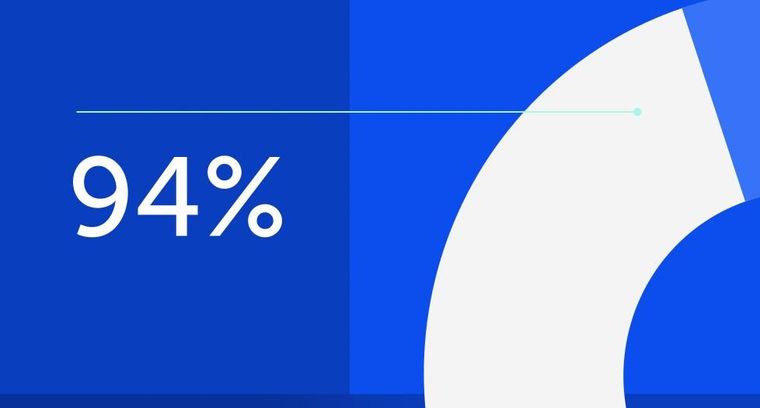
94% of researchers rate our articles as excellent or good
Learn more about the work of our research integrity team to safeguard the quality of each article we publish.
Find out more
EDITORIAL article
Front. Genet., 17 October 2022
Sec. Computational Genomics
Volume 13 - 2022 | https://doi.org/10.3389/fgene.2022.1057408
This article is part of the Research TopicIntegrative Approaches to Analyze Cancer Based on Multi-OmicsView all 8 articles
Editorial on the Research Topic
Integrative approaches to analyze cancer based on multi-omics
Cancer is a multifactorial malignant disease driven by environmental exposure, genetic polymorphism, somatic mutation events, and other downstream omics (Shen et al., 2021a; Sung et al., 2021). In the era of big data, leveraging high dimensional omics data and conducting computational studies can advance oncogenomics research. Integration of multi-omics tumor profiling data, supported by compatible algorithms, enables the establishment of novel cancer biomarkers and personalized treatment strategies aimed at reducing cancer-specific death and improving patient prognosis (Akhoundova and Rubin, 2022). Moreover, with the development of multi-omics designed studies, large-scale and high-quality omics databases are gradually established and open to the public (Table 1). While the omics data cost huge, most of the research articles on our topic leveraged publicly available data (e.g., The Cancer Genome Atlas) and made certain discoveries.
The large-scale cancer omics studies greatly promote the research of tumor etiology, progression, outcome, and treatment. The first glorious achievement is the identification of numerous cancer-related loci through genome-wide association studies (GWAS) (Tam et al., 2019). As the sample size increases with sufficient statistical power, causal single nucleotide polymorphisms (SNPs) have been reported for major cancers. However, the mechanistic gap between variants and traits is still hard to bridge, while the majority of the identified variants are located in non-coding regions and have been shown to have limited functions (Wu et al., 2018). Thus, it is essential to link the genetic variants to downstream omics to explain the biological functions. The first approach is leveraging the current in-silico databases to perform functional annotation analyses, such as expression, splice, methylation, metabolite, protein quantitative trait locus (QTL), histone modification, and protein-bound. The second approach is to predict trans-omics biomarkers based on QTL information and then evaluate the association of predicted biomarkers and cancer outcomes, such as transcriptome-wide association (TWAS) (Gusev et al., 2016) and Mendelian randomization (MR) (Zheng et al., 2020). These post-GWAS studies support the findings of GWAS and provide favorable evidence for exploring the relationship between multi-omics markers and cancers.
The second glorious achievement is the development of biotechnology and bioinformatics approaches to understand multi-omics data, including genomics, transcriptomics, epigenomics, metabolomics, and proteomics. They have updated our understanding of oncology and improved the accuracy of outcome prediction.
In genomics, somatic mutation events (e.g., point mutation, tumor mutation burden, rearrangements) derived from tumor tissues and matched normal tissues in next-generation sequencing (NGS) give us novel insights into tumor driver factors and are practical to guide clinical therapy, such as targeted therapy and immunotherapy. In transcriptomics, RNA sequencing of bulk and single-cell technology advances us to understand the various RNA biomarkers that play essential roles in tumor regulation, proliferation, differentiation, and metastasis (Zhang et al., 2022). While the protein-coding genes have been deeply investigated, the function of non-coding RNAs remains largely unknown, such as long non-coding RNA (lncRNA), circular RNA (circRNA), and PIWI-Interacting RNA (piRNA) (Shen et al., 2021b). Studies have found that non-coding RNAs had a close relationship with tumor microenvironment, immune checkpoints, and specific mechanisms, such as N6-Methyladenosine, ferroptosis, and autophagy (Sun et al.; Zhao et al., Lan et al., Yang et al.). In epigenomics, epigenetic modifications play important roles in the DNA chromatin structure and accessibility, affecting gene transcription and regulation. Among these, DNA methylation marks at the cytosine-phosphate-guanine (CpG) dinucleotide sites are extensively documented that regulate gene expression, genome stability, and cell fate (Shen et al., 2018). Numerous successful epigenome-wide association studies (EWAS) have discovered important CpG sites across human diseases (Campagna et al., 2021). In addition, mass spectrometry (MS)-based proteomics and metabolomics are downstream biomarkers with remarkable effects on cancer outcome, which could reflect the cancer course more directly and should be paid more attention (Lotta et al., 2021; Satpathy et al., 2021).
