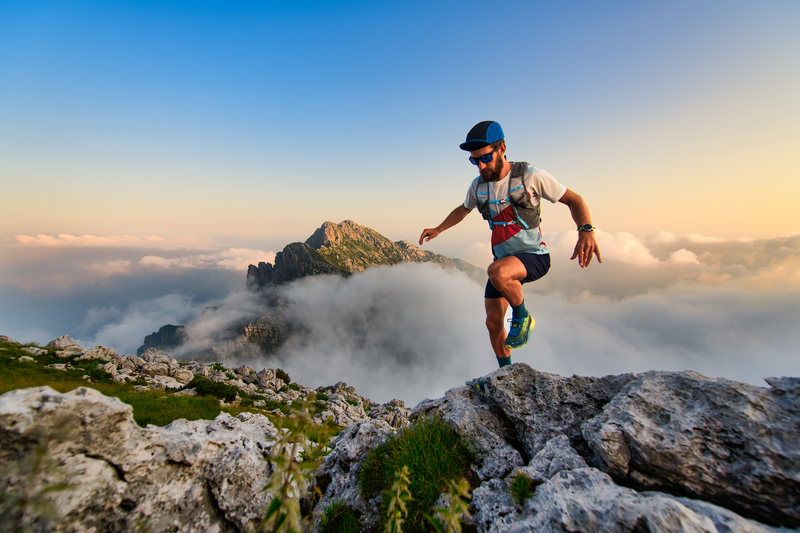
94% of researchers rate our articles as excellent or good
Learn more about the work of our research integrity team to safeguard the quality of each article we publish.
Find out more
ORIGINAL RESEARCH article
Front. Genet. , 30 November 2022
Sec. Cancer Genetics and Oncogenomics
Volume 13 - 2022 | https://doi.org/10.3389/fgene.2022.1052092
Background: Lung adenocarcinoma (LUAD) is a sex-biased and easily metastatic malignant disease. A signature based on 5 long non-coding RNAs (lncRNAs) has been established to promote the overall survival (OS) prediction effect on LUAD.
Methods: The RNA expression profiles of LUAD patients were obtained from The Cancer Genome Atlas. OS-associated lncRNAs were identified based on the differential expression analysis between LUAD and normal samples followed by survival analysis, univariate and multivariate Cox proportional hazards regression analyses. OS-associated lncRNA with sex dimorphism was determined based on the analysis of expression between males and females. Functional enrichment analysis of the Gene Ontology (GO) terms and the Kyoto Encyclopedia of Genes and Genomes (KEGG) pathways was performed to explore the possible mechanisms of 5-lncRNA signatures.
Results: A 5-lncRNA signature (composed of AC068228.1, SATB2-AS1, LINC01843, AC026355.1, and AL606489.1) was found to be effective in predicting high-risk LUAD patients as well as applicable to female and male subgroups and <65-year and ≥65-year age subgroups. The forecasted effect of the 5-lncRNA signature was more efficient and stable than the TNM stage and other clinical risk factors (such as sex and age). Functional enrichment analysis revealed that the mRNA co-expressed with these five OS-related lncRNAs was associated with RNA regulation within the nucleus. AL606489.1 demonstrated a sexual dimorphism that may be associated with microtubule activity.
Conclusion: Our 5-lncRNA signature could efficaciously predict the OS of LUAD patients. AL606489.1 demonstrated gender dimorphism, which provides a new direction for mechanistic studies on sexual dimorphism.
Lung cancer is the leading cause of death in cancer patients across the world (Bade and Cruz, 2020). Approximately 20% of the lung cancer cases are accounted for by small-cell lung cancer, while the remaining 80% are accounted for by non-small-cell lung cancer (Leung et al., 2016). Lung adenocarcinoma (LUAD) and lung squamous cell carcinoma are the most common subtypes of non-small-cell lung cancer (Herbst et al., 2018). LUAD shifts earlier than lung squamous cell carcinoma (Chen et al., 2017); therefore, it is very important to have effective early diagnostic methods for LUAD. Epidemiological studies have revealed that LUAD varies between males and females, with the highest incidence of occurrence among never-smokers and women (Couraud et al., 2012). Meanwhile, several other factors affect lung cancer (Paz-Ares et al., 2018). In addition to the well-known risk factors such as tobacco, a close association of genetic variants has been demonstrated in multiple studies with the risk of lung cancer (Li and Hemminki, 2004; Musolf et al., 2016; Cheng et al., 2019).
With the widespread development of the human genome program (Collins et al., 2003), several genes have been highlighted as being probably related to the onset of lung cancer, including AKT (Hyman et al., 2017), BRD4 (Zhang et al., 2021), FGFR1 (Yuan et al., 2017), BRAF (Lokhandwala et al., 2019), MET (Wu et al., 2020), PIK3CA (Wang et al., 2020), and EGFR (Zhao et al., 2021). However, the current reports on the long non-coding RNA (lncRNA) remain inadequate. LncRNA refers to any polyadenylated RNA of length >200 bp (Quinn and Chang, 2016), which forms a transcript of a large portion of the eukaryotic genome (Jathar et al., 2017). In recent years, lncRNA has received continuous attention from researchers for its important role in the eukaryotic gene expression and genome remodeling (Cech and Steitz, 2014; Fungtammasan et al., 2015), including from the tumor perspective (Hauptman and Glavač, 2013). Up to 37,595 non-coding genes (Snyder et al., 2020) have been identified, according to the latest data from the ENCODE Project Consortium 2018 (Davis et al., 2018). Clearly, the number of current studies on lncRNA are insufficient compared to this large number of genes.
In numerous lncRNA-related studies, lncRNA has been widely reported as a biomarker in the diseases of multiple systems (Zhang et al., 2018; Yu et al., 2019). Ideal biomarkers not only facilitate the early diagnosis of disease (Xu et al., 2020) but also predict patient prognosis (Chao and Zhou, 2019) as well as become potential drug therapeutic targets (Tamang et al., 2019). In the field of LUAD, the effect of lncRNA as a biomarker on tumor cells has been explored in terms of immunity (Li et al., 2020), ferroptosis (Lu et al., 2021), and cell pyroptosis (Li et al., 2018). However, for LUAD, as a typical sex-biased (Yuan et al., 2016) malignancy, no investigation has yet explored the possible mechanisms of sex-biased differences in LUAD through the biomarker role of lncRNA. The occurrence of cancer is affected by gender differences, which is a consistent finding in the field of cancer epidemiology (Dorak and Karpuzoglu, 2012). Liu et al. analyzed the sex differences in lncRNAs across different cancers and found that LINC 00263 acts as an oncogene associated with men and estrogens; these findings may help explore the differential gene regulatory mechanisms in sex-specific cancers (Liu et al., 2020).
