- 1Heping Hospital Affiliated to Changzhi Medical College, Changzhi, Shanxi, China
- 2Department of Orthopaedics, Heping Hospital Affiliated to Changzhi Medical College, Changzhi, Shanxi, China
- 3Institute of Evidence-Based Medicine, Heping Hospital Affiliated to Changzhi Medical College, Changzhi, Shanxi, China
- 4Department of Epidemiology, School of Public Health, Southern Medical University, Guangzhou, China
Background: Numerous studies have demonstrated an association between osteoprotegerin (OPG) polymorphisms (A163G (rs3102735), T245G (rs3134069), T950C (rs2073617), G1181C (rs2073618)) and osteoporosis risk. However, their conclusions are inconsistent. In addition, some new studies have been updated, and more importantly, previous meta-analyses have not tested for false-positive results. In order to further explore these associations, we recently conducted a meta-analysis.
Objectives: To study the relationship between OPG polymorphisms A163G, T245G, T950C, G1181C and the risk of osteoporosis.
Methods: PubMed, Medline, International Statistical Institute (ISI), China National Knowledge Infrastructure (CNKI) and China Wanfang Database were used for research searches. Associations were assessed with five genetic models using odds ratios (ORs) with 95% confidence intervals (CIs). In addition, confidence in statistically significant associations was assessed using false-positive report probability (FPRP), Bayesian probability of False discovery (BFDP), and Venice criteria.
Results: On the whole, the OPG A163G polymorphism was not significantly associated with risk of osteoporosis. However, in a subgroup analysis, we found that the OPG A163G polymorphism increased the risk of osteoporosis in Caucasians (AG + GG vs AA: OR = 1.35, 95% CI = 1.06–1.73; AA + GG vs AG: OR = 0.64, 95% CI = 0.49–0.82) and the female (G vs A: OR = 1.30, 95% CI = 1.03–1.64; AG + GG vs AA: OR = 1.42, 95% CI = 1.18–1.71). At the same time, the OPG G1181C polymorphism reduces the risk of osteoporosis (C vs G: OR = 0.84, 95% CI = 0.74–0.95; CC vs GG: OR = 0.75, 95% CI = 0.60–0.93; GC + CC vs GG: OR = 0.80, 95% CI = 0.67–0.95; CC vs GG + GC: OR = 0.84, 95% CI = 0.70–1.00). Moreover, a significantly decreased risk of osteoporosis was also discovered in Asian (C vs G: OR = 0.80, 95% CI = 0.66–0.98; CC vs GG: OR = 0.67, 95% CI = 0.47–0.95; GC + CC vs GG: OR = 0.74, 95% CI = 0.58–0.95) and the female (C vs G: OR = 0.85, 95% CI = 0.75–0.97; CC vs GG: OR = 0.77, 95% CI = 0.61–0.96; GC + CC vs GG: OR = 0.79, 95% CI = 0.66–0.95). Finally, we did not find a close association between OPG T245G and T950C polymorphisms and osteoporosis risk. However, when we retained only studies in the control group that was consistent with Hardy-Weinberg equilibrium (HWE) and high-quality scores, we observed that the OPG A163G polymorphism increased the risk of osteoporosis in the overall analysis (G vs A: OR = 1.40, 95% CI = 1.16–1.68; GG vs AA: OR = 1.96, 95% CI = 1.20–3.21; AG + GG vs AA: OR = 1.45, 95% CI = 1.22–1.72). Finally, after the credibility assessment, we concluded that all statistically significant association results in the meta-analysis in this study and those in the previous study were ‘positive results with low confidence’.
Conclusion: In conclusion, our study concluded that all meaningful results between OPG A163G and G1181C polymorphisms and osteoporosis risk were false-positive results rather than true associations.
Introduction
Osteoporosis is a systemic bone disease characterized by deterioration of femoral microstructure and low bone mass, which increases fracture susceptibility and bone fragility (Rachner et al., 2011). The World Health Organization has a specific definition of osteoporosis: bone mineral density is measured by dual energy X-ray (DEXA), and the obtained bone mineral density value is called T-score. The T-score is more than 2.5 standard deviations below the average of the normal population (young healthy people with peak bone mass), and osteoporosis can be diagnosed. (Genant et al., 1999). Osteoporosis affects about 200 million people worldwide, which affects about 12% of men and 30% of women. Once osteoporosis is present, there may be significant pain and deformities, which also increase morbidity and mortality (Lin and Lane, 2004; Uzzan et al., 2007; Pisani et al., 2016). In addition, osteoporosis increases the risk of various diseases, such as fractures and pneumonia (Rapp et al., 2015), and around nine million people worldwide suffer fractures every year because of osteoporosis (Compston et al., 2019). In China, about 2.3 million people suffered fractures due to osteoporosis in 2010, and at this rate, the number of fractures caused by osteoporosis will exceed six million by 2050 (Si et al., 2015), which will undoubtedly increase the burden on families and the healthcare environment as a whole. From the above, we can understand that osteoporosis has become an inescapable health problem. Therefore, we must explore which risk factors contribute to osteoporosis.
The risk of osteoporosis includes environmental factors and genetic factors. The main environmental factors are changes in living habits, including smoking, drinking and improper exercise (Ng et al., 2006; Kaufman et al., 2008; Binici and Gunes, 2010). With the development of medical treatment, many studies have shown that genetic factors play an important role in the pathogenesis. (Ralston, 2001; Recker and Deng, 2002). The heritability of osteoporosis-related traits is estimated to be as high as 60–80% (Uitterlinden et al., 2004). So far, many risk genes have been found for osteoporosis. Among these risk genes, ITGA1, ESR1, SPP1, LRP4, and LRP5 have been confirmed to be related to bone matrix composition, bone mineral density (BMD) homeostasis and bone remodeling, thereby affecting BMD (Prestwood et al., 1995), which can lead to osteoporosis. In addition, there are many candidate genes (VDR, TGFB1, COL1A1, and OPG), but the association of these polymorphisms with osteoporosis risk has not been thoroughly demonstrated (Prestwood et al., 1995; Jørgensen et al., 2012; Saccone et al., 2015; Tsukasaki et al., 2020).
