot. 72 (18), 6305–6318. doi:10.1093/jxb/erab297
Alqudah, A. M., Sallam, A., Stephen Baenziger, P., and Börner, A. (2020). Gwas: Fast-forwarding gene identification and characterization in temperate cereals: Lessons from barley – a review. J. Adv. Res. 22, 119–135. doi:10.1016/j.jare.2019.10.013
Anbessa, Y., and Juskiw, P. (2012). Nitrogen fertilizer rate and cultivar interaction effects on nitrogen recovery, utilization efficiency, and agronomic performance of spring barley. ISRN Agron. 2012, 1–8. doi:10.5402/2012/531647
Arendt, E. K., and Zannini, E. (2013). “Barley,” in Cereal grains for the food and beverage industries (United Kingdom: Woodhead Publishing), 155–201e. doi:10.1533/9780857098924.155
Bolormaa, S., Pryce, J. E., Reverter, A., Zhang, Y., Barendse, W., Kemper, K., et al. (2014). A multi-trait, meta-analysis for detecting pleiotropic polymorphisms for stature, fatness and reproduction in beef cattle. PLOS Genet. 10 (3), e1004198. doi:10.1371/JOURNAL.PGEN.1004198
Boudsocq, S., Niboyet, A., Lata, J. C., Raynaud, X., Loeuille, N., Mathieu, J., et al. (2012). Plant preference for ammonium versus nitrate: A neglected determinant of ecosystem functioning? Am. Nat. 180 (1), 60–69. doi:10.1086/665997
Britto, D. T., and Kronzucker, H. J. (2002). NH4 + toxicity in higher plants: A critical review. J. Plant Physiology 159, 567–584. doi:10.1078/0176-1617-0774
Britto, D. T., Yaeesh Siddiqi, M., M Glass, A. D., and Kronzucker, H. J. (2001). Futile transmembrane NH4+ cycling: A cellular hypothesis to explain ammonium toxicity in plants. Proc. Natl. Acad. Sci. 98 (7), 4255–4258. doi:10.1073/pnas.061034698
Bush, W. S. (2019). Genome-wide association studies. Encycl. Bioinforma. Comput. Biol. ABC Bioinforma. 1–3, 235–241. doi:10.1016/B978-0-12-809633-8.20232-X
Cai, S., and Zhang, G. (2016). Exploration and utilization of aluminum-tolerant barley germplasm. Exploration, identification and utilization of barley germplasm, 181–208. doi:10.1016/B978-0-12-802922-0.00007-8
Chen, H., Jia, Y., Xu, H., Wang, Y., Zhou, Y., Huang, Z., et al. (2020). Ammonium nutrition inhibits plant growth and nitrogen uptake in citrus seedlings. Scientia Horticulturae 272, 109526. doi:10.1016/J.SCIENTA.2020.109526
Clare, S. J., Duellman, K. M., Richards, J. K., Poudel, R. S., Merrick, L. F., Friesen, T. L., et al. (2022). Association mapping reveals a reciprocal virulence/avirulence locus within diverse US Pyrenophora teres f. maculata isolates. BMC Genomics 23 (1). doi:10.1186/S12864-022-08529-1
Coulter, M., Entizne, J. C., Guo, W., Bayer, M., Wonneberger, R., Milne, L., et al. (2022). BaRTv2: A highly resolved barley reference transcriptome for accurate transcript-specific RNA-seq quantification. Plant J. 111 (4), 1183–1202. doi:10.1111/TPJ.15871
del Mar Rubio-Wilhelmi, M., Sanchez-Rodriguez, E., Angel Rosales, M., Blasco, na, Jose Rios, J., Romero, L., et al. (2022). Cytokinin-Dependent improvement in transgenic P SARK : IPT Tobacco under nitrogen deficiency. J. Agric. Food Chem. 59, 10491–10495. doi:10.1021/jf202604k
Ehdaie, B., and Waines, J. (2008). “Larger root system increases water-nitrogen uptake and grain yield in bread wheat,” in The 11th international wheat genetics symposium proceedings. Editors R. Appels, R. Eastwood, E. Lagudah, P. Langridge, M. Mackay, L. Mclntyreet al. (Australia: Sydney University Press).