In conclusion, this study identified the prognostic models of LUAD through information mining from public databases and explored the possible mechanisms of sex differences in LUAD. Alternatively, as The Cancer Genome Atlas (TCGA) contains the most extensive lncRNA expression matrix (Tomczak et al., 2015), we prefer to conduct experiments in the TCGA database.
The lncRNA and mRNA expression dataset in the FPKM format as well as the clinical characters for 535 LUAD patients and 59 normal patients were directly downloaded from the TCGA (https://portal.gdc.cancer.gov/), updated until 5 December 2021. GEO database (https://www.ncbi.nlm.nih.gov/geo/) was used to perform external validation.
DELs between the LUAD and normal samples were isolated from all lncRNAs using the R software. The p-value of each lncRNA in the LUAD and normal samples was calculated by the rank-sum test, and the p-values were rectified by the False Discovery Rate (FDR) method. Only the lncRNAs with adjusted p-values < 0.05 and log2 | fold change | values > 2 were defined as differentially expressed lncRNAs. Volcano plot and heatmaps were visualized by the “plot” function and the “heatmap” package of the R, respectively.
First, we removed LUAD patients with OS < 0 days. Next, we employed univariate Cox proportional hazards regression (CPHR) analysis and Kaplan-Meier analysis to assess the presence of any significant correlations between the expression of each DELs and the OS of LUAD patients. Only lncRNAs with p < 0.01 from both the analyses were considered with a logical agreement in expression and prognostic effect and selected as the candidate OS-related lncRNAs. Then, half of the patients were randomly assigned as the “primary dataset” after removing patients with incomplete clinical information; the original complete dataset was called the “entire dataset”. In addition to randomization, the criteria for grouping included no statistical differences in the clinical characteristics between the “primary” and “entire datasets. In order to fit the prediction model with the best-prediction effect, multivariate CPHR analysis (stepwise model) of candidate OS-associated lncRNAs was performed with the R software in the “primary dataset”. To ensure the goodness of fitting and to avoid overfitting, the Akaike information criterion (AIC) was computed, and the prediction model with the lowest AIC was considered as the most ideal. LncRNAs included in the best prediction model were selected as OS-related lncRNAs.
We determined the coefficients for each lncRNA by another multivariate CPHR analysis in the “primary dataset”. Until this point, we confirmed a risk score formula with the expressions of the OS-related lncRNAs as the independent variables and weighted by the regression coefficients corresponding to the lncRNAs. The risk scoring formula used is given below:
where βi correspond to the correlation coefficient.
To determine whether the OS-related lncRNA signature was an independent predictor of OS, we applied both univariate and multivariate CPHR analyses of OS-related lncRNA signature and the routine clinical risk factors (such as sex, age, TNM stage, tumor stage, lymph node metastasis, and distant metastasis) in the LUAD patients. Next, we assessed whether the predictive effect of the OS-related lncRNA signature on OS was independent of the routine clinical risk factors by stratified analysis. Meanwhile, to evaluate the prognostic effect of the lncRNA-based classifiers across different time ranges, we plotted the time-dependent receiver operating characteristic (ROC) curves and then calculated the area under the time-dependent ROC curve (AUC) values for each dataset. Finally, the predictive effects of the 5-lncRNA classifier and the classifiers based on the other clinical risk factors were compared by AUC.
Whether the OS-related lncRNA was differentially expressed between the male and female patients was determined by the rank-sum test using p < 0.05 as the significance threshold. In both the male and female groups, the patients were assigned into two groups of high or low expression bounded by the median expression of an OS-related lncRNA, and the Kaplan–Meier curve was applied to analyze whether there were differences in survival time between the high and low expression groups. The lncRNA was considered to be with gender dimorphism if an OS-related lncRNA was differentially expressed in males and females while showing different prognostic association in males and females.
The co-expression degree of OS-related lncRNAs and mRNA was determined by Pearson’s correlational analysis. The mRNAs with a positive correlation coefficient >0.5 with OS-related lncRNAs were employed in the next step of enrichment analysis. The “cluster profile” package in R software was used for the functional enrichment analysis using the Gene Ontology (GO) terms and Kyoto Encyclopedia of Gene and Genomes (KEGG) pathways (Kanehisa and Goto, 2000; Kanehisa, 2019; Kanehisa et al., 2021), with p < 0.01 set as a significance threshold.
For the survival analysis, the survival curves were plotted by the Kaplan-Meier method, and the differential p-values were calculated by the log-rank test. The t-test was used to compare the presence of any significant differences between the “primary” and “entire datasets”. Unless otherwise specified, p < 0.05 was considered to indicate a statistical difference.
The flow chart illustrated in Figure 1 shows the overall design of this study and some of the main results. After data collation, we obtained the expression data of 14,142 lncRNAs and 19,658 mRNAs for 535 LUAD samples and 59 normal samples from the TCGA-LUAD database. Through statistical comparison, 1,223 DELs in tumor samples and normal samples were identified with a log2 | fold change |> 2 and adjusted p < 0.05. Of these 1,223 DELs, 1,044 lncRNA were upregulated and 179 were downregulated in the LUAD patients. Next, volcano plots and heatmaps of the differential genes were drawn using the “plot” function and the “pheatmap” package in the R software, the results of which are illustrated in Figures 2A,B.
FIGURE 2. Volcano plot and heatmap of lncRNAs. (A) Volcano plot of 1,223 lncRNAs in the LUAD samples. Yellow dots represent 1,044 upregulated lncRNAs, while blue dots represent 179 downregulated lncRNAs. (B) Heatmap of 1,223 lncRNAs expression levels in LUAD samples from the TCGA-LUAD project. N = normal samples, T = LUAD samples.