Osteoprotegerin (OPG), also called osteoclast genesis inhibitory factor, is a secreted glycoprotein discovered by Simonet et al., in 1997. It is a single copy gene cluster located on human chromosome 8q24.2. So far, many researches have shown that OPG gene has relationship to the pathogenesis of osteoporosis (Chung et al., 2003; Yu et al., 2013; Sun et al., 2014). The main mechanism is to inhibit osteoclast differentiation and maturation through the signal transduction system OPG/RANKL/RANK. The gene OPG can competitively bind RANK on the cell membrane surface of osteoclasts. Moreover, its binding ability is stronger than RANKL (Simonet et al., 1997), which can effectively interfere with the combination of RANKL and RANK. Common OPG polymorphisms associated with osteoporosis risk include A163G (rs3102735), T950C (rs2073617), and T245G (rs3134069), which are located at the promoter of the gene, while G1181C (rs2073618) is located at the first exon of the gene. Although researchers have published meta-analyses of OPG and osteoporosis risk. (Guo et al., 2014; Luo et al., 2014; Li et al., 2017; Li et al., 2021), but the results of studies on these gene polymorphisms are not completely consistent or even conflicting. The reasons for these different results are as follows: first of all, the number of samples is small and the included research literature is less; second, some studies have not carried out literature quality evaluation, which may lead to bias in the final results if low-quality literature is not excluded; at the same time, heterogeneity of selected literature, study design of variables, and whether or not to perform HWE tests also affect the results of meta-analysis. Finally, previously published meta-analyses have never evaluated positive results to determine multiple comparisons. In order to prove the relationship between the two, we need to provide more accurate and reliable theoretical evidence, so this is where we did an updated meta-analysis.
Materials and methods
This study was conducted according to the statement on preferred reporting items for systematic reviews and meta-analyses (Supplementary Table S5 PRISMA Checklist) (Moher et al., 2009).
Search strategy
The literature was searched using PubMed, Medline, International Statistical Institute (ISI), China National Knowledge Infrastructure (CNKI) and China Wanfang Database. The retrieval strategy was as follows (‘mutation’ OR ‘polymorphism’ OR ‘variation’ OR ‘variant’ OR ‘SNP’ OR ‘genetic association study’ OR ‘genome-wide association study’ OR ‘genotype’ OR ‘allele ‘) AND (osteoporosis OR osteoporoses) AND (OPG OR osteoprotegerin). The literature search was conducted until 20 March 2022. In addition, we carefully reviewed each reference list for meta-analyses in the literature to identify all eligible studies.
Selection criteria
The inclusion criteria were as follows: 1) to describe the relationship between OPG A163G, T245G, T950C and G1181C polymorphisms and the risk of osteoporosis (including the ethnic or demographic composition of the study subjects, the sex of the study subjects, and the genotyping technique used, Supplementary Table S2); 2) case-control study or cohort study, and all patients met the diagnostic criteria for osteoporosis; 3) detailed genotype data or odds ratio (OR) with 95% confidence interval (CI) could be extracted from the literature. Exclusion criteria were as follows: 1) not a case-control or cohort study; 2) failure to provide genotype data or duplicate genotype data; 3) meta-analyses, reviews, letters, case reports, and only including abstracts.
Data extraction and quality score assessment
We designed the data extraction form in advance. All literatures were screened according to the inclusion criteria and exclusion criteria, and then the literature data were extracted for statistical analysis. When the two authors obtained different results and still could not reach a consensus after discussion, the third author extracted the data again, and finally the three authors jointly checked and confirmed. If the data in the article is unclear or disputed, we will contact the original author to obtain the original data. The extracted information included: year of publication, first author of the article, country of study, ethnicity of study subjects, sex of study subjects, female menopause, polymorphism genotyping technique, type of control group, sample size and mean age for case and control groups, and quality score of all articles (Supplementary Table S2).
The quality assessment for all included articles was assessed separately by two authors. We referenced and improved the specific criteria for quality scores from previous studies (Guo et al., 2014; Luo et al., 2014; Li et al., 2017; Li et al., 2021). The full score of our reformulated quality assessment scale is 20 points, with more than 12 points as excellent (including) and less than 12 points as poor. The specific criteria of the quality evaluation scale are shown in Supplementary Table S3, and the score of each included paper is shown in Supplementary Table S4.
Statistical analysis
The correlation between OPG gene polymorphisms (A163G, T245G, T950C, and G1181C) and osteoporosis was expressed by ORs and corresponding 95% confidence intervals. The following five genetic models were selected for evaluation: 1) allele model (variant allele vs wildtype allele); 2) dominant mode (homozygous variant + heterozygous variant vs homozygous wildtype); 3) recessive model (homozygous variant vs heterozygous variant + homozygous wild type); 4) over-dominant model (homozygous wild type + homozygous variant vs heterozygous variant); 5) additive model (homozygous variant vs homozygous wild type). Chi-square q test and I2 value were used to evaluate the heterogeneity of the results. When p was less than 0.10 and/or I2 was greater than 50% (Li et al., 2005), the random effects model (MANTEL and HAENSZEL, 1959) is selected. If not, the fixed effects model is adopted (DerSimonian and Laird, 2015), and the goodness-of-fit test is further carried out to test the applicability of the model (Chen et al., 2015). At the same time, meta-regression was used to try to find the source of heterogeneity. Subgroup analyses were then performed according to ethnicity, sex, female menopause and control type. Two methods were used in the sensitivity analysis: 1) each study was excluded separately; 2) Only studies that met both high quality scores and HWE were retained. Prior to this, the control group was tested for HWE compliance using chi-square goodness of fit. p > 0.05 was defined as HWE, p < 0.05 is defined as HWD. Begg’s funnel plot (Begg and Mazumdar, 1994) and Egger’s test (Egger et al., 1997) were used to evaluate the existence of publication bias. If publication bias exists, the number of missing studies is estimated and supplemented using a non-parametric “trim and fill” approach (Dual and Tweedie, 2000). False-positive report probability (FPRP) (Wacholder et al., 2004), Bayesian False Discovery probability (BFDP) (Wakefield, 2007), and Venice criterion (Ioannidis et al., 2008) were used to assess the confidence of statistically significant associations. Stata 15.0 software was used to calculate all statistical analyses.
Results
Study characteristics
At the beginning of the period, 369 articles were searched from ISI, PubMed, Medline, CNKI and the China Wan-fang databases. There are 152 records left after duplicates are removed. After careful screening for titles and abstracts, we excluded 87 papers. In addition, 17 items were excluded because of data duplication or the inability to extract detailed data, and 18 items were excluded because of inadequate controls. Finally, 30 articles and 31 studies were included in our study (Supplementary Table S1). A total of 31 studies (Figure 1) were included in our study (including 8,402 osteoporosis cases and 7,517 controls), of which 14 studies reported OPG A163G (2,379 cases and 2,229 controls), nine studies investigated OPG T245G (941 cases and 1,019 controls), 12 studies investigated OPG T950C (1,610 cases and 1,234 controls), and 18 studies reported OPG G1181C (3,472 cases and 3,035 controls), as shown in Supplementary Table 1. In addition, OPG A163G had three low-quality studies and 11 high-quality articles, OPG T245G had two low-quality articles and seven high-quality articles, OPG T950C had five low-quality articles and seven high-quality articles, and OPG G1181C had two low-quality articles and 16 high-quality articles. The complete characteristics and genotype frequencies of the final included literatures are shown in Supplementary Table 1.
