- Lifebit Biotech Limited, London, United Kingdom
Since the first sequencing of the human genome, associated sequencing costs have dramatically lowered, leading to an explosion of genomic data. This valuable data should in theory be of huge benefit to the global community, although unfortunately the benefits of these advances have not been widely distributed. Much of today’s clinical-genomic data is siloed and inaccessible in adherence with strict governance and privacy policies, with more than 97% of hospital data going unused, according to one reference. Despite these challenges, there are promising efforts to make clinical-genomic data accessible and useful without compromising security. Specifically, federated data platforms are emerging as key resources to facilitate secure data sharing without having to physically move the data from outside of its organizational or jurisdictional boundaries. In this perspective, we summarize the overarching progress in establishing federated data platforms, and highlight critical considerations on how they should be managed to ensure patient and public trust. These platforms are enabling global collaboration and improving representation of underrepresented groups, since sequencing efforts have not prioritized diverse population representation until recently. Federated data platforms, when combined with advances in no-code technology, can be accessible to the diverse end-users that make up the genomics workforce, and we discuss potential strategies to develop sustainable business models so that the platforms can continue to enable research long term. Although these platforms must be carefully managed to ensure appropriate and ethical use, they are democratizing access and insights to clinical-genomic data that will progress research and enable impactful therapeutic findings.
1 Introduction
Genomic technologies are rapidly advancing the integration of genomics into clinical care, with evidence demonstrating their role in disease diagnosis, drug discovery and targeted therapeutics (Green et al., 2020; Atutornu et al., 2022; Borle et al., 2022). Digital health records, next generation sequencing, and artificial intelligence (AI) are also leading to an explosion of health data (Asiimwe et al., 2021). As observed within research, increased sample size improves the potential for discovery: genome wide association studies (GWAS) are prime examples, where it has been shown that a 10-fold increase in sample size can lead to a 100-fold increase in identified loci with significant disease associations (Visscher et al., 2017). Due to their sensitive nature, clinical, phenotypic and omics datasets are primarily distributed and stored in siloed, inaccessible locations (Asiimwe et al., 2021; Garden, 2021); in a stark example, the World Economic Forum estimates that 97% of all hospital data goes untouched1.
Between nations there exists strict national regulatory frameworks governing the movement of patient data and limiting transfer between national jurisdictions, which poses a significant barrier when trying to access international datasets (Mitchell et al., 2020). Adding to the complexity of using such data to derive meaningful insights, it is well-recognized and unfortunate that most genomic data does not represent diverse populations. A lack of diverse representation in clinical-genomic datasets ultimately limits the clinical utility of genetic findings as low sample sizes are insufficiently powered to identify disease-causing variants for specific populations (Atutornu et al., 2022; Lee et al., 2022).
Despite these challenges, there are ongoing efforts to increase the useability of clinical, phenotypic and multi-omic data for diagnosing and treating disease. Federated data platforms are emerging as means to achieve data accessibility, useability and security while adhering to governance and privacy regulations (Saunders et al., 2019; Blomberg and Lauer, 2020; Nik-Zainal et al., 2022). In this perspective, we explore how federated models for data access and analysis and end-to-end platforms can help to facilitate genomic benefits sharing; democratizing access to global data assets and insights facilitates the linking of diverse datasets to improve representation. We describe successful examples of how federation is being adopted across research and healthcare settings and discuss ongoing challenges and recommendations. Moving forward, it is imperative to build upon these technologies to ensure breakthroughs in genomic medicine for all. Safe and secure access to usable, diverse genomic data is poised to rapidly progress research and benefit patients.
2 Overcoming secure data sharing via federated platforms
2.1 Federated biomedical data platforms are emerging worldwide
Federation, in its simplest terms, is a software process that allows multiple databases to function as one. Federated architecture is a technological blueprint that facilitates interoperability and information sharing between autonomous, decentralized organizations. Within a federated architecture, data will remain within appropriate jurisdictional boundaries, while metadata are centralized and searchable. This is an alternative to a model in which data is moved or duplicated then centrally housed. Federated architectures of individual organizations may be connected together into a federated data platform, enabling data access and computation for users across organizations. We consider full federation to occur when both data and compute access are federated over distributed compute and databases to allow querying and joint analyses over the data (Chaterji et al., 2019). However, there also exists the potential for partial federation (I and II), when either compute access or data access are federated and compute or databases are distributed (Table 1). This is distinct from federated learning, which has tackled this problem in the context of Machine Learning (ML) in healthcare—researchers can train machine algorithms collaboratively on dispersed data, including health records, without infringing on data governance legislations (Mandl and Kohane, 2015; Stephens et al., 2015; De Fauw et al., 2018; Rieke et al., 2020; Xu et al., 2021; Pati et al., 2022).