After the exclusion of 45 LUAD samples with incomplete survival data, 490 LUAD samples were finally enrolled in the study. In these 490 samples, 1,223 DELs were analyzed by the Kaplan-Meier method and univariate CPHR analysis, where OS served as the dependent variable and lncRNA expression as the independent variable. The results of the univariate CPHR analysis are depicted in Supplementary Table S1, and a total of 15 lncRNAs were found to be statistically significantly associated with OS in LUAD patients (all p < 0.01). Of this 15 lncRNA, the high expression of 13 lncRNAs (namely, LINC02081, AC010343.3, LINC02086, AC068228.1, AC022784.1, SATB2-AS1, AL138789.1, LINC01843, LINC00519, AL606489.1, DEPDC1-AS1, AC087588.2, and FAM83A-AS1) was associated with a shorter OS. In contrast, the high expression of AC026355.1 and AL031600.2 was associated with a higher OS. Moreover, as shown in Supplementary Figure S1, the results of the Kaplan-Meier analysis conformed to those of the univariate CPHR analyses. To this point, 15 lncRNAs with some correlation between the gene expression volume and prognosis were included as the candidate OS-related lncRNAs.
After removing 11 samples without complete clinical features (such as TNM stage or age), 479 LUAD samples formed the “entire dataset”, of which 239 groups were randomly selected as the “primary dataset”. The differential analysis revealed no statistical differences in the baseline clinical risk factors and OS between the “entire” and “primary datasets” (all p > 0.05; Table 1).
TABLE 1. Baseline clinical characteristics and OS between the “entire dataset” and the “primary dataset”.
Candidate prognosis lncRNAs were further screened by multivariate-CPHR analysis (stepwise model) in the “primary dataset” using AIC to avoid overfitting. Five OS-related lncRNAs were picked with the largest fit and the lowest AIC values (Table 2), namely, is AC068228.1, SATB2-AS1, LINC01843, AC026355.1, and AL606489.1. Next, these 5 OS-related lncRNAs and their risk coefficients were integrated into the predictive signature to obtain a risk scoring using the following formula:
Next, we computed the risk score for LUAD patients in the “primary dataset” according to the 5 lncRNA signatures. Using the median risk score (0.09382005) as the cut-off value, 239 LUAD patients were classified into high- (n = 119) or low- (n = 120) risk groups. The risk score distributions, OS status, and the 5 lncRNA expression profiles in the “primary datasets” are depicted in Figure 3 (A–C). OS-related lncRNAs expression heatmaps revealed that the 4 upregulated lncRNA (i.e., AC068228.1, SATB2-AS1, LINC01843, and AL606489.1) demonstrated higher expression levels in the high-risk group, and the AC026355.1 expression levels were lower in the high-risk groups. As shown in Figure 3D, the Kaplan–Meier curve obviously showed that the OS time in the high-risk group was less than that in the low-risk group (p = 1.071E-04, log-rank test). Subsequently, in the “primary dataset”, as shown in Figures 3E–G, the AUC of the time-dependent ROC curve was 0.768 at 1 year, 0.668 at 3 years, and 0.702 at 5 years.
FIGURE 3. Assessment of the 5-lncRNA signature for predicting OS of LUAD in the “primary dataset”. (A) The risk score distribution in the “primary dataset”. (B) The OS status in the “primary dataset”. (C) The OS-related lncRNAs expression heatmaps of the 5-lncRNA signature in the “primary dataset”. (D) Kaplan–Meier curves comparing OS between the high-risk groups (n = 119) and low-risk groups (n = 120) in the “primary dataset”. Blue- and red-shaded sections indicate the confidence intervals for survival. Listed below the curve is the number of patients being at risk. (E) Time-dependent ROC curve based on 5-lncRNA signature predicting 1 year-OS in the “primary dataset”. (F) Time-dependent ROC curve based on 5-lncRNA signature predicting 3 years-OS in the “primary dataset”. (G) Time-dependent ROC curve based on 5-lncRNA signature predicting 5 years-OS in the “primary dataset”.
To verify the prediction of 5-lncRNA signatures obtained from the “primary dataset”, we applied 5-lncRNA signatures to the “entire dataset” (n = 479). Similarly, 479 patients were classified into the high-risk (n = 244) and low-risk (n = 235) groups according to the median risk score in the “primary dataset”. The risk score distributions, OS status, and the 5 lncRNA expression profiles in the “entire dataset” are depicted in Figures 4A–C. The results from the “entire dataset” are consistent with those from the “primary dataset”. Meanwhile, the Kaplan–Meier curve (Figure 4D) showed that the OS in the high-risk group (n = 244) was significantly shorter than that in the low-risk group (n = 235) (p = 5.587E-07, log-rank test). As shown in Figures 4E–G, the AUC of the time-dependent ROC curve was 0.738 at 1 year, 0.661 at 3 years, and 0.709 at 5 years. The 5-lncRNA signature showed a good prediction performance both in the “primary dataset” and the “entire dataset” of the LUAD patients. The prediction results of 5-lncRNA signature in “primary dataset” and the “entire dataset” were shown in Supplementary Table S2.
FIGURE 4. Assessment of the 5-lncRNA signature in the “entire dataset”. (A) The risk score distribution in the “entire dataset”. (B) The OS status in the “entire dataset”. (C) The OS-related lncRNAs expression heatmaps of the 5-lncRNA signature in the “entire dataset”. (D) The Kaplan–Meier curves comparing OS between the high-risk groups (n = 244) and the low-risk groups (n = 235) in the “entire dataset”. Blue- and red-shaded sections indicate the confidence intervals for survival. The number of patients at risk is listed below the curve. (E) Time-dependent ROC curve based on 5-lncRNA signature predicting 1 year-OS in the “entire dataset”. (F) Time-dependent ROC curve based on 5-lncRNA signature predicting 3 years-OS in the “entire dataset”. (G) Time-dependent ROC curve based on 5-lncRNA signature predicting the 5 years-OS in the “entire dataset”.
Next, to examine whether the prognostic performance of the 5-lncRNA features was independent of other conventional clinical risk factors, we performed multivariate CPHR analyses. The hazard ratio (HR) in the “entire dataset” (Table 3) was 1.085 (p < 0.001, 95% CI = 1.052–1.118), and in the “primary dataset” (Supplementary Table S3) was 1.065 (p < 0.001, 95% CI = 1.028–1.102). The abovementioned data indicates that these 5 lncRNA signatures could independently predict the prognosis of LUAD patients as an independent prognostic factor for LUAD.
