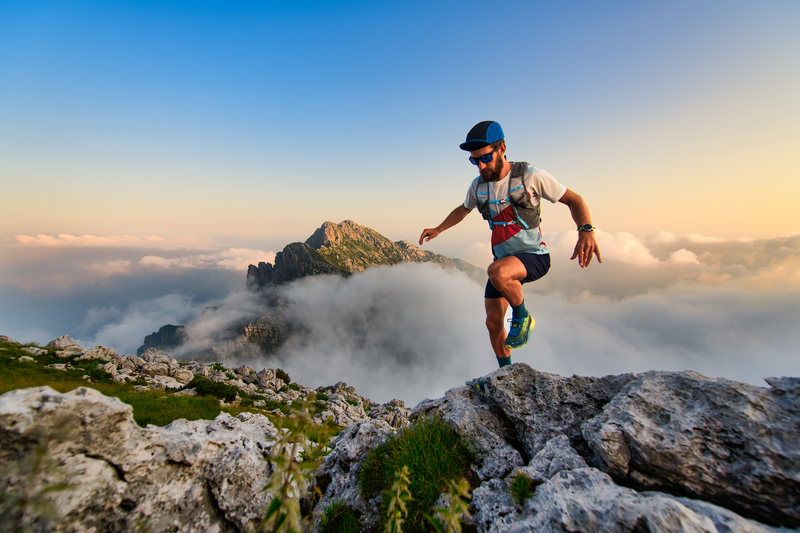
94% of researchers rate our articles as excellent or good
Learn more about the work of our research integrity team to safeguard the quality of each article we publish.
Find out more
ORIGINAL RESEARCH article
Front. Genet. , 15 September 2022
Sec. RNA
Volume 13 - 2022 | https://doi.org/10.3389/fgene.2022.1001608
This article is part of the Research Topic Machine Learning-Based Methods for RNA Data Analysis - Volume III View all 11 articles
Bladder cancer is the most common cancer of the urinary system. Bladder urothelial cancer accounts for 90% of bladder cancer. These two cancers have high morbidity and mortality rates worldwide. The identification of biomarkers for bladder cancer and bladder urothelial cancer helps in their diagnosis and treatment. circRNAs are considered oncogenes or tumor suppressors in cancers, and they play important roles in the occurrence and development of cancers. In this manuscript, we developed an Ensemble model, CDA-EnRWLRLS, to predict circRNA-Disease Associations (CDA) combining Random Walk with restart and Laplacian Regularized Least Squares, and further screen potential biomarkers for bladder cancer and bladder urothelial cancer. First, we compute disease similarity by combining the semantic similarity and association profile similarity of diseases and circRNA similarity by combining the functional similarity and association profile similarity of circRNAs. Second, we score each circRNA-disease pair by random walk with restart and Laplacian regularized least squares, respectively. Third, circRNA-disease association scores from these models are integrated to obtain the final CDAs by the soft voting approach. Finally, we use CDA-EnRWLRLS to screen potential circRNA biomarkers for bladder cancer and bladder urothelial cancer. CDA-EnRWLRLS is compared to three classical CDA prediction methods (CD-LNLP, DWNN-RLS, and KATZHCDA) and two individual models (CDA-RWR and CDA-LRLS), and obtains better AUC of 0.8654. We predict that circHIPK3 has the highest association with bladder cancer and may be its potential biomarker. In addition, circSMARCA5 has the highest association with bladder urothelial cancer and may be its possible biomarker.
Bladder cancer is considered to be the most common cancer in the urinary system (Kamat et al., 2016). It is the fourth most common malignant tumor in men and the eighth most common in women in the Western world. In the United States and Europe, it accounts for 5%–10% among all malignancies in men. The risk with the bladder cancer infection at less than 75 years is 2%–4% in men and 0.5%–1% for women (Kirkali et al., 2005). The incidence of bladder cancer has been increasing (Kamat et al., 2016). The majority of patients with bladder cancer suffer from the less aggressive non-muscle-invasive disease, while 30% of patients suffer from muscle-invasive disease (Lopez-Beltran et al., 2021; Tran et al., 2021; Yang et al., 2021).
Bladder cancer has a metastatic potential, and thus presents a worse prognosis. It is usually grouped into three pathological categories: bladder urothelial carcinoma, bladder squamous cell carcinoma, and bladder adenocarcinoma (Black and Black, 2020; Lopez-Beltran et al., 2021). Bladder urothelial carcinoma accounts for over 90% among all cases of bladder cancer. Furthermore, bladder urothelial carcinoma can be categorized into muscle-invasive bladder cancer, which accounts for about 75% of all cases, and non-muscle-invasive bladder cancer (Kirkali et al., 2005). The all-stage five-year survival rate of bladder urothelial cancer remains approximately 80% (Lopez-Beltran et al., 2021).
Recently, the treatment of bladder cancer has obtained great progresses worldwide. Besides traditional surgical resection, radiotherapy, and chemotherapy, immunotherapy is also a promising avenue for bladder cancer treatment (Gao et al., 2021; Mancini et al., 2021). However, postoperative recurrence and distant metastasis cause five-year survival rates to still be very low for advanced bladder cancer (Fabiano et al., 2021; Roviello et al., 2021). Advanced disease or relapse of radical cystectomy is closely associated with the poor outcomes (Nouhaud et al., 2021). The first-line therapy of metastatic bladder urothelial cancer usually adopts cisplatin-based combinations, and has been unaltered over the last decades (Powles et al., 2021; Renner et al., 2021; Walia et al., 2021). Unfortunately, almost all patients with bladder urothelial cancer will finally progress and die from bladder cancer, despite their initial response to cisplatin-based combinations (Bin Riaz et al., 2021; Lopez-Beltran et al., 2021). Consequently, inferring potential biomarkers for bladder cancer is a good way to diagnose and treat it (Peng et al., 2017; Peng et al., 2018).