Fernandes, S. B., Zhang, K. S., Jamann, T. M., Lipka, A. E., et al. (2021). How well can multivariate and univariate GWAS distinguish between true and spurious pleiotropy?. Front. Genet 11. doi:10.3389/fgene.2020.602526
Fu, Y., Liu, J., Xia, Z., Wang, Q., Zhang, S., Zhang, G., et al. (2022). Genome-wide association studies of maize seedling root traits under different nitrogen levels. Plants 11 (11). doi:10.3390/plants11111417
Gallais, Y., and Coque, M. (2005). Genetic variation and selection for nitrogen use efficiency in maize: A synthesis. Maydica 50, 531–547.
Garnett, T., Conn, V., and Kaiser, B. N. (2009). Root based approaches to improving nitrogen use efficiency in plant. Plant Cell Environ. 32 (9), 1272–1283. doi:10.1111/j.1365-3040.2009.02011.x
Garnett, T., Plett, D., Heuer, S., and Okamoto, M. (2015). Genetic approaches to enhancing nitrogen-use efficiency (NUE) in cereals: Challenges and future directions. Funct. Plant Biol. 42 (10), 921–941. doi:10.1071/FP15025
Gillespie, I. M. M., and Deacon, J. W. (1988). Effects of mineral nutrients on senescence of the cortex of wheat roots and root pieces. Soil Biol. Biochem. 20 (4), 525–531. doi:10.1016/0038-0717(88)90068-5
Gilroy, S., and Jones, D. L. (2000). Through form to function: Root hair development and nutrient uptake. Trends Plant Sci. 5 (2), 56–60. doi:10.1016/S1360-1385(99)01551-4
Han, M., Wong, J., Su, T., Beatty, P. H., and Good, A. G. (2016). Identification of nitrogen use efficiency genes in barley: Searching for QTLs controlling complex physiological traits. Front. Plant Sci. 7 (October 2016). doi:10.3389/fpls.2016.01587
He, X., Qu, B., Li, W., Zhao, X., Teng, W., and Ma, W. (2015). The nitrate-inducible NAC transcription factor TaNAC2-5A controls nitrate response and increases wheat yield. Plant Physiol. 169 (3), 1991–2005. doi:10.1104/pp.15.00568
Hochholdinger, F., Yu, P., and Marcon, C. (2018). Genetic control of root system development in maize. Trends Plant Sci. 23 (1), 79–88. doi:10.1016/J.TPLANTS.2017.10.004
Holubová, K., Hensel, G., Vojta, P., Tarkowski, P., Bergougnoux, V., and Galuszka, P. (2018). Modification of barley plant productivity through regulation of cytokinin content by reverse-genetics approaches. Front. Plant Sci. 9, 1676. doi:10.3389/fpls.2018.01676
Hove, R. M., Ziemann, M., and Bhave, M. (2015). Identification and expression analysis of the barley (hordeum vulgare L.) aquaporin gene family. PLoS ONE 10 (6), e0128025. doi:10.1371/journal.pone.0128025
Huang, M., Liu, X., Zhou, Y., Summers, R. M., and Zhang, Z. (2019). BLINK: A package for the next level of genome-wide association studies with both individuals and markers in the millions. GigaScience 8 (2), 1–12. doi:10.1093/GIGASCIENCE/GIY154
Jayakodi, M., Padmarasu, S., Haberer, G., Bonthala, V. S., Gundlach, H., Monat, C., et al. (2020). The barley pan-genome reveals the hidden legacy of mutation breeding. Nature 588 (7837), 284–289. doi:10.1038/s41586-020-2947-8
Joukhadar, R., and Daetwyler, H. D. (2022). “The barley pan-genome reveals the hidden legacy of mutation breeding,” in Methods in Molecular Biology. (Humana Press Inc.) Vol. 2481, 173–183. doi:10.1007/978-1-0716-2237-7_11
Kamoshita, A., Deshmukh, V., and Amezquita, N. (2019). User manual for line core sampler and WinRhizo for root analysis SATREPS development and adoption of Latin American low-input rice production system through genetic improvement and advanced field-management. Colombia: science and technology research partnership for sustainable development program.