TABLE 3. Univariate and multivariate Cox proportional hazards regression analyses results of 5-lncRNA signature and other clinical risk factors in the “entire dataset”.
To validate the scope of applicability of the risk score prediction, we conducted a stratified analysis of the “entire dataset”. First, considering the number of people, 479 LUAD patients were classified into stage I (n = 259; Figure 5A) and stage Ⅱ–Ⅳ (n = 220; Figure 5B) based on the TNM stage. Each subgroup was classified as the high-risk and low-risk groups and then Kaplan–Meier curves were accordingly plotted. Second, 479 patients were classified into no (n = 311, Figure 5C) or yes (n = 159, Figure 5D) subgroups according to the absence or presence of lymphoid tract metastasis, respectively. Next, 479 patients with LUAD were assigned into male (n = 219, Figure 5E) and female subgroups (n = 260, Figure 5F). Then, 479 patients with LUAD were assigned to the age ≥ 65 years (n = 266, Figure 5G) and <65 years subgroups (n = 213, Figure 5H). Finally, we noted that, in all subgroups, the survival time was significantly lower in the high-risk group than that in the low-risk groups, albeit it was not statistically significant in the female (p = 0.08) subgroup. To further validate the association between OS and 5-lncRNA, GEO database (GSE3141 and GSE19188) was used to perform external validation. The Kaplan-Meier curves for OS associated with the SATB2-AS1 expression were shown in Supplementary Figure S2 (GSE3141: p = 0.6312, GSE19188: p = 0.0914, GSE3141 + GSE19188: p = 0.1322). It is a pity that all three statistics did not show a significant effect. However, SATB2-AS1 still showed a clear trend towards promoting oncogenes, which is consistent with our findings in the TCGA database. All case ID involved in this study were shown in Supplementary Table S4.
FIGURE 5. Stratified analysis of the 5-lncRNA signature in LUAD patients. (A) Kaplan-Meier analysis of patients in the stage I subgroup, (B) stage Ⅱ–IV subgroup, (C) without lymph node metastasis subgroup, (D) with lymph node metastasis subgroup, (E) male subgroup, (F) female subgroup, (G) age ≥65 years subgroups, and (H) age <65 years subgroups. The differences between the two risk groups were assessed by two-sided log-rank tests.
We employed the time-dependent ROC curves to compare the predictive effects of different prognostic factors using the AUC as a comparison indicator. As shown in Figure 6, the stable predictive performance of the 5-lncRNA signature is more outstanding than the conventional clinical characters such as the TNM stage, and are efficient to predict the prognosis of LUAD patients.
FIGURE 6. The prognostic value of the 5-lncRNA signature in comparison with other clinical factors. Time-dependent ROC curve analysis of the 5-lncRNA signature for predicting (A) 1 year-OS, (B) 3 years-OS, and (C) 5 years-OS in the “primary dataset”. Time-dependent ROC curve analysis of the 5-lncRNA signature for predicting (D) 1 year-OS, (E) 3 years-OS, and (F) 5 years-OS in the “entire dataset”.
Among the 5 OS-related genes, AL606489.1, SATB2-AS1 and AC068228.1 was differentially expressed between males and females (Figures 7A–C. This significant difference was not shown in LINC01843 (p = 0.5833) and AC026355.1 (p = 0.5177), as shown in Supplementary Figures S3A,B. The Kaplan–Meier curves for the OS related with AL606489.1 expression in males (low = 109, high = 110) and females (low = 130, high = 130) are depicted in Figures 7D,G respectively. For SATB2-AS1, the Kaplan–Meier curves in males and females are depicted in Figures 7E,H respectively. For AC068228.1, the Kaplan–Meier curves in males and females are depicted in Figures 7F,I respectively. In males, the high expression of AL606489.1, SATB2-AS1 or AC068228.1 was associated with the shorter OS. In females, the high expression of SATB2-AS1 or AC068228.1 was associated with the shorter OS. Dissimilarly, the high expression of AL606489.1 in females was not significantly associated with the OS (p = 0.2704). Finally, to verify whether this discrepancy was attributable to AL606489.1 association with the gender, we noted no significant difference in the overall survival between males and females by the Kaplan-Meier analysis (Supplementary Figure S3).
FIGURE 7. The expression of AL606489.1, SATB2-AS1 and AC068228.1 in LUAD. (A) Differentially expressed AL606489.1 between 260 female and 219 male tumor samples. (B) Differentially expressed SATB2-AS1 between 260 female and 219 male tumor samples. (C) Differentially expressed AC068228.1 between 260 female and 219 male tumor samples. Kaplan–Meier curves for OS associated with the AL606489.1 expression in (D) male and (G) female. Kaplan–Meier curves for OS associated with the SATB2-AS1 expression in (E) male and (H) female. Kaplan–Meier curves for OS associated with the AC068228.1 expression in (F) male and (I) female.
To determine the possible function of 5 OS-related lncRNAs in the tumorigenic development of LUAD tumors, we conducted an function enrichment analysis on mRNAs co-expressed with OS-associated lncRNAs in 490 LUAD samples. The levels of the 928 mRNA expressions were positively associated with the level of at least one OS-related lncRNA (co-expression coefficient >0.50). The GO analysis indicated that these co-expressed mRNAs were enriched in 52 GO terms (Supplementary Table S5). These GO terms were mainly enriched in regulating the mRNA metabolic processes, RNA splicing, and ubiquitin-specific protease activity (Figure 8A). Similar findings were obtained from the KEGG pathway enrichment analysis (Figure 8B), such as the ubiquitin-mediated proteolysis pathway. Therefore, the characteristics of 5-lncRNA mainly affected the gene expression within the nucleus and may be related to cell cycle regulation.
FIGURE 8. GO and KEGG functional enrichment analysis of the mRNA co-expressed with 5 OS-related lncRNA. (A) GO enrichment analysis. (B) KEGG enrichment analysis.