Quantitative synthesis
In the overall analysis, no meaningful association was found between the OPG A163G polymorphism and the risk of osteoporosis. However, in the following ethnic subgroup analysis, we found that the OPG A163G polymorphism increased the risk of osteoporosis in Caucasians (AG + GG vs AA: OR = 1.35, 95% CI = 1.06–1.73; AA + GG vs AG: OR = 0.64, 95% CI = 0.49–0.82, Table 1 and Figure 2). Unfortunately, in spite of a significant association in the African population, the sample size was only one study, which may limit the statistical power of this finding and thus we do not consider it sufficient to state a significant association between the two populations in the African population. In the sex subgroup, the OPG A163G polymorphism can significantly increase the risk of osteoporosis in females (G vs A: OR = 1.30, 95% CI = 1.03–1.64; AG + GG vs AA: OR = 1.42, 95% CI = 1.18–1.71, Table 1 and Figure 2). Similarly, in the female menopause subgroup, the OPG A163G polymorphism also increases the risk of osteoporosis in both non-postmenopausal women (G vs A: OR = 1.68, 95% CI = 1.36–2.07; GG vs AA: OR = 2.74, 95% CI = 1.64–4.58; AG + GG vs AA: OR = 1.37, 95% CI = 1.04–1.79; GG vs AA + AG: OR = 2.31, 95% CI = 1.55–3.43, Table 1 and Figure 2) and postmenopausal women (AG + GG vs AA: OR = 1.40, 95% CI = 1.06–1.85; GG vs AA + AG: OR = 0.67, 95% CI = 0.54–0.82, Table 1 and Figure 2).
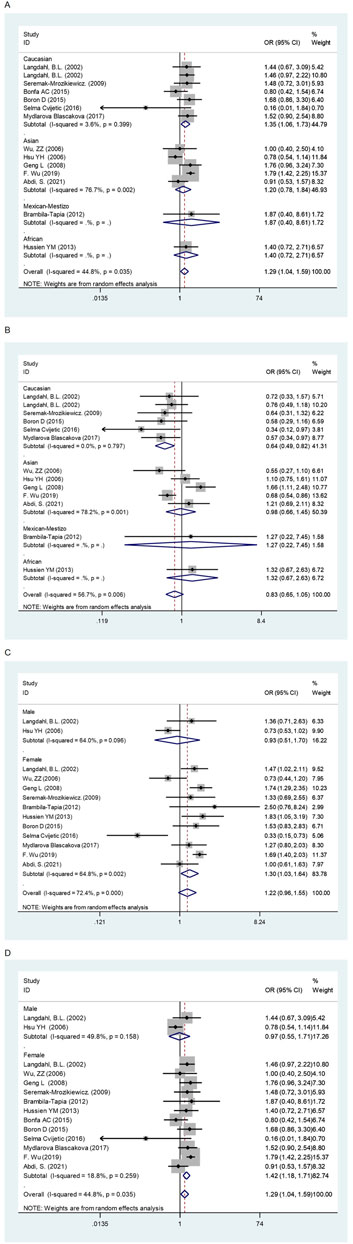
FIGURE 2. The forest plots of all selected studies on the adjusted association between the OPG A163G polymorphism and risk of osteoporosis in overall and subgroup analyses by ethnicity and sex (A: AG + GG vs AA; (B) AA + GG vs AG; (C) G vs A; (D) AG + GG vs AA).
Overall, the OPG G1181C polymorphism reduces the risk of osteoporosis (C vs G: OR = 0.84, 95% CI = 0.74–0.95; CC vs GG: OR = 0.75, 95% CI = 0.60–0.93; GC + CC vs GG: OR = 0.80, 95% CI = 0.67–0.95; CC vs GG + GC: OR = 0.84, 95% CI = 0.70–1.00, Table 4 and Figure 3). In subgroup analyses, the risk of osteoporosis was also significantly lower in Asian (C vs G: OR = 0.80, 95% CI = 0.66–0.98; CC vs GG: OR = 0.67, 95% CI = 0.47–0.95; GC + CC vs GG: OR = 0.74, 95% CI = 0.58–0.95, Table 4 and Figure 3) and female (C vs G: OR = 0.85, 95% CI = 0.75–0.97; CC vs GG: OR = 0.77, 95% CI = 0.61–0.96; GC + CC vs GG: OR = 0.79, 95% CI = 0.66–0.95, Table 4 and Figure 3). On this basis, we further found that OPG G1181C polymorphism could reduce the risk of osteoporosis in premenopausal female (C vs G: OR = 0.86, 95% CI = 0.73–1.00; CC vs GG + GC: OR = 0.71, 95% CI = 0.52–0.95, Table 4 and Figure 3).

FIGURE 3. The forest plots of all selected studies on the adjusted association between the OPG G1181C polymorphism and risk of osteoporosis in overall and subgroup analyses by ethnicity and sex (A: C vs G; (B) CC vs GG; (C) GC + CC vs GG; (D) C vs G; (E) CC vs GG; (F) GC + CC vs GG; (G) CC vs GG + GC).
Finally, we found that the OPG T245 and T950C polymorphisms did not increase or decrease the prevalence of osteoporosis in both global and subgroup analyses, that is, there was no association between them (Tables 2 and 3).
Heterogeneity and sensitivity analyses
During the statistical process, we found several possible sources of heterogeneity, including ethnicity, sex, sample size, female menopause, control type, quality score and HWE. Therefore, we used meta-regression analysis to determine the causes of heterogeneity. A meta-regression analysis revealed that the sample size (G vs A: p = 0.023) and HWE (G vs A: p = 0.010) were the source of heterogeneity between the OPG A163G polymorphism and the risk of osteoporosis. The type of controls (TG + GG vs. TT: p = 0.006) was the sources of heterogeneity between the OPG T245G polymorphism and the risk of osteoporosis. At the same time, the female menopause (C vs T: p = 0.016; CC vs. TT: p = 0.041; TC + CC vs. TT: p = 0.020; CC vs. TT + TC: p = 0.038), sample size (TC + CC vs. TT: p = 0.037) and sex (TT + CC vs. TC: p = 0.045) were the sources of heterogeneity between the OPG T950C polymorphism and the risk of osteoporosis. For the OPG G1181C, no covariate was found as a possible cause of between-study variation.