There is now an increasing prevalence of federated architectures to connect large-scale health data (Saunders et al., 2019; Blomberg and Lauer, 2020; Thorogood et al., 2021; Nik-Zainal et al., 2022). Given the sensitive nature of health data, it cannot be physically pooled or moved for legal and regulatory reasons. This poses a challenge for researchers who rely on access and sufficient sample size to progress research. National genomic programs are increasingly adopting platforms with federated architectures to bring together distributed national datasets (Stark et al., 2019). Australian Genomics is developing a federated repository of genomic and phenotypic data to bridge the gap between its national health system and state-funded genetic services (Stark et al., 2019). In Canada, each province has its own health data privacy legislation such that data generated in each province must follow provincial governance laws. The Canadian Distributed Infrastructure for Genomics (CanDIG) platform is tackling this with a fully distributed federated data model, enabling federated querying and analysis while making sure that local data governance laws are respected (Dursi et al., 2021).
Within Europe, initiatives such as ELIXIR are linking Europe’s leading research organizations to more easily find, share and analyze data (Saunders et al., 2019; Blomberg and Lauer, 2020). ELIXIR oversees sub-initiatives including the European Genome Archive (EGA) federated networks to enable access and sharing of genomic data. ELIXIR is further participating in the Beyond 1 Million genomes (B1MG)2, which aims to create a network of clinical and genomic data across Europe. At a global level, the Common Infrastructure for National Cohorts in Europe, Canada, and Africa (CINECA) project is working to federate data between cohorts and across continents (Dursi et al., 2021). Through employing federated architectures, each of these initiatives allow organizations to store and manage their data locally while researchers worldwide can access the data securely.
2.2 Important considerations in establishing federated platforms
Federated analysis integrates with the architectures described above so that disparate data can, once securely accessed, be analyzed in situ across multiple sites. Establishing federated architectures requires that computing environments, systems, devices and applications within and across organizational, regional and national boundaries are all interoperable—this means overcoming the differences in multiple healthcare reporting systems, which frequently use different data models and ontologies (Mulder et al., 2017; Stark et al., 2019). Health data exchange architectures, application programming interfaces (APIs) and standards can provide a common language and set of expectations to enable interoperability between systems or devices so that authorized researchers can access and share data regardless of when or where it originates (Thorogood et al., 2021)4.
International initiatives have come together to tackle the issue of interoperability in federated platforms. The Global Alliance for Genomics and Health (GA4GH) sets standards to promote the international sharing of genomic and health-related data, in part by setting interoperability standards and providing open-source APIs (Thorogood et al., 2021). The GO FAIR initiative aims to implement data principles in order to make it Findable, Accessible, Interoperable and Reusable (FAIR)5 and the Observational Health Data Sciences (OHDSI) community is developing open-source tools to implement a common data model for combining disparate datasets6. The importance of widespread interoperability is increasingly reflected in the long-term strategy and funding of research institutes; in the US, the National Institutes of Health (NIH) Cloud Platform Interoperability Effort (NCPI)7 is establishing and implementing guidelines and technical standards for a federated data ecosystem. In the UK, the UK Research and Innovation program has recently established the Data and Analytics Research Environments UK (DARE UK) program8 to design and deliver a more coordinated national data research infrastructure.
While data interoperability is hugely important for federated collaborations, the data must be of a high quality. As federated data platforms lower the barrier of access to data, there must be guidelines to ensure that the data utilized in analysis is of acceptable quality to yield reliable results. For example, low-quality sequencing reads are more likely to inaccurately call variants, which can derail research efforts; within the context of precision medicine efforts, this could even lead to inaccurate diagnoses. There is now a breadth of literature highlighting the importance of quality control within sequencing analysis (NCI-NHGRI Working Group on Replication in Association Studies, 2007; Miyagawa et al., 2008; Turner et al., 2011; DeLuca et al., 2012; Ma et al., 2019), with organizations such as ENCODE9 offering guidelines for appropriate sequencing coverage and quality controls. As federated data platforms continue to expand, it will be important that administrative authorities designate quality thresholds for data submission, and that these should be published within the metadata catalogs for researchers.
With the ability to process immense datasets, computational resources are an important consideration. The scale of distributed multi-omics and clinical datasets available today has brought an increasing shift towards commercial cloud infrastructure. The “elastic” nature of cloud computing means researchers only pay for what they need. Further, researchers can create near identical hardware and software setups remotely, regardless of whether they are near a data center (Langmead and Nellore, 2018). Cloud computing builds capacity for state-of-the-art capabilities in encryption, firewalls and monitoring. Despite this, there is still reticence towards adopting cloud computing for genomic data in some jurisdictions; it is not fully clear how existing privacy and data protection laws apply in the genomics context and as such there is a lack of community consensus on best practices (Dove et al., 2015). Defining standards and best practices, in addition to cloud companies providing transparency of security and technology infrastructure, will be essential to build trust across the industry and enable more organizations to harness the benefits of cloud computing.
