- 1National Engineering Laboratory of Animal Breeding, Key Laboratory of Animal Genetics, Department of Animal Genetics and Breeding, Breeding and Reproduction of Ministry of Agriculture and Rural Affairs, College of Animal Science and Technology, China Agricultural University, Beijing, China
- 2Beijing Dairy Cattle Center, Beijing, China
- 3Yantai Institute, China Agricultural University, Yantai, China
Our previous work had confirmed that pyruvate kinase L/R (PKLR) gene was expressed differently in different lactation periods of dairy cattle, and participated in lipid metabolism through insulin, PI3K-Akt, MAPK, AMPK, mTOR, and PPAR signaling pathways, suggesting that PKLR is a candidate gene to affect milk production traits in dairy cattle. Here, we verified whether this gene has significant genetic association with milk yield and composition traits in a Chinese Holstein cow population. In total, we identified 21 single nucleotide polymorphisms (SNPs) by resequencing the entire coding region and partial flanking region of PKLR gene, in which, two SNPs were located in 5′ promoter region, two in 5′ untranslated region (UTR), three in introns, five in exons, six in 3′ UTR and three in 3′ flanking region. The single marker association analysis displayed that all SNPs were significantly associated with milk yield, fat and protein yields or protein percentage (p ≤ 0.0497). The haplotype block containing all the SNPs, predicted by Haploview, had a significant association with fat yield and protein percentage (p ≤ 0.0145). Further, four SNPs in 5′ regulatory region and eight SNPs in UTR and exon regions were predicted to change the transcription factor binding sites (TFBSs) and mRNA secondary structure, respectively, thus affecting the expression of PKLR, leading to changes in milk production phenotypes, suggesting that these SNPs might be the potential functional mutations for milk production traits in dairy cattle. In conclusion, we demonstrated that PKLR had significant genetic effects on milk production traits, and the SNPs with significant genetic effects could be used as candidate genetic markers for genomic selection (GS) in dairy cattle.
Introduction
Milk is rich in nutrition and is an important food for the human body to obtain many essential nutrients. Fat and protein in milk have the characteristics of easy digestion and absorption, especially for children and the elderly, so the content and proportion of fat and protein in milk is of great significance. Studies have shown that drinking milk can reduce the incidence of dental caries (Rumbold et al., 2021), cardiovascular disease (Soedamah-Muthu and de Goede 2018), metabolic syndrome (Crichton et al., 2011) and obesity (Abargouei et al., 2012). Dairy cattle breeding is essential for the development of the dairy industry and human health. In dairy cattle breeding, one of the most important thing is to study the milk production traits, milk yield, fat yield, and percentage, and protein yield and percentage, which are quantitative traits and controlled by multiple minor polygenes, a few main efficient genes and greatly affected by the environment (Schrooten et al., 2000). However, the process of traditional breeding is very slow and unable to meet the growing consumer demand.
Meuwissen et al. (2001) first proposed genomic selection (GS) in 2001, which can better reflect the problem of minorgenes for quantitative traits (Wiggans et al., 2011). Especially for animals such as dairy cattle with long generation interval, GS can effectively shorten their generation interval and accelerate genetic progress (Stock and Reents 2013). Since 2009, GS has been formally applied to dairy cattle breeding, which has brought revolutionary changes to dairy cattle breeding (Wiggans et al., 2017). SNP (single nucleotide polymorphism) chips designed with SNP probes based on large-scale SNP genotype data to detect genomic polymorphism (Heffner et al., 2009) were used in GS to select target traits. In recent years, with the development of SNP chip technology, GS has been widely used in dairy cattle breeding (Jiang et al., 2013; Jiang et al., 2016). Through GS, a single marker whose effect is small can be captured (Goddard and Hayes 2007). Additionally, studies have shown that adding functional site information with large genetic effects on target traits can improve the accuracy of GS (Zhang et al., 2014; Brondum et al., 2015; Zhang et al., 2015; de Las Heras-Saldana et al., 2020). Therefore, in recent years, researchers have been using various methods such as quantitative trait locus (QTL) mapping, candidate gene analysis, genome-wide association study (GWAS) and high throughput omics strategy to explore functional genes and mutations related to milk production traits, so as to improve the accuracy of GS and accelerate the process of molecular breeding of dairy cattle (Gebreyesus et al., 2019; Lopdell et al., 2019; Liu et al., 2020; Korkuc et al., 2021). At present, in terms of milk producing traits of dairy cattle, many genes such as CDKN1A, FADS2, PRLR, SLC2A12, and SLC5A1 had been verified to be associated with milk yield and composition traits of Holstein cows (Maryam et al., 2015; Han et al., 2017; Yan et al., 2018; Shi et al., 2019; Valsalan et al., 2021; Zwierzchowski et al., 2021; Fu et al., 2022).
Previously, we obtained liver transcriptome data of Chinese Holstein cows at different lactations, and found that pyruvate kinase L/R (PKLR) gene was differentially expressed during periods and participated in lipid metabolism through insulin, PI3K-Akt, MAPK, AMPK, mTOR, and PPAR signaling pathways, suggesting that PKLR gene may play an important role for milk fat trait of dairy cattle (Liang et al., 2017). PKLR is involved in glycogen and lipid metabolisms in liver tissues (Wang et al., 2000; Ahrens et al., 2013), and has a wide association with a spectrum of liver damage from steatosis and inflammation to fibrosis via its regulation on mitochondrial dysfunction and subsequent hepatic triglyceride accumulation (Chella Krishnan et al., 2021). In addition, PKLR (chr.3: 15344765-15354042) is located 0.02 Mb to the peak of QTL regions for milk fat percentage (QTL_ID: 104486) and protein percentage (QTL_ID:104816, 104938) (Nayeri et al., 2016). Therefore, we considered this gene to be a potential candidate gene for milk producing traits in dairy cows.
Herein, we identified SNPs of the PKLR gene in a Chinese Holstein population and analyzed their genetic associations with milk yield, fat yield, fat percentage, protein yield and protein percentage. Further, we predicted the potential biological effects of identified SNPs on transcription factor binding site (TFBS) and mRNA secondary structure. The purpose of this study is to provide valuable SNP loci information for dairy GS, and also to provide some reference information for the in-depth study of the mechanism of candidate genes related to milk production traits in dairy cattle.
Materials and methods
Animals and phenotypic data
In this study, we used a total of 925 Chinese Holstein cows from 44 sire families for association analyses, and these cows were distributed in 21 dairy farms belonging to the Beijing Shounong Animal Husbandry Development Co., Ltd. (Beijing, China), where the cows were healthy with the same feeding conditions and had accurate pedigree information and standard dairy herd improvement (DHI) records. We used the phenotypic data of 925 cows in the first lactation and 633 in the second lactation (292 cows merely completed the milking of first lactation) for the association analyses and mainly analyzed five milk production traits, including 305-days milk yield, fat yield, fat percentage, protein yield and protein percentage. The descriptive statistics of phenotypic values for dairy production traits of the first and second lactations were presented in Supplementary Table S1.