In this meta-analysis, two methods were used for sensitivity analysis. In the first, each study was removed one by one, and the results did not change when a single study was removed each time. Second, literature that met both high quality scores and HWE was screened. When only control studies that met HWE and high-quality scores were retained, we found that in the overall analysis, the OPG A163G polymorphism significantly increases the risk of osteoporosis (G vs A: OR = 1.40, 95% CI = 1.16–1.68; GG vs AA: OR = 1.96, 95% CI = 1.20–3.21; AG + GG vs AA: OR = 1.45, 95% CI = 1.22–1.72, Table 1), and the same was observed in the female population (G vs A: OR = 1.39, 95% CI = 1.14–1.70; GG vs AA: OR = 1.96, 95% CI = 1.15–3.35; AG + GG vs AA: OR = 1.42, 95% CI = 1.17–1.72, Table 1). However, we did not find significant changes in OPG T245G, T950C and G1181C.
Publication bias
The results of Begg’s funnel plot and Egger’s test showed that only OPG G1181C polymorphism and risk of osteoporosis had publication bias (C vs G: p = 0.015; GC + CC vs. GG: p = 0.045, Table 4). Then, publication bias was adjusted using the nonparametric “trim and fill”method. However, when we applied a nonparametric “trim and fill” method (Figure 4), the analysis showed that no more study should be added, showing that the results were stable.
Credibility of previous meta-analyses
Since the previously published meta-analyses were not evaluated for credibility, we evaluated the results for accuracy, applying the FPRP, BFDP, and Venice criteria. Through calculation and statistics, we obtained results that Guo et al., 2014, Luo et al., 2014, Li et al., 2017 and Li et al., 2021 were classified as less credible. Table 5 specifically shows the previously published credibility results (FPRP >0.2 and BFDP >0.8, I2 > 50%, statistical power <80%).
Credibility of the current meta-analysis
Our study also used BFDP, FPRP and Venice criteria to evaluate the statistically significant credibility. Associations that met the following criteria were considered to have high confidence: 1) at least two genetic models were statistically significant; 2) statistical power >80%; 3) I2 < 50%; 4) FPRP <0.2 and BFDP <0.8. If all four criteria are not met, then all meaningful results are considered “less credible”. Table 6 specifically shows the results of the credibility assessment for all meaningful results. In the final calculation, we can find that all statistically significant results are “less likely".
Discussion
Osteoporosis is a systemic skeletal disease associated with the action of multiple genes. Evidence from many studies indicates that OPG polymorphism has been considered one of the potential genetic factors for osteoporosis. The OPG/RANKL/RANK is the main signal transduction pathway in osteoporosis, which can regulate the differentiation, induction, activation and maintenance of osteoclasts (Zhao et al., 2020; Udagawa et al., 2021). Osteoclasts are formed by the combination of RANKL and RANK on the surface of osteoclasts to form a complex (Nakashima et al., 2011; McCabe et al., 2015). OPG prevents osteoclast formation by blocking RANKL binding site and preventing RANKL from forming complex with RANK receptor, thus inhibiting bone resorption and thereby avoiding the occurrence of osteoporosis (Kurinami et al., 2016; Maria et al., 2018). Previous studies have focused on four OPG polymorphisms: G1181C, located in the first exon, and A163G, T245G, and T950C, located in the promoter. (Langdahl et al., 2002). Many investigators have attempted to demonstrate specific potential relationships between OPG polymorphisms and osteoporosis. However, so far there has not been enough meaningful evidence to confirm a relationship, which may be due to various factors, for instance, the small sample size, ethnic and geographical differences and so on. Our meta-analysis is a valid way to avoid some shortcomings.
Overall, we did not find a significant association between OPG T245G and T950C polymorphisms and osteoporosis. While the OPG A163G and G1181 C polymorphisms were associated with the risk of osteoporosis in several subgroups. Moreover, when we retained only high-quality score literature and HWE, we found that only the OPG A163G polymorphism was associated with increased the risk of osteoporosis in overall and several subgroup analyses. However, the pooled p-value must be adjusted on the meta-analyses of the gene polymorphism with risk of disease because they applied several subgroups and genetic models at the expense of multiple comparisons (Attia et al., 2003). Therefore, we investigated the false positive results based on the FPRP, BFDP, and Venice criterion. FPRP is a method that uses multiple hypothesis testing to assess the likelihood of important outcomes in molecular epidemiological investigations. In 2007, Wakefield introduced a test called Bayesian error, which is more accurate in genetic epidemiological surveys. Many reasons can lead to the bias of the results, among which statistical power is an important factor, of which statistical power is an important factor. Many studies have demonstrated that when statistical power> 80%, higher statistical significance or lower false discovery rates can be achieved. However, when we used the above methods to assess the credibility of the current study, all statistically significant demerits were considered “not credible” (FPRP >0.2 and BFDP >0.8, I2 > 50%, statistical power <80%), in other words, all statistically significant results were considered false-positive results.
Significant publication bias was only observed between the OPG G1181C polymorphism and risk of osteoporosis. As far as we know, some low quality and small sample sizes studies are common bias and errors. These studies are not always strict and the quality is poor, so the statistically significant small sample study is more likely to be accepted, it will be very easy to produce false positive results, making the conclusions of these original studies implausible. In the test of publication bias, the asymmetry of the funnel plot is caused by the study of low quality and small samples.
All four previous meta-analyses researched the correlation between OPG A163G, T245G, T950C and G1181C polymorphisms and osteoporosis. There is an obvious mismatch between all previous meta-analyses and this meta-analysis in the subgroup analysis classification. Moreover, after careful reading of the full text, we found that the previous meta-analysis had relevant information and data extraction errors in the process of data extraction, and we corrected them in the extraction process. In addition, the sample size of our study is large. A total of 31 studies were applied in this study (involving 8,402 osteoporosis cases and 7,517 controls), of which 14 studies showed the OPG A163G (2,379 cases and 2,229 controls), nine studies investigated the OPG T245G (941 cases and 1,019 controls), 12 studies investigated the OPG T950C (1,610 cases and 1,234 controls), and 18 studies reported the OPG G1181C (3,472 cases and 3,035 controls). In this study, five genetic models were compared separately. Li et al., 2017, however, applied four genetic models; Li et al., 2021, used only three genetic models. At the same time, Li et al., 2017, the ethnicity studied was limited to Chinese. It is important to note that previous meta-analyses did not perform credibility analyses on the results, and all statistically significant relationships were considered “less credible” when we assessed the credibility of previous meta-analyses using the same criteria. Therefore, their research results and conclusions may not be reliable, and they may also be false positive results.
