- 1Shanghai Innovation Center of Traditional Chinese Medicine Health Service, School of Public Health, Shanghai University of Traditional Chinese Medicine, Shanghai, China
- 2Bio-X Institutes, Key Laboratory for the Genetics of Developmental and Neuropsychiatric Disorders, Shanghai Jiao Tong University, Shanghai, China
- 3Graduate School of Sport Sciences, Waseda University, Saitama, Japan
- 4Zhangjiang Community Health Service Center of Pudong New District, Shanghai, China
- 5Institute of Digestive Diseases, Longhua Hospital, Shanghai University of Traditional Chinese Medicine, Shanghai, China
Objective: Non-alcoholic fatty liver disease (NAFLD) affects almost a quarter of the world’s population. Although NAFLD often co-exists with obesity, a substantial proportion of NAFLD patients are lean which is relatively unexplored. This study aimed to examine the association between genetic variation in candidate genes, e.g., TBC1D1 and the risk of lean NAFLD in the elderly Chinese Han population.
Methods: This is an extension of the research on physical examination in the Zhanjiang community center including 5387 residents, Shanghai, China, in 2017. According to the classification in adult Asian populations, participants were categorized into four groups: lean NAFLD (BMI <23, n = 106), non-lean NAFLD (BMI ≥23, n = 644), lean non-NAFLD (BMI <23, n = 216) and non-lean non-NAFLD (BMI ≥23, n = 253).116 NAFLD-related candidate genes, which cover 179 single nucleotide polymorphisms (SNPs) were included in the KEGG enrichment analysis. Independent samples t-test was adopted for the group comparison. The associations between genetic variations with the specific phenotype in five genetic models were analyzed with the “SNPassoc” R package and rechecked with logistic regression analysis. Mediation models were conducted to explore whether the certain phenotype can mediate the association between SNPs and the risk of lean NAFLD.
Results: Compared with lean non-NAFLD individuals, lean NAFLD patients had higher BMI, low-density lipoprotein and triglyceride, and lower HDL. The AMPK signaling pathway, which includes TBC1D1 and ADIPOQ genes, was the most significant (p < .001). The A allele frequency of rs2279028 in TBC1D1 (p = .006) and G allele frequency of rs17366568 in ADIPOQ (p = .038) were significantly lower in lean NAFLD. The association between rs2279028 and the risk of lean NAFLD was mediated by HDL (p = .014). No significant mediation effect was found between rs17366568 and the risk of lean NAFLD.
Conclusion: This study, for the first time, indicated that rs2279028 of TBC1D1 may contribute to the progression of lean NAFLD through HDL. This finding provides more evidence for exploring the mechanism of lean NAFLD and suggests practical solutions for the treatment of lean NAFLD.
Introduction
As one of the public health problems, non-alcoholic fatty liver disease (NAFLD) affects almost a quarter of the world population and triggers the occurrence and deterioration of complications. e.g., type 2 diabetes, cardiovascular diseases, and hepatocyte carcinoma (Younossi et al., 2018). Although NAFLD often co-exists with obesity, a substantial proportion of NAFLD patients are lean, with a prevalence of 5%–45% in Asians (Eslam et al., 2020) caused by diagnostic strategy selection and genetic variation induced by ethnicity, which is relatively unexplored.
NAFLD may be highly susceptible to genetic and environmental factors (Eslam and George, 2016; Eslam et al., 2018). It was estimated that the heritability of hepatic fat content in NAFLD in the general population was nearly 52 percent (Loomba et al., 2015). Moreover, genetic variations found in the genome-wide association study (GWAS) also govern the development of NAFLD (Dongiovanni et al., 2015; Anstee et al., 2020; Trepo and Valenti, 2020). The single nucleotide polymorphisms (SNPs) strongly related to the accumulation of triglyceride and inflammation in liver disease, i.e., rs738409 C>G polymorphism in patatin-like phospholipase domain containing 3 (PNPLA3), rs58542926 C>T variant in transmembrane 6 superfamily member 2 (TM6SF2), and rs641738C>T in membrane-bound O-acyltransferase domain-containing 7 (MBOAT7) were the most robust and well-replicated locus (Eslam et al., 2018). Notably, the distribution of the G allele in PNPLA3 and the T allele of TM6SF2 was much higher in lean NAFLD than in non-lean NAFLD (Feldman et al., 2017; Chen et al., 2020). Except the above SNPs involved in the progression of NAFLD or lean NAFLD, other potential candidate SNPs’ actions in the treatment of NAFLD or lean NAFLD are also worth investigating, which would provide some clues for the clarification of the pathogenesis of lean NAFLD.