For multi-omics data, various types of integration methods and algorithms are proposed, which could be generally classified into two fields: traditional methods and artificial intelligence (AI). The traditional statistical methods and bioinformatic algorithms are widely recognized. For example, Shen et al. (2017). performed variable selection based on DNA methylation using sure independence screening (SIS) and developed a trans-omics prognosis model including CpG sites and their corresponding gene expression based on Cox proportional hazards model to predict the overall survival of oral squamous cell carcinoma. The integrated model of clinical characteristics, methylation, and gene expression outperformed single omics. Moreover, bioinformatic methods are practical, such as gene co-expression network, unsupervised similar omics network fusion, pathway enrichment analysis, gene set variation analysis (Shen et al., 2019). Recently, AI is becoming a hotspot where machine learning and deep learning are widely applied in diagnosis and risk/prognosis prediction using cancer omics data (Arjmand et al.). AI generally has higher accuracy for cancer diagnosis and prediction, while it could consider the complex high-order interaction effects ignored in parametric statistical models. However, an enormous disadvantage of AI is the “black box” problem that it does not consider causal medical relationships and could not explain the potential pathogenesis mechanism.
However, challenges still exist for trans-omics studies. First, large-scale DNA sequencing [e.g., whole exome sequencing (WES), whole genome sequencing (WGS)] is gradually focused on for its high coverage of genetic variants. For example, the UK Biobank 150 k WGS project contains 585 million single nucleotide variants (SNVs). At the same time, most of them are rare variants (minor allele frequency <0.01) and ultra-rare variants (minor allele carrier <10), which should not be ignored and might explain part of “missing heritability” (Halldorsson et al., 2022). However, current QTL databases could not contain all rare variants that need novel methods to explore the trans-omics biomarkers, such as variant set-based design. Second, most prediction models only focus on the performance (e.g., Area Under Curve, C-index) but ignore the causal biological relationship (Shu et al.; Zhou et al.). Nonetheless, the mechanism should be comprehensively understood for adjuvant treatment and drug development to seek valuable and practical target therapy biomarkers. Third, although the definition of omics data is well established, deep data-mining of omics data is still insufficient. In addition, new biotechnological (e.g., single-cell sequencing, radiomics, electronic medical records) and computational methods (e.g., deep learning, natural language processing) have been developed, both of which require further research.
In conclusion, trans-omics tumor investigation approaches have rapidly developed, diving deeply into the molecular landscapes of tumors, and elucidating exciting novel aspects of cancer biology. Clinical application of multi-omics biomarkers will further improve our understanding of tumor biology and significantly shape cancer precision treatment in the future.
SS collected the database information and drafted the manuscript.
This study was supported by the National Natural Science Foundation of China (82103946) and Natural Science Foundation of the Jiangsu Higher Education Institutions of China (21KJB330004).
We want to acknowledge Yang Zhao, Xia Jiang, and Ping Zeng who organize this research topic.
The author declares that the research was conducted in the absence of any commercial or financial relationships that could be construed as a potential conflict of interest.
All claims expressed in this article are solely those of the authors and do not necessarily represent those of their affiliated organizations, or those of the publisher, the editors and the reviewers. Any product that may be evaluated in this article, or claim that may be made by its manufacturer, is not guaranteed or endorsed by the publisher.