Despite these advances, any computing environment that involves sensitive patient data is not without risk (Melis et al., 2018; Nasr et al., 2018). While data remains locally for federated architectures, there is still a component that is exchanged, such as intermediate ML models or aggregated results for federated learning and analysis, respectively. With federated learning, ML models can be susceptible to security risks such as inference attacks, feature leakage and data poisoning, which can result in the leakage of unintended information about participants’ training data (Melis et al., 2018; Nasr et al., 2018). Ongoing work is needed to investigate how parameters can be further protected and how the tradeoff between the privacy and security-level versus system performance and cost should be managed (Popovic, 2017). Likewise, federated models for data access introduce unique security risks, such as when new users or code are introduced into a data controller’s computing environment (Popovic, 2017). Careful logging and auditing of platform and user activity, as well as data/code export controls (e.g., airlocks10), are needed to monitor these risks.
2.3 Federation to promote global collaboration and representation in genomic datasets
To improve disease diagnostic capabilities for the greatest number of people, larger and more diverse cohorts are needed (Zoch et al., 2021). By facilitating international cooperation via secure data unification, federation can support more diverse population representation in genomic datasets (Vesteghem et al., 2020; Asiimwe et al., 2021; Garden, 2021; Powell, 2021; Zoch et al., 2021; Lee et al., 2022). In academic research, initiatives like Matchmaker Exchange (MME) are demonstrating how distributed datasets of genotypes and rare phenotypes can be combined using a federated network to facilitate rapid, secure data sharing to achieve faster diagnoses (Philippakis et al., 2015; Zoch et al., 2021). The Human Heredity and Health in Africa (H3Africa) initiative is promoting intra-continental collaborations to establish a network of African-based biorepositories (Abimiku et al., 2017; Mulder et al., 2017). Already, this program is highlighting deep regional variation for disease-related risk factors and has established critical tools (genotyping arrays and reference gene panel for imputation) that support the analysis of genetic data from individuals of African descent (Mulder et al., 2017).
Despite this progress, trust remains an important issue to recruit participants, especially in historically marginalized groups. As data custodians retain control over their dataset in a federated data access model, data access agreements must be negotiated in a manner that is acceptable for research participants to engender trust, particularly in historically underrepresented groups (Thorogood et al., 2021; Lee et al., 2022).
3 Democratizing access to data assets and insights via federated platforms
3.1 Considerations in democratizing genomic data
A core benefit of federated data platforms is that they can democratize access to health data in a secure manner. While this brings huge potential for advancing medical research, there must be strict regulations over how data is governed and accessed that are applied at the organizational- and researcher-level, in order to engender public and participant trust.
There is a valid concern of ownership over federated data platforms—a trusted independent party, a group of institutions, or the government could theoretically assume the role. In the United Kingdom, there is currently a concerted effort across the public sector towards the establishment of a federated, research data infrastructure11–13. In this model, patient data is stored in trusted research environments (TREs) or “secure data environments” and federated technology is used to virtually link these environments while data stays securely at its source, always within full control of the data custodian/controller. The TRE is fully owned and governed by the data controller(s)13; this means there is collective ownership across the multiple healthcare providers contributing to the data source.
In line with the surge in data regulations arising across global jurisdictions14–16, there is an increasing prevalence of accreditation schemes to audit and certify the “owner” of data management platforms14,17. To guarantee ethical and secure usage of federated platforms, the safety and governance of these infrastructures must be regularly reviewed and measured against all aspects relevant to data security and governance, from implementing industry-recognised data protection frameworks18, standards and information security measures to compliance with local data regulations and commitments to interoperability. Access to the data within these federated platforms must be appropriately reviewed and governed by the data controllers—identifying an efficient and secure process for approving access and democratization of this data is a community-wide work in progress. Implementing such governance and regulatory bodies that regulate the use of data can help foster trust in the wider public for genomics research among the wider public and ensure data use is in the interest of both the public and participants.
3.2 Enabling analytics via no/low-code tools and end-to-end platforms
The software industry is currently shifting towards “no/low-code” tools to support a wider range of end users with and without a data science background, thus enabling full democratization of access to genomic data and the insights derived. The Galaxy Community, an initiative within ELIXIR, is one such example offering a web-based platform to facilitate computational research for a variety of “omics” types (The Galaxy Community et al., 2022). There are also resources such as DepMap19 that offer easy-to-use graphical user interfaces to explore cancer vulnerabilities from available chemical and genetic perturbation data using analytical and visualization tools. Together, these tools enable users of diverse backgrounds to visualize the data directly or build reproducible pipelines and complex workflows for analyses.
While such low-/no-code tools are a huge first step, there should ideally be an end-to-end, federated solution for researchers as well as clinicians - providing the latter with the resources they require to understand their patients’ data (Kullo et al., 2013; Lau-Min et al., 2021). An end-to-end data platform, building upon the current advances of federated data architectures and capable of ingesting clinical and raw genomic data, can democratize access and accelerate the generation of clinically actionable insights. Such platforms could securely integrate between a country’s healthcare network, national genomic medicine initiatives and sequencing laboratories. When coupled with tools to enable anyone to run bioinformatic pipelines and workflows, such a platform could handle genetic services end-to-end: from patient recruitment, sample collection, sequencing, data standardization, analysis and clinical reporting (Stark et al., 2019) (Figure 1). By federating across distributed databases and systems as well as providing the necessary, easy to use tools to transform raw data into meaningful insights can bring more direct benefits to patients.
