- 1Sherbrooke Research and Development Centre, Agriculture and Agri-Food Canada, Sherbrooke, QC, Canada
- 2Department of Animal and Veterinary Sciences, University of Vermont, Burlington, VT, United States
- 3Department of Animal Science, McGill University, Ste-Anne-Be-Bellevue, QC, Canada
Johne’s Disease (JD), caused by Mycobacterium avium subsp paratuberculosis (MAP), is an incurable disease of ruminants and other animal species and is characterized by an imbalance of gut immunity. The role of MAP infection on the epigenetic modeling of gut immunity during the progression of JD is still unknown. This study investigated the DNA methylation patterns in ileal (IL) and ileal lymph node (ILLN) tissues from cows diagnosed with persistent subclinical MAP infection over a one to 4 years period. DNA samples from IL and ILLN tissues from cows negative (MAPneg) (n = 3) or positive for MAP infection (MAPinf) (n = 4) were subjected to whole genome bisulfite sequencing. A total of 11,263 and 62,459 differentially methylated cytosines (DMCs), and 1259 and 8086 differentially methylated regions (DMRs) (FDR<0.1) were found between MAPinf and MAPneg IL and ILLN tissues, respectively. The DMRs were found on 394 genes (denoted DMR genes) in the IL and on 1305 genes in the ILLN. DMR genes with hypermethylated promoters/5′UTR [3 (IL) and 88 (ILLN)] or hypomethylated promoters/5′UTR [10 (IL) and 25 (ILLN)] and having multiple functions including response to stimulus/immune response (BLK, BTC, CCL21, AVPR1A, CHRNG, GABRA4, TDGF1), cellular processes (H2AC20, TEX101, GLA, NCKAP5L, RBM27, SLC18A1, H2AC20BARHL2, NLGN3, SUV39H1, GABRA4, PPA1, UBE2D2) and metabolic processes (GSTO2, H2AC20, SUV39H1, PPA1, UBE2D2) are potential DNA methylation candidate genes of MAP infection. The ILLN DMR genes were enriched for more biological process (BP) gene ontology (GO) terms (n = 374), most of which were related to cellular processes (27.6%), biological regulation (16.6%), metabolic processes (15.4%) and response to stimulus/immune response (8.2%) compared to 75 BP GO terms (related to cellular processes, metabolic processes and transport, and system development) enriched for IL DMR genes. ILLN DMR genes were enriched for more pathways (n = 47) including 13 disease pathways compared with 36 enriched pathways, including 7 disease/immune pathways for IL DMR genes. In conclusion, the results show tissue specific responses to MAP infection with more epigenetic changes (DMCs and DMRs) in the ILLN than in the IL tissue, suggesting that the ILLN and immune processes were more responsive to regulation by methylation of DNA relative to IL tissue. Our data is the first to demonstrate a potential role for DNA methylation in the pathogenesis of MAP infection in dairy cattle.
Introduction
Infection with Mycobacterium avium subsp. paratuberculosis (MAP), the causative agent of Johne’s disease (JD) of bovine and other ruminants, is one of the major threats to cattle health and the economic profitability of the dairy industry (Whittington et al., 2019). JD is a long progressive and chronic enteric disease which progresses through three phases upon MAP infection (silent, subclinical, and clinical/advanced stages) and is characterized by imbalance in gut immunity, and MAP’s ability to escape host immune surveillance, and subvert host cell responses to ensure its intracellular survival and replication (Whitlock and Buergelt, 1996; Arsenault et al., 2014; Koets et al., 2015). Similar with other mycobacterial organisms, MAP infects and persists first in macrophages and is found in lymphatic tissues before inducing pathology in the gut or elsewhere, as a secondary process. MAP acquired propensity to infect macrophages helps it to exquisitely adapt and survive in an aggressive host immune response environment. The long-term subclinical period (2–5 years) between the infection period (phase I) and the clinical period (phase III) of JD provides an opportunity for MAP to adapt its environment for its survival. It is known that, pathogenic mycobacteria, including the Mycobacterium avium complex pathogens, respond to host immune responses with counterattack strategies, notably to escape the maturation of phagolysosomes of the phagocytotic cells, which function to destroy them (Liu and Modlin, 2008). The involvement of epigenetic mechanisms in the regulation of MAP survival in the host has been proposed (Ariel et al., 2020), requiring further investigations. Furthermore, reported potential connection between JD and human diseases like Crohn’s disease (CD) and arthritis rheumatoid (Over et al., 2011; Mcnees et al., 2015; Timms et al., 2016; Bo et al., 2020) emphasizes the importance of controlling JD. In addition, the effect of JD on animals, the environment and the economy highlights the need for deeper understanding of the host cellular processes that are perturbed or manipulated by MAP, in order to develop robust disease control strategies.
Several recent reviews have documented the contribution of epigenetic regulation (DNA methylation, histone modifications and miRNA and lncRNA expression) to economically important livestock traits, including disease susceptibility and the immune response (Doherty et al., 2014; Ibeagha-Awemu and Zhao, 2015; Triantaphyllopoulos et al., 2016; Thompson et al., 2020; Wang and Ibeagha-Awemu, 2021). For instance, miRNAs have been found to regulate the autophagic response to eliminate intracellular Mycobacterium (Wang et al., 2013; Wang et al., 2020), modulate inflammatory signaling and to control pathogen survival and replication including Mycobacterium tuberculosis (Sharbati et al., 2011; Mehta and Liu, 2014). Moreover, altered expression of miRNAs during bacterial (mycobacteria, salmonellae and listeriae) invasion of host cells have been shown to affect immune signalling pathways including apoptosis and autophagy related pathways (Sharbati et al., 2011; Hoeke et al., 2013; Pawar et al., 2016; Zur Bruegge et al., 2017). Meanwhile, DNA methylation, especially in CpG (cytosine-phosphate-guanosine) context, has been demonstrated for several cattle disease conditions caused by various pathogens, including Mycobacterium bovis (Doherty et al., 2016), etc. For example, the bovine CD4+ T cells methylome comparison revealed 196 differentially methylated regions (DMRs) that directly contributed to differential gene expression including key inflammatory genes in CD4+ cells isolated from Mycobacterium bovis-infected cows (Doherty et al., 2016). In addition, an in vitro study found that DNA methylation could regulate NLRP3 promoter activity and its gene expression, thereby implicating DNA methylation in NLRP3 inflammasome activation in response to Mycobacterium tuberculosis infection (Wei et al., 2016). Our recent studies reported that MAP impacted the expression of epigenetics genes (Ariel et al., 2019), the lncRNA pattern of macrophage, and the first host immune cell defense encountered while invading cows (Gupta et al., 2019; Marete et al., 2021b). Moreover, comparing the transcriptome landscape of macrophages and small intestinal tissues and draining lymph nodes from JD positive cows with JD negative cows, we observed modifications that show the apparent importance of immune and metabolic pathways hijacked by MAP to subvert the host immune system (Ariel et al., 2020; Ibeagha-Awemu et al., 2021) suggesting a role of epigenetic processes in host response to MAP infection. However, the effect of MAP on the bovine epigenome, specifically the DNA methylome remains unexplored. Considering that intracellular microbial infection can lead to alterations of the host DNA methylome; we hypothesize that epigenetics regulation would be among strategies used by MAP to establish a tolerant environment in infected cows. Therefore, this study used whole genome bisulfite sequencing (WGBS) technique to test the hypothesis that DNA methylation patterns of cow small intestinal tissues are altered during subclinical MAP infection.
Materials and Methods
Experimental Animals
Animal selection and diagnosis of MAP status has been described in details in our recent publication (Ibeagha-Awemu et al., 2021). Briefly, animals were from companion studies (Ariel et al., 2020; Marete et al., 2021b; Marete et al., 2021c) which includes 23 commercial dairy farms (tie and free stall) positive for JD and located in the provinces of Québec and Ontario, Canada. Blood and fecal samples collected twice a year and over a period of one to 4 years were diagnosed for MAP status as described previously (Fock-Chow-Tho et al., 2017). Two different tests, Pourquier serum ELISA assay (IDEXX Laboratories, Markham, Ontario, Canada) and MAP fecal PCR were used to diagnosis for the presence of MAP. Cows positive for both serological and fecal tests were grouped as MAP-infected (MAPinf) (n = 4) while cows negative for both tests constituted the MAP-negative (MAPneg) or control group (n = 5). Mycobacterial fecal culture (Laboratoire d’épidémiosurveillance animale du Québec, Saint-Hyacinthe, Québec, Canada) was used to confirm live MAP excretion, as previously described (Arango-Sabogal et al., 2016). Animals (n = 9) were humanely euthanized by intra-venous administration of 5 mg detomidin and 120 ml euthansol, and ileal (IL) and ileal lymph node (ILLN) tissues without visible Peyer’s patches were collected. Ileal tissue was taken at about 35 cm before the ileocecal valve and ILLN tissue was collected proximal to this point. Following collection of tissues, a third test, f57 real time qPCR was performed to confirm the presence or absence of MAP in the tissues according to our previous study (Ibeagha-Awemu et al., 2021). MAP was identified in tissues from all cows in the MAPinf group and its absence was demonstrated in all tissues from 3 cows out of 5 in MAPneg group (Ibeagha-Awemu et al., 2021). Only the 4 cows in MAPinf group and 3 cows in MAPneg group were further used.
Acid Fast Staining
Tissue pieces about 3–4 mm2, sampled for DNA methylation analysis were immediately embedded in OCT (Optimal cutting temperature compound) and stored in sealed containers at −80°C. Tissue sections (8 μm) were cut for acid fast staining at the Plateforme d’histologie et microscopie électronique, Faculté de médecine, Université de Sherbrooke.
Library Preparation and Whole Genome Bisulfite Sequencing
Genomic DNA was isolated from IL and ILLN tissues using DNeasy Blood and Tissue Kit (Qiagen). DNA was quantified using the Quant-iT™ PicoGreen® dsDNA Assay Kit (Life Technologies) and bisulfite converted using the EZ DNA Methylation-Lightning Kit (Zymo). Libraries were generated using 200 ng DNA/sample and the NEBNext Ultra II DNA Library Prep Kit for Illumina (New England BioLabs) according to manufacturer’s protocol, including CpG methylated pUC19 and unmethylated lambda phage spike-in controls. Size selection of libraries containing the desired insert size was achieved with SPRIselect beads (Beckman Coulter). Libraries were quantified using the Kapa Illumina GA with Revised Primers-SYBR Fast universal kit (Kapa Biosystems) and average size fragment was determined using a LabChip GX (PerkinElmer) instrument.
The libraries were normalized and pooled and then denatured in 0.05 N NaOH and neutralized using HT1 buffer. The pool was loaded at 225pM on an Illumina NovaSeq S4 lane using Xp protocol as per the manufacturer’s recommendations. The run was performed for 2 × 150 cycles (paired-end mode). A phiX library was used as a control and mixed with libraries at 5% level. Base calling was performed with RTA v3. Bcl2fastq2 v2.20 software was used to demultiplex samples and to generate fastq reads. Sequencing was performed to achieve an average depth of 20× using the Illumina NovaSeq S4 system (Illumina, United States). WGBS library preparation and sequencing was performed by the Centre d’expertise et de services Génome Québec (https://cesgq.com/).
Bioinformatics Processing of Whole Genome Bisulfite Sequencing Data
Initial quality control on raw reads to remove low quality reads (Phred score <20) and adaptor sequences was performed with TrimGalore v0.4.4 (Krueger, 2012) and CutAdapt v1.13 (Martin, 2011) programs using the default parameters. Read quality and metrics were visualised and curated using FastQC v0.11.3 (Andrews, 2010). The bovine reference genome, ARS-UCD1.2 was indexed using BSseeker2 -v2.1.7 (Guo et al., 2013). The cleaned data for each sample were then aligned to the indexed reference genome using bowtie2 aligner within BSseeker2 (Guo et al., 2013). Mapped reads were fixmated, sorted, and PCR duplicates removed with SAMtools-v1.7 (Li et al., 2009). The methylation level in each cytosine was then determined using BSseeker2 using the default parameters. Methylation data output included read coverage and percentage of methylated cytosines at each genomic cytosine position. Methylation sites in the context of CpG (cytosine-phosphate-guanosine) and non CpG (i.e. CHG or CHH where H denotes C (cytosine), A [adenosine) or T (thymine)] were extracted for each sample. Methylated cytosines were analysed in genic context including promoter (2000 bp upstream of the transcription start site), 5′UTR, exon, intron, 3′UTR and downstream region (2000 bp downstream of the transcription termination site) of genes, and in CpG island (CGI) context including regions surrounding CGIs such as CGI shores and CGI shelves. CGI shores included the 2 kb region upstream of the CpG island (CGI shore left) and 2 kb region downstream of the CpG island (CGI shore right). CGI shelves included the 2 kb region upstream of the CpG shore left (CpG shelf left) and 2 kb region downstream of the CpG shore right (CGI shelf right). Histograms of methylation sites, coverage and methylation distribution for all samples were performed with the R package methylKit -v1.12.0 (https://bioconductor.org/packages/release/bioc/html/methylKit.html) (Akalin et al., 2012). Moreover, the methylation levels of MAP-infected and negative IL or ILLN samples were merged and density plots generated according to chromosomes using the R package, Circlize (Gu et al., 2014) (https://cran.r-project.org/web/packages/circlize/index.html).