DNA extraction
The Beijing Dairy Cattle Center (Beijing, China) provides frozen semen of the 44 bulls and blood samples of 925 cows that were stored at −20°C for genomic DNA extraction. We extracted frozen semen DNAs by salt-out procedure, and extracted DNAs of blood samples by a TIANamp Blood DNA Kit (Tiangen, Beijing, China). Then, we used NanoDrop 2000 Spectrophotometer (Thermo Scientific, Hudson, NH, United States) and the gel electrophoresis to determine the quantity and quality of the extracted DNAs, respectively.
SNP identification and genotyping
According to the sequences of bovine PKLR gene (NC_037330) from GenBank (https://www.ncbi.nlm.nih.gov/genbank/), we used Primers3 (https://primer3.ut.ee/) to design the primers (Supplementary Table S2) in this gene’s coding region, parts of intron region and 2,000 bp of upstream and downstream regions. The primers were synthesized by Beijing Genomics Institute (BGI, Beijing, China). We mixed the semen DNAs equally, amplified them by PCR (Supplementary Table S3), and detected the PCR amplification products using 2% gel electrophoresis before Sanger sequencing by BGI. After sequencing, we identified the potential SNPs according to the reference sequences (ARS-UCD1.2) on NCBI-BLAST (https://blast.ncbi.nlm.nih.gov/Blast.cgi). Subsequently, we genotyped the identified SNPs in 925 cows using Genotyping by Target Sequencing (GBTS) technology by Boruidi Biotechnology Co., Ltd. (Hebei, China).
Linkage disequilibrium estimation and association analyses
We used Haploview4.2 (Broad Institute of MIT and Harvard, Cambridge, MA, United States) to estimate the extent of linkage disequilibrium (LD) between the identified SNPs.
The MIXED process in SAS 9.4 (SAS Institute Inc., Cary, NC, United States) software was used to carry out association analyses between the genotypes/haplotype blocks and the five milk production traits, milk yield, fat yield, fat percentage, protein yield, and protein percentage, on the first and second lactations. The following animal model was used for the association analysis:
Additionally, we calculated the additive effect (a), dominant effect (d), and substitution effect (α) by the following formulas:
Functional prediction of mutation sites
We predicted changes of TFBSs for the SNPs located in the 5′ region of PKLR gene by the MEME Suite (http://meme-suite.org/). We used RNAfold Web Server (http://rna.tbi.univie.ac.at/cgi-bin/RNAWebSuite/RNAfold.cgi) to predict changes in secondary structures of mRNA for SNPs in UTR and exon regions. The minimum free energy (MFE) of the optimal secondary structure reflects the stability of mRNA structure. The lower the MFE value, the more stable the mRNA structure is.
Results
SNPs identification
In this study, we totally found 21 SNPs in PKLR gene, all of which had been reported previously. Two SNPs, 3:g.15342877C>T and 3:g.15344349A>C, were located in 5′ promoter region, two (3:g.15345216C>T and 3:g.15345227T>C) in 5′ untranslated region (UTR), three (3:g.15349740A>G, 3:g.15350548C>T and 3:g.15350805T>C) in introns, five (3:g.15349768A>G, 3:g.15349978A>G, 3:g.15350655A>G, 3:g.15350898T>C and 3:g.15352855T>C) in exons, six (3:g.15353088A>C, 3:g.15353235T>C, 3:g.15353254T>C, 3:g.15353292C>G, 3:g.15353330A>G and 3:g.15353342C>T) in 3′ UTR, and three (3:g.15355389T>C, 3:g.15355514T>C and 3:g.15355833A>G) in 3′ flanking region. All the five SNPs in the exons were synonymous mutations (Table 1). The genotypic and allelic frequencies of all the identified SNPs were summarized in Table 1.
Associations between SNPs and five milk productions traits
We analyzed the associations between the 21 SNPs in PKLR and five milk production traits in dairy cattle. In the first lactation, there were four, nineteen, four and seventeen SNPs significantly associated with milk yield, fat yield, protein yield and protein percentage, respectively (p ≤ 0.0497; Table 2). Four SNPs, 3:g.15350898T>C, 3:g.15355389T>C, 3:g.15355514T>C and 3:g.15355833A>G, had extremely significant genetic effects on milk, fat and protein yields (p ≤ 0.0044), and 3:g.15355389T>C and 3:g.15355514T>C were also significantly associated with protein percentage (p ≤ 0.0374). As for the second lactation, there were sixteen, twenty and eighteen SNPs were significantly associated with milk yield, fat yield and protein percentage (p ≤ 0.0436), respectively. Additionally, thirteen SNPs were significantly associated with milk yield, fat yield and protein percentage (p ≤ 0.0063). During two lactation periods, six SNPs, 3:g.15353292C>G, 3:g.15353330A>G, 3:g.15353342C>T, 3:g.15355389T>C, 3:g.15355514T>C and 3:g.15355833A>G, had significant genetic effects on fat yield (p ≤ 0.0097). In addition, the results of allelic additive, dominant and substitution effects of the SNPs in PKLR gene were displayed in Supplementary Table S4.