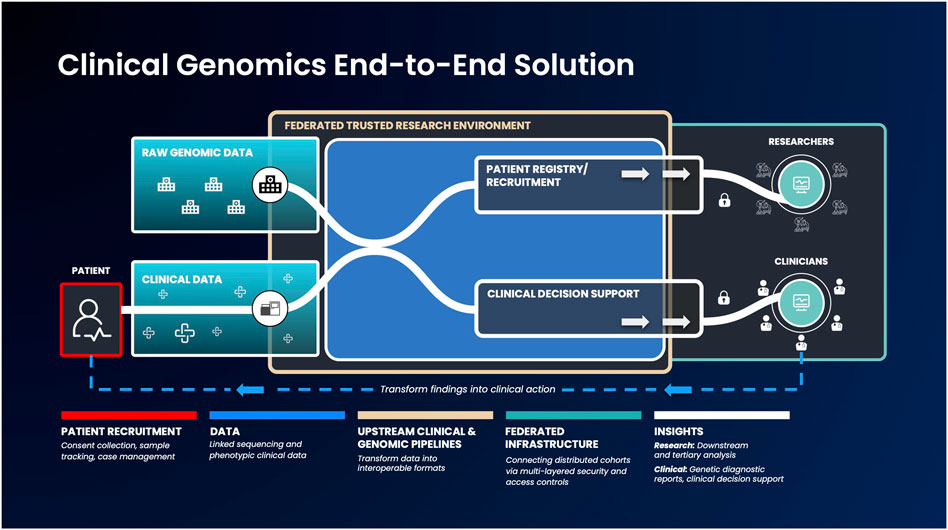
FIGURE 1. An example genomic medicine end-to-end solution that integrates federated architecture. Genomic or phenotypic clinical data is first collected and transformed into interoperable formats. Next, these data will be ingested into the federated architecture, which allows authorized users to access and combine this data with other disparate sources to build unique and valuable analysis cohorts. Strict security measures will facilitate results export to clinicians and researchers, to enable them progress therapeutic discovery and make informed clinical decisions.
3.3 Ensuring the sustainability of federation for future genomics research
While many countries are increasingly, and successfully, integrating genomics into healthcare (Stark et al., 2019; Kloypan et al., 2021), it is important to note that not all learnings described here are broadly applicable. Many countries and regions are faced with rapidly shifting health priorities and challenges including low levels of government support, absence of well-funded national healthcare systems, workforce skill shortages and gaps in infrastructure (Mulder et al., 2017; Stark et al., 2019; Maxmen, 2020). Data sharing, even in a fully federated system, is associated with significant costs (Chalmers et al., 2016)20. The long-term sustainability of the genomics ecosystem is reliant on more sustainable solutions and secure, long-term funding, something that will only be achieved through industry-wide collaboration.
Collaboration between biobanks and the broader life sciences industry can build larger and more representative data ecosystems and open sustainable funding mechanisms for population genomics initiatives and biobanks, particularly in countries with fewer resources for research. Specifically, extending collaboration into the private sector, biobanks can accelerate growth with highly lucrative and sustainable funding. There is increasing recognition among pharmaceutical companies that diversity among the patient-participant population of clinical trials is critical given large genetic variability in drug responses that is often correlated with ancestry (Gross et al., 2022).
As the private sectors will not freely disseminate their knowledge, there is a model by which genomic initiatives and biobanks can negotiate data access agreements with pharmaceutical companies who require large and diverse patient cohorts for R&D and drug discovery pipelines (Garden, 2021; Thorogood et al., 2021). An example is that of 54 Gene, a venture capital-backed biobank based in Nigeria, which will partner with pharmaceutical companies to fund its research by charging access fees, like the UK Biobank (Maxmen, 2020). By generating stable and sustainable funding mechanisms through collaborative partnerships, biobanks and precision medicine programs can generate holistic benefits sharing at scale (Maxmen, 2020; Thorogood et al., 2021; Bedeker et al., 2022).
4 Discussion
Here, we have presented a perspective on the overarching progress to develop federated data platforms to enable research and genomics efforts. While there has been significant progress within national and international endeavors to provide secure access to their large-scale health data, as well as tools to empower users to derive meaningful insights, frameworks and policies guiding the genomics community on best practices for data sharing are necessary to ensure successful collaboration. These must cover critical considerations discussed in this perspective including interoperability, secure data access, cloud computing, usability, democratized data access, clinical utility, ethical considerations and sustainability of the platforms (Thorogood et al., 2021; Lee et al., 2022). Governing agencies are indeed beginning to address the complexities associated with data sharing—the World Health Organization’s recent report serving as a notable example (WHO, 2022) Within a federated ecosystem, there are roles for the private and public sectors. In this perspective, we have highlighted an opportunity for pharma to invest in biobanking and federated data platforms in order to increase their access to data, which in turn funds the platforms. Further, it may be important to consider moving forward the role of DNA testing companies in building federated networks. These companies have access to the data of millions of individuals, and it will be interesting to determine whether there are any incentives for these to join the federated data ecosystems, while also adhering to governance and privacy policies.