Based on CpG methylation, correlation analysis to determine the relationship between samples and principal component analysis to visualize sample clustering was also performed with methylKit. DNA methylation dynamic plots were generated with Beanplot program in R package (https://cran.r-project.org/web/packages/beanplot/) (Kampstra, 2008).
Differentially Methylated Cytosines and Regions, and Annotation
Methylated sites were compared between MAPinf and MAPneg groups with the Radmeth function of Methpipe -v3.4.3 (Song et al., 2013). The original p values generated from regression with the Radmeth were adjusted for multiple testing and combining significance in the bins of 1:200:1 which gave the false discovery rate (FDR) corrected p value. The annotation files for the genic region and CGIs were downloaded from the UCSC genome browser by selecting ARS-UCD1.2 assembly for Cow genome. Differentially methylated cytosines (DMCs) and differentially methylated regions (DMRs) were annotated within genic regions (promoter, 5′UTR, exon, intron, 3′UTR and downstream region) and in CGI context (CGIs, CGI shores and CGI shelves) with R package annotatr -v1.8.0 (Cavalcante and Sartor, 2017). Significantly differentially methylated cytosines and DMRs were defined as having Benjaminin and Hochberg (Benjamini and Hochberg, 1995) corrected false discovery rate (FDR) < 0.1. DMRs were further filtered for length (<1 kb) and including at least 3 CpG sites.
Gene Ontology and Pathways Analysis
Genes harboring DMRs were subjected to functional enrichment analysis using ClueGO (http://apps.cytoscape.org/apps/cluego) (Bindea et al., 2009), which is an APP in Cytoscape (Shannon et al., 2003). Gene ontology (GO) terms and Kyoto Encyclopedia of Genes and Genomes (KEGG) pathways were adjusted with Benjamini-Hochberg correction FDR (Benjamini and Hochberg, 1995) and considered significantly enriched at FDR <0.05. GO terms were grouped in to biological processes (BP), molecular functions (MF) and cellular component (CC) categories. Enriched KEGG pathways were visualized with ClueGO.
Results
MAP is Present in the Analyzed Tissues
Following the results of f57 qPCR demonstrating the presence of MAP in the MAPinf tissues and absence in the MAPneg tissues (Ibeagha-Awemu et al., 2021), we performed acid fast staining to visualize the distribution of MAP in the analyzed tissues. The result of acid fast staining showed abundant presence of acid fast bacteria (Figures 1A,B) or sparse-acid fast staining indicative of fewer acid fast bacteria (Figures 1D,E) in IL and ILLN tissues from two MAPinf cows. Meanwhile, no evidence of acid fast bacteria indicative of the absence of MAP was seen in IL and ILLN tissues from a MAPneg cow (Figures 1C,F). This result is consistent with the copy numbers of MAP detected by the method of f57 qPCR (Ibeagha-Awemu et al., 2021).
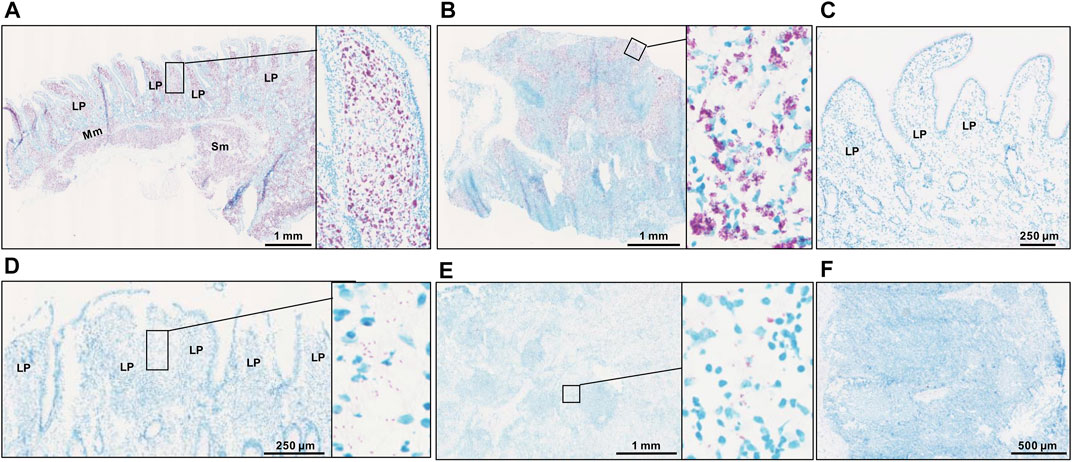
FIGURE 1. Acid-fast staining for acid-fast bacteria in ileum (A) and ileum lymph node (B) from a MAP-infected cow with high copies of MAP, showing abundant acid-fast staining (purple color). Panels (D) (ileum) and (E) (ileum lymph node) are tissues from a MAP-infected cow with fewer copies of MAP, showing very sparse acid-fast staining. No evidence of acid fast bacteria was seen in ileum (C) and ileum lymph node (F) tissues of a MAP-negative cow. LP, lamina propria; Mm, muscularis mucosa; SM, submucosa.
Mapping Statistics
Genome-wide DNA methylation sequencing was conducted on IL and ILLN tissues from four cows positive for MAP infection and three cows negative for MAP infection at a depth of 25× and read length of 150 bp. A total of 2.02 B (billion) and 1.87 B clean reads (adaptor sequences, and reads with Phred score <20 removed) were obtained for IL and ILLN samples, respectively (Supplementary Table S1). Out of these numbers, 70.39% (IL) and 71.67% (ILLN) were uniquely aligned to the bovine reference genome. Mapping rate of bases (A, T, or C) ranged from 68.93 to 71.09% for IL samples and from 69.27 to 76.79% for ILLN samples of the seven cows. The average rate of methylated cytosines in CpG (or mCG), mCHG and mCHH contexts were 67.72, 0.89, 0.90, 71.34, 0.86 and 0.87% in IL and ILLN samples, respectively (Supplementary Table S1). Examination of the percentage of methylation in the various contexts indicated that, the number of cytosines and percent methylation increased in CpG context whereas it decreased in non-CpG context (Figure 2). Since methylation rates in the mCHG and mCHH contexts were very low, subsequent analyses were based on CpG context only.
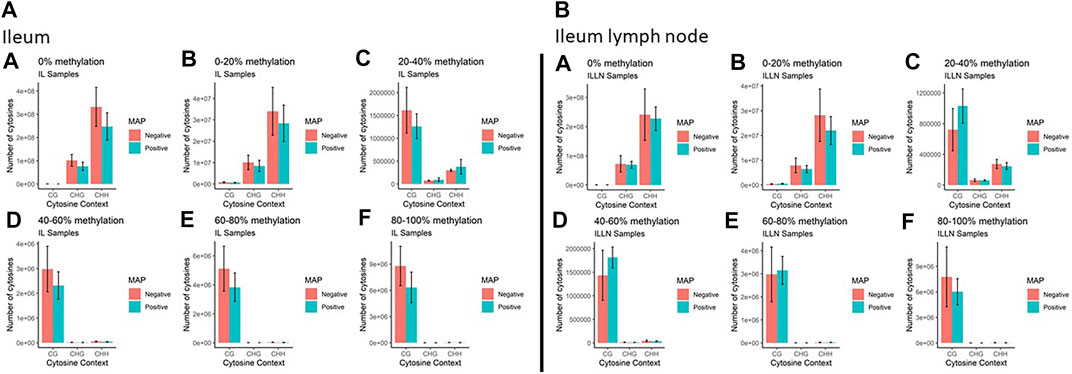
FIGURE 2. The total number of cytosines present in CpG and non-CpG (CHG and CHH, where H = A, T or C) context along with the methylation levels in MAP-infected and MAP-negative (A) ileum (IL) and (B) IL lymph node (ILLN) samples. Moving from A to F in both IL and ILLN samples, the number of cytosines and percent methylation increases in CpG context whereas it decreases in non-CpG.
Global Mapping of DNA Methylation Status
A total of 44.5 million (M) (MAPinf) and 38.6 M (MAPneg), and 38.8 M (MAPinf) and 28.4 M (MAPneg) CpG sites with read coverage per base ≥10× were found in IL and ILLN samples respectively. Out of these, 15.7 M (including 1,342,23 sites in genic regions) and 12.3 M (including 1,246,608 sites in genic regions) methylated sites in IL and ILLN tissues of the seven cows, respectively were used to determine significantly differential methylation between MAPinf and MAPneg cows in each tissue. Pearson correlation was performed to understand the relationship between samples. As shown in Supplementary Figure S1, samples in both tissues were highly correlated with a Pearson correlation coefficient range from 0.70 to 0.79. Global chromosomal dynamics of methylated cytosine distribution in each group and tissue are shown in Supplementary Figure S2. To gain further insight into the methylation profiles, the extent of methylation levels at different genic regions was also analysed. Bean plots show that most of the CpG sites in the 5′UTR and promoter had low levels of methylation (mean of ∼35 and ∼48% in IL or ∼47 and ∼57% in ILLN, respectively), whereas, most of the CpG sites in other genic regions had higher levels of methylation (mean of >72% in both tissues) (Supplementary Figures S3A,B). Moreover, the mean level of methylation was slightly higher in the promoters and intron regions of IL MAPinf samples as compared to IL MAPneg samples. The converse was true for ILLN samples whereby the methylation rates were slightly lower in ILLN MAPinf samples as compared to ILLN MAPneg samples (Supplementary Figures S3A,B).
Differentially Methylated Cytosines Between MAP-Infected and MAP-Negative Samples, and Distribution Patterns
A total of 11,263 and 62,459 significant DMCs (FDR<0.1) were found between MAPinf and MAPneg samples, including 1857 and 7703 in genic regions in IL and ILLN tissues, respectively (Supplementary Tables S2A–D and Supplementary Figure S4). Out of this number, 297 or 508, respectively were located in the promoter and 5′UTRs while highest numbers were located in intron regions. Chromosomal distribution of DMCs between MAPinf and MAPneg cows showed higher concentrations (n > 500) on Bos taurus chromosomes (BTA) 1, 2, 3, 4, 5, 6, 7, 8, 9 and X and lowest numbers (n < 100) on BTA 22, 28 and 29 in the IL (Supplementary Table S2E). Similarly, more significant DMCs (>3900) were located on BTA 1, 2, 3, 4, 5, 6, 7, 8, 9 and X and least numbers on BTA 25 (n = 397), 28 (n = 429) and 29 (n = 462) in ILLN samples (Supplementary Table S2E). A total of 835 DMCs, including 124 DMCs in genic regions, were common to both IL and ILLN tissues (Supplementary Tables S2F,G). The degree of methylation was further classified into hypermethylation (methylation difference (increase) between MAPinf and MAPneg samples is 25% or more), hypomethylation (methylation difference (decrease) between MAPinf and MAPneg samples is 25% or more) or none (neither hypermethylated or hypomethylated and having methylation difference < |25%|). In both tissues, a higher number of genic region DMCs were hypomethylated as compared to hypermethylation status (Figures 3C,D).
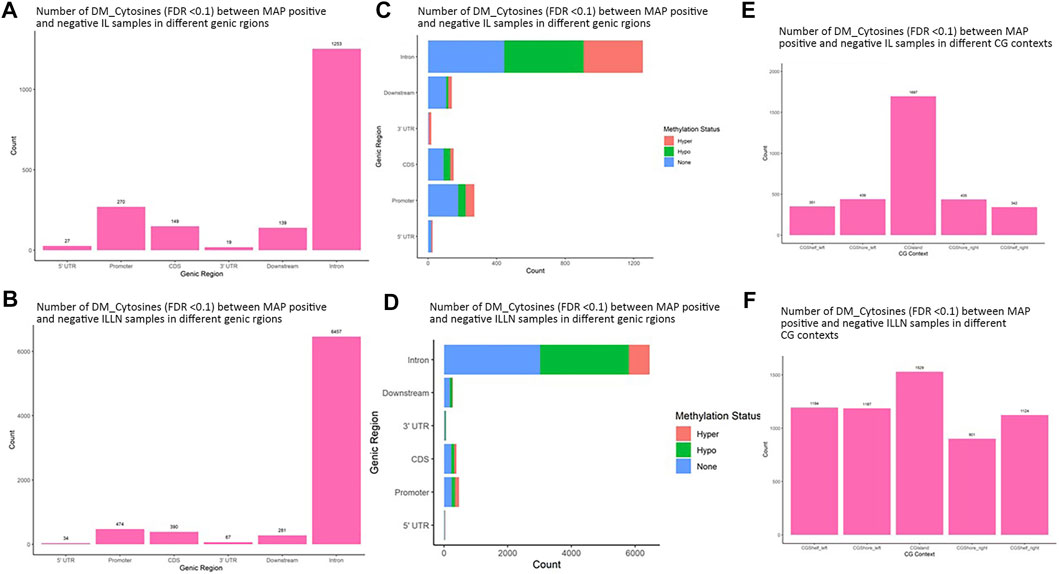
FIGURE 3. Distribution of significantly differentially methylated cytosines (DMC) (FDR<0.1) in different genic regions between MAP-infected (MAPinf) and MAP-negative (MAPneg) (A) ileum (IL) and (B) IL lymph node (ILLN) tissues, and corresponding methylation status in (D) IL and (C) ILLN. A DMC was considered hypermethylated if it had a positive methylation difference ≥25% between MAPinf and MAPneg samples. Similarly, a DMC was hypomethylated if it had a negative methylation difference ≥25% between MAPinf and MAPneg samples. A DMC methylation status of none implies that the methylation difference was less than 25% in both scenarios. Distribution of differentially methylated cytosines in CG context in (E) IL and (F) ILLN tissues between MAPinf and MAPneg samples.