Instead of drugs, physical exercise and energy intake control are prioritized for the treatment of NAFLD. According to the finding, lean NAFLD patients had high fructose, and cholesterol intake (Wong et al., 2018), and the genes strongly linked with glucose or lipid metabolism captured much attention [e.g., insulin receptor substrate 1 (IRS1) and leptin (LEP)]. As an energy-sensing enzyme, AMP-activated protein kinase (AMPK) seems to perform a vital role in preventing NAFLD (Smith et al., 2016). The activation of the AMPK a2 subunit in the liver can decrease blood glucose levels and inhibit gluconeogenesis (Foretz et al., 2005). Moreover, the deletion of AMPK can reduce the thermogenesis of brown adipose tissue and exacerbate insulin resistance and hepatic steatosis (Desjardins and Steinberg, 2018). Yet the role of the genetic variant in genes clustered in AMPK signaling pathway of lean NAFLD was still unclear.
In this study, we focused on the NAFLD-related genes [e.g., TBC1 domain family member 1, (TBC1D1)] enriched in the AMPK signaling pathway and aimed to examine the association between sixteen common polymorphisms and the risk of lean NAFLD in the elderly Chinese Han population. This knowledge may help identify the genetic variations contributing to the development of lean NAFLD and imply the strategy for the prevention and control of lean NAFLD.
Materials and methods
Subjects
Data were collected in a cross-sectional survey among 5387 residents (aged ≥60 years) in the Zhangjiang community center, Shanghai, China, in 2017. The study was approved by the Ethics Committee of Shanghai University of Traditional Chinese Medicine. Written informed consent was provided before the study. The study followed the declaration of Helsinki.
The residents in Shanghai who can complete the data measurements were included in this study. And participants with mental disorders, malignant tumors, or incomplete recorded information would be excluded. After an initial screening, 5338 potential subjects were included. Then, 1449 participants were randomly chosen for the genotyping analysis. However, 230 participants who lacked BMI data, abused alcohol (<140 g/week in males and <70 g/week in females), were carriers of hepatitis B or C, or had a history of drug-induced liver disease or autoimmune liver disease were excluded. Ultimately, 1219 participants (NAFLD, n = 750; non-NAFLD, n = 469) were included in the final analysis. According to the classification in adult Asian populations, lean NAFLD in this study was defined by body mass index (BMI) < 23 kg/m2 (Fan et al., 2017; Vilarinho et al., 2021). Finally, participants were categorized into four groups: lean NAFLD (BMI <23 kg/m2, n = 106), non-lean NAFLD (BMI ≥23 kg/m2, n = 644), lean non-NAFLD (BMI <23 kg/m2, n = 216) and non-lean non-NAFLD (BMI ≥23 kg/m2, n = 253) (Figure 1).
Measurement
A color ultrasound system was used to diagnose NAFLD (Fan et al., 2011) by two experienced radiologists, and it can be defined by the presence of at least two of three abnormal findings: diffusely increased liver near field ultrasound echo (‘bright liver’), liver echo greater than kidney, vascular blurring and the gradual attenuation of far field ultrasound echo. Information, i.e., age, gender, alcohol consumption, smoking, and medical history, was acquired by questionnaire. BMI was calculated as weight (kg) divided by height squared (m2). A non-stretch tape was used for the measurement of waist and hip circumference (WC/HC) by the trained professional. Electronic sphygmomanometers (Biospace, Cheonan, South Korea) were used to measure diastolic and systolic blood pressure (DBP and SBP). A biochemistry analyzer (Hitachi, Tokyo, Japan) was applied for testing fasting glucose, alanine transaminase (ALT), aspartate transaminase (AST), total cholesterol (TC), low-density lipoprotein (LDL), high-density lipoprotein (HDL) and triglyceride (TG).
Kyoto encyclopedia of genes and genomes (KEGG) pathway analysis
KEGG pathway analysis was performed in the online software DAVID (Sherman et al., 2022) and a p-value less than .05 was considered statistically significant. 116 NAFLD-related candidate genes, which cover 179 SNPs (Supplementary Table S1) were included in the preliminary selection for the enrichment analysis. Due to the vital roles of the AMPK signaling pathway in NAFLD (Smith et al., 2016), only seven genes [TBC1D1, IRS1, LEP, adiponectin, C1Q and collagen domain-containing (ADIPOQ), leptin receptor (LEPR), peroxisome proliferator-activated receptor gamma (PPARG) and sirtuin (SIRT1)] involved in the AMPK signaling pathway were described in detail in the following genotyping section to clarify the biological function of genetic variation occurring in the specific genes of lean NAFLD.