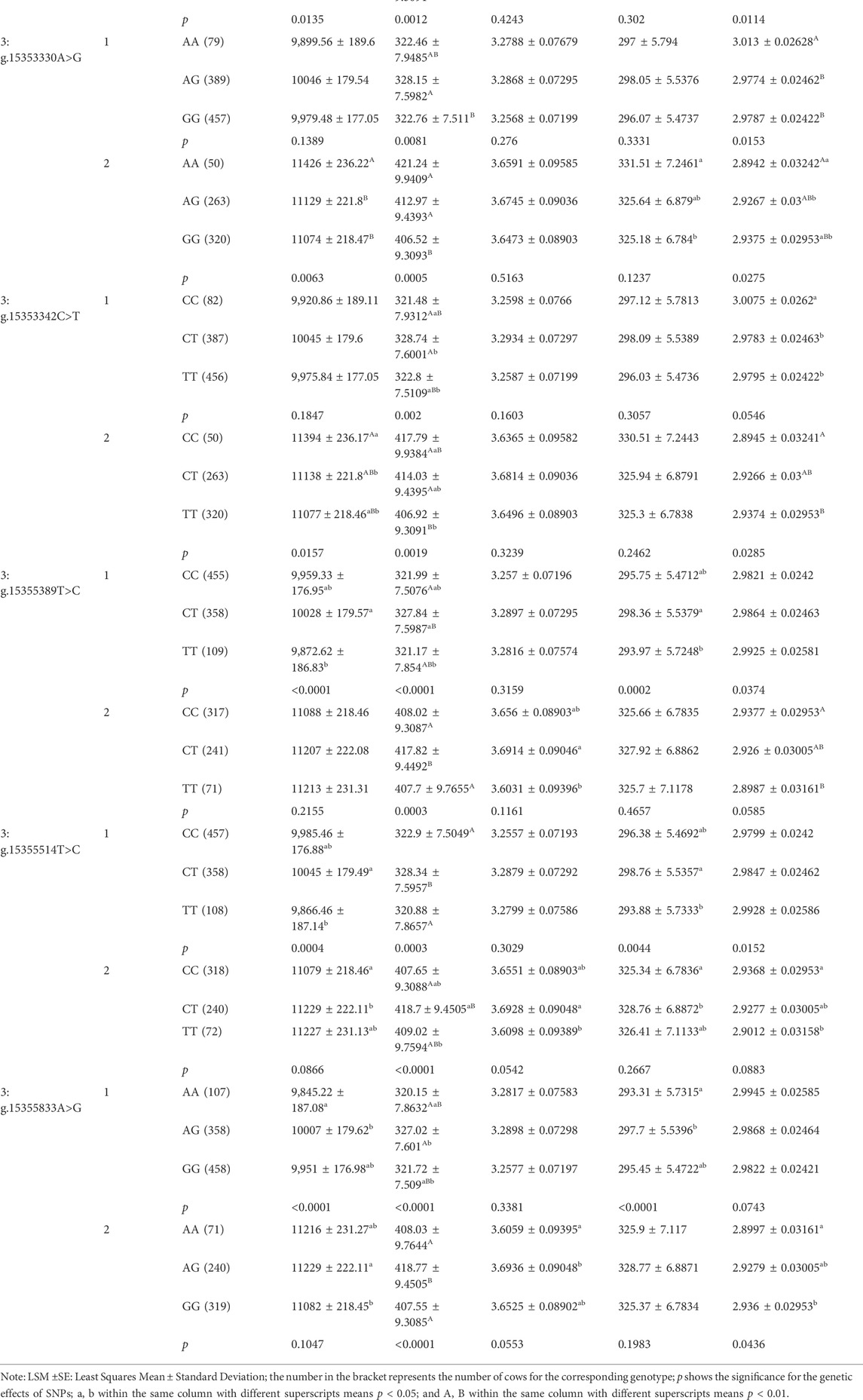
TABLE 2. Associations of 21 SNPs in PKLR with milk production traits in two lactations of Chinese Holstein cows (LSM ±SE).
Associations between haplotype block and five milk productions traits
We estimated the degree of linkage disequilibrium (LD) among the 21 identified SNPs in PKLR gene using Haploview4.2, and inferred one haplotype block including all the SNPs (Figure 1). The block consisted of four haplotypes, H1 (TCTCGGGTGCTCCCCGGTCCG), H2 (CACTAAACATTTATTCACTTA), H3 (TCTCGGGTGCCCCCCGGTCCG), and H4 (TCTCGGGTGCTCCCCGGTTTA) with the frequencies of 0.499, 0.287, 0.181, and 0.021, respectively. The haplotype combinations demonstrated significant associations with fat yield and protein percentage in the first and second lactations (p ≤ 0.0145), and milk yield (p = 0.0003) and protein yield (p = 0.0183) in the second lactation (Supplementary Table S5).
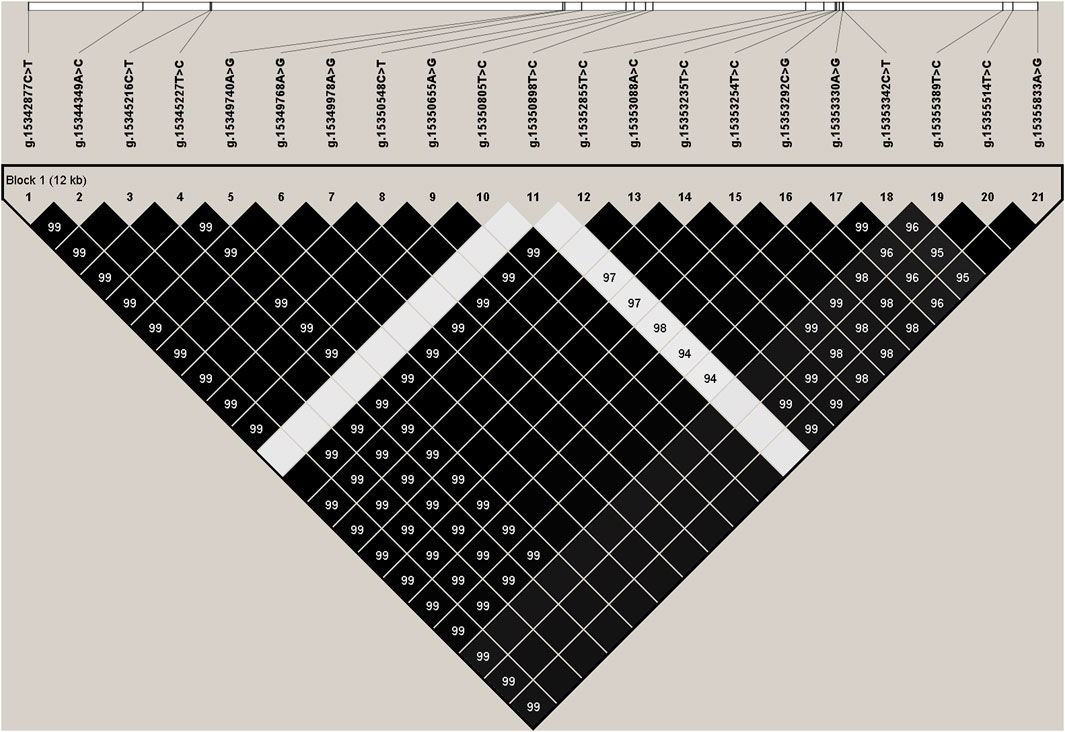
FIGURE 1. Linkage disequilibrium estimated between SNPs in PKLR gene. The values in the black boxes are pair-wise SNP correlations (R2).
Regulation of the 5′ region SNPs on transcriptional activity
We used the MEME Suite software to predict the changes of TFBSs caused by the four SNPs on the 5′ regulatory region of PKLR gene. The detailed results were shown in Table 3. The allele C of 3:g.15342877C>T created binding sites (BSs) for transcription factors (TFs) SP100 and ESRRA. In 3:g.15344349A>C, allele A created BSs for three TFs, MLX, ZBTB33 and IRF5, and the allele C created the BSs for ZNF524, YY2, and SREBF2. As for 3:g.15345216C>T, the allele C invented BS for RREB1, the allele T invented BSs for TWIST2, ZEB1, NAC007, BHLHE22, ZFP42, TCF3, NAC031, ZSCAN31, and TCF12. The allele C of 3:g.15345227T>C created BSs for TFs MYC, TFAP2A and TCF4.