Karunarathne, S. D., Han, Y., Zhang, X.-Q., and Li, C. (2020b). Advances in understanding the molecular mechanisms and potential genetic improvement for nitrogen use efficiency in barley. Agronomy 10 (5), 662. doi:10.3390/agronomy10050662
Karunarathne, S. D., Han, Y., Zhang, X. Q., Zhou, G., Hill, C. B., Chen, K., et al. (2020a). Genome-wide association study and identification of candidate genes for nitrogen use efficiency in barley (hordeum vulgare L.). Front. Plant Sci. 11, 571912. doi:10.3389/fpls.2020.571912
Kitomi, Y., Itoh, J. I., and Uga, Y. (2018). Genetic mechanisms involved in the formation of root system architecture. Rice Genomics, Genetics and Breeding, 241–274. doi:10.1007/978-981-10-7461-5_14/COVER
Korte, A., Vilhjálmsson, B. J., Segura, V., Platt, A., Long, Q., and Nordborg, M. (2018). A mixed-model approach for genome-wide association studies of correlated traits in structured populations. Nat. Genet. 44 (9), 1066–1071. doi:10.1038/ng.2376
Kovi, M. R., Fjellheim, S., Sandve, S. R., Larsen, A., Rudi, H., Asp, T., et al. (2015). Population structure, genetic variation, and linkage disequilibrium in perennial ryegrass populations divergently selected for freezing tolerance. Front. Plant Sci. 6 (November). doi:10.3389/fpls.2015.00929
Kumar, D., Sharma, S., Sharma, R., Pundir, S., Singh, V. K., Chaturvedi, D., et al. (2021). Genome-wide association study in hexaploid wheat identifies novel genomic regions associated with resistance to root lesion nematode (Pratylenchus thornei). Scientific Reports 11 (1), 1–14. doi:10.1038/s41598-021-80996-0
Liao, M., Palta, J. A., and Fillery, I. R. P. (2006). Root characteristics of vigorous wheat improve early nitrogen uptake. Aust. J. Agric. Res. 57 (10), 1097–1107. doi:10.1071/AR05439
Lin, D., and Zeng, D. (2010). Meta-analysis of genome-wide association studies: No efficiency gain in using individual participant data. Genet. Epidemiol. 34 (1), 60–66. doi:10.1002/GEPI.20435
Liu, B., Wu, J., Yang, S., Schiefelbein, J., and Gan, Y. (2020). Nitrate regulation of lateral root and root hair development in plants. J. Exp. Bot. 71 (15), 4405–4414. doi:10.1093/jxb/erz536
Liu, L. H., Ludewig, U., Frommer, W. B., and von Wirén, N. (2003). AtDUR3 encodes a new type of high-affinity urea/H+ symporter in arabidopsis. Plant Cell 15 (3), 790–800. doi:10.1105/tpc.007120
Liu, W., Wang, J., Wang, C., Ma, G., Wei, Q., Lu, H., et al. (2018). Root growth, water and nitrogen use efficiencies in winter wheat under different irrigation and nitrogen regimes in north China plain. Front. Plant Sci. 871, 1798. doi:10.3389/fpls.2018.01798
Liu, X., Huang, M., Fan, B., Buckler, E. S., and Zhang, Z. (2016). Iterative usage of fixed and random effect models for powerful and efficient genome-wide association studies. PLoS Genet. 12 (2). doi:10.1371/journal.pgen.1005767
Liu, Y., Lai, N., Gao, K., Chen, F., Yuan, L., and Mi, G. (2013). Ammonium inhibits primary root growth by reducing the length of meristem and elongation zone and decreasing elemental expansion rate in the root apex in Arabidopsis thaliana. PLoS ONE 8 (4), 61031. doi:10.1371/JOURNAL.PONE.0061031
Liu, Z., Fabiano, R., Giehl, H., Hartmann, A., Hajirezaei, M. R., Carpentier, S., et al. (2020). Seminal and nodal roots of barley differ in anatomy, proteome and nitrate uptake capacity. Plant Cell Physiol. 61 (7), 1297–1308. doi:10.1093/pcp/pcaa059