LUAD is one of the most widely diagnosed subtypes of lung cancer (Fong et al., 1999). Owing to the unknown pathogenesis and unsatisfactory treatment effect, the mortality of LUAD patients remains high (Jiang et al., 2019). In recent years, lncRNA has been applied as a potential tumor marker with promising research progress in LUAD (Li et al., 2014).
In this study, both univariate and multivariate CPHR analyses were performed to establish a 5-lncRNA signature. This model showed high accuracy in both the “entire” and “primary datasets”. In contrast, our prognostic model outperformed the other prognostic features. Risk stratification analysis suggested that our prediction model applied to different subgroups. Finally, we employed GO and KEGG to detect the biological function of our predictive model. Our results seemingly explored how these 5 OS-related lncRNAs are involved in tumor progression. Finally, the lncRNA AL606489.1 showed a possible association with sex dimorphism.
Our prognostic model consisted of 5 LncRNAs, 4 (i.e., AC068228.1, SATB2-AS1, AC026355.1, AL606489.1) of which have been previously reported to be related to the prognosis of LUAD. For instance, SATB2-AS1 has been reported to promote tumor cell growth in osteosarcoma (Liu et al., 2017), and NSCLC (Wu et al., 2021). However, in colorectal cancer (Xu et al., 2019), SATB2-AS1 has the effect of inhibiting tumor cell metastasis. Similar to our result, AC026355.1 was reported to be an immune-related gene with tumor suppressor effects by Li et al. (Li et al., 2020) In past studies, AL606489.1 has been reported to be associated with autophagy (Liu et al., 2021), ferroptosis (Guo et al., 2021), cuproptosis (Mo et al., 2022) and pyroptosis (Li et al., 2018; Song et al., 2021) processes in LUAD tumor cells. LINC 01843 was first shown to be associated with LUAD progression. These reports provide a new direction for gene sequence studies in LUAD.
In the GO and KEGG analysis results, the mRNAs co-expressed with prognostic-associated lncRNAs were associated with processing and RNA transport in the nucleus, such as in the regulation of mRNA metabolic process and the regulation of RNA splicing. Past studies have demonstrated that one of the prognostic-related genes, SATB2-AS1, acts as a miR-299-3p sponge, promoted the development of NSCLC. The underlying mechanism is the promotion of tumor cell proliferation, cell cycle progression, and survival (Wu et al., 2021). Thus, the results of GO and KEGG seem to appropriately reflect the place of action that was associated with prognosis, lncRNA affects the prognostic effect in patients with LUAD.
In the risk stratification analysis, this predictive model showed a slightly better performance in male patients (p < 0.05) than in female patients (p = 0.08), which prompted us to further explore the reasons for this discrepancy.
In our study, AL606489.1 was highly expressed in males relative to that in females. Moreover, on the premise that there is no significant difference in the prognosis between males and females with LUAD, AL606489.1 exhibited high levels of OS association in male patients, while showing no significant OS association in female patients. Therefore, we suggest that AL606489.1 demonstrates a gender dimorphism in terms of the prognostic effects in patients with LUAD. Meanwhile, this difference of AL606489.1 expression in females compared to males may be why the 5-lncRNA signature did not show significance in females in Figure 5F.
A person’s gender is one of the key factors affecting the occurrence and development of cancer throughout his or her lifetime. In addition to the sex-specificity of ovarian cancer in women and prostate cancer in men, several tumors are associated with a significant sex bias in terms of incidence (Li et al., 2018), metastatic (Kim et al., 2020), prognosis (Song et al., 2021), and therapeutic efficacy (Freudenstein et al., 2020). As the attention to gender differences has increased, gender dimorphism has been mentioned in increasing studies (Yuan et al., 2016).
In LUAD, sex bias is also associated with patients’ acquired behavior. For instance, Henschke et al. reported that women smoking was associated with a higher risk of lung cancer compared to men smoking, but after diagnosis of lung cancer, they had better survival rates (Henschke et al., 2006). The difference in prognosis between male and female patients may be related to natural differences in hormone levels. Multiple studies have demonstrated that sexual dimorphism may be due to differences in the estrogen content between men and women, which develops into different prognostic effects between male and female patients with LUAD. For example, LncRNA LINC00263 has been implicated as an oncogene in men and estrogen by Liu et al. (Liu et al., 2020). However, the specific role of lncRNA in sex dimorphism has not been well studied. In the present case, AL606489.1 can hence be a breakthrough.
In our study, the action mechanism of AL606489.1 was explored by co-expression analyses. In the co-expression analysis, AL606489.1 was found to be highly correlated with the sarcolemmal membrane-associated protein (SLMAP) expression (correlation coefficient = 0.64) (Supplementary Table S5). A subform of the SLMAP has been reported to be a component of the microtubule (Mt) tissue center (Guzzo et al., 2004). Mts is an important therapeutic target for tumor cells (Dumontet and Jordan, 2010). Clinically, some compounds that break Mt dynamics are also some of the most effective chemotherapeutics for cancer, such as vincristine alkaloids and taxanes (Checchi et al., 2003). Similarly, the mt-targeted drugs (MTDs) form a major family of anticancer drugs with anti-mitotic and antiangiogenic properties that inhibit tumor progression, mainly by changing the Mt dynamics of the tumor and endothelial cells (Bhat and Setaluri, 2007). However, there are no reliable markers that can be used for the prediction of the development of cancer sensitivity and resistance during treatment. In this study, AL606489.1 was found to be highly co-expressed with SLMAP and highly correlated with LUAD prognosis, indicating its potential as a reliable marker. Alternatively, the differential expression of AL606489.1 in males and females may be responsible for the clinical emergence of sex-differential efficacy of anticancer drugs that disrupt the Mt dynamics (Moore et al., 2003).
The limitations of the present study include the lack of external validation considering that the most lncRNAs required in this study were inaccessible in the GEO database. Second, as RNA testing in the TCGA database is constantly updated, this study is slightly sample-limited. Finally, the preliminary conclusion that AL606489.1 demonstrates sexual dimorphism, as derived in this study, needs to be further validated through in vitro and in vivo biological experiments, if the external conditions support it.
Our 5-lncRNA signature (composed of AC068228.1, SATB2-AS1, LINC01843, AC026355.1, and AL606489.1) could effectively predict the OS of LUAD patients, indicating its positive role in early screening and prognosis prediction of LUAD. Moreover, AL606489.1 demonstrated gender dimorphism, thereby providing a new direction for mechanistic studies on sexual dimorphism.