Distribution in CGI context indicated a higher number of significant DMCs in CGIs, CGI shores and shelves in IL (1697) as compared to 1529 in ILLN (Figures 3E,F, and Supplementary Tables S3A,B). Relative to CGIs, more DMCs were found in CGI shores left and right than in CGI shelves left and right in IL, meanwhile, CGI shore left and CGI shelf left contained slightly higher numbers of DMCs than CGI shore right and CGI shelf right, respectively in ILLN (Figures 3E,F and Supplementary Tables S3A,B).
The DMCs were annotated to 503 genes in the IL, including to the promoters and or 5′UTRs of 54 genes (Supplementary Table S2B). In the IL, the most significant DMCs were found in the promoter regions of TMEM15 (17 DMC sites, FDR from 5.89E-07 to 1.60E-04), CDKN2C (13 DMC sites, FDR from 2.46E-04 to 7.57E-04), CDS of HSPA1A (13 DMC sites, FDR = 1.49E-05 to 9.87E-04) and introns of CD38 (2 DMC sites, FDR = 2.78E-05 to 0.0019), CESP-1 (16 DMC site, FDR = 1.27E-04 to 0.004), BCL2 (22 DMC sites, FDR from 1.45E-04 to 0.003), NTRK3 (5 DMC sites, FDR from 4.65E-04 to 7.57E-04). A total of 89 genes harbored 6 to 89 DMCs, including bta-mir-2887–1 (89 DMC sites in downstream region), HSPA1A (48 sites in CDS), PGAM1 (25 DMC sites in promoter), BCL2 (22 DMC sites in intron), TMEM15 (22 DMC sites in promoter), CESP-1 (16 DMC sites in intron), VPS37B (16 DMC sites in intron), BCL6 (15 DMCs in promoter) and CD34 (15 DMCs in intron) (Table 1). Moreover, two genes harbored 14 (TKTL1) and 12 (TOP3A) DMC sites in their promoters, 5′UTRs and CDS.
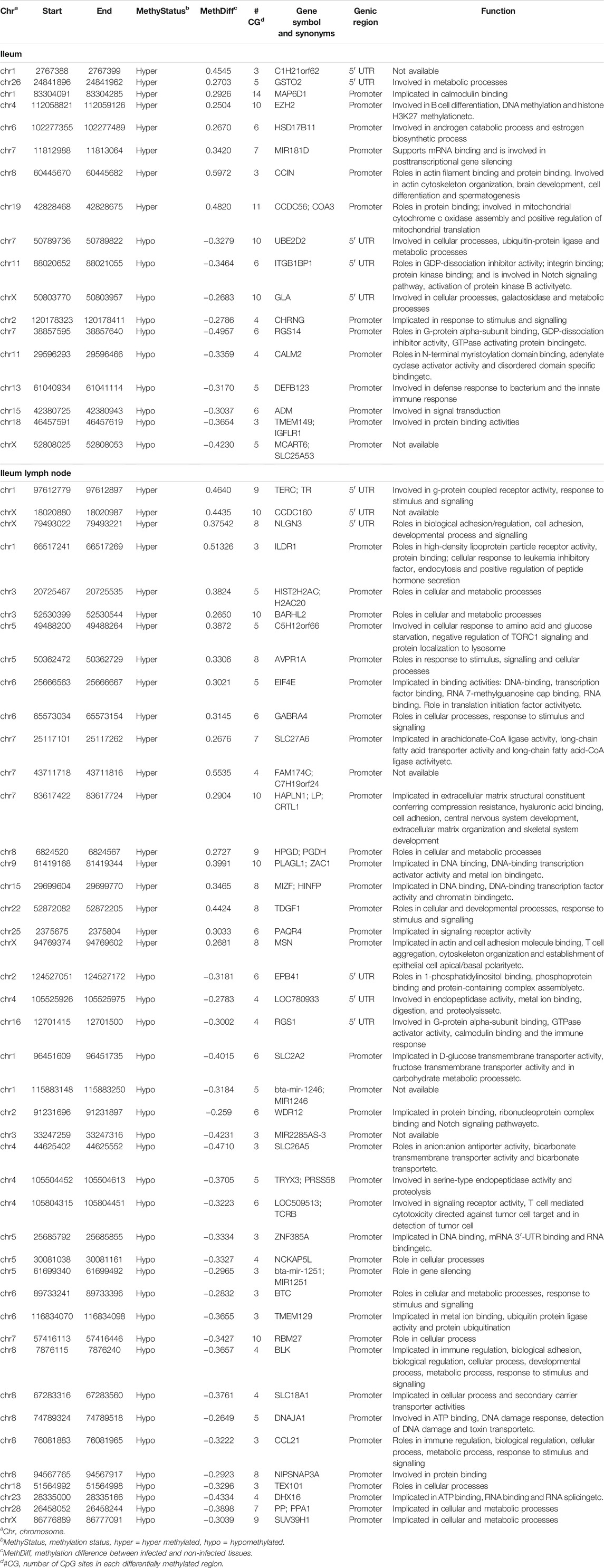
TABLE 1. Genes with hypermethylated or hypomethylated DMRs in their promoters or 5′UTRs in the ileum and ileum lymph node tissues of MAP-infected cows.
In the ILLN, the significant DMCs were annotated to 1717 genes including in the promoters or 5′UTRs of 119 genes (Supplementary Table S2D). The most significant DMCs were found in the introns of SOBP (5 DMC sites, FDR from 1.33E-05 to 3.67E-05), PDE5A (8 DMC sites, FDR from 1.92E-05 to 0.0083), PRDM5 (2 DMC sites, FDR = 1.54E-04), ZNF553, (14 DMC sites in downstream region, FDR from 2.41E-04 to 0.0091), UBAC2 (4 DMC sites, FDR from 8.43E-04 to 0.0018), RORB (16 DMC sites, FDR from 0.0022 to 0.0049) and promoter of RBM27 (9 DMC sites, FDR from 5.03E-04 to 0.0064). A total of 422 genes harbored 6 to 64 DMCs including NLGN1 (64 DMC sites in intron), bta-mir-2887–1 (57 sites in downstream region), PDE5A (52 sites in intron), PTPRK (38 sites in intron), TENM1 (37 sites in intron) and EFNAS (36 sites in 3′UTR and intron), etc. Moreover, about 36 genes harbored 6 to 21 DMC sites in their promoters including miR-387–1 (21 sites), SUV39H1 (18 sites), MSN (17 sites), OTUD5 (17 sites), NR2E1 (16 sites), TMEM15 (13 sites), etc.
Differentially Methylated Regions Between MAP-Infected and MAP-Negative Samples, and Distribution Patterns
Significant DMRs were 1259 and 8086 (FDR<0.1) between MAPinf and MAPneg IL and ILLN samples, respectively (Supplementary Tables S4A–D). Chromosomal distribution indicated a higher number of DMRs (≥60 DMRs) on BTA 1, 2, 3, 4, 5, 6, 7, 8 and 9 in IL and on (≥400 DMRs) BTA 1, 2, 3, 4, 5, 6, 7, 8, 9 and X in ILLN while the least numbers were found on BTA 22 (n = 8), 28 (n = 12) and 29 (n = 11) in the IL and on BTA 25 (n = 47), 28 (n = 57) and 29 (n = 69) in the ILLN (Supplementary Table S2E). Out of the total number of DMRs, 426 (IL) and 2057 (ILLN) were found in genic regions (Supplementary Tables S4B,D). Distribution of DMRs in different genic regions indicated highest proportions in intronic regions and the least numbers in 5′UTR and 3′UTR in both issues (Figures 4A,B). Similarly, more hypermethylated and hypomethylated DMRs were found in the introns and least numbers in 5′UTR and 3′UTR in both issues (Figures 4C,D).
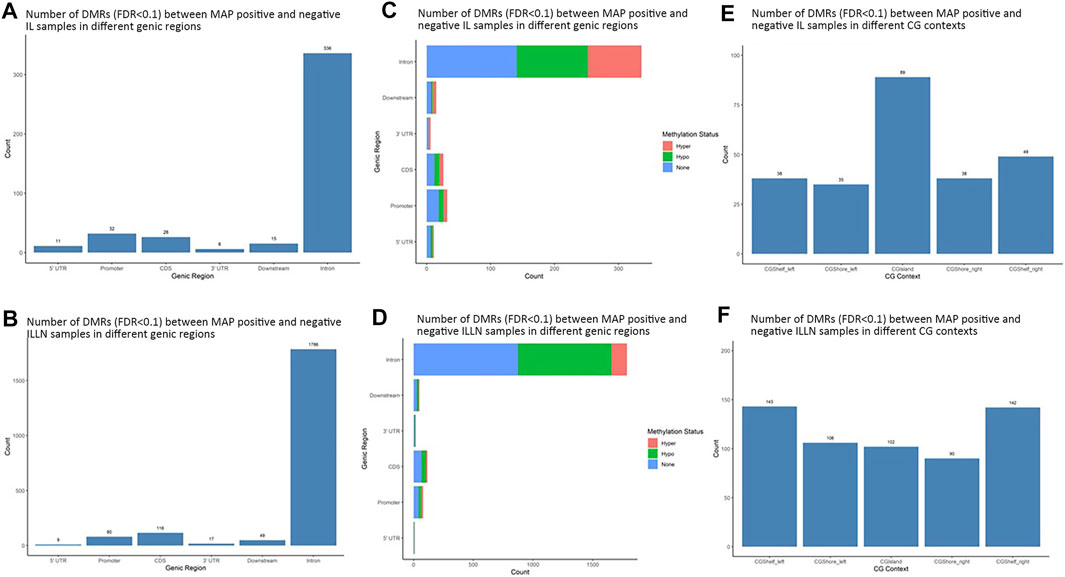
FIGURE 4. Distribution of significantly differentially methylated regions (DMR) in different genic regions between MAP-infected (MAPinf) and MAP-negative (MAPneg) cows in (A) ileum (IL) and (B) IL lymph node (ILLN), and corresponding methylation status in (D) IL and (C) ILLN. A DMR was considered hypermethylated if it had a positve methylation difference ≥25% between MAPinf and MAPneg samples. Similarly, a DMR was hypomethylated if it had a decreased methylation difference ≥25% between MAPinf and MAPneg samples. A DMR methylation status of none implies that the methylation difference was less than 25% in both scenarios. Distribution of differentially methylated cytosines in CpG island context in (E) IL and (F) ILLN samples between MAPinf and MAPneg samples.
Distribution of DMRs in CGI context indicated a total number of 249 and 583 DMRs in the IL and ILLN, respectively (Supplementary Tables S5A–B, Figures 4E,F). Out of these, 89 and 102 DMRs were located within CGIs. Relative to these CGIs, 73 and 87 were located in CG shelf left and right, respectively in IL, while more DMRs (249 and 232) were found in CGI shelf left and right, respectively, in ILLN (Figures 4E,F).
The DMRs were annotated to 394 genes (here referred to as DMR genes) in the IL and to 1305 genes in the ILLN (Supplementary Tables S4B,D). Out of these, promoter and 5′UTR region DMRs were annotated to 43 genes in the IL including eight genes (C1H21orf62, GST O 2, MAP6D1, EZH2, HSD17B11, bta-mir-181d, CCIN and, CCDC56) with hypermethylated promoters and or 5′UTRs and 10 genes (UBE2D2, ITGB1BP1, GLA, CHRNG, RGS14, CALM2, DEFB123, ADM, TMEM149, MCART6, SLC25A53) with hypomethylated promoters or 5′UTRs (Supplementary Table S4B and Table 1). In the ILLN, DMRs were annotated to the promoters of 88 genes including, 19 genes with hypermethylated promoters and or 5′UTRs and 25 genes with hypomethylated promoters or 5′UTRs (Supplementary Table S4D and Table 1). Notable examples of genes with hypermethylated promoters or 5′UTRs included TERC, EIF4E, PLAGL1, CCDC160, ILDR1, HIST2H2AC, C5H12orf66, FAM174C, TDGF1 etc., and with hypomethylated promoters or 5′UTRs included SLC18A1, bta-mir-2285as-3, SLC2A2, SLC26A5, TRYX3, TMEM129, BLK, SLC18A1, CCL21, DHX16, PPA1, etc. (Table 1). Browser screen shots of hypomethylated (RGS14) and hypermethylated (TERC) regions in two genes in the ILLN of MAPinf tissues are shown in Figure 5.