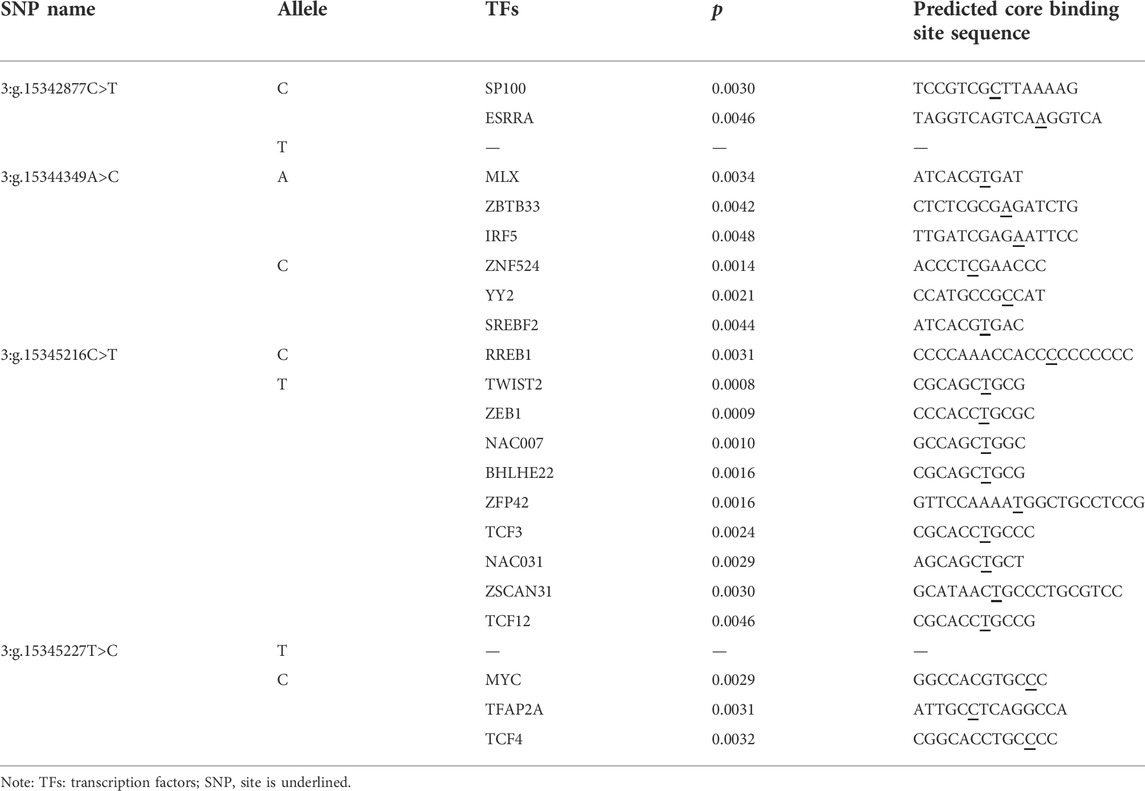
TABLE 3. Changes in transcription factors binding sites (TFBSs) caused by the SNPs in 5′ regulatory region of PKLR.
Prediction of changes in secondary structures of mRNA
We used the RNAfold Web Server to predict the changes of secondary structures of mRNA for thirteen SNPs in UTR and exon regions of PKLR gene. All the thirteen SNP mutation sites were predicted to change the MFE of mRNA secondary structures compared to the MFE of reference sequence (XM_024989616.1; ARS-UCD1.2; Table 4). Among them, six sites, 3:g.15345216T, 3:g.15345227C, 3:g.15349768G, 3:g.15350898C, 3:g.15353235C and 3:g.15353254C, could increase the MFE to cause the instability of PKLR mRNA secondary structure, and the other seven sites, 3:g.15349978G, 3:g.15350655G, 3:g.15352855C, 3:g.15353088C, 3:g.15353292G, 3:g.15353330G, and 3:g.15353342T, could decrease the MFE and make the mRNA secondary structure more stable.
Discussion
Our previous study considered PKLR gene to be a candidate to affect milk production traits in dairy cattle (Liang et al., 2017). In this study, we identified totally 21 SNPs in PKLR gene, and found that all the SNPs were significantly associated with at least one milk production trait, simultaneously, the results of haplotype association analysis were basically consistent with the single marker association analysis, which suggested that the PKLR gene had large genetic effect on milk production traits. Brondum et al. (2015) added the sequence data of a few significant variation into the conventional 54k SNPs for single marker analysis, and found it can improve the reliability of genomic prediction, for instance, the reliability of the Nordic Holstein cattle milk production traits increased by 4%, that of Nordic red bull increased by 3%, and that of France Holstein cows increased by 5%. Currently, four commercial gene chips, including illumina Bovine SNP50K BeadChip, illumina BovineHD Genotyping BeadChip, GeneSeek Genomic Profiler (GGP) Bovine 150K, and 100K arrays, do not contain SNPs identified in this study, after that, we could try to add significant functional SNPs in this study to gene chips to improve the accuracy of genomic prediction in dairy cattle.
PKLR converts phosphoenolpyruvic acid to pyruvate, the main carbon source, and its perturbation may significantly affect the pyruvate levels in cells (Liu et al., 2019). Moreover, pyruvate is an important intermediate in the glucose metabolism of all living organisms and the mutual transformation of various substances in the body. It can also convert sugars, fats and amino acids into each other through acetyl CoA and the tricarboxylic acid cycle (Gray et al., 2014). Studies have shown that PKLR regulates and influences key metabolic pathways related to lipid metabolism, steroid biosynthesis, PPAR signaling pathway, fatty acid synthesis and oxidation (Lee et al., 2017; Mardinoglu et al., 2018; Liu et al., 2019). It can be seen that PKLR gene can regulate the synthesis of milk components, especially milk lactose and fat.