Publicly available datasets were analyzed in this study. This data can be found here: https://portal.gdc.cancer.gov/. https://www.ncbi.nlm.nih.gov/geo/.
JL performed the statistical analysis and wrote the initial draft. WJ and HX provided advice and reviewed the manuscripts. All authors read and approved the final manuscript.
The authors gratefully acknowledge the free online resources from TCGA and GEO.
The authors declare that the research was conducted in the absence of any commercial or financial relationships that could be construed as a potential conflict of interest.
All claims expressed in this article are solely those of the authors and do not necessarily represent those of their affiliated organizations, or those of the publisher, the editors and the reviewers. Any product that may be evaluated in this article, or claim that may be made by its manufacturer, is not guaranteed or endorsed by the publisher.
The Supplementary Material for this article can be found online at: https://www.frontiersin.org/articles/10.3389/fgene.2022.1052092/full#supplementary-material
LUAD, lung adenocarcinoma; lncRNAs, long noncoding RNAs; OS, overall survival; GO, Gene Ontology; KEGG, Kyoto Encyclopedia of Genes and Genomes; TCGA, The Cancer Genome Atlas; DEL, differentially expressed lncRNA; FDR, False Discovery Rate; CPHR, Cox proportional hazards regression; AIC, Akaike information criterion; ROC, receiver operating characteristic; AUC, area under the time-dependent ROC curve; HR, hazard ratio; CI, confidence interval.
Bade, B. C., and Cruz, C. S. D. (2020). Lung cancer 2020: Epidemiology, etiology, and prevention. Clin. Chest Med. 41 (1), 1–24. doi:10.1016/j.ccm.2019.10.001
Bhat, K. M., and Setaluri, V. (2007). Microtubule-associated proteins as targets in cancer chemotherapy. Clin. Cancer Res. 13 (10), 2849–2854. doi:10.1158/1078-0432.CCR-06-3040
Cech, T. R., and Steitz, J. A. (2014). The noncoding RNA revolution—Trashing old rules to forge new ones. Cell. 157 (1), 77–94. doi:10.1016/j.cell.2014.03.008
Chao, Y., and Zhou, D. (2019). lncRNA-D16366 is a potential biomarker for diagnosis and prognosis of hepatocellular carcinoma. Med. Sci. Monit. 25, 6581–6586. doi:10.12659/MSM.915100
Checchi, P. M., Nettles, J. H., Zhou, J., Snyder, J. P., and Joshi, H. C. (2003). Microtubule-interacting drugs for cancer treatment. Trends Pharmacol. Sci. 24 (7), 361–365. doi:10.1016/S0165-6147(03)00161-5
Chen, M., Liu, X., Du, J., Wang, X.-J., and Xia, L. (2017). Differentiated regulation of immune-response related genes between LUAD and LUSC subtypes of lung cancers. Oncotarget 8 (1), 133–144. doi:10.18632/oncotarget.13346
Cheng, Y. I., Gan, Y. C., Liu, D., Davies, M. P., Li, W. M., and Field, J. K. (2019). Potential genetic modifiers for somatic EGFR mutation in lung cancer: A meta-analysis and literature review. BMC cancer 19 (1), 1068–1117. doi:10.1186/s12885-019-6317-6
Collins, F. S., Morgan, M., and Patrinos, A. (2003). The human genome project: Lessons from large-scale biology. Science 300 (5617), 286–290. doi:10.1126/science.1084564
Couraud, S., Zalcman, G., Milleron, B., Morin, F., and Souquet, P.-J. (2012). Lung cancer in never smokers–a review. Eur. J. Cancer 48 (9), 1299–1311. doi:10.1016/j.ejca.2012.03.007
Davis, C. A., Hitz, B. C., Sloan, C. A., Chan, E. T., Davidson, J. M., Gabdank, I., et al. (2018). The Encyclopedia of DNA elements (ENCODE): Data portal update. Nucleic Acids Res. 46 (D1), D794–D801. doi:10.1093/nar/gkx1081
Dorak, M. T., and Karpuzoglu, E. (2012). Gender differences in cancer susceptibility: An inadequately addressed issue. Front. Genet. 3, 268. doi:10.3389/fgene.2012.00268
Dumontet, C., and Jordan, M. A. (2010). Microtubule-binding agents: A dynamic field of cancer therapeutics. Nat. Rev. Drug Discov. 9 (10), 790–803. doi:10.1038/nrd3253
Fong, K. M., Sekido, Y., and Minna, J. D. (1999). Molecular pathogenesis of lung cancer. J. Thorac. Cardiovasc. Surg. 118 (6), 1136–1152. doi:10.1016/S0022-5223(99)70121-2
Freudenstein, D., Litchfield, C., Caramia, F., Wright, G., Solomon, B. J., Ball, D., et al. (2020). TP53 status, patient sex, and the immune response as determinants of lung cancer patient survival. Cancers 12 (6), 1535. doi:10.3390/cancers12061535
Fungtammasan, A., Ananda, G., Hile, S. E., Su, M. S.-W., Sun, C., Harris, R., et al. (2015). Accurate typing of short tandem repeats from genome-wide sequencing data and its applications. Genome Res. 25 (5), 736–749. doi:10.1101/gr.185892.114
Guo, Y., Qu, Z., Li, D., Bai, F., Xing, J., Ding, Q., et al. (2021). Identification of a prognostic ferroptosis-related lncRNA signature in the tumor microenvironment of lung adenocarcinoma. Cell. Death Discov. 7 (1), 190–211. doi:10.1038/s41420-021-00576-z
Guzzo, R. M., Sevinc, S., Salih, M., and Tuana, B. S. (2004). A novel isoform of sarcolemmal membrane-associated protein (SLMAP) is a component of the microtubule organizing centre. J. Cell. Sci. 117 (11), 2271–2281. doi:10.1242/jcs.01079
Hauptman, N., and Glavač, D. (2013). Long non-coding RNA in cancer. Int. J. Mol. Sci. 14 (3), 4655–4669. doi:10.3390/ijms14034655