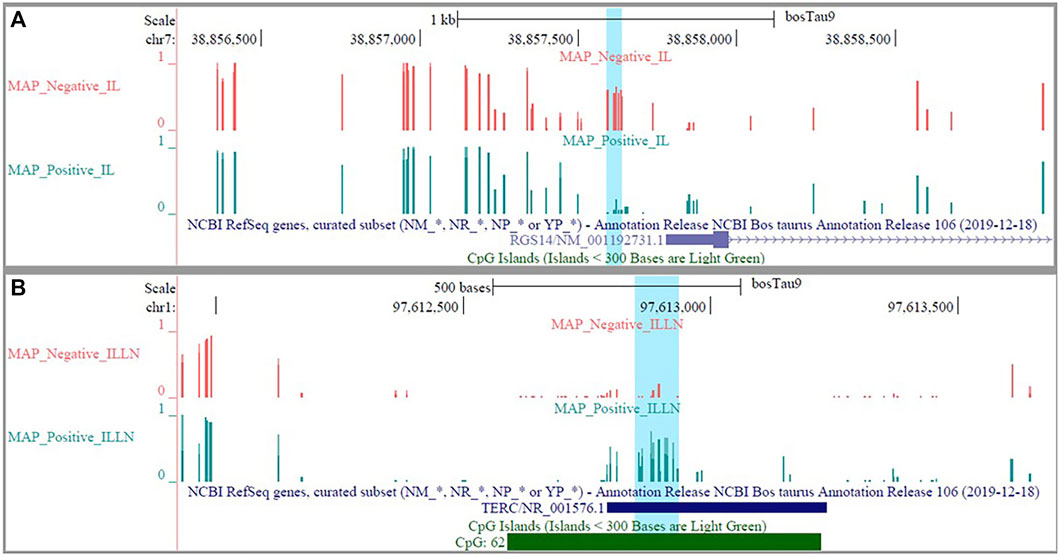
FIGURE 5. Browser shots showing (A) a hypomethylated DMR in a CpG island in RGS14 gene in IL MAP-infected compared with negative samples and (B) a hypermethylated DMR in a CpG island in TERC gene in ILLN MAP-infected compared with negative samples.
In order to understand the potential effects of methylation on the genes harboring DMRs, we examined their expression patterns in RNA-Seq data of the same samples (Ibeagha-Awemu et al., 2021). We found that, out of 394 and 1305 DMR genes in the IL and ILLN, respectively, 22 and 26, respectively, were also differentially expressed (FDR<0.1) at the mRNA level (Table 2).
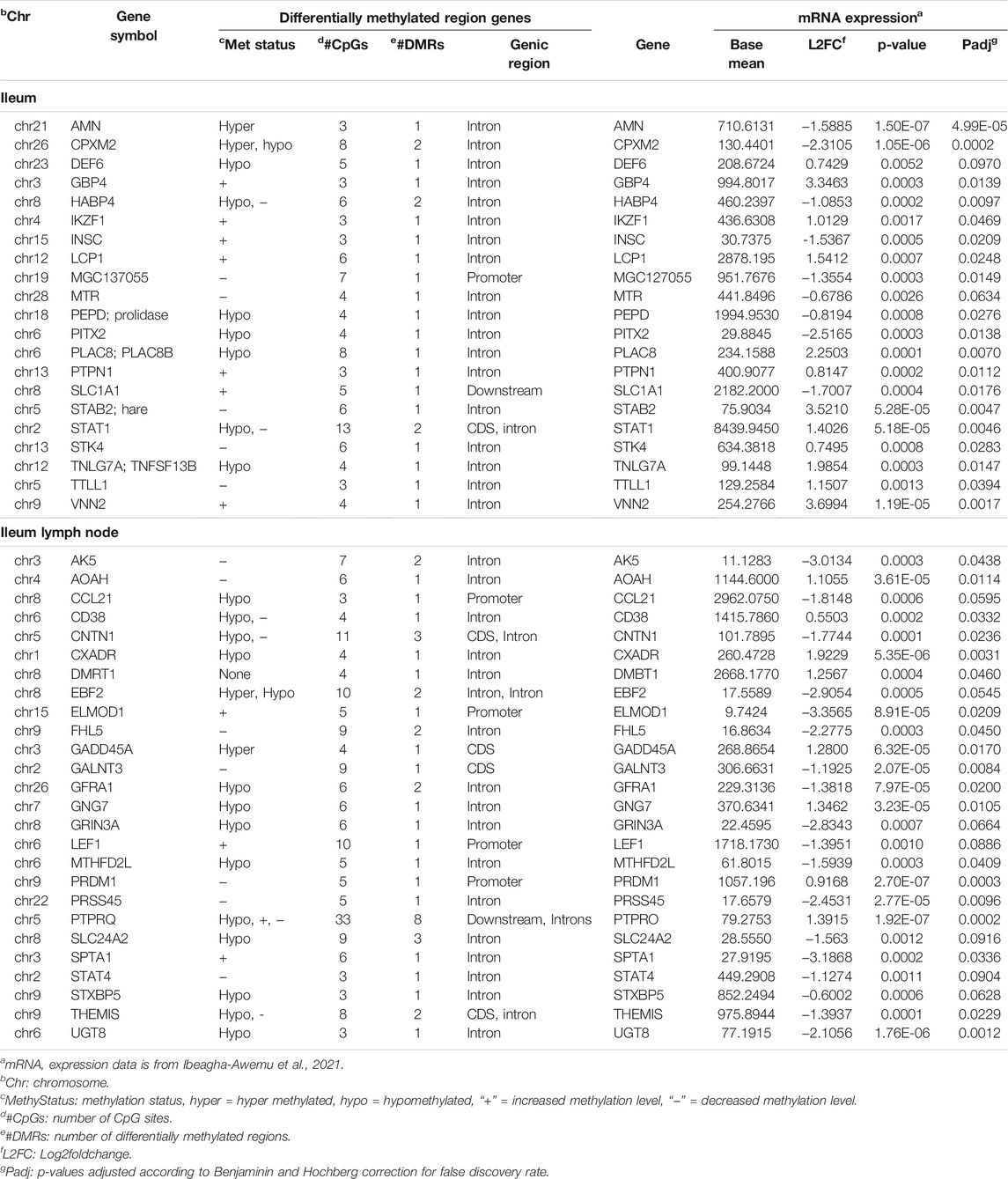
TABLE 2. Select genes harboring differentially methylated regions and their gene (mRNA) expression patterns in the ileum and ileum lymph node of MAP-infected (MAPinf) compared to uninfected (MAPneg) cows.
Functional Roles of Genes Harboring DMRs
To further determine the potential effects of cytosine methylation on the functions of genes, DMR genes were subjected to GO and pathways analysis. GO analysis of 394 DMR genes in the IL resulted in 75 BP, 11 MF and 19 CC GO terms (FDR<0.05) (Supplementary Tables S6A–C). Most of the enriched IL BP GO terms are related to cellular processes, metabolic processes, transport and system development while very few enriched terms (less than 1%) are related to disease and the immune process (Supplementary Table S6A). The most significant IL MF and CC GO terms were neurotransmitter receptor activity (FDR = 0.011) and synapse part (FDR = 0.006), respectively (Supplementary Tables S6B,C). A total of 36 KEGG pathways were enriched for IL DMR genes (Supplementary Table S6D) (Figure 6). The most enriched pathways included Gap junction (FDR = 0.009), Long-term depression (FDR = 0.009), Long-term potentiation (FDR = 0.011) and Sphingolipid signaling pathway (FDR = 0.011). Several disease and immune elated pathways were enriched for IL DMR genes, including C-type lectin receptor signaling pathway (FDR = 0.018), Inflammatory mediator regulation of TRP channels (FDR = 0.024), Cushing syndrome (FDR = 0.023); Hepatitis B (FDR = 0.037), Prostate cancer (FDR = 0.038), Non-small cell lung cancer (FDR = 0.037), Pancreatic cancer (FDR = 0.044), etc. Interestingly, some genes (n = 21) were enriched in many pathways, from 4 to 23 pathways (Supplementary Table S6E).
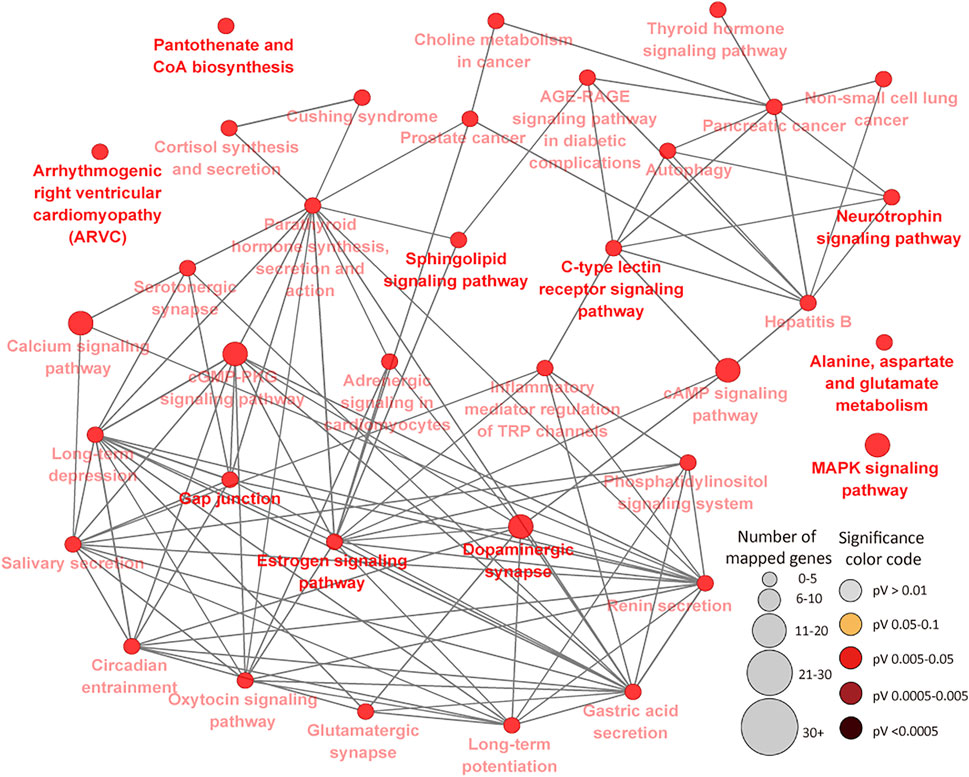
FIGURE 6. KEGG Pathways enriched for ileal tissue differentially methylated region genes showing connections between pathways. Each node represents a pathway and the size (the larger the node, the higher the number of enriched genes) and color (the deeper the color, the more significant the enriched pathway is) of the node represents the number of enriched genes and level of significance, respectively. Straight lines connect pathways.
The 1305 ILLN DMR genes were enriched for 374 BP, 91 MF and 89 CC GO terms (Supplementary Tables S7A–C). Most of the enriched BP terms are related to cellular processes (27.6%), biological regulation (16.6%), metabolic processes (15.4%) and response to stimulus (8.2%) (Supplementary Table S7A). More disease/immune related BP GO terms were enriched (FDR <0.05) for ILLN DMR genes as compared to IL DMR genes. Cytoskeletal protein binding (FDR = 6.94E-07) and plasma membrane part (FDR = 4.36E-12) were the most significantly enriched MF and CC GO terms, respectively (Supplementary Tables S7B,C). Forty-seven KEGG pathways were enriched for ILLN DMR genes including immune (e.g., Bacterial invasion of epithelial cells, Transcriptional misregulation in cancer, Cell adhesion molecules (CAMs), Inflammatory mediator regulation of TRP channels and Platelet activation, etc.) and disease (e.g., Cushing syndrome, pathways in cancer, Small cell lung cancer, Gastric cancer and Arrhythmogenic right ventricular cardiomyopathy (ARVC), etc.) related pathways (Figure 7; Supplementary Table S7D). Several metabolic pathways as well as cGMP-PKG signaling pathway were also enriched for ILLN DMR genes. A total of 63 genes were enriched in four to 28 pathways (Supplementary Table S7E).
