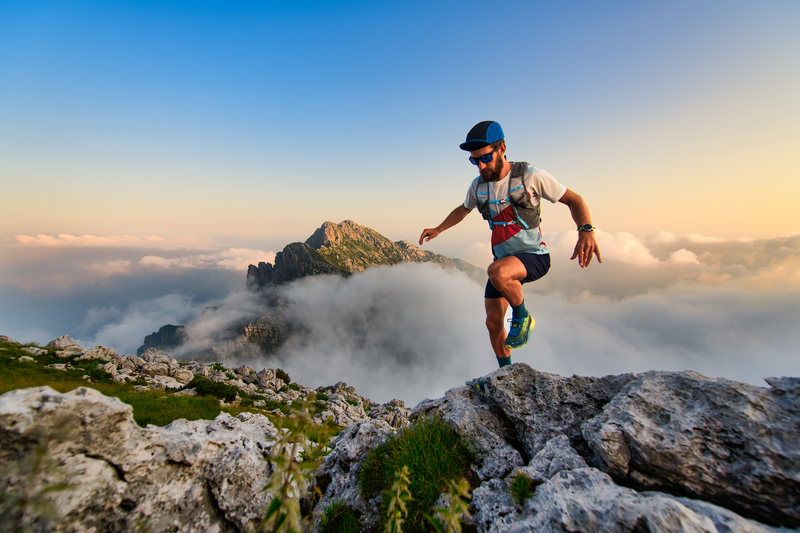
94% of researchers rate our articles as excellent or good
Learn more about the work of our research integrity team to safeguard the quality of each article we publish.
Find out more
ORIGINAL RESEARCH article
Front. Genet. , 14 December 2021
Sec. Genetics of Common and Rare Diseases
Volume 12 - 2021 | https://doi.org/10.3389/fgene.2021.791856
Noninvasive prenatal diagnosis (NIPD) of single-gene disorders has recently become the focus of clinical laboratories. However, reports on the clinical application of NIPD of Duchenne muscular dystrophy (DMD) are limited. This study aimed to evaluate the detection performance of haplotype-based NIPD of DMD in a real clinical environment. Twenty-one DMD families at 7–12 weeks of gestation were prospectively recruited. DNA libraries of cell-free DNA from the pregnant and genomic DNA from family members were captured using a custom assay for the enrichment of DMD gene exons and spanning single-nucleotide polymorphisms, followed by next-generation sequencing. Parental haplotype phasing was based on family linkage analysis, and fetal genotyping was inferred using the Bayes factor through target maternal plasma sequencing. Finally, the entire experimental process was promoted in the local clinical laboratory. We recruited 13 complete families, 6 families without paternal samples, and 2 families without probands in which daughter samples were collected. Two different maternal haplotypes were constructed based on family members in all 21 pedigrees at as early as 7 gestational weeks. Among the included families, the fetal genotypes of 20 families were identified at the first blood collection, and a second blood collection was performed for another family due to low fetal concentration. The NIPD result of each family was reported within 1 week. The fetal fraction in maternal cfDNA ranged from 1.87 to 11.68%. In addition, recombination events were assessed in two fetuses. All NIPD results were concordant with the findings of invasive prenatal diagnosis (chorionic villus sampling or amniocentesis). Exon capture and haplotype-based NIPD of DMD are regularly used for DMD genetic diagnosis, carrier screening, and noninvasive prenatal diagnosis in the clinic. Our method, haplotype-based early screening for DMD fetal genotyping via cfDNA sequencing, has high feasibility and accuracy, a short turnaround time, and is inexpensive in a real clinical environment.
Duchenne muscular dystrophy (DMD, OMIM# 310200), the most common X-linked recessive inherited muscle disease, affects approximately 1 in 3,600–6,000 live male births (Walter and Reilich, 2017; Coote et al., 2018; Fox et al., 2020). DMD is usually not recognized by ultrasound examination or serum screening, and is often diagnosed after delivery. No gold standard treatment for DMD has been established to date (van Deutekom and van Ommen, 2003; Verhaart and Aartsma-Rus, 2019). Consequently, prenatal diagnosis is necessary for most DMD families. Traditional prenatal diagnosis is associated with an invasive procedure similar to chorionic villus sampling (CVS) or amniocentesis. However, this invasive procedure may lead to miscarriage or stillbirth (incidence: 0.1–1.3%), and is not applicable to patients with sampling contraindications (Agarwal and Alfirevic, 2012; Bakker et al., 2017; Salomon et al., 2019; Di Mascio et al., 2020).