Henschke, C. I., Yip, R., and Miettinen, O. S. (2006). Women's susceptibility to tobacco carcinogens and survival after diagnosis of lung cancer. Jama 296 (2), 180–184. doi:10.1001/jama.296.2.180
Herbst, R. S., Morgensztern, D., and Boshoff, C. (2018). The biology and management of non-small cell lung cancer. Nature 553 (7689), 446–454. doi:10.1038/nature25183
Hyman, D. M., Smyth, L. M., Donoghue, M. T., Westin, S. N., Bedard, P. L., Dean, E. J., et al. (2017). AKT inhibition in solid tumors with AKT1 mutations. J. Clin. Oncol. 35 (20), 2251–2259. doi:10.1200/JCO.2017.73.0143
Jathar, S., Kumar, V., Srivastava, J., and Tripathi, V. (2017). Technological developments in lncRNA biology. Adv. Exp. Med. Biol. 1008, 283–323. doi:10.1007/978-981-10-5203-3_10
Jiang, M.-C., Ni, J.-J., Cui, W.-Y., Wang, B.-Y., and Zhuo, W. (2019). Emerging roles of lncRNA in cancer and therapeutic opportunities. Am. J. Cancer Res. 9 (7), 1354–1366. doi:10.3390/cancers13235970
Kanehisa, M., and Goto, S. (2000). Kegg: Kyoto Encyclopedia of genes and genomes. Nucleic Acids Res. 28 (1), 27–30. doi:10.1093/nar/28.1.27
Kanehisa, M., Furumichi, M., Sato, Y., Ishiguro-Watanabe, M., and Tanabe, M. (2021). Kegg: Integrating viruses and cellular organisms. Nucleic Acids Res. 49 (D1), D545–D551. doi:10.1093/nar/gkaa970
Kanehisa, M. (2019). Toward understanding the origin and evolution of cellular organisms. Protein Sci. 28 (11), 1947–1951. doi:10.1002/pro.3715
Kim, S. Y., Song, H. K., Lee, S. K., Kim, S. G., Woo, H. G., Yang, J., et al. (2020). Sex-biased molecular signature for overall survival of liver cancer patients. Biomol. Ther. 28 (6), 491–502. doi:10.4062/biomolther.2020.157
Leung, A. W., de Silva, T., Bally, M. B., and Lockwood, W. W. (2016). Synthetic lethality in lung cancer and translation to clinical therapies. Mol. Cancer 15 (1), 61–16. doi:10.1186/s12943-016-0546-y
Li, C. H., Haider, S., Shiah, Y.-J., Thai, K., and Boutros, P. C. (2018). Sex differences in cancer driver genes and biomarkers. Cancer Res. 78 (19), 5527–5537. doi:10.1158/0008-5472.CAN-18-0362
Li, J.-P., Li, R., Liu, X., Huo, C., Liu, T.-T., Yao, J., et al. (2020). A seven immune-related lncRNAs model to increase the predicted value of lung Adenocarcinoma. Front. Oncol. 10, 560779. doi:10.3389/fonc.2020.560779
Li, X., and Hemminki, K. (2004). Inherited predisposition to early onset lung cancer according to histological type. Int. J. Cancer 112 (3), 451–457. doi:10.1002/ijc.20436
Li, X., Wu, Z., Fu, X., and Han, W. (2014). lncRNAs: insights into their function and mechanics in underlying disorders. Mutat. Res. Rev. Mutat. Res. 762, 1–21. doi:10.1016/j.mrrev.2014.04.002
Liu, B., Zhao, Y., and Yang, S. (2021). A five autophagy-related long non-coding RNA prognostic model for patients with lung adenocarcinoma. Int. J. Gen. Med. 14, 7145–7158. doi:10.2147/IJGM.S334601
Liu, S.-H., Zhu, J.-W., Xu, H.-H., Zhang, G.-Q., Wang, Y., Liu, Y.-M., et al. (2017). A novel antisense long non-coding RNA SATB2-AS1 overexpresses in osteosarcoma and increases cell proliferation and growth. Mol. Cell. Biochem. 430 (1-2), 47–56. doi:10.1007/s11010-017-2953-9
Liu, S., Lai, W., Shi, Y., Liu, N., Ouyang, L., Zhang, Z., et al. (2020). Annotation and cluster analysis of long noncoding RNA linked to male sex and estrogen in cancers. NPJ Precis. Oncol. 4 (1), 5–13. doi:10.1038/s41698-020-0110-5
Lokhandwala, P. M., Tseng, L.-H., Rodriguez, E., Zheng, G., Pallavajjalla, A., Gocke, C. D., et al. (2019). Clinical mutational profiling and categorization of BRAF mutations in melanomas using next generation sequencing. BMC cancer 19 (1), 665–710. doi:10.1186/s12885-019-5864-1
Lu, L., Liu, L.-P., Zhao, Q.-Q., Gui, R., and Zhao, Q.-Y. (2021). Identification of a ferroptosis-related LncRNA signature as a novel prognosis model for lung adenocarcinoma. Front. Oncol. 11, 675545. doi:10.3389/fonc.2021.675545
Mo, X., Hu, D., Yang, P., Li, Y., Bashir, S., Nai, A., et al. (2022). A novel cuproptosis-related prognostic lncRNA signature and lncRNA MIR31HG/miR-193a-3p/TNFRSF21 regulatory axis in lung adenocarcinoma. Front. Oncol. 12, 927706. doi:10.3389/fonc.2022.927706
Moore, K. A., Mery, C. M., Jaklitsch, M. T., Estocin, A. P., Bueno, R., Swanson, S. J., et al. (2003). Menopausal effects on presentation, treatment, and survival of women with non–small cell lung cancer. Ann. Thorac. Surg. 76 (6), 1789–1795. doi:10.1016/S0003-4975(03)01024-5
Musolf, A. M., Simpson, C. L., De Andrade, M., Mandal, D., Gaba, C., Yang, P., et al. (2016). Parametric linkage analysis identifies five novel genome-wide significant loci for familial lung cancer. Hum. Hered. 82 (1-2), 64–74. doi:10.1159/000479028
Paz-Ares, L., Luft, A., Vicente, D., Tafreshi, A., Gumus, M., Mazieres, J., et al. (2018). Pembrolizumab plus chemotherapy for squamous non-small-cell lung cancer. N. Engl. J. Med. 379 (21), 2040–2051. doi:10.1056/NEJMoa1810865
Quinn, J. J., and Chang, H. Y. (2016). Unique features of long non-coding RNA biogenesis and function. Nat. Rev. Genet. 17 (1), 47–62. doi:10.1038/nrg.2015.10