Genotyping
The genomic DNA was extracted from peripheral venous blood using the standard phenol-chloroform method for genotyping by collecting 5 mL of blood from each subject. Sixteen SNPs including: rs2279026 and rs72279028 in TBC1D1, rs1801278 in IRS1, rs3828942 in LEP, rs182052, rs6773957, rs3774261 and rs17366568 in ADIPOQ, rs11208659, rs12409877, rs1805094 and rs4655537 in LEPR, rs1801282 in PPARG, rs11599176, rs12413112 and rs33957861 in SIRT1 from NCBI database of SNP database were analyzed. Matrix-assisted laser desorption/ionization time-off light mass spectrometer in MassARRAY Analyzer 4 platforms (Sequenom, San Diego, CA) was used for genotyping. Probes and primers were designed with online Assay Design Suite version 2.0 software. The polymerase chain reaction was performed according to the instructions of the manufacturers. More detailed information about primers and polymerase chain reaction conditions is available upon request.
Statistical analysis
Basic traits in participants were presented as mean and standard deviation (SD). Independent samples t-test was adopted for the group comparison. Categorical data were calculated as a percentage. Non-normally distributed data were analyzed by converting log to normally distributed data, and non-parametric testing, i.e., Mann-Whitney U, was used for data that cannot be converted into normally distributed data. Allelic and genotypic distributions and Hardy-Weinberg equilibrium were analyzed with the online software SHEsis (http://analysis.bio-x.cn/myAnalysis.php) (Shi and He, 2005).
“SNPassoc” R package (Gonzalez et al., 2007) was applied for the association analysis between SNPs with phenotypes in five genetic models (codominant, dominant, recessive, over-dominant, and log-additive models, respectively). To verify the association of HDL with rs2279028 or ALT/LDL/TC with rs17366568 in lean NAFLD, the logistic regression analysis was used. Only those statistically significant variables in both genetic association and regression analysis will be included in the subsequent mediation analysis. Mediation models conducted with mediation package in R software were used to explore whether specific phenotypes can mediate the association between SNPs and the risk of lean NAFLD. A recommended procedure with 95% confidence intervals of 1,000 bias-corrected and accelerated bootstrapping analyses were used to assess the significance of mediation effects. p < .05 was considered significant in this study.
Results
Demographics of study participants
Demographics of the lean participants are presented in Table 1 and Figure 2. Females constituted 64.2 and 58.8 percent of lean NAFLD (average age: 72.5 years) and lean non-NAFLD participants (average age: 73.5 years), respectively. And males accounted for 35.8 and 41.2 percent of lean NAFLD and lean non-NAFLD participants, respectively. In lean NAFLD patients, the weight, BMI, WC, and HC level was much higher than in lean non-NAFLD individuals (p < .05). And the serum trait, i.e., fasting glucose, TC, LDL, TG, and ALT, were statistically greater in lean NAFLD compared with lean non-NAFLD individuals (p < .05). By contrast, HDL was much lower in lean NAFLD than in lean non-NAFLD individuals (p < .05).
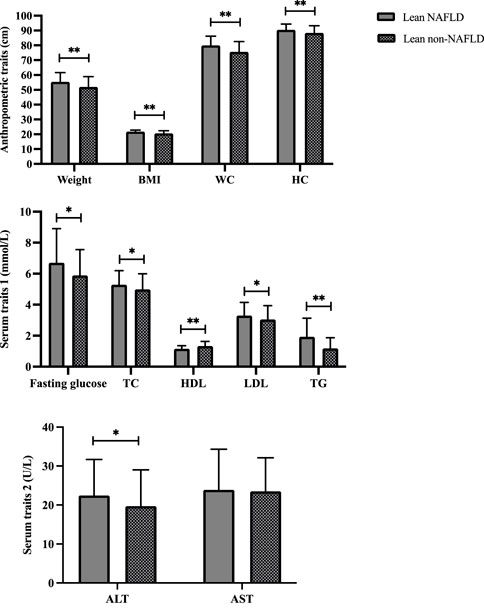
FIGURE 2. Demographics of lean NAFLD and lean non-NAFLD participants. ALT, Alanine aminotransferase; AST, Aspartate aminotransferase; BMI, Body mass index; HDL, High-density lipoprotein; LDL, Low-density lipoprotein; NAFLD, Non-alcoholic fatty liver disease; TC, Total cholesterol; TG, Triglyceride. * and ** indicate p < 0.05 and p < 0.001, respectively.
KEGG enrichment analysis based on the candidate SNPs related to NAFLD
According to KEGG pathway analysis, the candidate genes (SNPs) were significantly enriched in eight pathways related to NAFLD (p < .05) (Table 2). Among them, the AMPK signaling pathway, which includes TBC1D1, IRS1, LEP, ADIPOQ, LEPR, PPARG, and SIRT1 genes, was the most significant (p < .001). As the AMPK signaling pathway plays an essential role in the development of NAFLD (Smith et al., 2016; Carling, 2017), we next focused on the sixteen SNPs in the seven genes mentioned above.