Compared with previous meta-analyses, our study has the following advantages: 1) FPRP, BFDP test and Venice criterion were used for credibility assessment; 2) to evaluate the quality of qualified research; 3) compared with previous meta-analyses, the studies included in this study are more comprehensive, and the data collected are more detailed and accurate; 4) the subgroup analysis was more comprehensive, and it is important to note that not only did we subgroup sex, but we also grouped women separately based on whether they were postmenopausal or not; However, our study still has some potential limitations. First, the current meta-analysis included only published studies, and it is well known that positive results are studied more than negative results. Second, osteoporosis is a complicated polygenic disease, and individual SNPS are slightly associated with osteoporosis risk. However, we have not retrieved data on gene-gene and gene-environment combined effects. Third, although we objectively collected data from the included literature, the control: control rate was less than 1, which may also affect the accuracy of the association. At the same time, there have been relatively few studies of Africans in ethnic analyses and insufficient statistical power to excavate true associations. Therefore, future studies with large samples and sufficiently large subgroups will help validate our findings. Finally, the risk of osteoporosis includes not only genetic factors, but also environmental and lifestyle factors. Our study did not control for various osteoporosis variables, such as smoking, alcohol consumption, breastfeeding, physical activity, estrogen replacement therapy, corticosteroids, calcium supplements et al. In the future, we need to control for various other variables that contribute to osteoporosis, and investigate the association between osteoporosis and genetic, environmental, and lifestyle factors to help validate our findings.
In conclusion, this study indicates that all meaningful results between OPG A163G and G1181C polymorphisms and osteoporosis risk were false-positive results rather than true associations. At present, there is still no absolute strong evidence for the real association between OPG polymorphism and osteoporosis risk, and further large-scale epidemiological studies are needed to confirm or deny our findings.
Data availability statement
The original contributions presented in the study are included in the article/Supplementary Material, further inquiries can be directed to the corresponding authors.
Author contributions
XH: design research program, collect data, analyze statistics and write articles. LZ: collect data. Y-YM: review the collected data. H-ZL and X-FH: research design and paper review.
Acknowledgments
We are grateful to the authors of all the original studies included in the meta-analysis. We would also like to thank X-FH and H-ZL for their guidance in our study.
Conflict of interest
The authors declare that the research was conducted in the absence of any commercial or financial relationships that could be construed as a potential conflict of interest.
Publisher’s note
All claims expressed in this article are solely those of the authors and do not necessarily represent those of their affiliated organizations, or those of the publisher, the editors and the reviewers. Any product that may be evaluated in this article, or claim that may be made by its manufacturer, is not guaranteed or endorsed by the publisher.
Supplementary material
The Supplementary Material for this article can be found online at: https://www.frontiersin.org/articles/10.3389/fgene.2022.1032110/full#supplementary-material
Abbreviations
BFDP, Bayesian false discovery probability; CI s, confidence intervals; CNKI, China National Knowledge Infrastructure; FPRP, false-positive report probabilities; HWE, Hardy–Weinberg equilibrium; HWD, Hardy–Weinberg Disequilibrium; ISI, International Statistical Institute; OPG, osteoprotegerin; ORs, odds ratios
References
Abdi, S., Binbaz, R. A., Mohammed, A. K., Ansari, M., Wani, K., Amer, O. E., et al. (2021). Association of RANKL and OPG gene polymorphism in arab women with and without osteoporosis. Genes. 12 (2), 200. doi:10.3390/genes12020200
Attia, J., Thakkinstian, A., and D’Este, C. (2003). Meta-analyses of molecular association studies: Methodologic lessons for genetic epidemiology. J. Clin. Epidemiol. 56, 297–303. doi:10.1016/s0895-4356(03)00011-8
Begg, C. B., and Mazumdar, M. (1994). Operating characteristics of a rank correlation test for publication bias. Biometrics 50 (4), 1088–1101. doi:10.2307/2533446
Binici, D. N., and Gunes, N. (2010). Risk factors leading to reduced bone mineral density in hemodialysis patients with metabolic syndrome. Ren. Fail. 32 (4), 469–474. doi:10.3109/08860221003675260
Bonfá, A. C., Seguro, L. P., Caparbo, V., Bonfá, E., and Pereira, R. M. (2015). RANKL and OPG gene polymorphisms: Associations with vertebral fractures and bone mineral density in premenopausal systemic lupus erythematosus. Osteoporos. Int. 26 (5), 1563–1571. doi:10.1007/s00198-015-3029-x
Boroń, D., Kotrych, D., Bartkowiak-Wieczorek, J., Uzar, I., Bogacz, A., Kamiński, A., et al. (2015). Polymorphisms of OPG and their relation to the mineral density of bones in pre- and postmenopausal women. Int. Immunopharmacol. 28 (1), 477–486. doi:10.1016/j.intimp.2015.07.015
Brambila-Tapia, A. J., Durán-González, J., Sandoval-Ramírez, L., Mena, J. P., Salazar-Páramo, M., Gámez-Nava, J. I., et al. (2012). MTHFR C677T, MTHFR A1298C, and OPG A163G polymorphisms in Mexican patients with rheumatoid arthritis and osteoporosis. Dis. Markers 32 (2), 109–114. doi:10.3233/DMA-2011-0868
Chen, Z., Zhang, G., and Li, J. (2015). Goodness-of-fit test for meta-analysis. Sci. Rep. 5, 16983. doi:10.1038/srep16983
Chung, H. W., Seo, J. S., Hur, S. E., Kim, H. L., Kim, J. Y., Jung, J. H., et al. (2003). Association of interleukin-6 promoter variant with bone mineral density in pre-menopausal women. J. Hum. Genet. 48 (5), 243–248. doi:10.1007/s10038-003-0020-8
Compston, J. E., McClung, M. R., and Leslie, W. D. (2019). Lancet 393 (10169), 364–376. doi:10.1016/S0140-6736(18)32112-3
Cvijetic, S., Grazio, S., Kosovic, P., Uremovic, M., Nemcic, T., and Bobic, J. (2016). Osteoporosis and polymorphisms of osteoprotegerin gene in postmenopausal women - a pilot study. Reumatologia 54 (1), 10–13. doi:10.5114/reum.2016.58755
DerSimonian, R., and Laird, N. (2015). Meta-analysis in clinical trials revisited. Contemp. Clin. Trials 45, 139–145. doi:10.1016/j.cct.2015.09.002
Dinçel, E., Sepici-Dinçel, A., Sepici, V., Ozsoy, H., and Sepici, B. (2008). Hip fracture risk and different gene polymorphisms in the Turkish population. Clin. (Sao Paulo, Braz. 63 (5), 645–650. doi:10.1590/s1807-59322008000500013
Dual, S., and Tweedie, R. (2000). A nonparametric “trim and fill” method of accounting for publication bias in meta-analysis. J. Am. Stat. Assoc. 95, 89–98. doi:10.2307/2669529
Egger, M., Davey Smith, G., Schneider, M., and Minder, C. (1997). Bias in meta-analysis detected by a simple, graphical test. BMJ Clin. Res. ed.) 315, 629–634. doi:10.1136/bmj.315.7109.629
Genant, H. K., Cooper, C., Poor, G., Reid, I., Ehrlich, G., Kanis, J., et al. (1999). Interim report and recommendations of the world health organization task-force for osteoporosis. Osteoporos. Int. 10 (4), 259–264. doi:10.1007/s001980050224
Geng, L. (2008). Study on female osteoporosis and polymorphisms of related genes. Chongqing, China: Doctor Thesis of Chong-qing Medical University.