Finally, continued democratization of data access and analysis has the potential to broaden the reach for innovative technologies (Drake et al., 2018; Christopher et al., 2021). Future efforts to expand federated data platforms in an ethical manner will require broad coordination between non-governmental organizations, local governments, scientific researchers and industry to advocate for increased investments to build capacity and improve infrastructure21. Evolving federated data platforms, such as those discussed here, are already accelerating research by drawing research communities together to benefit patients. Further investment in and expansion of such sustainable platforms will continue to power research so that access and usability of data will no longer be a barrier to discovering powerful therapeutic insights.
Data availability statement
The original contributions presented in the study are included in the article/Supplementary Material, further inquiries can be directed to the corresponding author.
Author contributions
MA, CD, IK, TS, NR, PP, and MC contributed equally to the conception of the article. MA and IK wrote the first draft of the manuscript, HES and IK wrote the second draft of the manuscript. CD wrote sections of the manuscript. All authors contributed to manuscript revision, read, and approved the submitted version.
Acknowledgments
The authors would like to thank Divya Narasimhan for her initial contributions to the manuscript.
Conflict of interest
Authors MA, HES, IK, CD, NR, TS, PP, and MC are employed by Lifebit Biotech Limited.
Publisher’s note
All claims expressed in this article are solely those of the authors and do not necessarily represent those of their affiliated organizations, or those of the publisher, the editors and the reviewers. Any product that may be evaluated in this article, or claim that may be made by its manufacturer, is not guaranteed or endorsed by the publisher.
Footnotes
1World Economic Forum. 4 ways data is improving healthcare (2019). World Economic Forum. https://www.weforum.org/agenda/2019/12/four-ways-data-is-improving-healthcare/ [Accessed 27 July 2022].
2Beyond One Million Genomes Project (2022) https://b1mg-project.eu/ [Accessed 13 December 2022].
3Trustworthy Federated Data Analytics (TFDA) (2022). https://tfda.hmsp.center/ [Accessed 5 December 2022].
4Healthcare Information and Management Systems Society (2020). Interoperability in Healthcare. https://www.himss.org/resources/interoperability-healthcare [Accessed 16 August 2022].
5GO FAIR Initiative (2017). The GO FAIR Initiative. https://www.go-fair.org/go-fair-initiative/ [Accessed 16 August 2022].
6Observational Health Data Sciences and Informatics (2022). OMOP Common Data Model. https://www.ohdsi.org/data-standardization/the-common-data-model/ [Accessed 16 August 2022].
7NIH Cloud Platform Interoperability Effort (2022) https://datascience.nih.gov/nih-cloud-platform-interoperability-effort [Accessed 16 August 2022].
8Data and Analytics Research Environments UK (2021). https://dareuk.org.uk/about/ [Accessed 16 August 2022].
9ENCODE. (2022) https://www.encodeproject.org/ [Accessed 13 December 2022].
10Importing and exporting files using the Airlock (2022). https://re-docs.genomicsengland.co.uk/airlock/#importing-and-exporting-files-using-the-airlock [Accessed 13 December 2022].
11Genome UK: 2021 to 2022 implementation plan (2021) https://www.gov.uk/government/publications/genome-uk-2021-to-2022-implementation-plan [Accessed 16 August 2022].
12Better, broader, safer: using health data for research and analysis (2022) https://www.gov.uk/government/publications/better-broader-safer-using-health-data-for-research-and-analysis [Accessed 16 August 2022].
13Secure data environment for NHS health and social care data—policy guidelines (2022). https://www.gov.uk/government/publications/secure-data-environment-policy-guidelines/secure-data-environment-for-nhs-health-and-social-care-data-policy-guidelines [Accessed 13 August 2022].
14NIH Data Management and Sharing Policy (2022). https://sharing.nih.gov/data-management-and-sharing-policy [Accessed 13 December 2022].
15General Data Protection Regulation (2022). https://gdpr-info.eu/ [Accessed 13 December 2022].
16CS/HB 833 — Unlawful Use of DNA (2021). https://www.flsenate.gov/Committees/billsummaries/2021/html/2543 [Accessed 13 December 2022].
17Our Future Health opens consultation on trusted research environment accreditation process (2022). https://ourfuturehealth.org.uk/news/our-future-health-opens-consultation-on-trusted-research-environment-accreditation-process/ [Accessed 13 December 2022].
18What is the Five Safes framework? (2022) https://ukdataservice.ac.uk/help/secure-lab/what-is-the-five-safes-framework/ [Accessed 13 December 2022].
19Explore the Cancer Dependency Map (2021). https://depmap.org/portal/ [Accessed 13 December 2022].