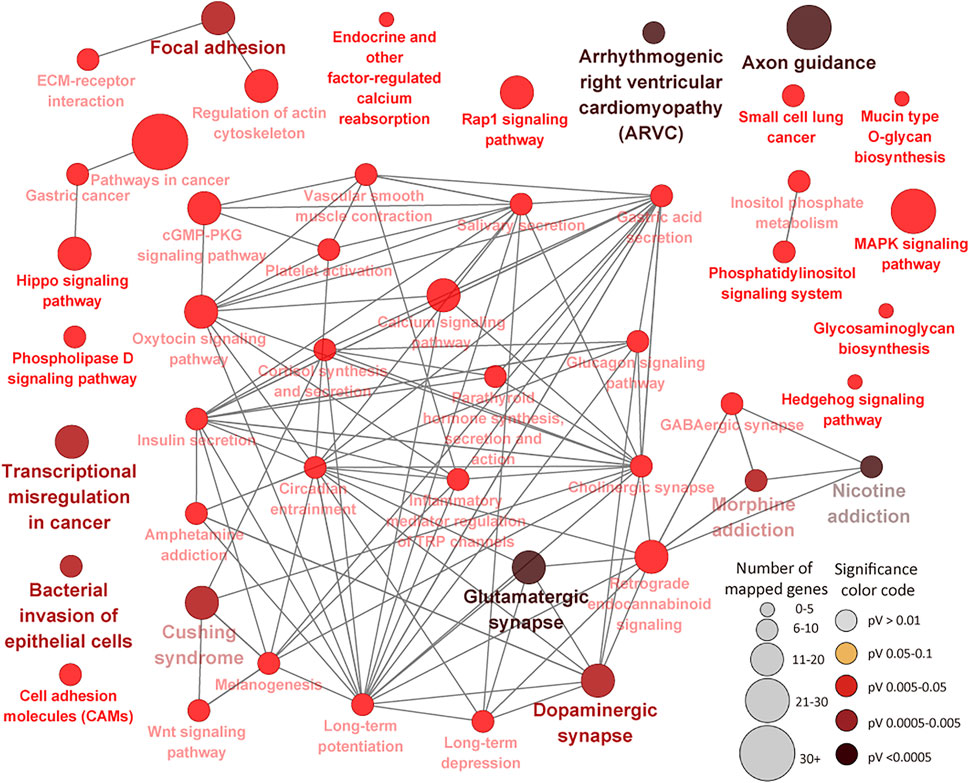
FIGURE 7. KEGG Pathways enriched for ileal lymph node differentially methylated region genes showing connections between pathways. Each node represents a pathway and the size of the node represents the number of enriched genes (the larger the node, the higher the number of enriched genes) while the color intensity represents the level of significance (the deeper the color, the more significant the enriched pathway is). Straight lines connect pathways.
A comparison of enriched pathways in both tissues indicated that 17 (FDR<0.05) pathways were commonly enriched for DMR genes in both tissues (SupplementaryTable S6F), including cGMP-PKG signaling pathway, MAPK signaling pathway, Oxytocin signaling pathway, Cushing syndrome, Arrhythmogenic right ventricular cardiomyopathy (ARVC) and Gastric acid secretion, etc. Furthermore, we compared the BP GO terms and pathways enriched for DMR genes with BP GO terms and pathways enriched for DE genes (Ibeagha-Awemu et al., 2021) in both tissues. Results indicated that 16 BP GO terms were commonly enriched for DMR genes and DE mRNAs in the IL, including regulation of cell migration, positive regulation of cell migration, negative regulation of growth, import into cell, positive regulation of B cell activation, regulation of mononuclear cell migration and lipid biosynthetic process, etc. (Supplementary Table S6G). Meanwhile, only C-type lectin receptor signaling pathway was commonly enriched for DMR genes and DE mRNAs in this tissue (IL) (Supplementary Table S6H). In the ILLN, only one BP GO term (regulation of B cell activation) was commonly enriched for DMR genes and DE genes (Supplementary Table S7F), while no KEGG pathway was commonly enriched.
Discussion
Several studies have shown that MAP alters the expression of genes, biological processes and pathways following MAP infection or during JD progression (Shin M. K. et al., 2015; Hempel et al., 2016; Alonso-Hearn et al., 2019; Ariel et al., 2020; Facciuolo et al., 2020; Ibeagha-Awemu et al., 2021). In addition, a few studies have reported the involvement of miRNAs in JD (Farrell et al., 2015; Gupta et al., 2018; Wang et al., 2019a; Wang et al., 2019b). Our recent studies suggested a possible role of epigenetic processes in the mRNA transcriptome response of bovine monocyte derived macrophages infected by MAP (Ariel et al., 2020; Marete et al., 2021a). Moreover, we observed that MAP initiated a heightened immune response in the IL as compared to a likely suppressed immune response in the ILLN tissues of cows with subclinical MAP infection, which could be underpinned by epigenetic processes (Ibeagha-Awemu et al., 2021). Thus, this study investigated the occurrence of epigenetic regulation through whole genome DNA methylation status analysis of ileal and ileal lymph node tissues in response to persistent MAP-infection in dairy cows.
Overall, DNA methylation data showed differential tissue responses to subclinical MAP infection between ileal and ileal lymph node tissues. More significant DMCs, DMRs and CGIs were recorded between MAPinf and MAPneg cows in the ILLN as compared to the IL. This suggests that, the DNA methylation state of cells in the ILLN were altered to a greater extent by the presence of MAP pathogen than in the IL. This observation corroborates data on differentially expressed mRNA transcriptome of the same samples which suggested a potentially suppressed immune state in the ILLN as compared to a heightened immune state of the IL (Ibeagha-Awemu et al., 2021). It seems that DNA methylation profile is a signature that distinguishes MAPinf status from MAPneg status. It has been revealed that disease-specific DNA methylation profiles of intestinal epithelial cells from the ascending colon could accurately separate Inflammatory bowel disease patients from healthy controls with a sensitivity of 75% while ileal methylation signatures were capable of distinguishing Crohn’s disease from ulcerative colitis patients with a precision of 77% (Howell et al., 2018). Moreover, a participatory role of DNA methylation in the pathogenesis of MAP infection in cattle demonstrated by our data corroborates data from several studies showing aberrant changes of DNA methylation in Crohn’s disease (similar pathology as JD) and other Inflammatory bowel disease conditions (Karatzas et al., 2014; Somineni et al., 2019; Zeng et al., 2019; Li Yim et al., 2020).
Generally, only a small proportion of significant DMCs and DMRs were annotated to genic regions, 15.4 and 33.84% in IL and 12.3 and 25.44% in ILLN, respectively, indicating that majority of DMCs that may paly a role in JD are located outside of protein coding genes. This observation is in line with results of numerous genome wide association studies which have associated more variants located outside of protein coding genes with cattle production and disease traits (Jiang et al., 2010; Wang et al., 2012; Ibeagha-Awemu et al., 2016). The functional role of the DMCs and DMRs in the intergenic regions are not yet known but their impact could be potential action in cis or trans to genes. Generally, the methylation levels in the regulatory regions (promoter and 5′UTR) of genes were lower as compared to other genic regions in both tissues. This result substantiates previous findings with the method of WGBS in cattle (Zhou et al., 2018; Duan et al., 2019). Meanwhile, higher numbers of DMCs and DMRs were recorded in the intron regions as compared to other genic regions in both tissues. It is generally acknowledged that DNA methylation in promoter regions provides a mechanism of regulation of gene expression. More hypomethylated and hypermethylated DMRs were identified in the promoter/5′UTRs of genes in the ILLN as compared to the IL (Table 1), suggesting potential effects of DNA methylation on gene expression, especially in the ILLN. Majority of the identified genes with hypo/hypermethylated DMRs in their promoters or 5′UTRs have roles in multiple processes like cellular and/or metabolic processes, and immune regulation/response to stimuli (e.g., BARHL2, BLK, BTC, CCL21, GLA, CHRNG,H2AC20, GABRA4, PPA1, SLC18A1. PLAGL1, EIF4E, TDGF1, etc.). Some of these genes have been associated with mycobacterial infections including JD and other human diseases. For example, BARHL2, a member of BarH family of homeodomain proteins is a transcriptional regulator and also known to impact cell fate specification, cell differentiation, survival and migration (Juraver-Geslin et al., 2011; Ding et al., 2012). Moreover, DNA methylation of BARHL2 in exosomal DNA from gastric juice has been recognized as a predictive biomarker of gastric cancer (Yamamoto et al., 2016). BLK, a non-receptor tyrosine kinase involved in cell proliferation and differentiation has roles in B-cell receptor signaling and B-cell development. BLK was among highly downregulated genes following MAP infection in mice (Shin M.-K. et al., 2015). CCL21 is a chemokine which functions in recruiting T cells and has been associated with inflammatory bowel disease in human (Kinchen et al., 2018) and JD in cattle (Ibeagha-Awemu et al., 2021). H2AC20 gene encodes a replication-dependent histone that is a member of the histone H2A family and is associated with Riddle Syndrome and Xeroderma Pigmentosum Group E disease. PPA1 like other inorganic pyrophosphatases is important for phosphate metabolism in cells. SLC18A1, SLC27A6, SLC2A2, SLC25A53 and SLC26A5, members of the solute carrier family have varied functions including participation in fatty acid/cholesterol transport, regulation of lymphocyte signalling and modulation of diverse metabolic pathways (Song et al., 2020). Gene expression data of the same samples indicated that 36 members of the solute carrier gene family were significantly regulated by the presence of MAP (Ibeagha-Awemu et al., 2021). It is known that MAP and other mycobacteria acquires and utilises host-derived fatty acids and cholesterol for their sustenance (Wilburn et al., 2018). For example, SCL27A6 encodes FATP6, a member of the fatty acid transport protein family known to mediate the uptake of long chain fatty acids (Stahl et al., 2001). A recent study demonstrated involvement of SCL27A6 in fatty acid transport into the mammary gland and how its overexpression alters fatty acid metabolism in the bovine mammary gland (Zhang et al., 2021). Therefore, alteration of the function of SCL27A6 and probably the other solute carriers due to DNA methylation may have consequences on pathogen survival during MAP infection. EIF4E expression has been reported as changed by MAP in Holstein cattle (Ibeagha-Awemu et al., 2021). PLAGL1 functions as a suppressor of cell growth and its promoter methylation or deletion has been reported in cancerous cells (Peille et al., 2013; Li et al., 2014).
Results of functional analysis of IL and ILLN DMR genes further portrayed the potent effect of MAP on DNA methylation in the ILLN tissue as compared to IL tissue, whereby more GO terms and KEGG pathways were enriched for ILLN DMR genes as compared to IL DMR genes. In the IL, majority of enriched BP GO terms are related to cellular processes, metabolic processes, transport and system development processes suggesting that these processes were more sensitive to DNA methylation as opposed to only about 1% of BP GO terms being disease/immune related (e.g. regulation of mononuclear cell migration and positive regulation of B cell activation). Meanwhile, more immune/disease pathways and BP GO terms were enriched for ILLN DMR genes, suggesting that the immune response was sensitive to regulation by DNA methylation in this tissue. This result corroborates our recent findings of an activated immune state in the IL and a depressed immune response in the ILLN in response to MAP presence (Ibeagha-Awemu et al., 2021).
In the IL, it was interesting to note that 22 genes were enriched for 4 or more pathways, including PLCB1 (23 pathways), GNAI1 (22 pathways), ITPR1 (21 pathways), RAF1 (20 pathways), CALM2 (16 pathways), PIK3R3 (15 pathways), MAPK10 (12 pathways), CREB5 (10 pathways) and BCL2 (9 pathways), etc., indicative of potentially important regulatory effects of DNA methylation of these genes and corresponding pathways during MAP infection. This is supported by the fact that some of them (genes and pathways) have been associated with JD in cattle and human diseases (e.g. cancer, tuberculosis, leishmaniasis, etc). For example, GNAI1 and GNAI3 were found to reduce colitis-associated tumorigenesis in mice through blocking of IL6 signaling and down-regulation of the expression of GNAI2 (Li et al., 2019). Moreover, GNAI1 was found as enriched in four pathways in human macrophages following infection by Leishmania (Fernandes et al., 2016). ITPR1 which regulates important molecules like platelets in the innate and adaptive immune system was recently found in a quantitative trait loci (QTL) region for JD in Jersey cattle (Kiser et al., 2017). Furthermore, many identified GWAS (genome wide association study) associated genes or genes within QTL regions for JD like PPFIBP1, ERGIC2, ITPR2, LEF1, DKK2, DEF6, CCDC91, etc., (Gao et al., 2018; Mallikarjunappa et al., 2018), presented different methylation status between MAPinf and MAPneg cows in this study, suggesting a possibility of mechanistic interactions between epigenetic and genetic signals in JD progression. CALM2, known to be involved in the regulation of a large number of enzymes and other proteins was reported as downregulated during MAP enteric colonization of ileal tissues of neonatal calves (Khare et al., 2012). Meanwhile, CREB5 was among upregulated genes during tuberculosis infection in humans (Alam et al., 2019). Majority of the pathways enriched for IL DMR genes were highly interconnected (Figure 6) and notable ones like MARK Signalling pathway, C-type lectin receptor signaling pathway, Cushing syndrome, Inflammatory mediator regulation of TRP channels, cAMP signaling pathway have reported associations with JD and other mycobacterial infections (Rybaczyk et al., 2009; Ariel et al., 2020; Ibeagha-Awemu et al., 2021) or are being associated with JD for the first time.