With the advance of sequencing technology, there are now massive amounts of RNA data (Ozsolak and Milos, 2011; Peng et al., 2020; Yang et al., 2020; Peng et al., 2022a), which help the prognosis and treatment of various diseases (Xu et al., 2020; Li et al., 2021). Circular RNAs (circRNAs) are a class of single-stranded noncoding RNA molecules that are lack of terminal 5′ caps and 3′ poly(A) tails (He et al., 2017). circRNAs are widely distributed in various organisms. They have circular features, and thus demonstrate more resistance to degradation by exonucleases and stronger stability than linear RNAs (Xia et al., 2018; Li G. et al., 2020). The estimated total number of circRNAs is approximately 1% of one of poly (A) molecules. In addition, the expression levels of the majority of circRNAs are estimated to be 5%–10% of the corresponding linear RNAs (Jeck and Sharpless, 2014; Zhang J. et al., 2021).
Although circRNAs were found in 1976, they were originally considered to be functionless by-products from aberrant RNA splicing and thus did not obtain enough attention over the past 3 decades. However, with the rapid advance of high-throughput sequencing technologies, massive differentially expressed circRNAs have been increasing discovered in human normal and malignant cells (Zhang et al., 2018; Li G. et al., 2020; Yang et al., 2021). circRNAs exist widely in various tissues, serum, and urine. The expression profiles of circRNAs demonstrate strong specificity in cell types, tissues, and developmental stages (Yang et al., 2021). Furthermore, circRNAs can regulate transcription or splicing, translate proteins, interact with RNA-binding proteins, and act as miRNA sponges (Sheng et al., 2018). A large body of evidence shows that circRNAs have dense associations with various diseases, including neurological dysfunction, cardiovascular diseases, and cancer. Here, circRNAs, as miRNA sponges, can inhibit the regulation from downstream cancer target genes. For instance, circCDR1as and circMTO1 can control gene regulation and further indirectly stimulate or inhibit tumors by binding to miR-7 and miR-9 (Vromman et al., 2021).
circRNAs have abundant associations with cancers and thus can be used as candidate cancer biomarkers (Zhang et al., 2018). An increasing amount of evidence has reported that circRNAs present in human biofluids and exosomes, and are a class of potential biomarkers of noninvasive liquid biopsies. For instance, circ-ZEB1.33 is overexpressed in hepatocellular cancer and has close links with the survival of hepatocellular cancer patients (Gong et al., 2018). In particular, substantial studies have demonstrated that circRNAs play key roles in the carcinogenesis and progression of bladder cancer. For example, circRNAs Cdr1as performs anti-oncogenic functions in bladder cancer through microRNA 135a (Li et al., 2018), BCRC-3 suppresses bladder cancer proliferation via sponging miR-182-5p/p27 (Xie et al., 2018), MYLK and circPDSS1 promote bladder cancer progression separately by modulating VEGFA/VEGFR2 signaling pathway and down-regulating miR-16 (Zhong et al., 2017; Yu et al., 2020), PRMT5 supports metastasis of bladder urothelial cancer through Sponging miR-30c (Chen et al., 2018), circSLC8A1 suppresses bladder cancer progression through regulating PTEN (Lu et al., 2019), and circMTO1 inhibits bladder cancer metastasis through sponging miR-221 (Li G. et al., 2019).
Many computational methods have been proposed to identify possible CDAs and further discovered possible circRNA biomarkers for various complex diseases including cancers by case studies (Wang CC. et al., 2021). For example, Lei et al. (Lei et al., 2018) designed a path weighted-based CDA prediction approach (PWCDA). Li et al. (Li Y. et al., 2019; Li J. et al., 2020) explored two CDA identification models (NCPCDA and DWNCPCDA) based on network consistency projection. Zhang et al. (Zhang et al., 2019) developed a linear neighborhood label propagation algorithm for CDA identification. Deepthi et al. (Deepthi and Jereesh (2020) used autoencoder and deep neural network and explored an ensemble model to predict CDAs. Lu et al. (Lu et al. (2021) improved CDA prediction using convolutional and recurrent neural networks. Wang et al. (Wang et al., 2020; Wang et al. 2021b; Wang et al., 2021c) proposed three CDA identification methods (GCNCDA, MGRCDA, and SGANRDA) based on graph convolutional network, metagraph recommendation, and semi-supervised generative adversarial network, respectively. These methods efficiently predicted possible CDAs.
In this study, inspired by computational CDA prediction methods, we develop an ensemble model, CDA-RWLRLS, to find potential circRNA biomarkers for bladder cancer and bladder urothelial cancer based on known CDAs. CDA-EnRWLRLS first computes circRNA similarity by integrating their functional similarity and association profile similarity, and it computes disease similarity by integrating their semantic similarity and association profile similarity. Second, CDA-EnRWLRLS computes the association probability for each circRNA-disease pair based on random walk with restart and Laplacian regularized least squares. Third, the prediction results obtained by these two models are integrated by the soft voting method. We finally use the proposed CDA-EnRWLRLS model to identify possible circRNAs associated with bladder cancer and bladder urothelial cancer.
circRNA-disease association data can be downloaded from the circR2Disease database (Fan et al., 2018a). This database provides 739 experimentally confirmed CDAs from 661 circRNAs and 100 diseases. We remove redundant elements related to mice and rats and achieve a human circRNA-disease association dataset containing 650 associations between 585 circRNAs and 88 diseases. In particular, suppose that
Many studies have computed disease semantic similarity to screen credible noncoding RNAs for a query disease. Inspired by these methods, we investigate disease similarity to improve the prediction performance. Disease semantic similarity can be computed based on corresponding disease ontology. The disease ontology is often represented using a directed acyclic graph and can be downloaded from http://disease-ontology.org/. For two query diseases and corresponding ontology term sets from the two diseases
To measure the functional similarity between two circRNAs, we utilize the semantic similarity of two diseases linking to the two circRNAs. In particular, suppose that
and
where
In this manuscript, we develop circRNA-Disease Association prioritization method (CDA-EnRWLRLS) by an Ensemble of Random Walk with restart and Laplacian Regularization Least Squares. First, CDA-EnRWLRLS measures circRNA functional similarity and disease semantic similarity. Second, it computes association profile similarity of circRNAs and diseases, respectively. Third, functional similarity and association profile similarity of circRNAs are combined to obtain the final circRNA similarity. Similarly, disease similarity is fused. Fourth, random walk with restart and Laplacian regularization least squares are used to score each circRNA-disease pair. Fifth, the final association score matrix is obtained by integrating the results from random walk with restart and Laplacian regularization least squares based on the soft voting strategy. Finally, CDA-EnRWLRLS is applied to find possible circRNA biomarkers for bladder cancer and bladder urothelial cancer. The flowchart of CDA-EnRWLRLS is shown in Figure 1.