Akhoundova, D., and Rubin, M. A. (2022). Clinical application of advanced multi-omics tumor profiling: Shaping precision oncology of the future. Cancer Cell 40 (9), 920–938. doi:10.1016/j.ccell.2022.08.011
Campagna, M. P., Xavier, A., Lechner-Scott, J., Maltby, V., Scott, R. J., Butzkueven, H., et al. (2021). Epigenome-wide association studies: Current knowledge, strategies and recommendations. Clin. Epigenetics 13 (1), 214. doi:10.1186/s13148-021-01200-8
Gusev, A., Ko, A., Shi, H., Bhatia, G., Chung, W., Penninx, B. W., et al. (2016). Integrative approaches for large-scale transcriptome-wide association studies. Nat. Genet. 48 (3), 245–252. doi:10.1038/ng.3506
Halldorsson, B. V., Eggertsson, H. P., Moore, K. H. S., Hauswedell, H., Eiriksson, O., Ulfarsson, M. O., et al. (2022). The sequences of 150, 119 genomes in the UK Biobank. Nature 607 (7920), 732–740. doi:10.1038/s41586-022-04965-x
Lotta, L. A., Pietzner, M., Stewart, I. D., Wittemans, L. B. L., Li, C., Bonelli, R., et al. (2021). A cross-platform approach identifies genetic regulators of human metabolism and health. Nat. Genet. 53 (1), 54–64. doi:10.1038/s41588-020-00751-5
Satpathy, S., Krug, K., Jean Beltran, P. M., Savage, S. R., Petralia, F., Kumar-Sinha, C., et al. (2021). A proteogenomic portrait of lung squamous cell carcinoma. Cell 184 (16), 4348–4371.e40. e4340. doi:10.1016/j.cell.2021.07.016
Shen, S., Wang, G., Shi, Q., Zhang, R., Zhao, Y., Wei, Y., et al. (2017). Seven-CpG-based prognostic signature coupled with gene expression predicts survival of oral squamous cell carcinoma. Clin. Epigenetics 9, 88. doi:10.1186/s13148-017-0392-9
Shen, S., Wang, G., Zhang, R., Zhao, Y., Yu, H., Wei, Y., et al. (2019). Development and validation of an immune gene-set based Prognostic signature in ovarian cancer. EBioMedicine 40, 318–326. doi:10.1016/j.ebiom.2018.12.054
Shen, S., Wei, Y., Li, Y., Duan, W., Dong, X., Lin, L., et al. (2021). A multi-omics study links TNS3 and SEPT7 to long-term former smoking NSCLC survival. NPJ Precis. Oncol. 5 (1), 39. doi:10.1038/s41698-021-00182-3
Shen, S., Zhang, R., Guo, Y., Loehrer, E., Wei, Y., Zhu, Y., et al. (2018). A multi-omic study reveals BTG2 as a reliable prognostic marker for early-stage non-small cell lung cancer. Mol. Oncol. 12 (6), 913–924. doi:10.1002/1878-0261.12204
Shen, S., Zhang, R., Jiang, Y., Li, Y., Lin, L., Liu, Z., et al. (2021). Comprehensive analyses of m6A regulators and interactive coding and non-coding RNAs across 32 cancer types. Mol. Cancer 20 (1), 67. doi:10.1186/s12943-021-01362-2
Sung, H., Ferlay, J., Siegel, R. L., Laversanne, M., Soerjomataram, I., Jemal, A., et al. (2021). Global cancer statistics 2020: GLOBOCAN estimates of incidence and mortality worldwide for 36 cancers in 185 countries. Ca. Cancer J. Clin. 71 (3), 209–249. doi:10.3322/caac.21660
Tam, V., Patel, N., Turcotte, M., Bosse, Y., Pare, G., and Meyre, D. (2019). Benefits and limitations of genome-wide association studies. Nat. Rev. Genet. 20 (8), 467–484. doi:10.1038/s41576-019-0127-1
Wu, L., Shi, W., Long, J., Guo, X., Michailidou, K., Beesley, J., et al. (2018). A transcriptome-wide association study of 229, 000 women identifies new candidate susceptibility genes for breast cancer. Nat. Genet. 50 (7), 968–978. doi:10.1038/s41588-018-0132-x
Zhang, Z., Wang, Z. X., Chen, Y. X., Wu, H. X., Yin, L., Zhao, Q., et al. (2022). Integrated analysis of single-cell and bulk RNA sequencing data reveals a pan-cancer stemness signature predicting immunotherapy response. Genome Med. 14 (1), 45. doi:10.1186/s13073-022-01050-w
Keywords: cancer, trans-omics, GWAS—genome-wide association study, bioinformatics and computational biology, next-generation sequencing
Citation: Shen S (2022) Editorial: Integrative Approaches to Analyze Cancer Based on Multi‐Omics. Front. Genet. 13:1057408. doi: 10.3389/fgene.2022.1057408
Received: 29 September 2022; Accepted: 07 October 2022;
Published: 17 October 2022.
Edited and reviewed by:
Richard D. Emes, University of Nottingham, United KingdomCopyright © 2022 Shen. This is an open-access article distributed under the terms of the Creative Commons Attribution License (CC BY). The use, distribution or reproduction in other forums is permitted, provided the original author(s) and the copyright owner(s) are credited and that the original publication in this journal is cited, in accordance with accepted academic practice. No use, distribution or reproduction is permitted which does not comply with these terms.
*Correspondence: Sipeng Shen, c3NoZW5AbmptdS5lZHUuY24=
Disclaimer: All claims expressed in this article are solely those of the authors and do not necessarily represent those of their affiliated organizations, or those of the publisher, the editors and the reviewers. Any product that may be evaluated in this article or claim that may be made by its manufacturer is not guaranteed or endorsed by the publisher.
Research integrity at Frontiers
Learn more about the work of our research integrity team to safeguard the quality of each article we publish.