Snyder, M. P., Gingeras, T. R., Moore, J. E., Weng, Z., Gerstein, M. B., Ren, B., et al. (2020). Perspectives on ENCODE. Nature 583 (7818), 693–698. doi:10.1038/s41586-020-2449-8
Song, J., Sun, Y., Cao, H., Liu, Z., Xi, L., Dong, C., et al. (2021). A novel pyroptosis-related lncRNA signature for prognostic prediction in patients with lung adenocarcinoma. Bioengineered 12 (1), 5932–5949. doi:10.1080/21655979.2021.1972078
Tamang, S., Acharya, V., Roy, D., Sharma, R., Aryaa, A., Sharma, U., et al. (2019). SNHG12: An LncRNA as a potential therapeutic target and biomarker for human cancer. Front. Oncol. 9, 901. doi:10.3389/fonc.2019.00901
Tomczak, K., Czerwińska, P., and Wiznerowicz, M. (2015). The cancer genome Atlas (TCGA): An immeasurable source of knowledge. Contemp. Oncol. 19 (1A), A68–A77. doi:10.5114/wo.2014.47136
Wang, Y., Wang, Y., Li, J., Li, J., and Che, G. (2020). Clinical significance of PIK3CA gene in non-small-cell lung cancer: A systematic review and meta-analysis. Biomed. Res. Int. 2020, 3608241. doi:10.1155/2020/3608241
Wu, X., Lu, J., Chen, W., Liang, M., Wang, N., andamxpbjI4NEAxNjMuY29tLA== Y. Z. (2021). SATB2-AS1 acts as miR-299-3p sponge to facilitate tumorigenesis in human non-small cell lung cancer. Arch. Med. Sci. doi:10.5114/aoms/119168
Wu, Y.-L., Cheng, Y., Zhou, J., Lu, S., Zhang, Y., Zhao, J., et al. (2020). Tepotinib plus gefitinib in patients with EGFR-mutant non-small-cell lung cancer with MET overexpression or MET amplification and acquired resistance to previous EGFR inhibitor (INSIGHT study): An open-label, phase 1b/2, multicentre, randomised trial. Lancet. Respir. Med. 8 (11), 1132–1143. doi:10.1016/S2213-2600(20)30154-5
Xu, M., Xu, X., Pan, B., Chen, X., Lin, K., Zeng, K., et al. (2019). LncRNA SATB2-AS1 inhibits tumor metastasis and affects the tumor immune cell microenvironment in colorectal cancer by regulating SATB2. Mol. Cancer 18 (1), 135–216. doi:10.1186/s12943-019-1063-6
Xu, W., Zhou, G., Wang, H., Liu, Y., Chen, B., Chen, W., et al. (2020). Circulating lncRNA SNHG11 as a novel biomarker for early diagnosis and prognosis of colorectal cancer. Int. J. Cancer 146 (10), 2901–2912. doi:10.1002/ijc.32747
Yu, J., Wu, X., Huang, K., Zhu, M., Zhang, X., Zhang, Y., et al. (2019). Bioinformatics identification of lncRNA biomarkers associated with the progression of esophageal squamous cell carcinoma. Mol. Med. Rep. 19 (6), 5309–5320. doi:10.3892/mmr.2019.10213
Yuan, H., Li, Z.-M., Shao, J., Ji, W.-X., Xia, W., and Lu, S. (2017). FGF2/FGFR1 regulates autophagy in FGFR1-amplified non-small cell lung cancer cells. J. Exp. Clin. Cancer Res. 36 (1), 72–14. doi:10.1186/s13046-017-0534-0
Yuan, Y., Liu, L., Chen, H., Wang, Y., Xu, Y., Mao, H., et al. (2016). Comprehensive characterization of molecular differences in cancer between male and female patients. Cancer Cell. 29 (5), 711–722. doi:10.1016/j.ccell.2016.04.001
Zhang, S., Chen, Y., Tian, C., He, Y., Tian, Z., Wan, Y., et al. (2021). Dual-target inhibitors based on BRD4: Novel therapeutic approaches for cancer. Curr. Med. Chem. 28, 1775–1795. doi:10.2174/0929867327666200610174453
Zhang, Z., Qian, W., Wang, S., Ji, D., Wang, Q., Li, J., et al. (2018). Analysis of lncRNA-associated ceRNA network reveals potential lncRNA biomarkers in human colon adenocarcinoma. Cell. Physiol. biochem. 49 (5), 1778–1791. doi:10.1159/000493623
Keywords: long noncoding RNAs (lncRNAs), The Cancer Genome Atlas (TCGA), gender dimorphism, prognostic prediction, lung adenocarcinoma (LUAD)
Citation: Liang J, Jin W and Xu H (2022) An efficient five-lncRNA signature for lung adenocarcinoma prognosis, with AL606489.1 showing sexual dimorphism. Front. Genet. 13:1052092. doi: 10.3389/fgene.2022.1052092
Received: 23 September 2022; Accepted: 16 November 2022;
Published: 30 November 2022.
Edited by:
Fan Zhang, University of North Texas Health Science Center, United StatesReviewed by:
Kenichi Takayama, Tokyo Metropolitan Institute of Gerontology, JapanCopyright © 2022 Liang, Jin and Xu. This is an open-access article distributed under the terms of the Creative Commons Attribution License (CC BY). The use, distribution or reproduction in other forums is permitted, provided the original author(s) and the copyright owner(s) are credited and that the original publication in this journal is cited, in accordance with accepted academic practice. No use, distribution or reproduction is permitted which does not comply with these terms.
*Correspondence: Huaping Xu, eHVfaHVhX3BpbmdAMTI2LmNvbQ==
Disclaimer: All claims expressed in this article are solely those of the authors and do not necessarily represent those of their affiliated organizations, or those of the publisher, the editors and the reviewers. Any product that may be evaluated in this article or claim that may be made by its manufacturer is not guaranteed or endorsed by the publisher.
Research integrity at Frontiers
Learn more about the work of our research integrity team to safeguard the quality of each article we publish.