González-Mercado, A., Sánchez-López, J. Y., Perea-Díaz, F. J., Magaña-Torres, M. T., Salazar-Páramo, M., González-López, L., et al. (2019). Association of polymorphisms of the TNFRSF11B and TNFSF11 genes with bone mineral density in postmenopausal women from Western Mexico. Arch. Med. Sci. 15 (5), 1352–1356. doi:10.5114/aoms.2019.87410
Guo, L., Tang, K., Quan, Z., Zhao, Z., and Jiang, D. (2014). Association between seven common OPG genetic polymorphisms and osteoporosis risk: A meta-analysis. DNA Cell. Biol. 33 (1), 29–39. doi:10.1089/dna.2013.2206
Hsu, Y. H., Niu, T., Terwedow, H. A., Xu, X., Feng, Y., Li, Z., et al. (2006). Variation in genes involved in the RANKL/RANK/OPG bone remodeling pathway are associated with bone mineral density at different skeletal sites in men. Hum. Genet. 118 (5), 568–577. doi:10.1007/s00439-005-0062-4
Hussien, Y. M., Shehata, A., Karam, R. A., Alzahrani, S. S., Magdy, H., and El-Shafey, A. M. (2013). Polymorphism in vitamin D receptor and osteoprotegerin genes in Egyptian rheumatoid arthritis patients with and without osteoporosis. Mol. Biol. Rep. 40 (5), 3675–3680. doi:10.1007/s11033-012-2443-9
Ioannidis, J. P., Boffetta, P., Little, J., O'Brien, T. R., Uitterlinden, A. G., Vineis, P., et al. (2008). Assessment of cumulative evidence on genetic associations: Interim guidelines. Int. J. Epidemiol. 37 (1), 120–132. doi:10.1093/ije/dym159
Jørgensen, L., Hansen, J. B., Ahmed, L., Bjørnerem, Å., Emaus, N., Joakimsen, R., et al. (2012). Osteoprotegerin is associated with hip fracture incidence: The tromso study. Int. J. Epidemiol. 41 (4), 1033–1039. doi:10.1093/ije/dys063
Kaufman, J. M., Ostertag, A., Saint-Pierre, A., Cohen-Solal, M., Boland, A., Van Pottelbergh, I., et al. (2008). Genome-wide linkage screen of bone mineral density (BMD) in European pedigrees ascertained through a male relative with low BMD values: Evidence for quantitative trait loci on 17q21-23, 11q12-13, 13q12-14, and 22q11. J. Clin. Endocrinol. Metab. 93 (10), 3755–3762. doi:10.1210/jc.2008-0678
Kim, J. G., Kim, J. H., Kim, J. Y., Ku, S. Y., Jee, B. C., Suh, C. S., et al. (2007). Association between osteoprotegerin (OPG), receptor activator of nuclear factor-kappaB (RANK), and RANK ligand (RANKL) gene polymorphisms and circulating OPG, soluble RANKL levels, and bone mineral density in Korean postmenopausal women. Menopause (New York, N.Y.) 14 (5), 913–918. doi:10.1097/gme.0b013e31802d976f
Kurinami, H., Shimamura, M., Nakagami, H., Shimizu, H., Koriyama, H., Kawano, T., et al. (2016). A novel therapeutic peptide as a partial agonist of RANKL in ischemic stroke. Sci. Rep. 6, 38062. doi:10.1038/srep38062
Langdahl, B. L., Carstens, M., Stenkjaer, L., and Eriksen, E. F. (2002). Polymorphisms in the osteoprotegerin gene are associated with osteoporotic fractures. J. Bone Min. Res. 17 (7), 1245–1255. doi:10.1359/jbmr.2002.17.7.1245
Li, Boyi, Niu, Ling, Ma, Rong, Zhang, Xian, Liu, Fang, Tang, Yan, et al. (2022). Relationship between osteoprotegerin gene promoter T950C polymorphism and osteoporosis in type 2 diabetes mellitus [J]. J. Kunming Med. Univ. 43 (03), 67–73.
Li, H. M., Miao, H., Lu, Y. B., and Cheng, J. L. (2005). Association between the polymorphism of human vitamin D receptor gene and the susceptibility of diabetic nephropathy in Chinese Han population. Chin. J. Clin. Rehabil. 9, 1–4.
Li, S., Jiang, H., and Du, N. (2017). Association between osteoprotegerin gene T950C polymorphism and osteoporosis risk in the Chinese population: Evidence via meta-analysis. PloS one 12 (12), e0189825. doi:10.1371/journal.pone.0189825
Li, X., Cheng, J., Dong, B., Yu, X., Zhao, X., and Zhou, Z. (2021). Common variants of the OPG gene are associated with osteoporosis risk: A meta-analysis. Genet. Test. Mol. Biomarkers 25 (9), 600–610. doi:10.1089/gtmb.2020.0282
Li, X. R. (2009). Association between OPG genetic polymorphisms and osteoporosis in elderly men. Shandong Med. J. 49, 71–72.