For two diseases with known ontology terms, we can compute their semantic similarity based on their ontology terms. However, semantic similarity computation may fail for two diseases without ontology terms. Thus, we introduce association profile similarity to further complement similarity measurement of circRNAs and diseases.
Suppose that the association profile
where
Similarly, association profile similarity between two diseases (i.e.,
where
circRNA functional similarity
The parameter
Random walk algorithm has been widely used and obtained better performance in various association prediction fields (Peng et al., 2021a). In this study, we utilize Random Walk with Restart for CDA prediction on the heterogeneous circRNA-disease network (CDA-RWR). We first train the random walk with restart model on the CDA dataset and screen possible CDAs with the highest association probability from unknown circRNA-disease pairs on the dataset.
First, circRNA similarity network
Second, we compute the transition probability of random walk on the heterogeneous circRNA-disease network. Suppose that
The transition probability from circRNA
The transition probability from diseases
The transition probability from disease
For a query circRNA/disease, it can either stay in the current network with a restart probability
where
We can calculate association probability for each circRNA-disease pair based on random walk with restart. However, for random walk with restart, the jump probability is measured by known CDAs and the circRNA and disease similarity matrices. For a circRNA
First, we compute the circRNA Laplacian matrix
where
Second, we define the loss functions of Laplacian regularization least squares in the circRNA and disease spaces based on the Laplacian matrices
where
Finally, the association probability for each circRNA-disease pair by Laplacian regularized least squares can be computed by Eq. 19:
Ensemble learning integrates multiple results from individual models and demonstrates better performance compared to individual models (Zhou et al., 2021a; Peng et al., 2022b). Therefore, in this study, we develop an ensemble learning model by combining random walk with restart and Laplacian regularized least squares to improve the CDA’s prediction performance by Eq. 20:
where
For similarity computation, the weights between biological feature similarity and association profile similarity
Several comparative experiments are conducted to measure the performance of our proposed CDA-EnRWLRLS model. CD-LNLP (Zhang et al., 2019), DWNN-RLS (Yan et al., 2018), KATZHCDA (Fan et al., 2018b), and CDA-EnRWLRLS are conducted on the preprocessed CDA dataset. CD-LNLP (Zhang et al., 2019) is a linear neighborhood label propagation-based algorithm for CDA prediction. DWNN-RLS (Yan et al., 2018) used regularized least squares to predict possible CDAs. KATZHCDA (Fan et al., 2018b) discovered CDA candidates based on the KATZ measurement (Zhou et al., 2020). Figure 2 shows the AUC values computed by these four CDA prediction methods.
From Figure 2, we can find that CDA-EnRWLRLS is significantly better than CD-LNLP (Zhang et al., 2019), DWNN-RLS (Yan et al., 2018), and KATZHCDA (Fan et al., 2018b) based on the AUC value. Compared to the three models, CDA-EnRWLRLS obtains the highest AUC of 0.8654, outperforming 7.60%, 24.86%, and 0.25%, respectively. In particular, DWNN-RLS used regularized least squares with Kronecker product kernel for CDA prediction. Disease similarity was computed by their semantic similarity and Gaussian association profile similarity. Meanwhile, circRNA similarity was computed by their Gaussian association profiles. CDA-EnRWLRLS uses an ensemble model to identify possible CDAs. Similar to DWNN-RLS, CDA-EnRWLRLS computes disease similarity. However, CDA-EnRWLRLS computes circRNA similarity by their functional similarity and Gaussian association profile similarity. Furthermore, CDA-EnRWLRLS still computes association score between each circRNA-disease pair using random walk with restart except Laplacian regularized least squares and integrates the results from the two models by the soft voting technique. Therefore, CDA-EnRWLRLS outperforms DWNN-RLS, which demonstrates its powerful CDA prediction ability.
Our proposed CDA-EnRWLRLS model is an ensemble of two state-of-the-art models (i.e., random walk with restart and Laplacian regularized least squares). To evaluate the performance of ensemble learning model and individual models, we conducted 5-fold cross validation experiment for CDA-EnRWLRLS and random walk with restart (CDA-RWR) and Laplacian regularized least squares (CDA-LRLS) on the CDA dataset. Figure 3 shows the AUC values computed by CDA-EnRWLRLS, CDA-RWR, and CDA-LRLS. From Figure 3, we can find that CDA-EnRWLRLS obtains better AUC than two individual models, CDA-RWR and CDA-LRLS, which shows that the proposed ensemble learning-based model can outperforms individual models.
In this study, we ensemble two individual models, random walk with restart and Laplacian regularized least squares. However, the two models may have different effects on the CDA prediction performance. To evaluate their effect on the performance, we consider
From Figure 4, we can find that AUC computed by CDA-EnRWLRLS gradually increases when the parameter
We consequently compute the association score for each circRNA-disease pair. In particular, we compute association abilities between all circRNAs and bladder cancer and bladder urothelial cancer to analyze any possible associations between these circRNAs and the two cancers, and to further screen for potential circRNA biomarkers for them.
Bladder cancer is a heterogeneous disease with high morbidity and mortality rates (Kamat et al., 2016). It has been estimated that about 73,510 new cases of bladder cancer were diagnosed in the United States in 2012. During the same period, about 14,880 patients died from bladder cancer (Clark et al., 2013). To analyze circRNA biomarkers for bladder cancer, we compute association between all circRNAs and bladder cancer after training CDA-EnRWLRLS. Table 1 gives the top 20 circRNAs that are predicted to have the highest association scores with bladder cancer.