Genetic association between SNPs and lean NAFLD
Sixteen tested SNPs (rs2279026 and rs72279028) in TBC1D1, (rs1801278) in IRS1, (rs3828942) in LEP, (rs182052, rs6773957, rs3774261 and rs17366568) in ADIPOQ, (rs11208659, rs12409877, rs1805094 and rs4655537) in LEPR, (rs1801282) in PPARG, (rs11599176, rs12413112 and rs33957861) in SIRT1 which met Hardy-Weinberg equilibrium (p > .05) were shown in Table 3. The frequency of above sixteen SNPs between males and females is shown in supplementary Table 2.
The allele and genotype distributions of sixteen SNPs are shown in Table 4. The A allele frequency of rs2279028 in TBC1D1 was significantly lower in lean NAFLD comparing with lean non-NAFLD individuals (OR = .603, 95%CI = .419–.866, p = .006, adjusted p = .046). The genotypic frequency of rs2279028 was significantly different between lean NAFLD and lean non-NAFLD individuals (p = .024). Moreover, G allele frequency of rs17366568 in ADIPOQ was lower in lean NAFLD comparing with lean non-NAFLD individuals (OR = 2.625, 95%CI = 1.020–6.752, p = .038). However, there were no significant differences between the allele or among the genotype distributions of the other fourteen SNPs (Supplementary Table 3).
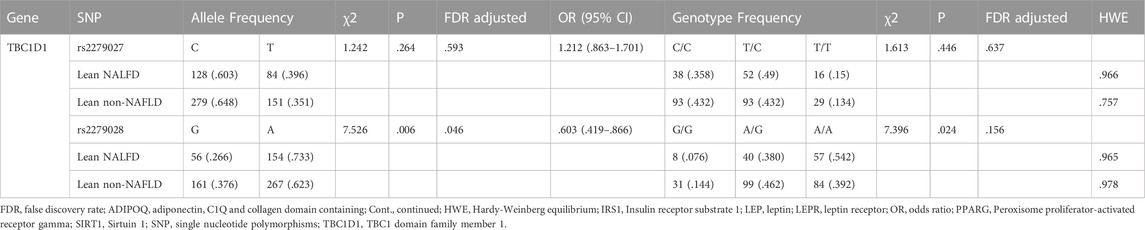
TABLE 4. Analyzed genes allele and genotype distribution in lean individuals (Cont. see Additional file 1).
Association of SNPs with specific phenotypes in lean NAFLD individuals
Only associations between two SNPs, i.e., rs2279028 in TBC1D1 and rs17366568 in ADIPOQ, and specific phenotypes, e.g., HDL, ALT, LDL, and TC, were significant. The association between the polymorphism in TBC1D1 and HDL using five genetic models is presented in Table 5. TBC1D1 polymorphism rs2279028 was significantly associated with HDL under the over-dominant model. The GA genotype of rs2279028 polymorphism was statistically related to lower HDL (p = .035). We also used rs2279028 as a logistic regression predictor to examine its associations with HDL. And rs2279028 genotype was still significant associated with HDL (β = -.084, R2 = .054, p = .009) after adjusting gender and age (Table 7).
The association between ADIPOQ SNPs and specific phenotypes using five genetic models are presented in Table 6. ADIPOQ polymorphism rs17366568 was significantly associated with ALT under the codominant, dominant and over-dominant models (p = .024, p = .023 and p = .010, respectively). Moreover, rs17366568 was significantly associated with LDL under the codominant and recessive models (p = .041 and p = .014, respectively). In addition, rs17366568 was significantly associated with TC under the recessive model (p = .032). Similarly, rs17366568 was also used as a predictor of logistic regression to examine its associations with ALT, LDL, and TC, respectively. And all the associations were significant (rs17366568 vs. ALT: β = -5.083, R2 = .082, p = .028; rs17366568 vs. LDL: β = -2.056, R2 = .066, p = .018; rs17366568 vs. TC: β = -2.023, R2 = .140, p = .029) after adjusting gender and age (Table 7).
Mediation effect of the specific phenotype on the association between SNPs and the risk of lean NAFLD
As shown in Figure 3, mediation analysis showed that rs2279026 had no significant direct effect on lean NAFLD (β = -.047, 95%CI: -.155–.060), while rs1260326 had a significant indirect effect on lean NAFLD incidence via HDL (β = -.050, 95%CI: -.094∼-.010, p = .014). In addition, ALT, LDL, and TC have no mediation effects on the association between 17366568 in ADIPOQ and the risk of lean NAFLD.
