MultiCapsNet: A General Framework for Data Integration and Interpretable Classification
- 1Shulan (Hangzhou) Hospital Affiliated to Zhejiang Shuren University Shulan International Medical College, Hangzhou, China
- 2China National Center for Bioinformation, Beijing, China
- 3Key Laboratory of Genomic and Precision Medicine, Beijing Institute of Genomics, Chinese Academy of Sciences, Beijing, China
- 4University of Chinese Academy of Sciences, Beijing, China
- 5School of Systems Science, Beijing Normal University, Beijing, China
A corrigendum on
MultiCapsNet: A General Framework for Data Integration and Interpretable Classification
by Wang, L., Miao, X., Nie, R., Zhang, Z., Zhang, J., and Cai, J. (2021). Front. Genet. 12:767602. doi:10.3389/fgene.2021.767602
In the original article, there was a mistake in the number labeling for Figure 3 and Figure 4 as published. Figure 3 should be labeled as Figure 4, and vice versa. The correct legend appears below.
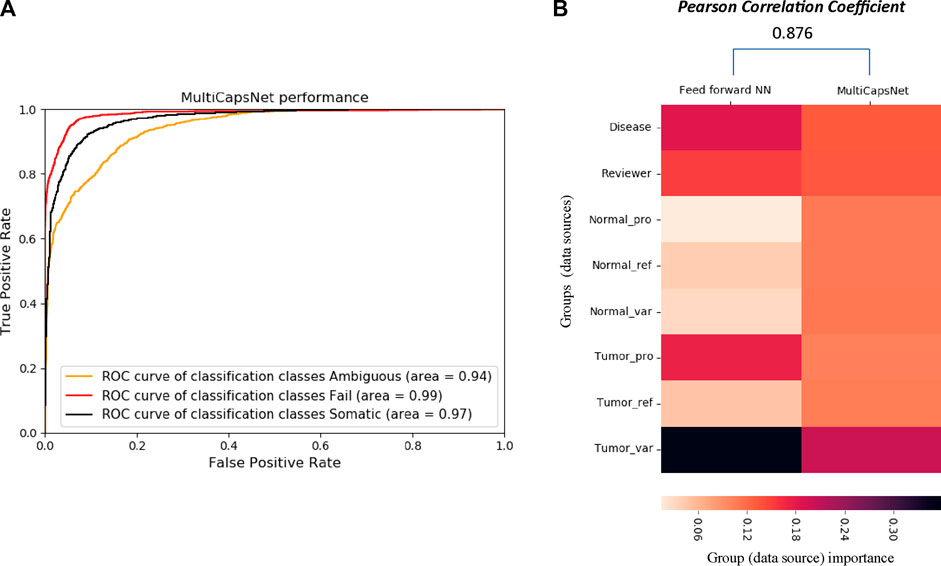
FIGURE 3. Architecture of MultiCapsNet integrated with prior knowledge. (A) The model has two layers. The first layer consists of 696 parallel neural networks corresponding to 696 primary capsules labeled with either transcription factor (348) or protein-protein interaction cluster node (348). The inputs of each primary capsule include genes regulated by a transcription factor or in a protein-protein interactions sub-network. The second layer is the Keras implementation of CapsNet for classification. The length of each final layer type capsule represents the probability of input data belonging to the corresponding classification category. (B) Alternative representation of MultiCapsNet integrated with prior knowledge. Genes that are regulated by a transcription factor or in a protein-protein interactions sub-network, are groups together as a data source for MultiCapsNet. Figures 3A,B are equivalent with different representation.
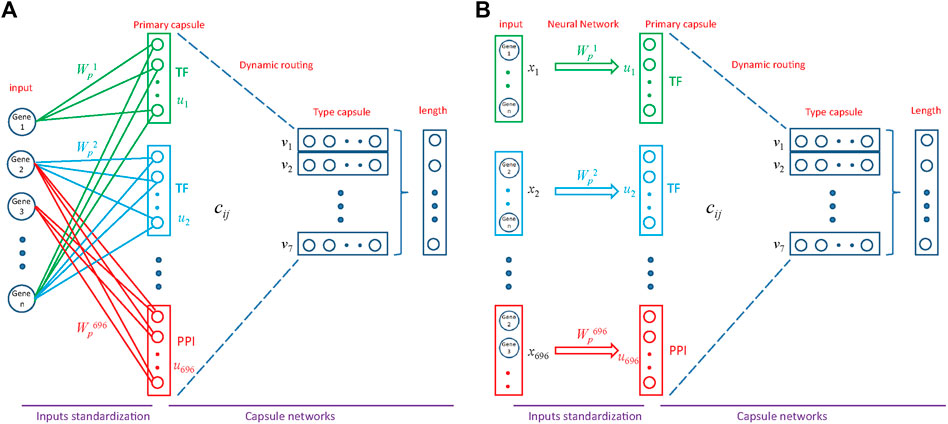
FIGURE 4. The comparison between MultiCapsNet and feed forward neural network shows the high performance and interpretability of MultiCapsNet. (A) The AUC scores demonstrate that the MultiCapsNet model achieves very high classification performances in all three classification categories. (B) The normalized group (data source) importance scores generated by MultiCapsNet and feed forward neural network are highly correlated.
The authors apologize for this error and state that this does not change the scientific conclusions of the article in any way. The original article has been updated.
Publisher’s Note
All claims expressed in this article are solely those of the authors and do not necessarily represent those of their affiliated organizations, or those of the publisher, the editors and the reviewers. Any product that may be evaluated in this article, or claim that may be made by its manufacturer, is not guaranteed or endorsed by the publisher.
Keywords: capsule network, classification, data integration, interpretability, modular feature
Citation: Wang L, Miao X, Nie R, Zhang Z, Zhang J and Cai J (2022) Corrigendum: MultiCapsNet: A General Framework for Data Integration and Interpretable Classification. Front. Genet. 12:822045. doi: 10.3389/fgene.2021.822045
Received: 25 November 2021; Accepted: 26 November 2021;
Published: 27 January 2022.
Approved by:
Frontiers Editorial Office, Frontiers Media SA, SwitzerlandCopyright © 2022 Wang, Miao, Nie, Zhang, Zhang and Cai. This is an open-access article distributed under the terms of the Creative Commons Attribution License (CC BY). The use, distribution or reproduction in other forums is permitted, provided the original author(s) and the copyright owner(s) are credited and that the original publication in this journal is cited, in accordance with accepted academic practice. No use, distribution or reproduction is permitted which does not comply with these terms.
*Correspondence: Jiang Zhang, zhangjiang@bnu.edu.cn; Jun Cai, juncai@big.ac.cn