In the CDA dataset, 15 circRNAs are known to associate with bladder cancer among 585 circRNAs. From Table 1, we can find that the 15 circRNAs are predicted to have the highest association scores with bladder cancer and are ranked as the top 15. Furthermore, we predict that circHIPK3 may associate with bladder cancer with the ranking of 16. Furthermore, circHIPK3 is a promising cancer-related circRNA (Zhang et al., 2020). It can regulate cell growth through sponging multiple miRNAs (Zheng et al., 2016). For instance, circHIPK3 can regulate cell proliferation and migration in hepatocellular cancer by sponging miR-124 (Chen X. et al., 2018), modulate autophagy in STK11 mutant lung cancer (Chen et al., 2020), and promote glioma progression as a prognostic marker (Jin et al., 2018). The overexpression of circHIPK3 can accelerate the proliferation and invasion of prostate cancer cells (Cai et al., 2019). Its inhibition can block angiotensin II-induced cardiac fibrosis (Ni et al., 2019). In this study, we infer that circHIPK3 may be a biomarker of bladder cancer and need experimental validation. Figure 5 shows the association information between the top 20 circRNAs with bladder cancer.
FIGURE 5. Associations between the top 20 circRNAs with bladder cancer. Black lines represent associations that have validated in the CDA dataset. Sky blue lines represent associations that are unknown in the CDA dataset but can be validated by related literatures. Yellow lines represent association that are unknown in the CDA dataset and need validation.
Over 90% bladder cancer is bladder urothelial cancer. Bladder urothelial cancer is a common malignancy with high morbidity and mortality worldwide (Cancer Genome Atlas Research Network, 2014). In the United Sates, bladder urothelial cancer is one of the main histologic subtypes (Clark et al., 2013). However, no molecularly targeted agent has been applied to the treatment, until now. To infer potential circRNA biomarkers for bladder urothelial cancer, we compute association scores between all circRNAs and bladder urothelial cancer using CDA-EnRWLRLS. Table 2 gives the top 20 circRNAs that are predicted to have the highest association scores with bladder urothelial cancer.
In the CDA dataset, only one circRNA, circHIPK3, associates with bladder urothelial cancer among all potential 585 circRNAs. We predict that SMARCA5 may associate with bladder urothelial cancer with the ranking of 2. SMARCA5 is a member of the ISWI family that is involved in chromatin remodeling. It can regulate chromosome remodeling through diverse mechanisms, hinder cell proliferation, and assist apoptosis by sponging miRNAs. Its expression may boost the susceptibility of cells to chemotherapy, boost the sensitivity of cancer detection, promote early diagnosis, and help the treatment of chemotherapy-resistant cancers (Qin and Wan, 2022). Its expression level has a certain association with clinical features of many cancers. For instance, SMARCA5 can promote cell proliferation in bladder cancer and prostate cancer (Tan et al., 2019), suppress colorectal cancer progression (Miao et al., 2020), inhibit tumor metastasis in cervical cancer (Zhang X. et al., 2021) and inhibit cell proliferation, migration, and invasion in non-small cell lung cancer (Wang et al., 2019), and boost cell migration and invasion as well as inhibit cell apoptosis in bladder cancer (Kong et al., 2017; Tan et al., 2019). Many studies have reported that circSMARCA5 plays a key role in the occurrence and development of cancer. Moreover, it also serves as a reliable indicator of tumor screening or cancer prognosis evaluation (Qin and Wan, 2022). Therefore, SMARCA5 is a diagnostic and prognostic biomarker of cancer and has obtained wide attention. In this study, we predict that SMARCA5 may be potential biomarker of bladder urothelial cancer; however, this needs validation. Figure 6 shows the association information between the top 20 circRNAs with bladder urothelial cancer.
FIGURE 6. Associations between the top 20 circRNAs with bladder urothelial cancer. Black lines represent associations that have validated in the CDA dataset. Sky blue lines represent associations that are unknown in the CDA dataset but can be validated by related literatures. Blue lines represent association that are unknown in the CDA dataset and need validation.
Bladder cancer, including bladder urothelial cancer, is a common and complex disease. These cancers have caused high morbidity and mortality. The identification of biomarkers for bladder cancer and bladder urothelial cancer can help in their prognosis and treatment. In this manuscript, we developed an ensemble learning model, CDA-EnRWLRLS, to discover potential circRNA biomarkers for the two cancers based on CDA association prediction.
CDA-EnRWLRLS first computes circRNA similarity and disease similarity by fusing semantic similarity and association profile similarity of diseases and functional similarity and association profile similarity of circRNAs. Second, it scores each circRNA-disease pair by random walk with restart and Laplacian regularized least squares, respectively. Third, the results computed by random walk with restart and Laplacian regularized least squares are integrated by the soft voting approach based on ensemble learning. Finally, it is applied to discover potential circRNA biomarkers for bladder cancer and bladder urothelial cancer.
CDA-EnRWLRLS is compared to three classical CDA prediction methods (CD-LNLP, DWNN-RLS, and KATZHCDA) and two individual models (CDA-RWR and CDA-LRLS). The results show that CDA-EnRWLRLS computes relatively better AUC, which demonstrates its relatively powerful CDA prediction ability. We predict that circHIPK3 and SMARCA5 may be potential biomarkers of bladder cancer and bladder urothelial cancer, respectively.
CDA-EnRWLRLS has two advantages: on the one hand, it better fuses biological features and association features of diseases and circRNAs; while on the other hand, it combines two individual classical association prediction models to obtain the powerful association prediction performance from different bioinformatics tools. Although CDA-EnRWLRLS computed better CDA inference ability, the circRNA functional similarity was calculated indirectly by disease semantic similarity. Moreover, its prediction performance needs further improvement. In the future, we will consider biological features of circRNAs and develop more efficient machine learning, especially ensemble learning models (Zhou et al., 2021a; Peng et al., 2022a) and deep learning models (Peng et al., 2021b; Zhou et al., 2021b; Sun et al., 2022; Yang et al., 2022) to discover potential biomarkers for bladder cancer and bladder urothelial cancer.