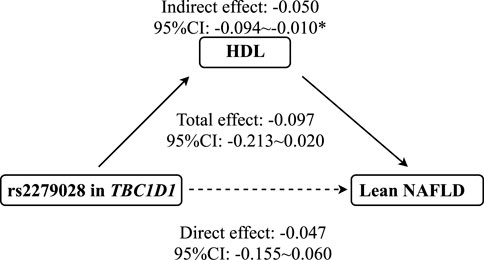
FIGURE 3. The mediation effect of HDL on the association between rs2279028 and the risk of lean NAFLD. CI, Confidence interval; HDL, High-density lipoprotein; NAFLD, Non-alcoholic fatty liver disease; TBC1D1, TBC1 domain family member 1. * indicates p < 0.05.
Discussion
There were significant differences in anthropometric measures (e.g., weight, BMI, WC, and HC), serum parameters (e.g., TC, LDL, HDL, and TG), and allele and genotype distributions (e.g., rs2279028 in TBC1D1) between lean NAFLD and lean non-NAFLD participants. Moreover, polymorphisms in TBC1D1 and ADIPOQ, which are involved in the AMPK signaling pathway, were linked with cardiovascular risk factors (i.e., HDL, LDL, TC, and TG). This study reinforced the mediation effect of HDL on the association between rs2279028 in TBC1D1 and the risk of lean NAFLD in the elderly Chinese Han population for the first time.
Currently, the pathophysiology of lean NAFLD remains to be resolved incompletely. The impaired glucose tolerance with increased hepatic fat accumulation and low adiponectin concentrations has been proved in obese and lean NAFLD patients (Feldman et al., 2017). It was reported that not only does insulin resistance contribute to obese NAFLD, but lean NAFLD (Bugianesi et al., 2005). Furthermore, insulin resistance was associated with reduced HDL (Fossati and Romon-Rousseaux, 1987). Notably, the antiatherogenic factor, i.e., HDL, could activate AMPK, which is highly sensitive to glucose (Lin and Hardie, 2018). All these findings hint at the crucial role of HDL in lean NAFLD. Additionally, in cases with a lack of AMPK in adipocytes, insulin resistance and simple steatosis were triggered and aggravated (Mottillo et al., 2016). And the symptom (i.e., steatosis and inflammation) of NAFLD was improved through the AMPK signaling pathway after exercise intervention in obese mice (Diniz et al., 2021). Thus, we proposed that AMPK might play a vital role in the occurrence, development, prevention, and even treatment of lean NAFLD via regulating glucose and lipid metabolism.
Lean NAFLD might result from genetic variation in genes related to AMPK signaling due to its regulatory effect on glucose and lipid. TBC1D1 is the downstream target of AMPK, and glucose uptake by AMPK in skeletal muscle needs the phosphorylation of TBC1D1 (Thomas et al., 2018). And ADIPOQ deficiency might lead to insulin resistance and NAFLD by mediating the AMPK signaling pathway (Shehzad et al., 2012). But more than that, different SNPs in NAFLD-related genes, e.g., PNPLA3, ADIPOQ, and TBC1D1, appear to significantly influence the development of lean NAFLD. Zou et al. found that the number of lean NAFLD patients carrying rs738409 polymorphism in PNPLA3 was much greater than obese NAFLD and lean controls (Zou et al., 2020). In addition, Hsiao et al. reported that rs266729 in ADIPOQ was associated with TC in obese subjects but not in non-obese ones (Hsiao and Lin, 2016). Of note, Apalasamy et al. confirmed that rs17366568 in ADIPOQ was related to the risk of obesity (Apalasamy et al., 2014). Although the association between polymorphism in ADIPOQ and the risk of lean NAFLD failed to fit the mediation model, our study still showed that rs17366568 was significantly correlated with TC, LDL, and TG after the regression analysis, which is in agreement with the previous findings. Researchers have implied that TBC1D1 could determine fat and muscle deposition. Knuppel et al. demonstrated that rs637797 in TBC1D1 was strongly linked with BMI (Knuppel et al., 2013). And Fontanesi et al. explored the association between the genetic variant in TBC1D1 and fat thickness and lean mass (Fontanesi et al., 2011). Chadt et al. showed that mutation of TBC1D1 could inhibit obesity by promoting the utilization of lipids (Chadt et al., 2008). Consistently, our study showed that rs1260328 polymorphism in TBC1D1 was associated with the risk of lean NAFLD via HDL, which further partially supported the above assumption.