The discovery of cell-free fetal DNA (cffDNA) in maternal circulation led to a new era of noninvasive prenatal testing (Lo et al., 1997; Chiu et al., 2002). Recently, several teams have reported using haplotype-based noninvasive prenatal diagnosis (NIPD) as an alternative solution to overcome these limitations while maintaining high accuracy (Xu et al., 2015; Yoo et al., 2015; Parks et al., 2016). Nevertheless, because of high costs or long turnaround times, cffDNA technologies for DMD remain at the experimental laboratory stage. The practicability of clinical applications of haplotype-based NIPD thus requires further evaluation. In clinical practice, the ideal platform for DMD prenatal diagnosis needs to be designed for fetal genotyping and be equally applicable to the proband and carrier. Previously, before detection of fetal genotype, multiplex ligation-dependent probe amplification plus Sanger sequencing required multiple steps to validate the mother as a carrier (Lalic et al., 2005). In this study, we developed a high-accuracy assay that can simultaneously be utilized for the NIPD of the fetal genotype and gene detection for the proband and mother.
In this study, we performed carrier detection and NIPD on 21 enrolled DMD families as a routine test. The procedure of sample collection, DNA extraction, variant calling, and haplotype analysis were all completed by the in-house staff of First Affiliated Hospital of Zhengzhou University, Zhengzhou, Henan, China.
As shown in Figure 1, the SNP-based NIPT workflow involves several steps. First, whole blood samples from members of at-risk DMD families were collected. Then, the average depth of each sample was evaluated. The maternal pathogenic and wild-type haplotype were further constructed on the basis of family-based linkage analysis to ascertain type 1 SNPs and type 2 SNPs. Meanwhile, the cfDNA was also sequenced to calculate fetal gender, fetal fraction, and relative haplotype dosage (RHDO). Finally, combined with the results of haplotype phasing, the RHDO was further used in recombination analysis by the circular binary segmentation (CBS) algorithm and predicting fetal genotypes using the Bayes factor.
FIGURE 1. Workflow of NIPD of DMD. After genetic counseling, samples from the qualified 21 DMD family members were collected for non-invasive prenatal diagnosis (NIPD), and the results were released within 1 week when all samples met quality control requirements. Finally, a proper invasive diagnosis was recommended to confirm the accuracy of NIPD testing.
Twenty-one DMD families (named P1-P21) were enrolled from December 2020 to August 2021 after genetic counseling and a receipt of informed consent (Supplementary Figure S1). The average gestational age of the recruited families was 10+3 weeks (Table 1). Fifteen families had complete pedigrees, whereas two families only included one daughter (P12 was unaffected, P18 was a carrier). Six families had incomplete pedigrees; i.e., no paternal samples were available (P1, P9, P13, P17, P19, and P21) (Supplementary Table S1). For each family, we collected 10 ml of peripheral blood from the pregnant mother and 2 ml of blood from the proband and father (when available). The study was approved by the Ethics Committee of First Affiliated Hospital of Zhengzhou University.
A 288.612-kb capture panel TargetSeq® One kit (iGeneTech, China), enrichment of DMD gene exons and spanning SNPs, was designed to selectively enrich target regions based on the reference genome (GRCh37/hg19) (Figure 2). The probes covered all exonic regions (including untranslated regions), about 500-bp of intronic regions adjacent to exons, and 10,000-bp of flanking regions of the DMD gene. In addition, 1,511 common SNPs (MAF >0.10, 1000 Genomes Project Phase 3) spanning a 0.5-Mb region upstream and downstream of the DMD gene were included. To determine fetal gender and maternal chimerism, the probes also covered 35 sites on the Y chromosome and 203 common SNPs (MAF >0.45, 1000 Genomes Project Phase 3) on the X chromosome. Another 213 common SNPs (MAF >0.45, 1000 Genomes Project Phase 3) scattered on autosomes were used to calculate fetal fraction.
FIGURE 2. Illustration of the capture probe design. (a) Distribution of capture probes, with blue denoting the DMD gene that spans a 0.5-Mb region, green indicating the X chromosome, orange representing the Y chromosome, purple showing chromosomes 1–22, and dashes representing regions covered by capture probes; (b) Reference allele frequency of the SNPs in the East Asian population; (c) Position of exons of the DMD gene; (d) Intronic region adjacent to exons covered by capture probes, in log10 scale; (e) Number of SNPs in each region.