The original contributions presented in the study are included in the article/supplementary material, and further inquiries can be directed to the corresponding author.
Conceptualization: QS and LW; Methodology: QS, QT, and LW; Project administration: QS, XL, and LW; Software: QS, QT, XL, and LW; Writing—original draft: QS; Writing—review and editing: QS and LW.
This research was funded by the Natural Science Foundation of Hunan province (Grant 2020JJ5996).
The authors declare that the research was conducted in the absence of any commercial or financial relationships that could be construed as a potential conflict of interest.
All claims expressed in this article are solely those of the authors and do not necessarily represent those of their affiliated organizations, or those of the publisher, the editors and the reviewers. Any product that may be evaluated in this article, or claim that may be made by its manufacturer, is not guaranteed or endorsed by the publisher.
Bin Riaz, I., Khan, A. M., Catto, J. W. F., and Hussain, S. A. (2021). Bladder cancer: Shedding light on the most promising investigational drugs in clinical trials.. Expert Opin. Investig. Drugs 30 (8), 837–855. doi:10.1080/13543784.2021.1948999
Black, A. J., and Black, P. C. (2020). Variant histology in bladder cancer: Diagnostic and clinical implications. Transl. Cancer Res. 9 (10), 6565–6575. doi:10.21037/tcr-20-2169
Cai, C., Zhi, Y., Wang, K., Zhang, P., Ji, Z., Xie, C., et al. (2019). CircHIPK3 overexpression accelerates the proliferation and invasion of prostate cancer cells through regulating miRNA-338-3p.. Onco. Targets. Ther. 12, 3363–3372. doi:10.2147/OTT.S196931
Cancer Genome Atlas Research Network (2014). Comprehensive molecular characterization of urothelial bladder carcinoma[J]. Nature 507 (7492), 315. doi:10.1038/nature12965
Chen, G., Shi, Y., Liu, M., and Sun, J. (2018). circHIPK3 regulates cell proliferation and migration by sponging miR-124 and regulating AQP3 expression in hepatocellular carcinoma.. Cell. Death Dis. 9 (2), 1–13. doi:10.1038/s41419-017-0204-3
Chen, X., Chen, R. X., Wei, W. S., Li, Y. H., Feng, Z. H., Tan, L., et al. (2018). PRMT5 circular RNA promotes metastasis of urothelial carcinoma of the bladder through sponging miR-30c to induce epithelial-mesenchymal transition.. Clin. Cancer Res. 24 (24), 6319–6330. doi:10.1158/1078-0432.CCR-18-1270
Chen, X., Mao, R., Su, W., Yang, X., Geng, Q., Guo, C., et al. (2020). Circular RNA circHIPK3 modulates autophagy via MIR124-3p-STAT3-PRKAA/AMPKα signaling in STK11 mutant lung cancer.. Autophagy 16 (4), 659–671. doi:10.1080/15548627.2019.1634945
Clark, P. E., Agarwal, N., Biagioli, M. C., Eisenberger, M. A., Greenberg, R. E., Herr, H. W., et al. (2013). Bladder cancer.. J. Natl. Compr. Canc. Netw. 11 (4), 446–475. doi:10.6004/jnccn.2013.0059
Deepthi, K., and Jereesh, A. S. (2020). An ensemble approach for CircRNA-disease association prediction based on autoencoder and deep neural network.. Gene 762, 145040. doi:10.1016/j.gene.2020.145040
Fabiano, E., Durdux, C., Dufour, B., Mejean, A., Thiounn, N., Chretien, Y., et al. (2021). Long-term outcomes after bladder-preserving tri-modality therapy for patients with muscle-invasive bladder cancer.. Acta Oncol. 60 (6), 794–802. doi:10.1080/0284186X.2021.1915498
Fan, C., Lei, X., Fang, Z., Jiang, Q., and Wu, F-X. (2018a). CircR2Disease: A manually curated database for experimentally supported circular RNAs associated with various diseases[J]. Database 2018, bay044. doi:10.1093/database/bay044
Fan, C., Lei, X., and Wu, F. X. (2018b). Prediction of CircRNA-disease associations using KATZ model based on heterogeneous networks.. Int. J. Biol. Sci. 14 (14), 1950–1959. doi:10.7150/ijbs.28260
Gao, S., Yang, X., Xu, J., Qiu, N., and Zhai, G. (2021). Nanotechnology for boosting cancer immunotherapy and remodeling tumor microenvironment: The horizons in cancer treatment. ACS Nano 15 (8), 12567–12603. doi:10.1021/acsnano.1c02103
Gong, Y., Mao, J., Wu, D. I., Wang, X., Li, L., Zhu, L., et al. (2018). Circ-ZEB1.33 promotes the proliferation of human HCC by sponging miR-200a-3p and upregulating CDK6.. Cancer Cell. Int. 18 (1), 116–119. doi:10.1186/s12935-018-0602-3
He, J., Xie, Q., Xu, H., Li, J., and Li, Y. (2017). Circular RNAs and cancer.. Cancer Lett. 396, 138–144. doi:10.1016/j.canlet.2017.03.027
Jeck, W. R., and Sharpless, N. E. (2014). Detecting and characterizing circular RNAs.. Nat. Biotechnol. 32 (5), 453–461. doi:10.1038/nbt.2890
Jin, P., Huang, Y., Zhu, P., Zou, Y., Shao, T., and Wang, O. (2018). CircRNA circHIPK3 serves as a prognostic marker to promote glioma progression by regulating miR-654/IGF2BP3 signaling.. Biochem. Biophys. Res. Commun. 503 (3), 1570–1574. doi:10.1016/j.bbrc.2018.07.081
Kamat, A. M., Hahn, N. M., Efstathiou, J. A., Lerner, S. P., Malmstrom, P. U., Choi, W., et al. (2016). Bladder cancer.. Lancet 388 (10061), 2796–2810. doi:10.1016/S0140-6736(16)30512-8