Enrichment of more immune/disease related pathways for ILLN DMR genes as compared to IL DMR genes including notable pathways like Bacterial invasion of epithelial cells, Cushing syndrome, Transcriptional misregulation in cancer, Pathways in cancer, Inflammatory mediator regulation of TRP channels, Cell adhesion molecules (CAMs), Platelet activation, amongst others, emphases the involvement of DNA methylation in the immune response processes to MAP presence in this tissue. Many ILLN DMR genes (n = 63) were enriched in four to 28 pathways suggesting central roles in JD. For example, the top ILLN pathways driving genes like CACNA1A (10 pathways), CAMK2A (14 pathways), CAMK2D (16 pathways), CREB3L2 (10 pathways), GNAQ (24 pathways), GSK3B (10 pathways), ITPR2 (19 pathways), PLCB1 (28 pathways), PPP3CA (10 pathways), PRKACB (26 pathways), PRKCB (27 pathways), ADCY1 (22 pathways), etc., are mainly involved in binding, catalytic and transporter activities. Apart from a reported role of GSK3B in the regulation of milk synthesis and proliferation via mTOR/S6K1 signaling pathway in dairy cow mammary gland epithelial cells (Zhang et al., 2014) or report that MAP fibronectin attachment protein (FAP) mediated dendritic cell CD8+ T cell proliferation and cytotoxic T lymphocyte activity is via GSK-3 (Noh et al., 2012), there are no reports of association of these genes with bovine JD. Considering that these genes are enriched in an appreciable number of immune/disease pathways during subclinical MAP infection, their roles in JD pathogenesis is worth investigating.
The Bacterial invasion of epithelial cells pathway (FDR = 0.002) and Focal adhesion pathway (FDR = 0.002) were among the top enriched pathways for ILLN DMR genes. The Focal adhesion pathway plays crucial roles in key biological processes such as cell motility, cell proliferation, cell differentiation, regulation of gene expression and cell survival, etc. Signalling events initiated by cell-matrix adhesions results in morphological alterations and modulation of gene expression. Activated focal adhesion kinase complexes with Src family kinases and other proteins to initiate multiple downstream signaling pathways to regulate diverse cellular functions, and are implicated in various human diseases including cancers (Zhao and Guan, 2011). Cell adhesion and Cell communication pathways have been linked to paratuberculosis in sheep (Gossner et al., 2017) and inflammatory bowel diseases (ulcerative and Crohn’s disease) in humans and model animals (Ma et al., 2010; Brooks et al., 2017). Emergence of the Bacterial invasion of epithelial cells pathway as a top pathway in this study is not surprising knowing that MAP through various mechanisms internalizes in sub-epithelial macrophages where it effectively subverts host cell responses to promote intracellular survival and replication, and DNA methylation may be one of the regulating mechanisms. Although several pathways were commonly enriched in both tissues (e.g. cGMP-PKG signaling pathway, Cushing syndrome, Inflammatory mediator regulation of TRP channels, MAPK signaling pathway, Gastric acid secretion pathway, etc.), the majority of the implicated DMR genes where different between the two tissues (Supplementary Tables S6D,F, S7D), again attesting to the differential regulation of the two tissues by DNA methylation alteration.
Interestingly, comparison of the present data with mRNA-Seq data (Ibeagha-Awemu et al., 2021) of the same samples indicated that mostly BP GO terms in the IL (n = 16) were commonly enriched for IL DMR genes (this data) and genes expressed (mRNA-seq) in the IL tissues. Most of the terms are implicated in the movement of cells. Meanwhile in the ILLN only regulation of B cell activation BP GO term was commonly enriched for DMR genes and DE genes, while no KEGG pathway was commonly enriched. This is not surprising given that only a limited number of BP GO terms (n = 10) and KEGG pathways (n = 7.) were enriched for differentially expressed genes in this tissue (ILLN) (Ibeagha-Awemu et al., 2021). Given the high number of DMCs and DMRs identified in the ILLN and the lower number of DE genes identified in the same samples (Ibeagha-Awemu et al., 2021), it can be deduced that, the DNA methylation state of the cells, repressed the host cellular immune response to the presence of MAP, and therefore suggest a role for DNA methylation in the regulation of the host response to MAP infection. Moreover, comparison of the DNA methylation data with gene expression data of the same animals (Table 2) suggested that DNA methylation in other gene regions also potentially affected the expression of the corresponding genes. For example, the promoter methylation levels of three genes (ELMOD1, LEF1 and PRDM1) and intron region methylation levels of 12 genes (AMN, DEF6, PLAC8, STAB2, STAT1, STK4, TNFSF13B, AOAH, CD38, CXADR, GNG7 and SPTA1) were inversely related to gene expression. In most cases however, the state of DNA methylation was not inversely related to gene expression. This data suggests that JD development may be associated with anomalous methylation.
Conclusion
This data is the first to demonstrate a participatory role of DNA methylation in the pathogenesis of MAP infection in dairy cattle. DNA methylation analysis demonstrated differential tissue responses to MAP infection with more DMCs and DMRs identified in ILLN tissues as compared to IL tissues of MAPinf compared to MAPneg cows, indicating that the ILLN was more impacted by regulation by DNA methylation as compared to IL tissue. Moreover, immune processes in the ILLN were more impacted by DNA methylation compared to the IL. Genes with hypermethylated (e.g. GSTO2, CCDC56, TERC, NLGN3, H2AC20, BARHL2, AVPR1A, EIF4E, GABRA4, PGDH, etc.) or hypomethylated (e.g. UBE2D2, ITGB1BP1, GLA, CHRNG, NCKAP5L, BTC, RBM27, BLK, SLC18A1, CCL21, TEX101, PPA1 and SUV39H1, etc.) promoters as well as pathways driving DMR genes (e.g. CACNA1A, CAMK2A, CAMK2D, CREB3L2, GNAQ, GSK3B, ITPR2, PLCB1, PPP3CA, PRKACB, PRKCB, ADCY1, etc.) identified between MAPinf and MAPneg cows in this study could be potential biomarkers of MAP infection, which can support development of improved diagnostic and therapeutic solutions for JD management.
Data Availability Statement
The datasets presented in this study can be found in online repositories and in the article/Supplementary Material. The raw sequence reads generated for this study have been deposited in the NCBI Sequence Read Archive (SRA) under the BioProject ID PRJNA771921.
Ethics Statement
The animal study was reviewed and approved by the Animal Care and Ethics Committee of Agriculture and Agri-Food Canada at the Sherbrooke Research and Development Center (AAFC-SRDC no. 466). Written informed consent for the use of animals was obtained from the farmers that participated in the study.
Author Contributions
Design of the study EI-A and NB; methodology, EI-A and SM; data curation and formal analysis, P-LD, SB, and MW; writing, EI-A; project administration and funding acquisition, NB; supervision of animal/tissue sampling and diagnosis, NB; supervision of laboratory and bioinformatics analyses, EI-A. All authors provided input in the interpretation of the results, have read and agreed to the published version of the manuscript.
Funding
This research was supported by a contribution from the Dairy Research Cluster 3 (Dairy Farmers of Canada, Canadian Dairy Network and Agriculture, and Agri-Food Canada) under the Canadian Agricultural Partnership AgriScience Program (Project AAFC J-002095). Animal sampling, diagnosis, animal selection/biopsies and some aspects of laboratory and bioinformatics analyses were supported by Projects AAFC J-000075, J-000079 and J-002223.
Conflict of Interest
The authors declare that the research was conducted in the absence of any commercial or financial relationships that could be construed as a potential conflict of interest.
Publisher’s Note
All claims expressed in this article are solely those of the authors and do not necessarily represent those of their affiliated organizations, or those of the publisher, the editors and the reviewers. Any product that may be evaluated in this article, or claim that may be made by its manufacturer, is not guaranteed or endorsed by the publisher.
Acknowledgments
We appreciate the invaluable collaboration of the veterinarians and dairy producers in the Province of Quebec and the Centre d’expertise et de services Génome Québec for performing the WGBS library preparation and sequencing.
Supplementary Material
The Supplementary Material for this article can be found online at: https://www.frontiersin.org/articles/10.3389/fgene.2021.797490/full#supplementary-material
References
Akalin, A., Kormaksson, M., Li, S., Garrett-Bakelman, F. E., Figueroa, M. E., Melnick, A., et al. (2012). methylKit: a Comprehensive R Package for the Analysis of Genome-wide DNA Methylation Profiles. Genome Biol. 13, R87. doi:10.1186/gb-2012-13-10-r87
Alam, A., Imam, N., Ahmed, M. M., Tazyeen, S., Tamkeen, N., Farooqui, A., et al. (2019). Identification and Classification of Differentially Expressed Genes and Network Meta-Analysis Reveals Potential Molecular Signatures Associated with Tuberculosis. Front. Genet. 10, 932. doi:10.3389/fgene.2019.00932
Alonso-Hearn, M., Canive, M., Blanco-Vazquez, C., Torremocha, R., Balseiro, A., Amado, J., et al. (2019). RNA-seq Analysis of Ileocecal Valve and Peripheral Blood from Holstein Cattle Infected with Mycobacterium avium subsp. paratuberculosis Revealed Dysregulation of the CXCL8/IL8 Signaling Pathway. Sci. Rep. 9, 14845. doi:10.1038/s41598-019-51328-0
Andrews, S. (2010). FastQC: A Quality Control Tool for High Throughput Sequence Data. Available at: https://www.bioinformatics.babraham.ac.uk/projects/fastqc/.
Arango-Sabogal, J. C., Côté, G., Paré, J., Labrecque, O., Roy, J. P., Buczinski, S., et al. (2016). Detection of Mycobacterium avium subsp. paratuberculosis in Tie-Stall Dairy Herds Using a Standardized Environmental Sampling Technique and Targeted Pooled Samples. Can. J. Vet. Res. 80, 175–182.
Ariel, O., Gendron, D., Dudemaine, P.-L., Gévry, N., Ibeagha-Awemu, E. M., and Bissonnette, N. (2020). Transcriptome Profiling of Bovine Macrophages Infected by Mycobacterium avium Spp. paratuberculosis Depicts Foam Cell and Innate Immune Tolerance Phenotypes. Front. Immunol. 10, 2874. doi:10.3389/fimmu.2019.02874
Ariel, O., Gendron, D., Dudemaine, P. L., Gévry, N., Ibeagha-Awemu, E. M., and Bissonnette, N. (2019). Transcriptome Profiling of Bovine Macrophages Infected by Mycobacterium avium subsp. paratuberculosis Depicts Foam Cell and Innate Immune Tolerance Phenotypes. Front. Immunol. 10, 2874. doi:10.3389/fimmu.2019.02874
Arsenault, R. J., Maattanen, P., Daigle, J., Potter, A., Griebel, P., and Napper, S. (2014). From Mouth to Macrophage: Mechanisms of Innate Immune Subversion by Mycobacterium avium subsp. paratuberculosis. Vet. Res. 45, 54. doi:10.1186/1297-9716-45-54
Benjamini, Y., and Hochberg, Y. (1995). Controlling the False Discovery Rate: A Practical and Powerful Approach to Multiple Testing. J. R. Stat. Soc. Ser. B (Methodological) 57, 289–300. doi:10.1111/j.2517-6161.1995.tb02031.x
Bindea, G., Mlecnik, B., Hackl, H., Charoentong, P., Tosolini, M., Kirilovsky, A., et al. (2009). ClueGO: a Cytoscape Plug-In to Decipher Functionally Grouped Gene Ontology and Pathway Annotation Networks. Bioinformatics 25, 1091–1093. doi:10.1093/bioinformatics/btp101
Bo, M., Jasemi, S., Uras, G., Erre, G. L., Passiu, G., and Sechi, L. A. (2020). Role of Infections in the Pathogenesis of Rheumatoid Arthritis: Focus on Mycobacteria. Microorganisms 8, 1459. doi:10.3390/microorganisms8101459
Brooks, J., Watson, A., and Korcsmaros, T. (2017). Omics Approaches to Identify Potential Biomarkers of Inflammatory Diseases in the Focal Adhesion Complex. Genomics, Proteomics & Bioinformatics 15, 101–109. doi:10.1016/j.gpb.2016.12.003
Cavalcante, R. G., and Sartor, M. A. (2017). Annotatr: Genomic Regions in Context. Bioinformatics (Oxford, England) 33, 2381–2383. doi:10.1093/bioinformatics/btx183
Ding, Q., Joshi, P. S., Xie, Z.-H., Xiang, M., and Gan, L. (2012). BARHL2 Transcription Factor Regulates the Ipsilateral/contralateral Subtype Divergence in Postmitotic dI1 Neurons of the Developing Spinal Cord. Proc. Natl. Acad. Sci. 109, 1566–1571. doi:10.1073/pnas.1112392109
Dobson, B., O’brien, R., and Griffin, F. (2021). “The Search for Candidate Genes that Characterise Susceptibility or Resistance to Mycobacterium avium subsp. paratuberculosis,”in Proceedings of 11 International Colloquium on Paratuberculosis. Editors S. S. Nielsen, and D. Bakker (Sydney, Australia: International Association For Paratuberculosis) 2001 Jul, 92–93.