Lin, J. T., and Lane, J. M. (2004). Osteoporosis: A review. Clin. Orthop. Relat. Res. 425 (425), 126–134. doi:10.1097/01.blo.0000132404.30139.f2
Liu, J., Miao, Y. D., Zhang, Y. J., He, P. Y., Bao, H., Zhu, L., et al. (2010). 8-Quinolineboronic acid as a potential phosphorescent molecular switch for the determination of alpha-fetoprotein variant for the prediction of primary hepatocellular carcinoma. Anal. Chim. Acta 16, 184–189. doi:10.1016/j.aca.2010.01.030
Luo, Y., Hu, Z., Hao, J., Jiang, W., Shen, J., and Zhao, J. (2014). Significant associations between the A163G and G1181C polymorphisms of the osteoprotegerin gene and risk of osteoporosis, especially in postmenopausal women: A meta-analysis. Genet. Test. Mol. Biomarkers 18 (3), 211–219. doi:10.1089/gtmb.2013.0420
Mantel, N., and Haenszel, W. (1959). Statistical aspects of the analysis of data from retrospective studies of disease. J. Natl. Cancer Inst. 22 (4), 719–748.
Maria, S., Samsonraj, R. M., Munmun, F., Glas, J., Silvestros, M., Kotlarczyk, M. P., et al. (2018). Biological effects of melatonin on osteoblast/osteoclast cocultures, bone, and quality of life: Implications of a role for MT2 melatonin receptors, MEK1/2, and MEK5 in melatonin-mediated osteoblastogenesis. J. Pineal Res. 64 (3), e12465. doi:10.1111/jpi.1246510.1111/jpi.12465
McCabe, L., Britton, R. A., and Parameswaran, N. (2015). Prebiotic and probiotic regulation of bone health: Role of the intestine and its microbiome. Curr. Osteoporos. Rep. 13 (6), 363–371. doi:10.1007/s11914-015-0292-x
Mencej-Bedrac, S., Prezelj, J., Kocjan, T., Teskac, K., Ostanek, B., Smelcer, M., et al. (2009). The combinations of polymorphisms in vitamin D receptor, osteoprotegerin and tumour necrosis factor superfamily member 11 genes are associated with bone mineral density. J. Mol. Endocrinol. 42 (3), 239–247. doi:10.1677/JME-08-0108
Mencej-Bedrač, S., Preželj, J., and Marc, J. (2011). TNFRSF11B gene polymorphisms 1181G > C and 245T > G as well as haplotype CT influence bone mineral density in postmenopausal women. Maturitas 69 (3), 263–267. doi:10.1016/j.maturitas.2011.02.010
Moher, D., Liberati, A., Tetzlaff, J., and Altman, D. G.PRISMA Group (2009). Preferred reporting items for systematic reviews and meta-analyses: The PRISMA statement. J. Clin. Epidemiol. 62 (10), 1006–1012. doi:10.1016/j.jclinepi.2009.06.005
Mydlárová Blaščáková, M., Blaščáková, Ľ., Poráčová, J., Mydlár, J., Vašková, J., Bernasovská, J., et al. (2017). Relationship between A163G osteoprotegerin gene polymorphism and other osteoporosis parameters in Roma and non-Roma postmenopausal women in eastern Slovakia. J. Clin. Lab. Anal. 31 (5), e22093. doi:10.1002/jcla.22093
Mydlárová Blaščáková, M., Mydlár, J., Petrejčíková, E., Vašková, J., Poráčová, J., Bauerová, M., et al. (2021). Preliminary results of ethnic divergence of G1181C (rs2073618) and C290T (rs9525641) OPG gene polymorphisms in groups of postmenopausal Slovak women. Eur. Rev. Med. Pharmacol. Sci. 25 (5), 2182–2192. doi:10.26355/eurrev_202103_25209
Nakashima, T., Hayashi, M., Fukunaga, T., Kurata, K., Oh-Hora, M., Feng, J. Q., et al. (2011). Evidence for osteocyte regulation of bone homeostasis through RANKL expression. Nat. Med. 17 (10), 1231–1234. doi:10.1038/nm.2452
Nava-Valdivia, C. A., Saldaña-Cruz, A. M., Corona-Sanchez, E. G., Murillo-Vazquez, J. D., Moran-Moguel, M. C., Salazar-Paramo, M., et al. (2017). Polymorphism rs2073618 of the TNFRSF11B (OPG) gene and bone mineral density in Mexican women with rheumatoid arthritis. J. Immunol. Res. 2017, 7680434. doi:10.1155/2017/7680434
Ng, M. Y., Sham, P. C., Paterson, A. D., Chan, V., and Kung, A. W. (2006). Effect of environmental factors and gender on the heritability of bone mineral density and bone size. Ann. Hum. Genet. 70, 428–438. doi:10.1111/j.1469-1809.2005.00242.x
Pisani, P., Renna, M. D., Conversano, F., Casciaro, E., Di Paola, M., Quarta, E., et al. (2016). Major osteoporotic fragility fractures: Risk factor updates and societal impact. World J. Orthop. 7 (3), 171–181. doi:10.5312/wjo.v7.i3.171
Prestwood, K. M., Pilbeam, C. C., and Raisz, L. G. (1995). Treatment of osteoporosis. Annu. Rev. Med. 46, 249–256. doi:10.1146/annurev.med.46.1.249
Rachner, T. D., Khosla, S., and Hofbauer, L. C. (2011). Osteoporosis: Now and the future. Lancet (London, Engl. 377 (9773), 1276–1287. doi:10.1016/S0140-6736(10)62349-5
Ralston, S. H. (2001). Genetics of osteoporosis. Rev. Endocr. Metab. Disord. 2 (1), 13–21. doi:10.1023/a:1010098706338
Rapp, K., Rothenbacher, D., Magaziner, J., Becker, C., Benzinger, P., König, H. H., et al. (2015). Risk of nursing home admission after femoral fracture compared with stroke, myocardial infarction, and pneumonia. J. Am. Med. Dir. Assoc. 16 (8), e7–e715. doi:10.1016/j.jamda.2015.05.013
Recker, R. R., and Deng, H. W. (2002). Role of genetics in osteoporosis. Endocrine 17 (1), 55–66. doi:10.1385/ENDO:17:1:55
Saccone, D., Asani, F., and Bornman, L. (2015). Regulation of the vitamin D receptor gene by environment, genetics and epigenetics. Gene 561 (2), 171–180. doi:10.1016/j.gene.2015.02.024
Seremak-Mrozikiewicz, A., Tatuśko, J., Drews, K., Barlik, M., Krajewski, P., Spaczyński, M., et al. (2009). [Polymorphism of osteoprotegerin gene and osteoporosis in postmenopausal women]. Ginekol. Pol. 80 (5), 354–360.