Kirkali, Z., Chan, T., Manoharan, M., Algaba, F., Busch, C., Cheng, L., et al. (2005). Bladder cancer: Epidemiology, staging and grading, and diagnosis.. Urology 66 (6), 4–34. doi:10.1016/j.urology.2005.07.062
Kong, Z., Wan, X., Zhang, Y., Zhang, P., Zhang, Y., Zhang, X., et al. (2017). Androgen-responsive circular RNA circSMARCA5 is up-regulated and promotes cell proliferation in prostate cancer.. Biochem. Biophys. Res. Commun. 493 (3), 1217–1223. doi:10.1016/j.bbrc.2017.07.162
Li, G., Luo, J., Wang, D., Liang, C., Xiao, Q., Ding, P., et al. (2020). Potential circRNA-disease association prediction using DeepWalk and network consistency projection.. J. Biomed. Inf. 112, 103624. doi:10.1016/j.jbi.2020.103624
Li, G., Yue, Y., Liang, C., Xiao, Q., Ding, P., and Luo, J. (2019a). Ncpcda: Network consistency projection for circRNA-disease association prediction.. RSC Adv. 9 (57), 33222–33228. doi:10.1039/c9ra06133a
Li, J., Sun, D., Pu, W., Wang, J., and Peng, Y. (2020). Circular RNAs in cancer: Biogenesis, function, and clinical significance.. Trends Cancer 6 (4), 319–336. doi:10.1016/j.trecan.2020.01.012
Li, P., Yang, X., Yuan, W., Yang, C., Zhang, X., Han, J., et al. (2018). CircRNA-Cdr1as exerts anti-oncogenic functions in bladder cancer by sponging MicroRNA-135a.. Cell.. Physiol. biochem. 46 (4), 1606–1616. doi:10.1159/000489208
Li, T., Huang, T., Guo, C., Wang, A., Shi, X., Mo, X., et al. (2021). Genomic variation, origin tracing, and vaccine development of SARS-CoV-2: A systematic review.. Innovation. 2 (2), 100116. doi:10.1016/j.xinn.2021.100116
Li, Y., Wan, B., Liu, L., Zhou, L., and Zeng, Q. (2019b). Circular RNA circMTO1 suppresses bladder cancer metastasis by sponging miR-221 and inhibiting epithelial-to-mesenchymal transition.. Biochem. Biophys. Res. Commun. 508 (4), 991–996. doi:10.1016/j.bbrc.2018.12.046
Lopez-Beltran, A., Cimadamore, A., Blanca, A., Massari, F., Vau, N., Scarpelli, M., et al. (2021). Immune checkpoint inhibitors for the treatment of bladder cancer. Cancers 13 (1), 131. doi:10.3390/cancers13010131
Lu, C., Zeng, M., Wu, F. X., Li, M., and Wang, J. (2021). Improving circRNA–disease association prediction by sequence and ontology representations with convolutional and recurrent neural networks. Bioinformatics 36 (24), 5656–5664. doi:10.1093/bioinformatics/btaa1077
Lu, Q., Liu, T., Feng, H., Yang, R., Zhao, X., Chen, W., et al. (2019). Circular RNA circSLC8A1 acts as a sponge of miR-130b/miR-494 in suppressing bladder cancer progression via regulating PTEN.. Mol. Cancer 18 (1), 111–113. doi:10.1186/s12943-019-1040-0
Mancini, M., Righetto, M., and Noessner, E. (2021). Checkpoint inhibition in bladder cancer: Clinical expectations, current evidence, and proposal of future strategies based on a tumor-specific immunobiological approach.. Cancers 13 (23), 6016. doi:10.3390/cancers13236016
Miao, X., Xi, Z., Zhang, Y., Li, Z., Huang, L., Xin, T., et al. (2020). Circ-SMARCA5 suppresses colorectal cancer progression via downregulating miR-39-3p and upregulating ARID4B.. Dig. Liver Dis. 52 (12), 1494–1502. doi:10.1016/j.dld.2020.07.019
Ni, H., Li, W., Zhuge, Y., Xu, S., Wang, Y., Chen, Y., et al. (2019). Inhibition of circHIPK3 prevents angiotensin II-induced cardiac fibrosis by sponging miR-29b-3p.. Int. J. Cardiol. 292, 188–196. doi:10.1016/j.ijcard.2019.04.006
Nouhaud, F. X., Chakroun, M., Lenormand, C., ouzaid, I., Peyronnet, B., Gryn, A., et al. (2021). Comparison of the prognosis of primary vs. progressive muscle invasive bladder cancer after radical cystectomy: Results from a large multicenter study. Urologic Oncol. Seminars Orig. Investigations 39 (3), 195.e1–195.e6. doi:10.1016/j.urolonc.2020.09.006
Ozsolak, F., and Milos, P. M. (2011). RNA sequencing: Advances, challenges and opportunities.. Nat. Rev. Genet. 12 (2), 87–98. doi:10.1038/nrg2934
Peng, L., Shen, L., Xu, J., Tian, X., Liu, F., Wang, J., et al. (2021a). Prioritizing antiviral drugs against SARS-CoV-2 by integrating viral complete genome sequences and drug chemical structures[J]. Sci. Rep. 11 (1), 1–11. doi:10.1038/s41598-021-83737-5
Peng, L., Tan, J., Tian, X., and Zhou, L. (2022b). EnANNDeep: An ensemble-based lncRNA–protein interaction prediction framework with adaptive k-nearest neighbor classifier and deep models[J]. Interdiscip. Sci. Comput. Life Sci., 1–24. doi:10.1007/s12539-021-00483-y
Peng, L., Tian, X., Tian, G., Xu, J., Huang, X., Weng, Y., et al. (2020). Single-cell RNA-seq clustering: Datasets, models, and algorithms.. RNA Biol. 17 (6), 765–783. doi:10.1080/15476286.2020.1728961
Peng, L., Wang, F., Wang, Z., Tan, J., Huang, L., Tian, X., et al. (2022a). Cell-cell communication inference and analysis in the tumour microenvironments from single-cell transcriptomics: Data resources and computational strategies.. Brief. Bioinform. 23 (4), bbac234. doi:10.1093/bib/bbac234