20Network Computing (2019). 3 Hidden Public Cloud Costs and How to Avoid Them. https://www.networkcomputing.com/cloud-infrastructure/3-hidden-public-cloud-costs-and-how-avoid-them [Accessed 16 August 2022].
21World Health Organization (2022). WHO’s Science Council launches report calling for equitable expansion of genomics. https://www.who.int/news/item/12-07-2022-who-s-science-council-launches-report-calling-for-equitable-expansion-of-genomics. [Accessed 28 July 2022].
References
Abimiku, A., Mayne, E. S., Joloba, M., Beiswanger, C. M., Troyer, J., and Wideroff, L.H3Africa Biorepository Working Grou (2017). H3Africa biorepository program: Supporting genomics research on african populations by sharing high-quality biospecimens. Biopreservation Biobanking 15, 99–102. doi:10.1089/bio.2017.0005
The Galaxy Community Afgan, E., Nekrutenko, A., Grüning, B. A., Blankenberg, D., Goecks, J., Schatz, M. C., et al. (2022). The Galaxy platform for accessible, reproducible and collaborative biomedical analyses: 2022 update. Nucleic Acids Res. 50, W345–W351. doi:10.1093/nar/gkac247
Asiimwe, R., Lam, S., Leung, S., Wang, S., Wan, R., Tinker, A., et al. (2021). From biobank and data silos into a data commons: Convergence to support translational medicine. J. Transl. Med. 19, 493. doi:10.1186/s12967-021-03147-z
Atutornu, J., Milne, R., Costa, A., Patch, C., and Middleton, A. (2022). Towards equitable and trustworthy genomics research. eBioMedicine 76, 103879. doi:10.1016/j.ebiom.2022.103879
Bedeker, A., Nichols, M., Allie, T., Tamuhla, T., van Heusden, P., Olorunsogbon, O., et al. (2022). A framework for the promotion of ethical benefit sharing in health research. BMJ Glob. Health 7, e008096. doi:10.1136/bmjgh-2021-008096
Blomberg, N., and Lauer, K. B. (2020). Connecting data, tools and people across Europe: ELIXIR’s response to the COVID-19 pandemic. Eur. J. Hum. Genet. 28, 719–723. doi:10.1038/s41431-020-0637-5
Borle, K., Kopac, N., Dragojlovic, N., Rodriguez Llorian, E., Friedman, J. M., Elliott, A. M., et al. GenCOUNSEL Study (2022). Where is genetic medicine headed? Exploring the perspectives of Canadian genetic professionals on future trends using the delphi method. Eur. J. Hum. Genet. 30, 496–504. doi:10.1038/s41431-021-01017-2
Chalmers, D., Nicol, D., Kaye, J., Bell, J., Campbell, A. V., Ho, C. W. L., et al. (2016). Has the biobank bubble burst? Withstanding the challenges for sustainable biobanking in the digital era. BMC Med. Ethics 17, 39. doi:10.1186/s12910-016-0124-2
NCI-NHGRI Working Group on Replication in Association Studies Chanock, S. J., Manolio, T., Boehnke, M., Boerwinkle, E., Hunter, D. J., et al. (2007). Replicating genotype–phenotype associations. Nature 447, 655–660. doi:10.1038/447655a
Chaterji, S., Koo, J., Li, N., Meyer, F., Grama, A., and Bagchi, S. (2019). Federation in genomics pipelines: Techniques and challenges. Brief. Bioinform 20, 235–244. doi:10.1093/bib/bbx102
Christopher, H., Burns, A., Josephat, E., Makani, J., Schuh, A., and Nkya, S. (2021). Using DNA testing for the precise, definite, and low-cost diagnosis of sickle cell disease and other haemoglobinopathies: Findings from Tanzania. BMC Genomics 22, 902. doi:10.1186/s12864-021-08220-x
De Fauw, J., Ledsam, J. R., Romera-Paredes, B., Nikolov, S., Tomasev, N., Blackwell, S., et al. (2018). Clinically applicable deep learning for diagnosis and referral in retinal disease. Nat. Med. 24, 1342–1350. doi:10.1038/s41591-018-0107-6
DeLuca, D. S., Levin, J. Z., Sivachenko, A., Fennell, T., Nazaire, M.-D., Williams, C., et al. (2012). RNA-SeQC: RNA-seq metrics for quality control and process optimization. Bioinforma. Oxf. Engl. 28, 1530–1532. doi:10.1093/bioinformatics/bts196
Dove, E. S., Joly, Y., Tassé, A.-M., and Knoppers, B. M. (2015). Public population project in genomics and society (P3G) international steering committee, international cancer genome consortium (ICGC) ethics and policy CommitteeGenomic cloud computing: Legal and ethical points to consider. Eur. J. Hum. Genet. EJHG 23, 1271–1278. doi:10.1038/ejhg.2014.196
Drake, T. M., Knight, S. R., Harrison, E. M., and Søreide, K. (2018). Global inequities in precision medicine and molecular cancer research. Front. Oncol. 8, 346. doi:10.3389/fonc.2018.00346