Transcription factors are a group of protein molecules that bind to TFBSs to ensure that the target gene is expressed at a specific intensity at a specific time and space (Jolma et al., 2013). When the mutation site changes that it will affect the binding of TFs to TFBSs, and then inhibiting or enhancing gene expression (Spivakov et al., 2012). In this study, four SNPs in 5′ region of PKLR were predicted to change the TFBSs that would be affect the expression of the downstream gene. For the 3:g.15342877C>T, the allele C could bind SP100 and ESRRA, and the milk and fat yields of CC genotype cows was significantly higher than that of TT individuals. In addition, it has reported that ESRRA enhanced the transcriptional activation of numerous autophagy-related (Atg) genes, Atg5, Atg16l1, and Becn1 (Kim et al., 2018). SP100 may function as a nuclear hormone receptor transcriptional coactivator (Bloch et al., 2000). It can be inferred that the increase of CC genotype phenotype may be due to the combination of transcription factors SP100 and ESRRA at the C site, which together activate the expression of gene PKLR. The allele A in 3:g.15344349A>C could bind MLX, ZBTB33, and IRF5, and the allele C binds ZNF524, YY2, and SREBF2, meanwhile, the milk and fat yields of AA genotype cows was significantly higher than that of CC individuals. MLX plays a role in transcriptional activation of glycolytic target genes and the Mondo family (Billin et al., 2000; Sans et al., 2006). ZBTB33 activated transcription from exogenous methylated promoters (Zhenilo et al., 2018). IRF5 directly activated transcription of the genes IL-12p40, IL-12p35, and IL-23p19 and contributed to the plasticity of macrophage polarization (Krausgruber et al., 2011). YY2 reduces the activity of the c-Myc and CXCR4 promoter (Nguyen et al., 2004). SREBF2 can activate the transcription of genes involved in cholesterol biosynthesis (Xu et al., 2020; Sellers et al., 2021). The functional role of TF ZNF524 is unclear so far. It is speculated that the higher milk yield of AA genotype cows may be the result of combined activation of transcription factors MLX, ZBTB33 and IRF5 or the inhibition of ZNF524, YY2, and SREBF2 on the expression of gene PKLR. For the 3:g.15345216C>T, the allele C binds RREB1, and allele T could bind TWIST2, ZEB1, NAC007, BHLHE22, ZFP42, TCF3, NAC031, ZSCAN31, and TCF12, as well as, the milk and fat yields of CC genotype cows was significantly higher than that of TT individuals. RREB1 is a transcriptional activator of calcitonin in response to Ras signaling (Deng et al., 2020). TWIST2 can suppress the expression of FGF21 to activate the AMPK/mTOR signalling pathway which inhibits the progression of various cancers (Song et al., 2021). ZEB1 as a direct transcriptional repressor of E-cadherin by physically binding to the proximal promoter of E-cadherin in breast cancers (Eger et al., 2005). BHLHE22 is a transcriptional repressor and is involved in cell differentiation in neuron development (Ross et al., 2012; Darmawi et al., 2022), TCF3 combined with HDAC3 down-regulates the expression of miR-101 that is a type of tumor suppressor gene, thereby promoting the proliferation of BL cells and inhibiting their apoptosis (Dong et al., 2021). TCF12 functions as transcriptional repressor of E-cadherin (Lee et al., 2012). The function of some transcription factors, NAC007, ZFP42, NAC031, and ZSCAN31, is still unclear. Therefore, it can be speculated that the increased phenotype of CC genotype individuals may be caused by the activation of PKLR gene expression by binding the TF RREB1, or the co-inhibition of PKLR gene expression by TFs TWIST2, ZEB1, NAC007, BHLHE22, ZFP42, TCF3, NAC031, ZSCAN31, and TCF12. For the 3:g.15345227T>C, the allele C could bind MYC, TFAP2A and TCF4, and the milk and fat yields of CC genotype cows was significantly lower than that of TT individuals. MYC represses transcription when tethered to promoters by Miz1 or other proteins (Adhikary and Eilers 2005). TFAP2A appeared to strengthen the binding of Smad2/3 to target promoters and affect transcriptional responses in knockdown experiments (Koinuma et al., 2009). TCF4 is involved in the initiation of neuronal differentiation by binding to the E box to activate transcription (Teixeira et al., 2021). It can be speculated that the decrease of CC genotype phenotype may be due to the combination of TFs MYC, TFAP2A, and TCF4 to inhibit the expression of PKLR gene. Thus, we speculated that these four SNP mutations changed the TFBSs to modulate the gene expression of PKLR, resulting in changes of phenotypic data.
The secondary structure of mRNA is formed by the complementary pairing of bases on the single chain, and the same mRNA molecules can be folded to form a variety of configurations. The secondary structure of mRNA, as the skeleton of the higher functions of RNA, plays an important role in various life processes, including protein folding and transport, initiation and extension of translation process, regulation of translation rate and direct influence the stability of mRNA itself (Wan et al., 2011; Dethoff et al., 2012). The base change of SNP may change the secondary structure of mRNA, so we used RNAfold to predict the secondary structure of mRNA, and MFE was used as an indicator to measure the stability of the secondary structure in this study. Five sites, 3:g.15345216T, 3:g.15349768G, 3:g.15350898C, 3:g.15353235C, and 3:g.15353254C, with higher MFEs compared that to the reference sites, caused the instability of PKLR mRNA secondary structure to inhibit its expression, additionally, our study found that the five loci were significantly associated with milk fat yield, and the phenotypic value of fat yield of homozygous individuals at the mutation site was significantly reduced. On the contrary, three sites, 3:g.15353292G, 3:g.15353330G, and 3:g.15353342T, had lower MFEs and more stable mRNA structure, also had significant genetic effects on fat yield, and the phenotypes of fat yield of homozygous cows at these sites were significant increased. It suggested that these eight SNP sites might affect milk fat yield of dairy cows by influencing the instability of mRNA secondary structure of PKLR. Further, we speculated that the changes of mRNA secondary structures caused by SNPs may affect the stability of its higher-order structure and gene expression, leading to an influence on milk production phenotypes of dairy cows.
Conclusion
In summary, a total of 21 SNPs were identified in PKLR gene, and their significant genetic associations with milk production traits of dairy cows have been confirmed. Eleven SNPs might be the potential causal mutations for the milk production traits in dairy cattle that needs more in-depth validation, of which, 3:g.15342877C>T, 3:g.15344349A>C, 3:g.15345216C>T, and 3:g.15345227T>C might change the TFBSs to regulate expression of the PKLR gene, and eight SNPs, 3:g.15345216C>T, 3:g.15349768A>G, 3:g.15350898T>C, 3:g.15353235T>C, 3:g.15353254T>C, 3:g.15353292C>G, 3:g.15353330A>G, and 3:g.15353342C>T, could change the secondary structure of mRNA and the phenotypic value of fat yield. The valuable SNPs could be used as candidate genetic markers for dairy cattle molecular breeding for the development of GS chip.
Data availability statement
The original contributions presented in the study are included in the article/Supplementary Material, further inquiries can be directed to the corresponding author.
Ethics statement
The animal study was reviewed and approved by the Institutional Animal Care and Use Committee (IACUC) at China Agricultural University. Written informed consent was obtained from the owners for the participation of their animals in this study.