Liu, Z., Gao, K., Shan, S., Gu, R., Wang, Z., Craft, E. J., et al. (2017). Comparative analysis of root traits and the associated QTLs for maize seedlings grown in paper roll, hydroponics and vermiculite culture system. Front. Plant Sci. 8. doi:10.3389/fpls.2017.00436
Lu, J.-L., Ertl, J. R., and Chen, C.-M. (1990). Cytokinin enhancement of the light induction of nitrate reductase transcript levels in etiolated barley leaves. Plant Mol. Biol. 14, 585–594. doi:10.1007/BF00027504
Lynch, J. P. (2013). Steep, cheap and deep: An ideotype to optimize water and N acquisition by maize root systems. Ann. Bot. 112 (2), 347–357. doi:10.1093/aob/mcs293
Mairhofer, S., Zappala, S., Tracy, S. R., Sturrock, C., Bennett, M., Mooney, S. J., et al. (2012). RooTrak: Automated recovery of three-dimensional plant root architecture in soil from x-ray microcomputed tomography images using visual tracking. Plant Physiol. 158 (2), 561–569. doi:10.1104/PP.111.186221
Masclaux-Daubresse, C., Daniel-Vedele, F., Dechorgnat, J., Chardon, F., Gaufichon, L., and Suzuki, A. (2010). Nitrogen uptake, assimilation and remobilization in plants: Challenges for sustainable and productive agriculture. Ann. Bot. 105, 1141–1157. doi:10.1093/aob/mcq028
Naz, S., Shen, Q., Wa Lwalaba, J. L., and Zhang, G. (2021). Genotypic difference in the responses to nitrogen fertilizer form in tibetan wild and cultivated barley. Plants 10 (3), 1–12. doi:10.3390/plants10030595
O’Sullivan, C., Palta, J., Farrell, M., and Treble, K. (2016). “Genotypic variability in wheat for preference of NH4+ , NO3-or NH4NO3,” in Solutions to Improve Nitrogen Use Efficiency for the World, Melbourne, 4–8, 2016.
Özdemir, B., Okay, F. Y., Sarikamiş, G., Yüksel Özmen, C., Kibar, U., and Ergül, A. (2021). Crosstalk between flowering and cold tolerance genes in almonds (Amygdalus spp.). Turkish J. Agric. For. 45 (4), 484–494. doi:10.3906/tar-2103-101
Plett, D. C., Ranathunge, K., Melino, V. J., Kuya, N., Uga, Y., and Kronzucker, H. J. (2020). The intersection of nitrogen nutrition and water use in plants: New paths toward improved crop productivity. J. Exp. Bot. 71 (15), 4452–4468. doi:10.1093/jxb/eraa049
Pourkheirandish, M., Hensel, G., Kilian, B., Senthil, N., Chen, G., Sameri, M., et al. (2015). Evolution of the grain dispersal system in barley. Cell 162 (3), 527–539. doi:10.1016/j.cell.2015.07.002
Ramireddy, E., Hosseini, S. A., Eggert, K., Gillandt, S., Gnad, H., von Wirén, N., et al. (2018). Root engineering in barley: Increasing cytokinin degradation produces a larger root system, mineral enrichment in the shoot and improved drought tolerance. Plant Physiol. 177 (3), 1078–1095. doi:10.1104/pp.18.00199
Raun, W. R., and Johnson, G. v. (1999). Improving nitrogen use efficiency for cereal production. Agron J. 91 (3), 357–363. doi:10.2134/agronj1999.00021962009100030001x
Robinson, H., Kelly, A., Fox, G., Franckowiak, J., Borrell, A., and Hickey, L. (2018). Root architectural traits and yield: exploring the relationship in barley breeding trials. Euphytica 214 (9). doi:10.1007/s10681-018-2219-y
Sadras, V. O., Hayman, P. T., Rodriguez, D., Monjardino, M., Bielich, M., Unkovich, M., et al. (2016). Interactions between water and nitrogen in Australian cropping systems: Physiological, agronomic, economic, breeding and modelling perspectives. Crop Pasture Sci. 67 (10), 1019–1053. doi:10.1071/CP16027