The advantage of this study is that it is the first time to examine the mediation effect of HDL on the association between rs2279028 and the risk of lean NAFLD in the elderly Chinese Han population. And this research on further pathogenesis mechanism of lean NAFLD has great significance. The limitation of this study is that the mediation effect of HDL on the association between rs2279028 and the risk of lean NAFLD was acquired only based on the elderly Chinese Han population; more similar studies should be conducted to validate the finding in this manuscript which should cover different populations with more sample sizes, diverse age, ethics, regions and environmental exposure factors for avoiding the potential confounding factors. In addition, GGT, as a key marker of liver injury, should also be tested for reflecting hepatocellular damage.
Conclusion
In conclusion, this study indicated that rs2279028 in TBC1D1 may contribute to the progression of lean NAFLD through HDL. This finding presents more evidence for further exploring the mechanism of lean NAFLD, and suggests the possible strategy for the prevention and treatment of lean NAFLD.
Data availability statement
The datasets presented in this study can be found in online repositories. The names of the repository/repositories and accession number(s) can be found below: https://pan.baidu.com/s/1LgXoUgWFZ162l8zE8cyv_w, 1rc3.
Ethics statement
The studies involving human participants were reviewed and approved by the Ethics Committee of Shanghai University of Traditional Chinese Medicine. All participants provided informed written consent before the study (2017LCSY069). The patients/participants provided their written informed consent to participate in this study.
Author contributions
BL and GH designed research; NW, FY, JL, DL, and JW conducted research; NW and XZ analyzed and interpreted data; NW wrote the paper; BL, GH, GJ, LZ, and YS reviewed the manuscript critically. None of the authors reported a conflict of interest related to the study. All authors have read and agreed to the published version of the manuscript.
Funding
This study was supported by Three-year action plan for Shanghai [project number: ZY (2021-2023)-0211], National Natural Science Foundation of China (81973730), Local Colleges Faculty Constitution of Shanghai MSTC 2022 (22010504300), Shanghai Collaborative Innovation Center for Chronic Disease Prevention and Health Services (2021 Science and Technology 02-37), Shanghai Health Commission for Traditional Chinese Medicine Research (2022QN014), China Postdoctoral Science Foundation, No. 72 General Fund, 2022 (2022M722164).
Acknowledgments
The authors would like to thank all study participants.
Conflict of interest
The authors declare that the research was conducted in the absence of any commercial or financial relationships that could be construed as a potential conflict of interest.
Publisher’s note
All claims expressed in this article are solely those of the authors and do not necessarily represent those of their affiliated organizations, or those of the publisher, the editors and the reviewers. Any product that may be evaluated in this article, or claim that may be made by its manufacturer, is not guaranteed or endorsed by the publisher.
Supplementary material
The Supplementary Material for this article can be found online at: https://www.frontiersin.org/articles/10.3389/fgene.2022.1026725/full#supplementary-material
SUPPLEMENTARY FIGURE S1 | Mediation effect of variables on the association between rs17366568 in ADIPOQ and the risk of lean NAFLD.
SUPPLEMENTARY TABLE S1 | 179 SNPs included in this study (see the online repository).
SUPPLEMENTARY TABLE S2 | The frequency of above sixteen SNPs between males and females.
SUPPLEMENTARY TABLE S3 | Cont. Analyzed genes allele and genotype distribution in lean individuals.
References