Peng, L. H., Chen, Y. Q., Ma, N., and Chen, X. (2017). Narrmda: Negative-aware and rating-based recommendation algorithm for miRNA-disease association prediction.. Mol. Biosyst. 13 (12), 2650–2659. doi:10.1039/c7mb00499k
Peng, L. H., Sun, C. N., Guan, N. N., Qiang, J., and Chen, X. (2018). Hnmda: Heterogeneous network-based miRNA-disease association prediction.. Mol. Genet. Genomics 293 (4), 983–995. doi:10.1007/s00438-018-1438-1
Peng, L., Wang, C., Tian, X., Zhou, L., and Li, K. (2021b). Finding lncRNA-protein interactions based on deep learning with dual-net neural architecture[J]. IEEE/ACM Trans. Comput. Biol. Bioinforma. 2021, 29. doi:10.1109/TCBB.2021.3116232
Powles, T., Csőszi, T., Özgüroğlu, M., Matsubara, N., Geczi, L., Cheng, S. Y. S., et al. (2021). Pembrolizumab alone or combined with chemotherapy versus chemotherapy as first-line therapy for advanced urothelial carcinoma (KEYNOTE-361): A randomised, open-label, phase 3 trial.. Lancet. Oncol. 22 (7), 931–945. doi:10.1016/S1470-2045(21)00152-2
Renner, A., Burotto, M., Valdes, J. M., Roman, J. C., and Walton-Diaz, A. (2021). Neoadjuvant immunotherapy for muscle invasive urothelial bladder carcinoma: Will it change current standards? Ther. Adv. Urol. 13, 17562872211029779. doi:10.1177/17562872211029779
Roviello, G., Catalano, M., Santi, R., Palmieri, V. E., Vannini, G., Galli, I. C., et al. (2021). Immune checkpoint inhibitors in urothelial bladder cancer: State of the art and future perspectives.. Cancers 13 (17), 4411. doi:10.3390/cancers13174411
Shen, L., Liu, F. X., Huang, L., Liu, G. Y., Zhou, L. Q., and Peng, L. H. (2022). VDA-RWLRLS: An anti-SARS-CoV-2 drug prioritizing framework combining an unbalanced bi-random walk and Laplacian regularized least squares. Comput. Biol. Med. 140, 105119. doi:10.1016/j.compbiomed.2021.105119
Sheng, J. Q., Liu, L., Wang, M. R., and Li, P. Y. (2018). Circular RNAs in digestive system cancer: Potential biomarkers and therapeutic targets.. Am. J. Cancer Res. 8 (7), 1142–1156.
Sun, F., Sun, J., and Zhao, Q. (2022). A deep learning method for predicting metabolite-disease associations via graph neural network.. Brief. Bioinform. 23 (4), bbac266. doi:10.1093/bib/bbac266
Tan, Y., Zhang, T., and Liang, C. (2019). Circular RNA SMARCA5 is overexpressed and promotes cell proliferation, migration as well as invasion while inhibits cell apoptosis in bladder cancer.. Transl. Cancer Res. 8 (5), 1663–1671. doi:10.21037/tcr.2019.08.08
Tran, L., Xiao, J. F., Agarwal, N., Duex, J. E., and Theodorescu, D. (2021). Advances in bladder cancer biology and therapy.. Nat. Rev. Cancer 21 (2), 104–121. doi:10.1038/s41568-020-00313-1
Vromman, M., Vandesompele, J., and Volders, P. J. (2021). Closing the circle: Current state and perspectives of circular RNA databases.. Brief. Bioinform. 22 (1), 288–297. doi:10.1093/bib/bbz175
Walia, A. S., Sweis, R. F., Agarwal, P. K., Kader, A. K., and Modi, P. K. (2021). Cost-effectiveness of immune checkpoint inhibitors in urothelial carcinoma-A review.. Cancers 14 (1), 73. doi:10.3390/cancers14010073
Wang, C. C., Han, C. D., Zhao, Q., and Chen, X. (2021a). Circular RNAs and complex diseases: From experimental results to computational models.. Brief. Bioinform. 22 (6), bbab286. doi:10.1093/bib/bbab286
Wang, L., Yan, X., You, Z. H., Zhou, X., Li, H. Y., and Huang, Y. A. (2021c). Sganrda: Semi-supervised generative adversarial networks for predicting circRNA-disease associations.. Brief. Bioinform. 22 (5), bbab028. doi:10.1093/bib/bbab028
Wang, L., You, Z. H., Huang, D. S., and Li, J-Q. (2021b). Mgrcda: Metagraph recommendation method for predicting CircRNA-disease association[J]. IEEE Trans. Cybern. 2021. doi:10.1109/TCYB.2021.3090756
Wang, L., You, Z. H., Li, Y. M., Zheng, K., and Huang, Y. A. (2020). Gcncda: A new method for predicting circRNA-disease associations based on graph convolutional network algorithm.. PLoS Comput. Biol. 16 (5), e1007568. doi:10.1371/journal.pcbi.1007568
Wang, Y., Li, H., Lu, H., and Qin, Y. (2019). Circular RNA SMARCA5 inhibits the proliferation, migration, and invasion of non-small cell lung cancer by miR-19b-3p/HOXA9 axis.. Onco. Targets. Ther. 12, 7055–7065. doi:10.2147/OTT.S216320
Xia, L., Song, M., Sun, M., Wang, F., and Yang, C. (2018).. Circular RNAs, 171–187. doi:10.1007/978-981-13-1426-1_14Circular RNAs as biomarkers for cancer[J]
Xie, F., Li, Y., Wang, M., Huang, C., Tao, D., Zheng, F., et al. (2018). Circular RNA BCRC-3 suppresses bladder cancer proliferation through miR-182-5p/p27 axis.. Mol. Cancer 144, 1–12. doi:10.1186/s12943-018-0892-z
Xu, J., Cai, L., Liao, B., Zhu, W., and Yang, J. (2020). CMF-impute: An accurate imputation tool for single-cell RNA-seq data.. Bioinformatics 36 (10), 3139–3147. doi:10.1093/bioinformatics/btaa109
Yan, C., Wang, J., and Wu, F. X. D. W. N. N-R. L. S. (2018). Regularized least squares method for predicting circRNA-disease associations[J]. BMC Bioinforma. 19 (19), 73–81.