Schneider, H. M., Postma, J. A., Kochs, J., Pflugfelder, D., Lynch, J. P., and van Dusschoten, D. (2020). Spatio-temporal variation in water uptake in seminal and nodal root systems of barley plants grown in soil. Front. Plant Sci. 11, 1247. doi:10.3389/fpls.2020.01247
Schneider, H. M., Postma, J. A., Wojciechowski, T., Kuppe, C., and Lynch, J. P. (2017a). Root cortical senescence improves growth under suboptimal availability of N, P, and K. Plant Physiol. 174 (4), 2333–2347. doi:10.1104/pp.17.00648
Schneider, H. M., Wojciechowski, T., Postma, J. A., Brown, K. M., Lücke, A., Zeisler, V., et al. (2017b). Root cortical senescence decreases root respiration, nutrient content and radial water and nutrient transport in barley. Plant Cell Environ. 40 (8), 1392–1408. doi:10.1111/pce.12933
Seethepalli, A., Dhakal, K., Griffiths, M., Guo, H., Freschet, G. T., and York, L. M. (2021). RhizoVision Explorer: Open-source software for root image analysis and measurement standardization. AoB PLANTS 13 (6), plab056. doi:10.1093/AOBPLA/PLAB056
Singh, S., Jighly, A., Sehgal, D., Burgueño, J., Joukhadar, R., Singh, S. K., et al. (2021). Direct introgression of untapped diversity into elite wheat lines. Nat. Food 2 (10), 819–827. doi:10.1038/s43016-021-00380-z
Skiba, U. M., and Rees, R. M. (2014). Nitrous oxide, climate change and agriculture. CAB Rev. Perspect. Agric. Veterinary Sci. Nutr. Nat. Resour. 9, 1–7. doi:10.1079/PAVSNNR20149010
Sýkorová, B., Kurešová, G., Daskalova, S., Trčková, M., Hoyerová, K., Raimanová, I., et al. (2008). Senescence-induced ectopic expression of the A. tumefaciens ipt gene in wheat delays leaf senescence, increases cytokinin content, nitrate influx, and nitrate reductase activity, but does not affect grain yield. J. Exp. Bot. 59 (2), 377–387. doi:10.1093/jxb/erm319
Tam, V., Patel, N., Turcotte, M., Bossé, Y., Paré, G., and Meyre, D. (2019). Benefits and limitations of genome-wide association studies. Nat. Rev. Genet. 20 (88), 467–484. doi:10.1038/s41576-019-0127-1
Tang, C., Weligama, C., and Sale, P. (2013). Subsurface soil acidification in farming systems: Its possible causes and management options. Mol. Environ. Soil Sci. 2013, 389–412. doi:10.1007/978-94-007-4177-5_13
Teramoto, S., Kitomi, Y., Nishijima, R., Takayasu, S., Maruyama, N., and Uga, Y. (2019). Backhoe-assisted monolith method for plant root phenotyping under upland conditions. Breed. Sci. 69 (3), 508–513. doi:10.1270/JSBBS.19019
Teramoto, S., Takayasu, S., Kitomi, Y., Arai-Sanoh, Y., Tanabata, T., and Uga, Y. (2020). High-throughput three-dimensional visualization of root system architecture of rice using X-ray computed tomography. Plant Methods 16 (1), 66–14. doi:10.1186/s13007-020-00612-6
Tolley, S., and Mohammadi, M. (2020). Variation in root and shoot growth in response to reduced nitrogen. Plants 9 (2), 144. doi:10.3390/plants9020144
Tsay, Y. F., and Hsu, P. K. (2010)., 19. Berlin, Heidelberg: Springer, 223–236. doi:10.1007/978-3-642-13431-9_9The role of plasma membrane Nitrogen transporters in Nitrogen acquisition and utilizationPlant Cell Monogr.
von Bothmer, R., Sato, K., Komatsuda, T., Yasuda, S., and Fischbeck, G. (2003). “The domestication of cultivated barley,” in Diversity in barley. Editors R. von Bothmer, T. van Hintum, H. Knüpffer, and K. Sato (Hordeum vulgare: Elsevier).