Anstee, Q. M., Darlay, R., Cockell, S., Meroni, M., Govaere, O., Tiniakos, D., et al. (2020). Genome-wide association study of non-alcoholic fatty liver and steatohepatitis in a histologically characterised cohort. J. Hepatol. 73, 505–515. doi:10.1016/j.jhep.2020.04.003
Apalasamy, Y. D., Rampal, S., Salim, A., Moy, F. M., Bulgiba, A., and Mohamed, Z. (2014). Association of ADIPOQ gene with obesity and adiponectin levels in Malaysian Malays. Mol. Biol. Rep. 41, 2917–2921. doi:10.1007/s11033-014-3147-0
Bugianesi, E., Gastaldelli, A., Vanni, E., Gambino, R., Cassader, M., Baldi, S., et al. (2005). Insulin resistance in non-diabetic patients with non-alcoholic fatty liver disease: Sites and mechanisms. Diabetologia 48, 634–642. doi:10.1007/s00125-005-1682-x
Carling, D. (2017). AMPK signalling in health and disease. Curr. Opin. Cell Biol. 45, 31–37. doi:10.1016/j.ceb.2017.01.005
Chadt, A., Leicht, K., Deshmukh, A., Jiang, L. Q., Scherneck, S., Bernhardt, U., et al. (2008). Tbc1d1 mutation in lean mouse strain confers leanness and protects from diet-induced obesity. Nat. Genet. 40, 1354–1359. doi:10.1038/ng.244
Chen, F., Esmaili, S., Rogers, G. B., Bugianesi, E., Petta, S., Marchesini, G., et al. (2020). Lean NAFLD: A distinct entity shaped by differential metabolic adaptation. Hepatology 71, 1213–1227. doi:10.1002/hep.30908
Desjardins, E. M., and Steinberg, G. R. (2018). Emerging role of AMPK in Brown and beige adipose tissue (BAT): Implications for obesity, insulin resistance, and type 2 diabetes. Curr. Diab Rep. 18, 80. doi:10.1007/s11892-018-1049-6
Diniz, T. A., de Lima Junior, E. A., Teixeira, A. A., Biondo, L. A., da Rocha, L. A. F., Valadao, I. C., et al. (2021). Aerobic training improves NAFLD markers and insulin resistance through AMPK-PPAR-alpha signaling in obese mice. Life Sci. 266, 118868. doi:10.1016/j.lfs.2020.118868
Dongiovanni, P., Romeo, S., and Valenti, L. (2015). Genetic factors in the pathogenesis of nonalcoholic fatty liver and steatohepatitis. Biomed. Res. Int. 2015, 460190. doi:10.1155/2015/460190
Eslam, M., Chen, F., and George, J. (2020). NAFLD in lean Asians. Clin. Liver Dis. Hob. 16, 240–243. doi:10.1002/cld.930
Eslam, M., and George, J. (2016). Genetic and epigenetic mechanisms of NASH. Hepatol. Int. 10, 394–406. doi:10.1007/s12072-015-9689-y
Eslam, M., Valenti, L., and Romeo, S. (2018). Genetics and epigenetics of NAFLD and NASH: Clinical impact. J. Hepatol. 68, 268–279. doi:10.1016/j.jhep.2017.09.003
Fan, J. G., Jia, J. D., Li, Y. M., Wang, B. Y., Lu, L. G., Shi, J. P., et al. (2011). Guidelines for the diagnosis and management of nonalcoholic fatty liver disease: Update 2010: (published in Chinese on Chinese journal of hepatology 2010; 18:163-166. J. Dig. Dis. 12, 38–44. doi:10.1111/j.1751-2980.2010.00476.x
Fan, J. G., Kim, S. U., and Wong, V. W. (2017). New trends on obesity and NAFLD in Asia. J. Hepatol. 67, 862–873. doi:10.1016/j.jhep.2017.06.003
Feldman, A., Eder, S. K., Felder, T. K., Kedenko, L., Paulweber, B., Stadlmayr, A., et al. (2017). Clinical and metabolic characterization of lean caucasian subjects with non-alcoholic fatty liver. Am. J. Gastroenterol. 112, 102–110. doi:10.1038/ajg.2016.318
Fontanesi, L., Colombo, M., Tognazzi, L., Scotti, E., Buttazzoni, L., Dall'Olio, S., et al. (2011). The porcine TBC1D1 gene: Mapping, SNP identification, and association study with meat, carcass and production traits in Italian heavy pigs. Mol. Biol. Rep. 38, 1425–1431. doi:10.1007/s11033-010-0247-3
Foretz, M., Ancellin, N., Andreelli, F., Saintillan, Y., Grondin, P., Kahn, A., et al. (2005). Short-term overexpression of a constitutively active form of AMP-activated protein kinase in the liver leads to mild hypoglycemia and fatty liver. Diabetes 54, 1331–1339. doi:10.2337/diabetes.54.5.1331
Fossati, P., and Romon-Rousseaux, M. (1987). Insulin and HDL-cholesterol metabolism. Diabete Metab. 13, 390–394.