Yang, J., Ju, J., Guo, L., Ji, B., Shi, S., Yang, Z., et al. (2022). Prediction of HER2-positive breast cancer recurrence and metastasis risk from histopathological images and clinical information via multimodal deep learning.. Comput. Struct. Biotechnol. J. 20, 333–342. doi:10.1016/j.csbj.2021.12.028
Yang, J., Peng, S., Zhang, B., Houten, S., Schadt, E., Zhu, J., et al. (2020). Human geroprotector discovery by targeting the converging subnetworks of aging and age-related diseases.. Geroscience 42 (1), 353–372. doi:10.1007/s11357-019-00106-x
Yang, X., Ye, T., Liu, H., Lv, P., Duan, C., Wu, X., et al. (2021). Expression profiles, biological functions and clinical significance of circRNAs in bladder cancer[J]. Mol. cancer 20 (1), 1–25. doi:10.1186/s12943-020-01300-8
Yu, G., Wang, L. G., Yan, G. R., and He, Q. Y. (2015). Dose: An R/bioconductor package for disease ontology semantic and enrichment analysis.. Bioinformatics 31 (4), 608–609. doi:10.1093/bioinformatics/btu684
Yu, Q., Liu, P., Han, G., Xue, X., and Ma, D. (2020). CircRNA circPDSS1 promotes bladder cancer by down-regulating miR-16.. Biosci. Rep. 40 (1), BSR20191961. doi:10.1042/BSR20191961
Zhang, J., Hou, L., Zuo, Z., Ji, P., Zhang, X., Xue, Y., et al. (2021a). Comprehensive profiling of circular RNAs with nanopore sequencing and CIRI-long.. Nat. Biotechnol. 39 (7), 836–845. doi:10.1038/s41587-021-00842-6
Zhang, W., Yu, C., Wang, X., and Liu, F. (2019). Predicting CircRNA-disease associations through linear neighborhood label propagation method. Ieee Access 7, 83474–83483. doi:10.1109/access.2019.2920942
Zhang, X., Zhang, Q., Zhang, K., Wang, F., Qiao, X., and Cui, J. (2021b). Circ SMARCA5 inhibited tumor metastasis by interacting with SND1 and downregulating the YWHAB gene in cervical cancer. Cell. Transpl. 30, 096368972098378. doi:10.1177/0963689720983786
Zhang, Y., Liu, Q., and Liao, Q. (2020). CircHIPK3: A promising cancer-related circular RNA.. Am. J. Transl. Res. 12 (10), 6694–6704.
Zhang, Z., Yang, T., and Xiao, J. (2018). Circular RNAs: Promising biomarkers for human diseases.. EBioMedicine 34, 267–274. doi:10.1016/j.ebiom.2018.07.036
Zhao, Q., Yang, Y., Ren, G., Ge, E., and Fan, C. (2019). Integrating bipartite network projection and KATZ measure to identify novel CircRNA-disease associations.. IEEE Trans. Nanobioscience 18 (4), 578–584. doi:10.1109/TNB.2019.2922214
Zheng, Q., Bao, C., Guo, W., Li, S., Chen, J., Chen, B., et al. (2016). Circular RNA profiling reveals an abundant circHIPK3 that regulates cell growth by sponging multiple miRNAs.. Nat. Commun. 7 (1), 1–13. doi:10.1038/ncomms11215
Zhong, Z., Huang, M., Lv, M., He, Y., Duan, C., Zhang, L., et al. (2017). Circular RNA MYLK as a competing endogenous RNA promotes bladder cancer progression through modulating VEGFA/VEGFR2 signaling pathway.. Cancer Lett. 403, 305–317. doi:10.1016/j.canlet.2017.06.027
Zhou, L., Wang, J., Liu, G., Lu, Q., Dong, R., Tian, G., et al. (2020). Probing antiviral drugs against SARS-CoV-2 through virus-drug association prediction based on the KATZ method.. Genomics 112 (6), 4427–4434. doi:10.1016/j.ygeno.2020.07.044
Zhou, L., Duan, Q., Tian, X., Tang, J., and Peng, L. H. (2021a). LPI-HyADBS: A hybrid framework for lncRNA-protein interaction prediction integrating feature selection and classification[J]. BMC Bioinforma. 22 (1), 1–31.
Keywords: bladder cancer, bladder urothelial cancer, circRNA, biomarker, circRNA-disease association, ensemble learning
Citation: Su Q, Tan Q, Liu X and Wu L (2022) Prioritizing potential circRNA biomarkers for bladder cancer and bladder urothelial cancer based on an ensemble model. Front. Genet. 13:1001608. doi: 10.3389/fgene.2022.1001608
Received: 23 July 2022; Accepted: 15 August 2022;
Published: 15 September 2022.
Edited by:
Liqian Zhou, Hunan University of Technology, ChinaReviewed by:
Min Chen, Hunan Institute of Technology, ChinaCopyright © 2022 Su, Tan, Liu and Wu. This is an open-access article distributed under the terms of the Creative Commons Attribution License (CC BY). The use, distribution or reproduction in other forums is permitted, provided the original author(s) and the copyright owner(s) are credited and that the original publication in this journal is cited, in accordance with accepted academic practice. No use, distribution or reproduction is permitted which does not comply with these terms.
*Correspondence: Ling Wu, d2xfbmFuY3kwOEAxNjMuY29t
Disclaimer: All claims expressed in this article are solely those of the authors and do not necessarily represent those of their affiliated organizations, or those of the publisher, the editors and the reviewers. Any product that may be evaluated in this article or claim that may be made by its manufacturer is not guaranteed or endorsed by the publisher.
Research integrity at Frontiers
Learn more about the work of our research integrity team to safeguard the quality of each article we publish.