Dursi, L. J., Bozoky, Z., de Borja, R., Li, H., Bujold, D., Lipski, A., et al. (2021). CanDIG: Federated network across Canada for multi-omic and health data discovery and analysis. Cell Genomics 1, 100033. doi:10.1016/j.xgen.2021.100033
Garden, H. (2021). Building and sustaining collaborative platforms in genomics and biobanks for health innovation (OECD Science, Technology and Industry Policy Papers No. 102). OECD Sci. Technol. Industry Policy Pap 102. doi:10.1787/11d960b7-en
Green, E. D., Gunter, C., Biesecker, L. G., Di Francesco, V., Easter, C. L., Feingold, E. A., et al. (2020). Strategic vision for improving human health at the Forefront of Genomics. Nature 586, 683–692. doi:10.1038/s41586-020-2817-4
Gross, A. S., Harry, A. C., Clifton, C. S., and Della Pasqua, O. (2022). Clinical trial diversity: An opportunity for improved insight into the determinants of variability in drug response. Br. J. Clin. Pharmacol. 88, 2700–2717. doi:10.1111/bcp.15242
Kloypan, C., Koomdee, N., Satapornpong, P., Tempark, T., Biswas, M., and Sukasem, C. (2021). A comprehensive review of HLA and severe cutaneous adverse drug reactions: Implication for clinical pharmacogenomics and precision medicine. Pharmaceuticals 14, 1077. doi:10.3390/ph14111077
Kullo, I. J., Jarvik, G. P., Manolio, T. A., Williams, M. S., and Roden, D. M. (2013). Leveraging the electronic health record to implement genomic medicine. Genet. Med. 15, 270–271. doi:10.1038/gim.2012.131
Langmead, B., and Nellore, A. (2018). Cloud computing for genomic data analysis and collaboration. Nat. Rev. Genet. 19, 208–219. doi:10.1038/nrg.2017.113
Lau-Min, K. S., Asher, S. B., Chen, J., Domchek, S. M., Feldman, M., Joffe, S., et al. (2021). Real-world integration of genomic data into the electronic health record: The PennChart genomics initiative. Genet. Med. 23, 603–605. doi:10.1038/s41436-020-01056-y
Lee, S. S.-J., Appelbaum, P. S., and Chung, W. K. (2022). Challenges and potential solutions to health disparities in genomic medicine. Cell 185, 2007–2010. doi:10.1016/j.cell.2022.05.010
Ma, X., Shao, Y., Tian, L., Flasch, D. A., Mulder, H. L., Edmonson, M. N., et al. (2019). Analysis of error profiles in deep next-generation sequencing data. Genome Biol. 20, 50. doi:10.1186/s13059-019-1659-6
Mandl, K. D., and Kohane, I. S. (2015). Federalist principles for healthcare data networks. Nat. Biotechnol. 33, 360–363. doi:10.1038/nbt.3180
Maxmen, A. (2020). The next chapter for African genomics. Nature 578, 350–354. doi:10.1038/d41586-020-00454-1
Melis, L., Song, C., De Cristofaro, E., and Shmatikov, V. (2018). Exploiting unintended feature leakage in collaborative learning. Vancuver, BC: International Conference on Learning Representations. doi:10.48550/ARXIV.1805.04049
Mitchell, C., Ordish, J., Johnson, E., Brigden, T., and Hall, A. (2020). The GDPR and genomic data. Cambridge: PHG Foundation.
Miyagawa, T., Nishida, N., Ohashi, J., Kimura, R., Fujimoto, A., Kawashima, M., et al. (2008). Appropriate data cleaning methods for genome-wide association study. J. Hum. Genet. 53, 886–893. doi:10.1007/s10038-008-0322-y
Mulder, N., Adebamowo, C. A., Adebamowo, S. N., Adebayo, O., Adeleye, O., Alibi, M., et al. (2017). Genomic research data generation, analysis and sharing – challenges in the african setting. Data Sci. J. 16, 49. doi:10.5334/dsj-2017-049
Nasr, M., Shokri, R., and Houmansadr, A. (2018). Comprehensive privacy analysis of deep learning: Passive and active white-box inference attacks against centralized and federated learning. San Francisco, CA: IEEE Symposium on Security and Privacy. doi:10.48550/ARXIV.1812.00910
Nik-Zainal, P. S., Seeger, T., Fennessy, R., Hall, E., Moss, P., Coles, G., et al. (2022). Multi-party trusted research environment federation: Establishing infrastructure for secure analysis across different clinical-genomic datasets. Zenodo. doi:10.5281/ZENODO.7085536
Pati, S., Baid, U., Edwards, B., Sheller, M., Wang, S.-H., Reina, G. A., et al. (2022). Federated learning enables big data for rare cancer boundary detection. Nat. Commun. 13, 7346. doi:10.1038/s41467-022-33407-5
Philippakis, A. A., Azzariti, D. R., Beltran, S., Brookes, A. J., Brownstein, C. A., Brudno, M., et al. (2015). The matchmaker exchange: A platform for rare disease gene discovery. Hum. Mutat. 36, 915–921. doi:10.1002/humu.22858