Gonzalez, J. R., Armengol, L., Sole, X., Guino, E., Mercader, J. M., Estivill, X., et al. (2007). SNPassoc: an R package to perform whole genome association studies. Bioinformatics 23, 644–645. doi:10.1093/bioinformatics/btm025
Hsiao, T. J., and Lin, E. (2016). A validation study of adiponectin rs266729 gene variant with type 2 diabetes, obesity, and metabolic phenotypes in a Taiwanese population. Biochem. Genet. 54, 830–841. doi:10.1007/s10528-016-9760-y
Knuppel, S., Rohde, K., Meidtner, K., Drogan, D., Holzhutter, H. G., Boeing, H., et al. (2013). Evaluation of 41 candidate gene variants for obesity in the EPIC-Potsdam cohort by multi-locus stepwise regression. PLoS One 8, e68941. doi:10.1371/journal.pone.0068941
Lin, S. C., and Hardie, D. G. (2018). Ampk: Sensing glucose as well as cellular energy status. Cell Metab. 27, 299–313. doi:10.1016/j.cmet.2017.10.009
Loomba, R., Schork, N., Chen, C. H., Bettencourt, R., Bhatt, A., Ang, B., et al. (2015). Heritability of hepatic fibrosis and steatosis based on a prospective twin study. Gastroenterology 149, 1784–1793. doi:10.1053/j.gastro.2015.08.011
Mottillo, E. P., Desjardins, E. M., Crane, J. D., Smith, B. K., Green, A. E., Ducommun, S., et al. (2016). Lack of adipocyte AMPK exacerbates insulin resistance and hepatic steatosis through Brown and beige adipose tissue function. Cell Metab. 24, 118–129. doi:10.1016/j.cmet.2016.06.006
Shehzad, A., Iqbal, W., Shehzad, O., and Lee, Y. S. (2012). Adiponectin: Regulation of its production and its role in human diseases. Horm. (Athens) 11, 8–20. doi:10.1007/BF03401534
Sherman, B. T., Hao, M., Qiu, J., Jiao, X., Baseler, M. W., Lane, H. C., et al. (2022). DAVID: A web server for functional enrichment analysis and functional annotation of gene lists (2021 update). Nucleic Acids Res. 50, W216–W221. doi:10.1093/nar/gkac194
Shi, Y. Y., and He, L. (2005). SHEsis, a powerful software platform for analyses of linkage disequilibrium, haplotype construction, and genetic association at polymorphism loci. Cell Res. 15, 97–98. doi:10.1038/sj.cr.7290272
Smith, B. K., Marcinko, K., Desjardins, E. M., Lally, J. S., Ford, R. J., and Steinberg, G. R. (2016). Treatment of nonalcoholic fatty liver disease: Role of AMPK. Am. J. Physiol. Endocrinol. Metab. 311, E730–E740. doi:10.1152/ajpendo.00225.2016
Thomas, E. C., Hook, S. C., Gray, A., Chadt, A., Carling, D., Al-Hasani, H., et al. (2018). Isoform-specific AMPK association with TBC1D1 is reduced by a mutation associated with severe obesity. Biochem. J. 475, 2969–2983. doi:10.1042/BCJ20180475
Trepo, E., and Valenti, L. (2020). Update on NAFLD genetics: From new variants to the clinic. J. Hepatol. 72, 1196–1209. doi:10.1016/j.jhep.2020.02.020
Vilarinho, S., Ajmera, V., Zheng, M., and Loomba, R. (2021). Emerging role of genomic analysis in clinical evaluation of lean individuals with NAFLD. Hepatology 74, 2241–2250. doi:10.1002/hep.32047
Wong, V. W., Wong, G. L., Chan, R. S., Shu, S. S., Cheung, B. H., Li, L. S., et al. (2018). Beneficial effects of lifestyle intervention in non-obese patients with non-alcoholic fatty liver disease. J. Hepatol. 69, 1349–1356. doi:10.1016/j.jhep.2018.08.011
Younossi, Z., Anstee, Q. M., Marietti, M., Hardy, T., Henry, L., Eslam, M., et al. (2018). Global burden of NAFLD and NASH: Trends, predictions, risk factors and prevention. Nat. Rev. Gastroenterol. Hepatol. 15, 11–20. doi:10.1038/nrgastro.2017.109
Keywords: lean NAFLD, TBC1D1 gene, high-density lipoprotein, mediation effect, polymorphisms
Citation: Wu N, Zhai X, Yuan F, Li J, Li D, Wang J, Zhang L, Shi Y, Ji G, He G and Liu B (2023) Genetic variation in TBC1 domain family member 1 gene associates with the risk of lean NAFLD via high-density lipoprotein. Front. Genet. 13:1026725. doi: 10.3389/fgene.2022.1026725
Received: 01 September 2022; Accepted: 23 December 2022;
Published: 12 January 2023.
Edited by:
Gilberto Vargas Alarcón, Instituto Nacional de Cardiologia Ignacio Chavez, MexicoReviewed by:
Berenice Palacios-Gonzalez, Instituto Nacional de Medicina Genómica (INMEGEN), MexicoUmberto Vespasiani-Gentilucci, Campus Bio-Medico University, Italy
Copyright © 2023 Wu, Zhai, Yuan, Li, Li, Wang, Zhang, Shi, Ji, He and Liu. This is an open-access article distributed under the terms of the Creative Commons Attribution License (CC BY). The use, distribution or reproduction in other forums is permitted, provided the original author(s) and the copyright owner(s) are credited and that the original publication in this journal is cited, in accordance with accepted academic practice. No use, distribution or reproduction is permitted which does not comply with these terms.
*Correspondence: Guang Ji, jg@shutcm.edu.cn; Guang He, heguangbiox@163.com; Baocheng Liu, baochliu_lab@163.com, baochliu@shutcm.edu.cn
†These authors have contributed equally to this work