Author contributions
BH, DS, and KC: conceptualization, methodology, and funding acquisition. LX and YL: formal analysis. FZ: investigation and resources. AD: visualization. AD: writing—original draft preparation. BH: writing, review and editing. All authors contributed to the article and approved the submitted version.
Funding
This work was financially supported by Shandong Provincial Natural Science Foundation (ZR2020MC165), National Natural Science Foundation of China (32072716, 31872330), China Agriculture Research System of MOF and MARA (CARS-36), and the Program for Changjiang Scholar and Innovation Research Team in University (IRT_15R62).
Acknowledgments
We appreciate Beijing Dairy Cattle Center for providing the semen and blood samples and phenotypic data.
Conflict of interest
The authors declare that the research was conducted in the absence of any commercial or financial relationships that could be construed as a potential conflict of interest.
Publisher’s note
All claims expressed in this article are solely those of the authors and do not necessarily represent those of their affiliated organizations, or those of the publisher, the editors and the reviewers. Any product that may be evaluated in this article, or claim that may be made by its manufacturer, is not guaranteed or endorsed by the publisher.
Supplementary material
The Supplementary Material for this article can be found online at: https://www.frontiersin.org/articles/10.3389/fgene.2022.1002706/full#supplementary-material
References
Abargouei, A. S., Janghorbani, M., Salehi-Marzijarani, M., and Esmaillzadeh, A. (2012). Effect of dairy consumption on weight and body composition in adults: a systematic review and meta-analysis of randomized controlled clinical trials. Int. J. Obes. 36, 1485–1493. doi:10.1038/ijo.2011.269
Adhikary, S., and Eilers, M. (2005). Transcriptional regulation and transformation by Myc proteins. Nat. Rev. Mol. Cell Biol. 6, 635–645. doi:10.1038/nrm1703
Ahrens, M., Ammerpohl, O., von Schonfels, W., Kolarova, J., Bens, S., Itzel, T., et al. (2013). DNA methylation analysis in nonalcoholic fatty liver disease suggests distinct disease-specific and remodeling signatures after bariatric surgery. Cell Metab. 18, 296–302. doi:10.1016/j.cmet.2013.07.004
Billin, A. N., Eilers, A. L., Coulter, K. L., Logan, J. S., and Ayer, D. E. (2000). MondoA, a novel basic helix-loop-helix-leucine zipper transcriptional activator that constitutes a positive branch of a max-like network. Mol. Cell. Biol. 20, 8845–8854. doi:10.1128/mcb.20.23.8845-8854.2000
Bloch, D. B., Nakajima, A., Gulick, T., Chiche, J. D., Orth, D., de La Monte, S. M., et al. (2000). Sp110 localizes to the PML-Sp100 nuclear body and may function as a nuclear hormone receptor transcriptional coactivator. Mol. Cell. Biol. 20, 6138–6146. doi:10.1128/mcb.20.16.6138-6146.2000
Brondum, R. F., Su, G., Janss, L., Sahana, G., Guldbrandtsen, B., Boichard, D., et al. (2015). Quantitative trait loci markers derived from whole genome sequence data increases the reliability of genomic prediction. J. Dairy Sci. 98, 4107–4116. doi:10.3168/jds.2014-9005
Chella Krishnan, K., Floyd, R. R., Sabir, S., Jayasekera, D. W., Leon-Mimila, P. V., Jones, A. E., et al. (2021). Liver pyruvate kinase promotes NAFLD/NASH in both mice and humans in a sex-specific manner. Cell. Mol. Gastroenterol. Hepatol. 11, 389–406. doi:10.1016/j.jcmgh.2020.09.004
Crichton, G. E., Bryan, J., Buckley, J., and Murphy, K. J. (2011). Dairy consumption and metabolic syndrome: a systematic review of findings and methodological issues. Obes. Rev. 12, e190–201. doi:10.1111/j.1467-789X.2010.00837.x
Darmawi, , Chen, L. Y., Su, P. H., Liew, P. L., Wang, H. C., Weng, Y. C., Huang, R. L., et al. (2022). BHLHE22 expression is associated with a proinflammatory immune microenvironment and confers a favorable prognosis in endometrial cancer. Int. J. Mol. Sci. 23, 7158. doi:10.3390/ijms23137158
de Las Heras-Saldana, S., Lopez, B. I., Moghaddar, N., Park, W., Park, J. E., Chung, K. Y., et al. (2020). Use of gene expression and whole-genome sequence information to improve the accuracy of genomic prediction for carcass traits in Hanwoo cattle. Genet. Sel. Evol. 52, 54. doi:10.1186/s12711-020-00574-2
Deng, Y. N., Xia, Z., Zhang, P., Ejaz, S., and Liang, S. (2020). Transcription factor RREB1: From target genes towards biological functions. Int. J. Biol. Sci. 16, 1463–1473. doi:10.7150/ijbs.40834
Dethoff, E. A., Chugh, J., Mustoe, A. M., and Al-Hashimi, H. M. (2012). Functional complexity and regulation through RNA dynamics. Nature 482, 322–330. doi:10.1038/nature10885
Dong, L., Huang, J., Zu, P., Liu, J., Gao, X., Du, J., et al. (2021). Transcription factor 3 (TCF3) combined with histone deacetylase 3 (HDAC3) down-regulates microRNA-101 to promote Burkitt lymphoma cell proliferation and inhibit apoptosis. Bioengineered 12, 7995–8005. doi:10.1080/21655979.2021.1977557
Eger, A., Aigner, K., Sonderegger, S., Dampier, B., Oehler, S., Schreiber, M., et al. (2005). DeltaEF1 is a transcriptional repressor of E-cadherin and regulates epithelial plasticity in breast cancer cells. Oncogene 24, 2375–2385. doi:10.1038/sj.onc.1208429
Fu, Y., Jia, R., Xu, L., Su, D., Li, Y., Liu, L., et al. (2022). Fatty acid desaturase 2 affects the milk-production traits in Chinese Holsteins. Anim. Genet. 53, 422–426. doi:10.1111/age.13192
Gebreyesus, G., Buitenhuis, A. J., Poulsen, N. A., Visker, M., Zhang, Q., van Valenberg, H. J. F., et al. (2019). Multi-population GWAS and enrichment analyses reveal novel genomic regions and promising candidate genes underlying bovine milk fatty acid composition. BMC Genomics 20, 178. doi:10.1186/s12864-019-5573-9