Doherty, R., Farrelly, C. O., and Meade, K. G. (2014). Comparative Epigenetics: Relevance to the Regulation of Production and Health Traits in Cattle. Anim. Genet. 45, 3–14. doi:10.1111/age.12140
Doherty, R., Whiston, R., Cormican, P., Finlay, E. K., Couldrey, C., Brady, C., et al. (2016). The CD4+ T Cell Methylome Contributes to a Distinct CD4+ T Cell Transcriptional Signature in Mycobacterium Bovis-Infected Cattle. Sci. Rep. 6, 31014. doi:10.1038/srep31014
Duan, J. E., Jiang, Z. C., Alqahtani, F., Mandoiu, I., Dong, H., Zheng, X., et al. (2019). Methylome Dynamics of Bovine Gametes and In Vivo Early Embryos. Front. Genet. 10, 512. doi:10.3389/fgene.2019.00512
Facciuolo, A., Lee, A. H., Gonzalez Cano, P., Townsend, H. G. G., Falsafi, R., Gerdts, V., et al. (2020). Regional Dichotomy in Enteric Mucosal Immune Responses to a Persistent Mycobacterium avium subsp. paratuberculosis Infection. Front. Immunol. 11, 1020. doi:10.3389/fimmu.2020.01020
Farrell, D., Shaughnessy, R. G., Britton, L., Machugh, D. E., Markey, B., and Gordon, S. V. (2015). The Identification of Circulating MiRNA in Bovine Serum and Their Potential as Novel Biomarkers of Early Mycobacterium avium subsp paratuberculosis Infection. PLoS One 10, e0134310. doi:10.1371/journal.pone.0134310
Fernandes, M. C., Dillon, L. A., Belew, A. T., Bravo, H. C., Mosser, D. M., and El-Sayed, N. M. (2016). Dual Transcriptome Profiling of Leishmania-Infected Human Macrophages Reveals Distinct Reprogramming Signatures. mBio 7, e00027–00016. doi:10.1128/mBio.00027-16
Fock-Chow-Tho, D., Topp, E., Ibeagha-Awemu, E. A., and Bissonnette, N. (2017). Comparison of Commercial DNA Extraction Kits and Quantitative PCR Systems for Better Sensitivity in Detecting the Causative Agent of Paratuberculosis in Dairy Cow Fecal Samples. J. Dairy Sci. 100, 572–581. doi:10.3168/jds.2016-11384
Gao, Y., Jiang, J., Yang, S., Cao, J., Han, B., Wang, Y., et al. (2018). Genome-wide Association Study of Mycobacterium avium subsp. paratuberculosis Infection in Chinese Holstein. BMC Genomics 19, 972. doi:10.1186/s12864-018-5385-3
Gossner, A., Watkins, C., Chianini, F., and Hopkins, J. (2017). Pathways and Genes Associated with Immune Dysfunction in Sheep Paratuberculosis. Sci. Rep. 7, 46695. doi:10.1038/srep46695
Gu, Z., Gu, L., Eils, R., Schlesner, M., and Brors, B. (2014). Circlize Implements and Enhances Circular Visualization in R. Bioinformatics 30, 2811–2812. doi:10.1093/bioinformatics/btu393
Guo, W., Fiziev, P., Yan, W., Cokus, S., Sun, X., Zhang, M. Q., et al. (2013). BS-Seeker2: a Versatile Aligning Pipeline for Bisulfite Sequencing Data. BMC Genomics 14, 774. doi:10.1186/1471-2164-14-774
Gupta, P., Peter, S., Jung, M., Lewin, A., Hemmrich-Stanisak, G., Franke, A., et al. (2019). Analysis of Long Non-coding RNA and mRNA Expression in Bovine Macrophages Brings up Novel Aspects of Mycobacterium avium subsp. paratuberculosis Infections. Sci. Rep. 9, 1571. doi:10.1038/s41598-018-38141-x
Gupta, S. K., Maclean, P. H., Ganesh, S., Shu, D., Buddle, B. M., Wedlock, D. N., et al. (2018). Detection of microRNA in Cattle Serum and Their Potential Use to Diagnose Severity of Johne's Disease. J. Dairy Sci. 101, 10259–10270. doi:10.3168/jds.2018-14785
Hempel, R. J., Bannantine, J. P., and Stabel, J. R. (2016). Transcriptional Profiling of Ileocecal Valve of Holstein Dairy Cows Infected with Mycobacterium avium subsp. paratuberculosis. PloS one 11, e0153932. doi:10.1371/journal.pone.0153932
Hoeke, L., Sharbati, J., Pawar, K., Keller, A., Einspanier, R., and Sharbati, S. (2013). Intestinal Salmonella typhimurium Infection Leads to miR-29a Induced Caveolin 2 Regulation. PLOS ONE 8, e67300. doi:10.1371/journal.pone.0067300
Howell, K. J., Kraiczy, J., Nayak, K. M., Gasparetto, M., Ross, A., Lee, C., et al. (2018). DNA Methylation and Transcription Patterns in Intestinal Epithelial Cells from Pediatric Patients with Inflammatory Bowel Diseases Differentiate Disease Subtypes and Associate with Outcome. Gastroenterology 154, 585–598. doi:10.1053/j.gastro.2017.10.007
Ibeagha-Awemu, E. M., Bissonnette, N., Do, D. N., Dudemaine, P.-L., Wang, M., Facciuolo, A., et al. (2021). Regionally Distinct Immune and Metabolic Transcriptional Responses in the Bovine Small Intestine and Draining Lymph Nodes during a Subclinical Mycobacterium avium subsp. paratuberculosis Infection. Front. Immunol. 12, 760931. doi:10.3389/fimmu.2020.01020
Ibeagha-Awemu, E. M., Peters, S. O., Akwanji, K. A., Imumorin, I. G., and Zhao, X. (2016). High Density Genome Wide Genotyping-By-Sequencing and Association Identifies Common and Low Frequency SNPs, and Novel Candidate Genes Influencing Cow Milk Traits. Sci. Rep. 6, 31109. doi:10.1038/srep31109
Ibeagha-Awemu, E. M., and Zhao, X. (2015). Epigenetic marks: Regulators of Livestock Phenotypes and Conceivable Sources of Missing Variation in Livestock Improvement Programs. Front. Genet. 6, 302. doi:10.3389/fgene.2015.00302
Jiang, L., Liu, J., Sun, D., Ma, P., Ding, X., Yu, Y., et al. (2010). Genome Wide Association Studies for Milk Production Traits in Chinese Holstein Population. PloS one 5, e13661. doi:10.1371/journal.pone.0013661
Juraver-Geslin, H. A., Ausseil, J. J., Wassef, M., and Durand, B. C. (2011). Barhl2 Limits Growth of the Diencephalic Primordium through Caspase3 Inhibition of -catenin Activation. Proc. Natl. Acad. Sci. 108, 2288–2293. doi:10.1073/pnas.1014017108
Kampstra, P. (2008). Beanplot: A Boxplot Alternative for Visual Comparison of Distributions. J. Stat. Softw. 28, 1. doi:10.18637/jss.v028.c01
Karatzas, P. S., Gazouli, M., Safioleas, M., and Mantzaris, G. J. (2014). DNA Methylation Changes in Inflammatory Bowel Disease. Ann. Gastroenterol. 27, 125–132. doi:10.1093/ecco-jcc/jjv176
Khare, S., Lawhon, S. D., Drake, K. L., Nunes, J. E. S., Figueiredo, J. F., Rossetti, C. A., et al. (2012). Systems Biology Analysis of Gene Expression during In Vivo Mycobacterium avium Paratuberculosis Enteric Colonization Reveals Role for Immune Tolerance. PLOS ONE 7, e42127. doi:10.1371/journal.pone.0042127
Kinchen, J., Chen, H. H., Parikh, K., Antanaviciute, A., Jagielowicz, M., Fawkner-Corbett, D., et al. (2018). Structural Remodeling of the Human Colonic Mesenchyme in Inflammatory Bowel Disease. Cell 175, 372–386. e317. doi:10.1016/j.cell.2018.08.067
Kiser, J. N., White, S. N., Johnson, K. A., Hoff, J. L., Taylor, J. F., and Neibergs, H. L. (2017). Identification of Loci Associated with Susceptibility to Mycobacterium avium subsp. paratuberculosis (Map) Tissue Infection in Cattle. J. Anim. Sci. 95, 1080–1091. doi:10.2527/jas.2016.1152
Koets, A. P., Eda, S., and Sreevatsan, S. (2015). The within Host Dynamics of Mycobacterium avium subsp. paratuberculosis Infection in Cattle: where Time and Place Matter. Vet. Res. 46, 61. doi:10.1186/s13567-015-0185-0
Krueger, F. (2012). Galore: A Wrapper Tool Around Cutadapt and FastQC to Consistently Apply Quality and Adapter Trimming to FastQ Files, with Some Extra Functionality for RRBS Data. Available at: https://www.bioinformatics.babraham.ac.uk/projects/trim_galore/.