Popovic, J. R. (2017). Distributed data networks: A blueprint for big data sharing and healthcare analytics. Ann. N. Y. Acad. Sci. 1387, 105–111. doi:10.1111/nyas.13287
Powell, K. (2021). The broken promise that undermines human genome research. Nature 590, 198–201. doi:10.1038/d41586-021-00331-5
Rieke, N., Hancox, J., Li, W., Milletarì, F., Roth, H. R., Albarqouni, S., et al. (2020). The future of digital health with federated learning. Npj Digit. Med. 3, 119. doi:10.1038/s41746-020-00323-1
Saunders, G., Baudis, M., Becker, R., Beltran, S., Béroud, C., Birney, E., et al. (2019). Leveraging European infrastructures to access 1 million human genomes by 2022. Nat. Rev. Genet. 20, 693–701. doi:10.1038/s41576-019-0156-9
Stark, Z., Dolman, L., Manolio, T. A., Ozenberger, B., Hill, S. L., Caulfied, M. J., et al. (2019). Integrating genomics into healthcare: A global responsibility. Am. J. Hum. Genet. 104, 13–20. doi:10.1016/j.ajhg.2018.11.014
Stephens, Z. D., Lee, S. Y., Faghri, F., Campbell, R. H., Zhai, C., Efron, M. J., et al. (2015). Big data: Astronomical or genomical? PLoS Biol. 13, e1002195. doi:10.1371/journal.pbio.1002195
Thorogood, A., Rehm, H. L., Goodhand, P., Page, A. J. H., Joly, Y., Baudis, M., et al. (2021). International federation of genomic medicine databases using GA4GH standards. Cell Genomics 1, 100032. doi:10.1016/j.xgen.2021.100032
Turner, S., Armstrong, L. L., Bradford, Y., Carlson, C. S., Crawford, D. C., Crenshaw, A. T., et al. (2011). Quality control procedures for genome-wide association studies. Curr. Protoc. Hum. Genet. Chapter 1, Unit1.19. doi:10.1002/0471142905.hg0119s68
Vesteghem, C., Brøndum, R. F., Sønderkær, M., Sommer, M., Schmitz, A., Bødker, J. S., et al. (2020). Implementing the FAIR data principles in precision oncology: Review of supporting initiatives. Brief. Bioinform. 21, 936–945. doi:10.1093/bib/bbz044
Visscher, P. M., Wray, N. R., Zhang, Q., Sklar, P., McCarthy, M. I., Brown, M. A., et al. (2017). 10 Years of GWAS discovery: Biology, function, and translation. Am. J. Hum. Genet. 101, 5–22. doi:10.1016/j.ajhg.2017.06.005
WHO (2022). Accelerating access to genomics for global health: Promotion, implementation, collaboration, and ethical, legal, and social issues. Geneva: A report of the WHO Science CouncilWorld Health Organization.
Xu, J., Glicksberg, B. S., Su, C., Walker, P., Bian, J., and Wang, F. (2021). Federated learning for healthcare Informatics. J. Healthc. Inf. Res. 5, 1–19. doi:10.1007/s41666-020-00082-4
Zoch, M., Gierschner, C., Peng, Y., Gruhl, M., Leutner, Liz.A., Sedlmayr, M., et al. (2021). “Adaption of the OMOP CDM for rare diseases,” in Studies in health technology and Informatics. Editors J. Mantas, L. Stoicu-Tivadar, C. Chronaki, A. Hasman, P. Weber, P. Galloset al. (Amsterdam: IOS Press). doi:10.3233/SHTI210136
Keywords: federation, genomics, cloud computing, trusted research environment, clinical genomics
Citation: Alvarellos M, Sheppard HE, Knarston I, Davison C, Raine N, Seeger T, Prieto Barja P and Chatzou Dunford M (2023) Democratizing clinical-genomic data: How federated platforms can promote benefits sharing in genomics. Front. Genet. 13:1045450. doi: 10.3389/fgene.2022.1045450
Received: 15 September 2022; Accepted: 19 December 2022;
Published: 10 January 2023.
Edited by:
Dominique Sprumont, University of Neuchâtel, SwitzerlandReviewed by:
Apostolos Pyrgelis, Swiss Federal Institute of Technology Lausanne, SwitzerlandCopyright © 2023 Alvarellos, Sheppard, Knarston, Davison, Raine, Seeger, Prieto Barja and Chatzou Dunford. This is an open-access article distributed under the terms of the Creative Commons Attribution License (CC BY). The use, distribution or reproduction in other forums is permitted, provided the original author(s) and the copyright owner(s) are credited and that the original publication in this journal is cited, in accordance with accepted academic practice. No use, distribution or reproduction is permitted which does not comply with these terms.
*Correspondence: Ingrid Knarston, ingrid@lifebit.ai
†These authors have contributed equally to this work and share first authorship