Goddard, M. E., and Hayes, B. J. (2007). Genomic selection. J. Anim. Breed. Genet. 124, 323–330. doi:10.1111/j.1439-0388.2007.00702.x
Gray, L. R., Tompkins, S. C., and Taylor, E. B. (2014). Regulation of pyruvate metabolism and human disease. Cell. Mol. Life Sci. 71, 2577–2604. doi:10.1007/s00018-013-1539-2
Han, B., Liang, W., Liu, L., Li, Y., and Sun, D. (2017). Determination of genetic effects of ATF3 and CDKN1A genes on milk yield and compositions in Chinese Holstein population. BMC Genet. 18, 47. doi:10.1186/s12863-017-0516-4
Heffner, E. L., Sorrells, M. E., and Jannink, J. L. (2009). Genomic selection for crop improvement. Crop Sci. 49, 1–12. doi:10.2135/cropsci2008.08.0512
Jiang, L., Jiang, J., Yang, J., Liu, X., Wang, J., Wang, H., et al. (2013). Genome-wide detection of copy number variations using high-density SNP genotyping platforms in Holsteins. BMC Genomics 14, 131. doi:10.1186/1471-2164-14-131
Jiang, J., Gao, Y., Hou, Y., Li, W., Zhang, S., Zhang, Q., et al. (2016). Whole-genome resequencing of Holstein bulls for indel discovery and identification of genes associated with milk composition traits in dairy cattle. PLoS One 11, e0168946. doi:10.1371/journal.pone.0168946
Jolma, A., Yan, J., Whitington, T., Toivonen, J., Nitta, K. R., Rastas, P., et al. (2013). DNA-binding specificities of human transcription factors. Cell 152, 327–339. doi:10.1016/j.cell.2012.12.009
Kim, S. Y., Yang, C. S., Lee, H. M., Kim, J. K., Kim, Y. S., Kim, Y. R., et al. (2018). ESRRA (estrogen-related receptor alpha) is a key coordinator of transcriptional and post-translational activation of autophagy to promote innate host defense. Autophagy 14, 152–168. doi:10.1080/15548627.2017.1339001
Koinuma, D., Tsutsumi, S., Kamimura, N., Taniguchi, H., Miyazawa, K., Sunamura, M., et al. (2009). Chromatin immunoprecipitation on microarray analysis of Smad2/3 binding sites reveals roles of ETS1 and TFAP2A in transforming growth factor beta signaling. Mol. Cell. Biol. 29, 172–186. doi:10.1128/MCB.01038-08
Korkuc, P., Arends, D., May, K., Konig, S., and Brockmann, G. A. (2021). Genomic loci affecting milk production in German black pied cattle (DSN). Front. Genet. 12, 640039. doi:10.3389/fgene.2021.640039
Krausgruber, T., Blazek, K., Smallie, T., Alzabin, S., Lockstone, H., Sahgal, N., et al. (2011). IRF5 promotes inflammatory macrophage polarization and TH1-TH17 responses. Nat. Immunol. 12, 231–238. doi:10.1038/ni.1990
Lee, C. C., Chen, W. S., Chen, C. C., Chen, L. L., Lin, Y. S., Fan, C. S., et al. (2012). TCF12 protein functions as transcriptional repressor of E-cadherin, and its overexpression is correlated with metastasis of colorectal cancer. J. Biol. Chem. 287, 2798–2809. doi:10.1074/jbc.M111.258947
Lee, S., Zhang, C., Liu, Z., Klevstig, M., Mukhopadhyay, B., Bergentall, M., et al. (2017). Network analyses identify liver-specific targets for treating liver diseases. Mol. Syst. Biol. 13, 938. doi:10.15252/msb.20177703
Liang, R., Han, B., Li, Q., Yuan, Y., Li, J., and Sun, D. (2017). Using RNA sequencing to identify putative competing endogenous RNAs (ceRNAs) potentially regulating fat metabolism in bovine liver. Sci. Rep. 7, 6396. doi:10.1038/s41598-017-06634-w
Liu, Z., Zhang, C., Lee, S., Kim, W., Klevstig, M., Harzandi, A. M., et al. (2019). Pyruvate kinase L/R is a regulator of lipid metabolism and mitochondrial function. Metab. Eng. 52, 263–272. doi:10.1016/j.ymben.2019.01.001
Liu, L., Zhou, J., Chen, C. J., Zhang, J., Wen, W., Tian, J., et al. (2020). GWAS-based identification of new loci for milk yield, fat, and protein in Holstein cattle. Animals. 10, 2048. doi:10.3390/ani10112048
Lopdell, T. J., Tiplady, K., Couldrey, C., Johnson, T. J. J., Keehan, M., Davis, S. R., et al. (2019). Multiple QTL underlie milk phenotypes at the CSF2RB locus. Genet. Sel. Evol. 51, 3. doi:10.1186/s12711-019-0446-x
Mardinoglu, A., Uhlen, M., and Boren, J. (2018). Broad views of non-alcoholic fatty liver disease. Cell Syst. 6, 7–9. doi:10.1016/j.cels.2018.01.004
Maryam, J., Babar, M. E., Nadeem, A., Yaqub, T., and Hashmi, A. S. (2015). Identification of functional consequence of a novel selection signature in CYP11b1 gene for milk fat content in Bubalus bubalis. Meta Gene 6, 85–90. doi:10.1016/j.mgene.2015.09.002
Meuwissen, T. H., Hayes, B. J., and Goddard, M. E. (2001). Prediction of total genetic value using genome-wide dense marker maps. Genetics 157, 1819–1829. doi:10.1093/genetics/157.4.1819
Nayeri, S., Sargolzaei, M., Abo-Ismail, M. K., May, N., Miller, S. P., Schenkel, F., et al. (2016). Genome-wide association for milk production and female fertility traits in Canadian dairy Holstein cattle. BMC Genet. 17, 75. doi:10.1186/s12863-016-0386-1
Nguyen, N., Zhang, X., Olashaw, N., and Seto, E. (2004). Molecular cloning and functional characterization of the transcription factor YY2. J. Biol. Chem. 279, 25927–25934. doi:10.1074/jbc.M402525200
Ross, S. E., McCord, A. E., Jung, C., Atan, D., Mok, S. I., Hemberg, M., et al. (2012). Bhlhb5 and Prdm8 form a repressor complex involved in neuronal circuit assembly. Neuron 73, 292–303. doi:10.1016/j.neuron.2011.09.035
Rumbold, P., McCullogh, N., Boldon, R., Haskell-Ramsay, C., James, L., Stevenson, E., et al. (2021). The potential nutrition-physical- and health-related benefits of cow's milk for primary-school-aged children. Nutr. Res. Rev. 35, 50–69. doi:10.1017/s095442242100007x
Sans, C. L., Satterwhite, D. J., Stoltzman, C. A., Breen, K. T., and Ayer, D. E. (2006). MondoA-mlx heterodimers are candidate sensors of cellular energy status: mitochondrial localization and direct regulation of glycolysis. Mol. Cell. Biol. 26, 4863–4871. doi:10.1128/MCB.00657-05