Wilhelmi, M. M. R., Sanchez-Rodriguez, E., Angel Rosales, M., Blasco, N., Jose Rios, J., Romero, L., et al. (2011). Cytokinin-dependent improvement in transgenic P SARK ::IPT tobacco under nitrogen deficiency. J. Agric. Food Chem. 59, 10491–10495. doi:10.1021/jf202604k
Willcox, G. (2013). Anthropology. The roots of cultivation in southwestern Asia. Science 341 (6141), 39–40. doi:10.1126/science.1240496
Witte, C. P. (2011). Urea metabolism in plants. Plant Sci. 180 (3), 431–438. doi:10.1016/j.plantsci.2010.11.010
Wright, F. A., Shabalin, A. A., and Rusyn, I. (2012). Computational tools for discovery and interpretation of expression quantitative trait loci. Pharmacogenomics 13 (3), 343–352. doi:10.2217/PGS.11.185
Wu, Y., Zheng, Z., Visscher, P. M., and Yang, J. (2017). Quantifying the mapping precision of genome-wide association studies using whole-genome sequencing data. Genome Biol. 18 (86), 86. doi:10.1186/s13059-017-1216-0
Xu, Y., Xu, C., and Xu, S. (2017). Prediction and association mapping of agronomic traits in maize using multiple omic data. Heredity 119 (3), 174–184. doi:10.1038/hdy.2017.27
Yang, J. J., Grissa, D., Lambert, C. G., Bologa, C. G., Mathias, S. L., Waller, A., et al. (2021). Tiga: Target illumination GWAS analytics. Bioinformatics 37 (21), 3865–3873. doi:10.1093/BIOINFORMATICS/BTAB427
Yang, L., Hu, H., Zhu, B., Jin, X., Wu, F., and Zhang, G. (2014). Genotypic variations of N useefficiency in Tibetan wild and cultivated barleys. J. Zhejiang Univ. 40 (2), 155–164. doi:10.3785/j.issn.1008-9209.2013.09.021
Yin, R., Kwoh, C. K., and Zheng, J. (2019). Whole genome sequencing analysis. Encycl. Bioinforma. Comput. Biol. ABC Bioinforma. 1–3, 176–183. doi:10.1016/B978-0-12-809633-8.20095-2
Zanin, L., Tomasi, N., Wirdnam, C., Meier, S., Komarova, N. Y., Mimmo, T., et al. (2014). Isolation and functional characterization of a high affinity urea transporter from roots of Zea mays. BMC Plant Biol. 14 (1), 222. doi:10.1186/s12870-014-0222-6
Zhang, H., and Forde, B. G. (2000). Regulation of Arabidopsis root development by nitrate availability. J. Exp. Bot. 51 (342), 51–59. doi:10.1093/JEXBOT/51.342.51
Zhong, H., Liu, S., Meng, X., Sun, T., Deng, Y., and Kong, W. (2021). Uncovering the genetic mechanisms regulating panicle architecture in rice with GPWAS and GWAS. BMC Genomics 22 (1), 1–13. doi:10.1186/S12864-021-07391-X/FIGURES/4
Keywords: nitrogen use efficiency, genome-wide association study, root architecture, nitrogen transport, root phenotyping
Citation: Huang C, Butterly CR, Moody D and Pourkheirandish M (2023) Mini review: Targeting below-ground plant performance to improve nitrogen use efficiency (NUE) in barley. Front. Genet. 13:1060304. doi: 10.3389/fgene.2022.1060304
Received: 03 October 2022; Accepted: 19 December 2022;
Published: 02 March 2023.
Edited by:
Noureddine Benkeblia, University of the West Indies, JamaicaCopyright © 2023 Huang, Butterly, Moody and Pourkheirandish. This is an open-access article distributed under the terms of the Creative Commons Attribution License (CC BY). The use, distribution or reproduction in other forums is permitted, provided the original author(s) and the copyright owner(s) are credited and that the original publication in this journal is cited, in accordance with accepted academic practice. No use, distribution or reproduction is permitted which does not comply with these terms.
*Correspondence: Clayton R. Butterly, Y2xheXRvbi5idXR0ZXJseUB1bmltZWxiLmVkdS5hdQ==; Mohammad Pourkheirandish, bW9oYW1tYWQucEB1bmltZWxiLmVkdS5hdQ==
Disclaimer: All claims expressed in this article are solely those of the authors and do not necessarily represent those of their affiliated organizations, or those of the publisher, the editors and the reviewers. Any product that may be evaluated in this article or claim that may be made by its manufacturer is not guaranteed or endorsed by the publisher.
Research integrity at Frontiers
Learn more about the work of our research integrity team to safeguard the quality of each article we publish.