Si, L., Winzenberg, T. M., Jiang, Q., Chen, M., and Palmer, A. J. (2015). Projection of osteoporosis-related fractures and costs in China: 2010-2050. Osteoporos. Int. 26 (7), 1929–1937. doi:10.1007/s00198-015-3093-2
Simonet, W. S., Lacey, D. L., Dunstan, C. R., Kelley, M., Chang, M. S., Lüthy, R., et al. (1997). Osteoprotegerin: A novel secreted protein involved in the regulation of bone density. Cell. 89 (2), 309–319. doi:10.1016/s0092-8674(00)80209-3
Sui, M. M., Na, S. P., Xie, R. J., Wang, M. G., and Mu, S. H. (2008). Relationship between the polymorphisms of T149C and T950C in osteoprotegerin gene and the glucocorticoid-induced osteoporosis. Chin. J. Blood Purif. 17, 375–379.
Sun, T., Chen, M., Lin, X., Yu, R., Zhao, Y., and Wang, J. (2014). The influence of osteoprotegerin genetic polymorphisms on bone mineral density and osteoporosis in Chinese postmenopausal women. Int. Immunopharmacol. 22 (1), 200–203. doi:10.1016/j.intimp.2014.06.023
Tao, Y. H. (2011). “Relationships between the OPG gene T950C and G1181C polymorphisms, blood lipids, BMD and carotid artery plaque formation in postmenopausal women,”. Master Thesis of Fujian Medical Univer-sity. (article in Chinese).
Tsukasaki, M., Asano, T., Muro, R., Huynh, N. C., Komatsu, N., Okamoto, K., et al. (2020). OPG production matters where it happened. Cell. Rep. 32 (10), 108124. doi:10.1016/j.celrep.2020.108124
Udagawa, N., Koide, M., Nakamura, M., Nakamichi, Y., Yamashita, T., Uehara, S., et al. (2021). Osteoclast differentiation by RANKL and OPG signaling pathways. J. Bone Min. Metab. 39 (1), 19–26. doi:10.1007/s00774-020-01162-6
Uitterlinden, A. G., Fang, Y., Van Meurs, J. B., Pols, H. A., and Van Leeuwen, J. P. (2004). Genetics and biology of vitamin D receptor polymorphisms. Gene 338 (2), 143–156. doi:10.1016/j.gene.2004.05.014
Uzzan, B., Cohen, R., Nicolas, P., Cucherat, M., and Perret, G. Y. (2007). Effects of statins on bone mineral density: A meta-analysis of clinical studies. Bone 40 (6), 1581–1587. doi:10.1016/j.bone.2007.02.019
Vidal, C., Brincat, M., and Xuereb Anastasi, A. (2006). TNFRSF11B gene variants and bone mineral density in postmenopausal women in Malta. Maturitas 53 (4), 386–395. doi:10.1016/j.maturitas.2005.11.003
Wacholder, S., Chanock, S., Garcia-Closas, M., El Ghormli, L., and Rothman, N. (2004). Assessing the probability that a positive report is false: An approach for molecular epidemiology studies. J. Natl. Cancer Inst. 96 (6), 434–442. doi:10.1093/jnci/djh075
Wakefield, J. (2007). A Bayesian measure of the probability of false discovery in genetic epidemiology studies. Am. J. Hum. Genet. 81 (2), 208–227. doi:10.1086/519024
Wu, Z. Z., Feng, J. Q., Liu, M., Lin, W., Huang, Y., Shi, Q. W., et al. (2007). The relationship between body fat ratio combined with G209A and T245G polymorphism of osteoprotegerin promoter region and osteoporosis. Chin. J. Geriatr. 12, 2409–2413.
Wu, Z. Z., Liu, M., and Feng, J. Q. (2005). Relationship among single T950C mutation polymorphism in the promoter region of the osteoprotegerin gene, bone mineral density and metabolism. Chin. J. Geriatr. 3, 247–250.
Wu, Z. Z., and Liu, M. (2006). The effect of low-body weight combined with T149C and A163G polymorphism of osteoprotegerin promoter region on osteoporosis. Chin. J. Geriatr. 25, 645–650.
Yu, F., Huang, X., Miao, J., Guo, L., and Tao, D. (2013). Association between osteoprotegerin genetic variants and osteoporosis in Chinese postmenopausal women. Endocr. J. 60 (12), 1303–1307. doi:10.1507/endocrj.ej13-0308
Zavala-Cerna, M. G., Moran-Moguel, M. C., Cornejo-Toledo, J. A., Gonzalez-Montoya, N. G., Sanchez-Corona, J., Salazar-Paramo, M., et al. (2015). Osteoprotegerin polymorphisms in a Mexican population with rheumatoid arthritis and generalized osteoporosis: A preliminary report. J. Immunol. Res. 2015, 376197. doi:10.1155/2015/376197
Zhao, H. Y., Liu, J. M., Ning, G., Zhao, Y. J., Zhang, L. Z., Sun, L. H., et al. (2005). The influence of Lys3Asn polymorphism in the osteoprotegerin gene on bone mineral density in Chinese postmenopausal women. Osteoporos. Int. 16 (12), 1519–1524. doi:10.1007/s00198-005-1865-9
Keywords: osteoprotegerin, OPG, polymorphism, meta-analysis, BFDP, FPRP
Citation: Han X, Zheng L, Mu Y-Y, Li H-Z and He X-F (2022) Association between OPG polymorphisms and osteoporosis risk: An updated meta-analysis. Front. Genet. 13:1032110. doi: 10.3389/fgene.2022.1032110
Received: 30 August 2022; Accepted: 24 October 2022;
Published: 09 November 2022.
Edited by:
Yujie Ning, Xi’an Jiaotong University, ChinaReviewed by:
Patricia Canto, Universidad Nacional Autónoma de México, MexicoManuel De Jesús Castillejos López, Instituto Nacional de Enfermedades Respiratorias-México (INER), Mexico
Copyright © 2022 Han, Zheng, Mu, Li and He. This is an open-access article distributed under the terms of the Creative Commons Attribution License (CC BY). The use, distribution or reproduction in other forums is permitted, provided the original author(s) and the copyright owner(s) are credited and that the original publication in this journal is cited, in accordance with accepted academic practice. No use, distribution or reproduction is permitted which does not comply with these terms.
*Correspondence: Hong-Zhuo Li, lihz0999@sina.com; Xiao-Feng He, 393120823@qq.com