Schrooten, C., Bovenhuis, H., Coppieters, W., and Van Arendonk, J. A. M. (2000). Whole genome scan to detect quantitative trait loci for conformation and functional traits in dairy cattle. J. Dairy Sci. 83, 795–806. doi:10.3168/jds.S0022-0302(00)74942-3
Sellers, J., Brooks, A., Fernando, S., Westenberger, G., Junkins, S., Smith, S., et al. (2021). Fasting-Induced upregulation of MKP-1 modulates the hepatic response to feeding. Nutrients 13, 3941. doi:10.3390/nu13113941
Shi, L., Liu, L., Lv, X., Ma, Z., Yang, Y., Li, Y., et al. (2019). Polymorphisms and genetic effects of PRLR, MOGAT1, MINPP1 and CHUK genes on milk fatty acid traits in Chinese Holstein. BMC Genet. 20, 69. doi:10.1186/s12863-019-0769-1
Soedamah-Muthu, S. S., and de Goede, J. (2018). Dairy consumption and cardiometabolic diseases: systematic review and updated meta-analyses of prospective cohort studies. Curr. Nutr. Rep. 7, 171–182. doi:10.1007/s13668-018-0253-y
Song, Y., Zhang, W., Zhang, J., You, Z., Hu, T., Shao, G., et al. (2021). TWIST2 inhibits EMT and induces oxidative stress in lung cancer cells by regulating the FGF21-mediated AMPK/mTOR pathway. Exp. Cell Res. 405, 112661. doi:10.1016/j.yexcr.2021.112661
Spivakov, M., Akhtar, J., Kheradpour, P., Beal, K., Girardot, C., Koscielny, G., et al. (2012). Analysis of variation at transcription factor binding sites in Drosophila and humans. Genome Biol. 13, R49. doi:10.1186/gb-2012-13-9-r49
Stock, K. F., and Reents, R. (2013). Genomic selection: Status in different species and challenges for breeding. Reprod. Domest. Anim. 48, 2–10. doi:10.1111/rda.12201
Teixeira, J. R., Szeto, R. A., Carvalho, V. M. A., Muotri, A. R., and Papes, F. (2021). Transcription factor 4 and its association with psychiatric disorders. Transl. Psychiatry 11, 19. doi:10.1038/s41398-020-01138-0
Valsalan, J., Sadan, T., Venkatachalapathy, T., Anilkumar, K., and Aravindakshan, T. V. (2021). Identification of novel single-nucleotide polymorphism at exon1 and 2 region of B4GALT1 gene and its association with milk production traits in crossbred cattle of Kerala, India. Anim. Biotechnol. 10, 1–9. doi:10.1080/10495398.2020.1866591
Wan, Y., Kertesz, M., Spitale, R. C., Segal, E., and Chang, H. Y. (2011). Understanding the transcriptome through RNA structure. Nat. Rev. Genet. 12, 641–655. doi:10.1038/nrg3049
Wang, H., Maechler, P., Antinozzi, P. A., Hagenfeldt, K. A., and Wollheim, C. B. (2000). Hepatocyte nuclear factor 4alpha regulates the expression of pancreatic beta -cell genes implicated in glucose metabolism and nutrient-induced insulin secretion. J. Biol. Chem. 275, 35953–35959. doi:10.1074/jbc.M006612200
Wiggans, G. R., Vanraden, P. M., and Cooper, T. A. (2011). The genomic evaluation system in the United States: Past, present, future. J. Dairy Sci. 94, 3202–3211. doi:10.3168/jds.2010-3866
Wiggans, G. R., Cole, J. B., Hubbard, S. M., and Sonstegard, T. S. (2017). Genomic selection in dairy cattle: the USDA experience. Annu. Rev. Anim. Biosci. 5, 309–327. doi:10.1146/annurev-animal-021815-111422
Xu, D., Wang, Z., Xia, Y., Shao, F., Xia, W., Wei, Y., et al. (2020). The gluconeogenic enzyme PCK1 phosphorylates INSIG1/2 for lipogenesis. Nature 580, 530–535. doi:10.1038/s41586-020-2183-2
Yan, W., Zhou, H., Hu, J., Luo, Y., and Hickford, J. G. H. (2018). Variation in the FABP4 gene affects carcass and growth traits in sheep. Meat Sci. 145, 334–339. doi:10.1016/j.meatsci.2018.07.007
Zhang, Z., Ober, U., Erbe, M., Zhang, H., Gao, N., He, J., et al. (2014). Improving the accuracy of whole genome prediction for complex traits using the results of genome wide association studies. PLoS One 9, e93017. doi:10.1371/journal.pone.0093017
Zhang, Z., Erbe, M., He, J., Ober, U., Gao, N., Zhang, H., et al. (2015). Accuracy of whole-genome prediction using a genetic architecture-enhanced variance-covariance matrix. G3 (Bethesda) 5, 615–627. doi:10.1534/g3.114.016261
Zhenilo, S., Deyev, I., Litvinova, E., Zhigalova, N., Kaplun, D., Sokolov, A., et al. (2018). DeSUMOylation switches Kaiso from activator to repressor upon hyperosmotic stress. Cell Death Differ. 25, 1938–1951. doi:10.1038/s41418-018-0078-7
Zwierzchowski, L., Ostrowska, M., Zelazowska, B., and Bagnicka, E. (2021). Single nucleotide polymorphisms in the bovine SLC2A12 and SLC5A1 glucose transporter genes - the effect on gene expression and milk traits of Holstein Friesian cows. Anim. Biotechnol. 6, 1–11. doi:10.1080/10495398.2021.1954934
Keywords: PKLR, milk production traits, association analysis, GS, SNP chips
Citation: Du A, Zhao F, Liu Y, Xu L, Chen K, Sun D and Han B (2022) Genetic polymorphisms of PKLR gene and their associations with milk production traits in Chinese Holstein cows. Front. Genet. 13:1002706. doi: 10.3389/fgene.2022.1002706
Received: 25 July 2022; Accepted: 12 August 2022;
Published: 02 September 2022.
Edited by:
Ibrar Muhammad Khan, Fuyang Normal University, ChinaReviewed by:
Muhammad Zahoor Khan, University of Agriculture, Dera Ismail Khan, PakistanKaiyuan Ji, Anhui Agricultural University, China
Copyright © 2022 Du, Zhao, Liu, Xu, Chen, Sun and Han. This is an open-access article distributed under the terms of the Creative Commons Attribution License (CC BY). The use, distribution or reproduction in other forums is permitted, provided the original author(s) and the copyright owner(s) are credited and that the original publication in this journal is cited, in accordance with accepted academic practice. No use, distribution or reproduction is permitted which does not comply with these terms.
*Correspondence: Bo Han, Ym9oYW5AY2F1LmVkdS5jbi9oYW5ib185OEAxMjYuY29t