Li, H., Handsaker, B., Wysoker, A., Fennell, T., Ruan, J., Homer, N., et al. Genome Project Data Processing Subgroup (2009). The Sequence Alignment/Map Format and SAMtools. Bioinformatics 25, 2078–2079. doi:10.1093/bioinformatics/btp352
Li Yim, A. Y. F., Duijvis, N. W., Ghiboub, M., Sharp, C., Ferrero, E., Mannens, M. M. A. M., et al. (2020). Whole-genome DNA Methylation Profiling of CD14+ Monocytes Reveals Disease Status and Activity Differences in Crohn's Disease Patients. J. Clin. Med. 9, 1055. doi:10.3390/jcm9041055
Li, Z.-W., Sun, B., Gong, T., Guo, S., Zhang, J., Wang, J., et al. (2019). GNAI1 and GNAI3 Reduce Colitis-Associated Tumorigenesis in Mice by Blocking IL6 Signaling and Down-Regulating Expression of GNAI2. Gastroenterology 156, 2297–2312. doi:10.1053/j.gastro.2019.02.040
Li, Z., Ding, Y., Zhu, Y., Yin, M., Le, X., Wang, L., et al. (2014). Both gene Deletion and Promoter Hyper-Methylation Contribute to the Down-Regulation of ZAC/PLAGL1 Gene in Gastric Adenocarcinomas: a Case Control Study. Clin. Res. Hepatol. Gastroenterol. 38, 744–750. doi:10.1016/j.clinre.2013.06.007
Liu, P. T., and Modlin, R. L. (2008). Human Macrophage Host Defense against Mycobacterium tuberculosis. Curr. Opin. Immunol. 20, 371–376. doi:10.1016/j.coi.2008.05.014
Ma, Y., Semba, S., Maemoto, A., Takeuchi, M., Kameshita, I., Ishida, A., et al. (2010). Oxazolone-induced Over-expression of Focal Adhesion Kinase in Colonic Epithelial Cells of Colitis Mouse Model. FEBS Lett. 584, 3949–3954. doi:10.1016/j.febslet.2010.07.054
Mallikarjunappa, S., Sargolzaei, M., Brito, L. F., Meade, K. G., Karrow, N. A., and Pant, S. D. (2018). Short Communication: Uncovering Quantitative Trait Loci Associated with Resistance to Mycobacterium avium subsp. paratuberculosis Infection in Holstein Cattle Using a High-Density Single Nucleotide Polymorphism Panel. J. Dairy Sci. 101, 7280–7286. doi:10.3168/jds.2018-14388
Marete, A., Ariel, O., Ibeagha-Awemu, E., and Bissonnette, N. (2021b). Identification of Long Non-coding RNA Isolated from Naturally Infected Macrophages and Associated with Bovine Johne's Disease in Canadian Holstein Using a Combination of Neural Networks and Logistic Regression. Front. Vet. Sci. 8, 639053. doi:10.3389/fvets.2021.639053
Marete, A., Ariel, O., Ibeagha-Awemu, E., and Bissonnette, N. (2021a). Identification of Long Non-coding RNA Isolated from Naturally Infected Macrophages and Associated with Bovine Johne's Disease in Canadian Holstein Using a Combination of Neural Networks and Logistic Regression. Front. Vet. Sci. 8, 639053. doi:10.3389/fvets.2021.639053
Marete, A., Schenkel, F., Kelton, D., Chesnais, J., Miglior, F., Ibeagha-Awemu, E., et al. (2021c). Sequence-based GWAS Identifies Candidate Genes for Susceptibility to MAP Infection in Canadian Holstein Journal Of Genetics And Genomics Submitted. BMC Genomics. 22. 162. doi:10.1186/s12864-021-07487-4
Martin, M. (2011). Cutadapt Removes Adapter Sequences from High-Throughput Sequencing Reads. EMBnet j. 17, 10–12. doi:10.14806/ej.17.1.200
Mcnees, A. L., Markesich, D., Zayyani, N. R., and Graham, D. Y. (2015). Mycobacterium Paratuberculosis as a Cause of Crohn's Disease. Expert Rev. Gastroenterol. Hepatol. 9, 1523–1534. doi:10.1586/17474124.2015.1093931
Mehta, M. D., and Liu, P. T. (2014). microRNAs in Mycobacterial Disease: Friend or Foe? Front. Genet. 5, 231. doi:10.3389/fgene.2014.00231
Noh, K. T., Shin, S. J., Son, K. H., Jung, I. D., Kang, H. K., Lee, S. J., et al. (2012). The Mycobacterium avium subsp. paratuberculosis fibronect in Attachment Protein, a Toll-like Receptor 4 Agonist, Enhances Dendritic Cell-Based Cancer Vaccine Potency. Exp. Mol. Med. 44, 340–349. doi:10.3858/emm.2012.44.5.038
Over, K., Crandall, P. G., O’Bryan, C. A., and Ricke, S. C. (2011). Current Perspectives onMycobacterium aviumsubsp.paratuberculosis,Johne's Disease, and Crohn's Disease: a Review. Crit. Rev. Microbiol. 37, 141–156. doi:10.3109/1040841x.2010.532480
Pawar, K., Sharbati, J., Einspanier, R., and Sharbati, S. (2016). Mycobacterium Bovis BCG Interferes with miR-3619-5p Control of Cathepsin S in the Process of Autophagy. Front Cel Infect Microbiol 6, 27. doi:10.3389/fcimb.2016.00027
Peille, A.-L., Brouste, V., Kauffmann, A., Lagarde, P., Le Morvan, V., Coindre, J.-M., et al. (2013). Prognostic Value of PLAGL1-specific CpG Site Methylation in Soft-Tissue Sarcomas. PLoS One 8, e80741. doi:10.1371/journal.pone.0080741
Rybaczyk, L., Rozmiarek, A., Circle, K., Grants, I., Needleman, B., Wunderlich, J. E., et al. (2009). New Bioinformatics Approach to Analyze Gene Expressions and Signaling Pathways Reveals Unique Purine Gene Dysregulation Profiles that Distinguish between CD and UC. Inflamm. Bowel Dis. 15, 971–984. doi:10.1002/ibd.20893
Shannon, P., Markiel, A., Ozier, O., Baliga, N. S., Wang, J. T., Ramage, D., et al. (2003). Cytoscape: a Software Environment for Integrated Models of Biomolecular Interaction Networks. Genome Res. 13, 2498–2504. doi:10.1101/gr.1239303
Sharbati, J., Lewin, A., Kutz-Lohroff, B., Kamal, E., Einspanier, R., and Sharbati, S. (2011). Integrated microRNA-mRNA-Analysis of Human Monocyte Derived Macrophages upon Mycobacterium avium Subsp. Hominissuis Infection. PLoS One 6, e20258. doi:10.1371/journal.pone.0020258
Shin, M.-K., Park, H., Shin, S. W., Jung, M., Lee, S.-H., Kim, D.-Y., et al. (2015a). Host Transcriptional Profiles and Immunopathologic Response Following Mycobacterium avium subsp. Paratuberculosis Infection in Mice. PLOS ONE 10, e0138770. doi:10.1371/journal.pone.0138770
Shin, M. K., Shin, S. W., Jung, M., Park, H., Park, H. E., and Yoo, H. S. (2015b). Host Gene Expression for Mycobacterium avium subsp. paratuberculosis Infection in Human THP-1 Macrophages. Pathog. Dis. 73, ftv031. doi:10.1093/femspd/ftv031
Somineni, H. K., Venkateswaran, S., Kilaru, V., Marigorta, U. M., Mo, A., Okou, D. T., et al. (2019). Blood-Derived DNA Methylation Signatures of Crohn's Disease and Severity of Intestinal Inflammation. Gastroenterology 156, 2254–2265. e2253. doi:10.1053/j.gastro.2019.01.270
Song, Q., Decato, B., Hong, E. E., Zhou, M., Fang, F., Qu, J., et al. (2013). A Reference Methylome Database and Analysis Pipeline to Facilitate Integrative and Comparative Epigenomics. PloS one 8, e81148. doi:10.1371/journal.pone.0081148
Song, W., Li, D., Tao, L., Luo, Q., and Chen, L. (2020). Solute Carrier Transporters: the Metabolic Gatekeepers of Immune Cells. Acta Pharmaceutica Sinica B 10, 61–78. doi:10.1016/j.apsb.2019.12.006
Stahl, A., Gimeno, R. E., Tartaglia, L. A., and Lodish, H. F. (2001). Fatty Acid Transport Proteins: a Current View of a Growing Family. Trends Endocrinol. Metab. 12, 266–273. doi:10.1016/s1043-2760(01)00427-1
Thompson, R. P., Nilsson, E., and Skinner, M. K. (2020). Environmental Epigenetics and Epigenetic Inheritance in Domestic Farm Animals. Anim. Reprod. Sci. 220, 106316. doi:10.1016/j.anireprosci.2020.106316
Timms, V. J., Daskalopoulos, G., Mitchell, H. M., and Neilan, B. A. (2016). The Association of Mycobacterium avium subsp. paratuberculosis with Inflammatory Bowel Disease. PLOS ONE 11, e0148731. doi:10.1371/journal.pone.0148731
Triantaphyllopoulos, K. A., Ikonomopoulos, I., and Bannister, A. J. (2016). Epigenetics and Inheritance of Phenotype Variation in Livestock. Epigenetics & Chromatin 9, 31. doi:10.1186/s13072-016-0081-5
Wang, J., Yang, K., Zhou, L., Wu, Y., Zhu, M., Lai, X., et al. (2013). MicroRNA-155 Promotes Autophagy to Eliminate Intracellular Mycobacteria by Targeting Rheb. Plos Pathog. 9, e1003697. doi:10.1371/journal.ppat.1003697
Wang, M., Bissonnette, N., Griebel, P., Dudemaine, P.-L., Do, D. N., and Ibeagha-Awemu, E. M. (2019a). PSVI-15 Transcriptome Analysis of Ileal Lymph Nodes Identifies Key microRNAs Affecting Disease Progression in Holstein Cows with Subclinical Johne's Disease. J. Anim. Sci. 97, 207–208. doi:10.1093/jas/skz258.426
Wang, M., Bissonnette, N., Griebel, P., Dudemaine, P.-L., Do, D. N., Mao, Y., et al. (2019b). PSVI-14 Differentially Expressed microRNAs with Potential Regulatory Roles in Ileum of Holstein Cows with Subclinical Johne's Disease. J. Anim. Sci. 97, 206–207. doi:10.1093/jas/skz258.425
Wang, M., and Ibeagha-Awemu, E. M. (2021). Impacts of Epigenetic Processes on the Health and Productivity of Livestock. Front. Genet. 11, 1812. doi:10.3389/fgene.2020.613636
Wang, X., Wurmser, C., Pausch, H., Jung, S., Reinhardt, F., Tetens, J., et al. (2012). Identification and Dissection of Four Major QTL Affecting Milk Fat Content in the German Holstein-Friesian Population. PloS one 7, e40711. doi:10.1371/journal.pone.0040711
Wang, Y., Chen, C., Xu, X.-D., Li, H., Cheng, M.-H., Liu, J., et al. (2020). Levels of miR-125a-5p Are Altered in Mycobacterium Avium-Infected Macrophages and Associate with the Triggering of an Autophagic Response. Microbes Infect. 22, 31–39. doi:10.1016/j.micinf.2019.07.002
Wei, M., Wang, L., Wu, T., Xi, J., Han, Y., Yang, X., et al. (2016). NLRP3 Activation Was Regulated by DNA Methylation Modification during Mycobacterium tuberculosis Infection. Biomed. Res. Int. 2016, 4323281. doi:10.1155/2016/4323281
Whitlock, R. H., and Buergelt, C. (1996). Preclinical and Clinical Manifestations of Paratuberculosis (Including Pathology). Vet. Clin. North America: Food Anim. Pract. 12, 345–356. doi:10.1016/s0749-0720(15)30410-2
Whittington, R., Donat, K., Weber, M. F., Kelton, D., Nielsen, S. S., Eisenberg, S., et al. (2019). Control of Paratuberculosis: Who, Why and How. A Review of 48 Countries. BMC Vet. Res. 15, 198. doi:10.1186/s12917-019-1943-4
Wilburn, K. M., Fieweger, R. A., and Vanderven, B. C. (2018). Cholesterol and Fatty Acids Grease the Wheels of Mycobacterium tuberculosis Pathogenesis. Pathog. Dis. 76, fty021. doi:10.1093/femspd/fty021
Yamamoto, H., Watanabe, Y., Oikawa, R., Morita, R., Yoshida, Y., Maehata, T., et al. (2016). BARHL2 Methylation Using Gastric Wash DNA or Gastric Juice Exosomal DNA Is a Useful Marker for Early Detection of Gastric Cancer in an H. pylori -Independent Manner. Clin. Transl Gastroenterol. 7, e184. doi:10.1038/ctg.2016.40
Zeng, Z., Mukherjee, A., and Zhang, H. (2019). From Genetics to Epigenetics, Roles of Epigenetics in Inflammatory Bowel Disease. Front. Genet. 10, 1017. doi:10.3389/fgene.2019.01017
Zhang, H., Shen, Z., Yang, Z., Jiang, H., Chu, S., Mao, Y., et al. (2021). Abundance of Solute Carrier Family 27 Member 6 (SLC27A6) in the Bovine Mammary Gland Alters Fatty Acid Metabolism. Food Funct. 12, 4909–4920. doi:10.1039/d0fo03289a
Zhang, X., Zhao, F., Si, Y., Huang, Y., Yu, C., Luo, C., et al. (2014). GSK3β Regulates Milk Synthesis in and Proliferation of Dairy Cow Mammary Epithelial Cells via the mTOR/S6K1 Signaling Pathway. Molecules 19, 9435–9452. doi:10.3390/molecules19079435
Zhao, X., and Guan, J.-L. (2011). Focal Adhesion Kinase and its Signaling Pathways in Cell Migration and Angiogenesis. Adv. Drug Deliv. Rev. 63, 610–615. doi:10.1016/j.addr.2010.11.001
Zhou, Y., Connor, E. E., Bickhart, D. M., Li, C., Baldwin, R. L., Schroeder, S. G., et al. (2018). Comparative Whole Genome DNA Methylation Profiling of Cattle Sperm and Somatic Tissues Reveals Striking Hypomethylated Patterns in Sperm. GigaScience 7, giy039. doi:10.1093/gigascience/giy039
Keywords: DNA methylation, Johne’s disease, Mycobacterium avim subsp. paratuberculosis, dairy cow, ileum and ileum lymph node, differentially methylated cytosines and differentially methylated regions
Citation: Ibeagha-Awemu EM, Bissonnette N, Bhattarai S, Wang M, Dudemaine P-L, McKay S and Zhao X (2021) Whole Genome Methylation Analysis Reveals Role of DNA Methylation in Cow’s Ileal and Ileal Lymph Node Responses to Mycobacterium avium subsp. paratuberculosis Infection. Front. Genet. 12:797490. doi: 10.3389/fgene.2021.797490
Received: 18 October 2021; Accepted: 22 November 2021;
Published: 21 December 2021.
Edited by:
Dhirendra Kumar, National Institute of Environmental Health Sciences (NIEHS), United StatesReviewed by:
Yufeng Qin, Nanjing Medical University, ChinaSatish Sati, University of Pennsylvania, United States
Copyright © 2021 Suraj Bhattarai, Stephanie McKay, Xin Zhao and Her Majesty the Queen in Right of Canada, as represented by the Minister of Agriculture and Agri-Food Canada for the contribution of Eveline M. Ibeagha-Awemu, Nathalie Bissonnette, Mengqi Wang and Pier-Luc Dudemaine. This is an open-access article distributed under the terms of the Creative Commons Attribution License (CC BY). The use, distribution or reproduction in other forums is permitted, provided the original author(s) and the copyright owner(s) are credited and that the original publication in this journal is cited, in accordance with accepted academic practice. No use, distribution or reproduction is permitted which does not comply with these terms.
*Correspondence: Eveline M. Ibeagha-Awemu, Eveline.ibeagha-awemu@agr.gc.ca; Nathalie Bissonnette, nathalie.bissonnette@agr.gc.ca