Circulating cell-free DNA (cfDNA) was extracted using a cfDNA extraction kit following the manufacturer’s instructions (Nahai Bio, Chengdu, China). Genomic DNA (gDNA) was extracted from the leucocytes of peripheral blood samples of the proband and parent (when the father was available) using an in-house protocol, and then fragmented into an average length of 200 bp. cfDNA and fragmented gDNA were subsequently captured after end-repair, barcode adapter ligation, and PCR amplification. The post-capture libraries were then subjected to PCR amplification and sequenced on the Ion Proton platform (Thermo Fisher Scientific, Lithuania).
For sequencing quality control, we calculated total reads, the average sequencing depth of target regions, areas with over 30× coverage, and on-target rates. In addition, second-time sequencing was required for both gDNA samples with an average sequencing depth of <30× and cfDNA samples with an average sequencing depth of <70×.
To detect DMD sequence variations in the mothers and probands, sequencing reads of gDNA were aligned to the human reference genome (GRCh37/hg19) using TMAP software (version 5.2.25). Small variants were identified with Torrent Variant Caller software (version 5.2.25) using default parameters, after removing duplicated reads. To identify microdeletions and duplications, we used the CNVkit (version 0.9.6) software (Talevich et al., 2016) with a sliding window of 200 bp and a step length of 100 bp using normal samples as reference. Variant annotations were accomplished by ANNOVAR (Wang et al., 2010). Finally, the genotypes of all family members were identified, and probands were confirmed to inherit the same pathogenic variants from the mother.
We selected autosomal SNP loci as described in the probe design to calculate fetal fractions. After filtering out loci with depths of <100× or Phred quality scores of <13, we calculated the fetal fraction in maternal plasma (f) using the following equation:
Fetal gender was determined by the ratio between the average depth of Y loci and 213 autosomal SNP loci (chrY_ratio) in the maternal cfDNA. The threshold was set to 0.3%. A ratio of <0.3% indicated a female fetus; otherwise, it indicated a male fetus.
Haplotype phasing of maternal heterozygous SNPs was conducted based on family composition. We defined the maternal haplotype linked with the pathogenic variant as Hap1 and the other haplotype as Hap2. Then, according to fetal gender, we further classified Hap1 and Hap2 alleles into Type 1 and Type 2 (Figure 3). For a male fetus, the Type 1 and Type 2 alleles were the same as the Hap 1 and Hap 2 alleles, respectively. For a female fetus, if the paternal haplotype was available, then the Hap 1 and Hap 2 alleles identical to the paternal alleles were classified as Type 1 and Type 2. However, if the paternal haplotype was unavailable, then the method of classifying Type 1 and Type 2 alleles was the same as that of the male fetus. After haplotype phasing, quality control for SNP numbers was performed. When the number of Type 1 or Type 2 alleles was <10, this indicated consanguineous marriage. Haplotype-based NIPD was not suitable for such a family, and an invasive diagnosis was suggested.
FIGURE 3. Principle of haplotype-based noninvasive prenatal diagnosis. Haplotype phasing of the SNPs in the DMD gene and its spanning region was achieved by Mendelian Law, as shown in the left part of the figure. The maternal haplotype was shared with the proband and carried the pathogenic variant; this was defined as Hap 1. The other haplotype was defined as Hap 2. For a male fetus, Type 1 and Type 2 alleles were the same as Hap 1 and Hap 2 alleles, respectively. For a female fetus, the paternal haplotype was also assessed. Only the Hap 1 and Hap 2 alleles that were identical to paternal alleles were classified as Type 1 and Type 2. Additional fetal sequences caused an imbalance of allele dosage compared to the maternal gDNA, as shown in the right part of the figure, and were used in deducing the fetal haplotype.
To avoid the adverse influence of recombination events on RHDO, we predicted recombination events using the function “segmentByCBS” of the R package “PSCBS” (Olshen et al., 2011). Finally, based on allele frequency imbalance, we estimated the probability of inherited pathogenic haplotypes using the Bayesian method. Fetal allelic frequency was calculated separately using the following equation:
To evaluate the predicted comprehensive performance of fetal fraction, sequencing depth, and number of SNPs, we built an in silico model. The group of simulated allele dosage changes was randomly generated based on a binomial distribution by using quality control data from testing samples. The simulated process was repeated 1,000,000 times and the theoretical BF sensitivity, specificity, and no-call rate were then calculated based on the simulated data. If an expected sensitivity was <95%, another blood draw from the pregnant mother was performed 2 weeks later to obtain more reliable NIPD results.
To validate accuracy, we performed invasive prenatal diagnosis for each family according to the results of NIPD by MLPA or Sanger sequencing, wherein chorionic villus sampling (CVS) for affected fetuses or amniocentesis for carriers and unaffected fetuses was performed.
After bioinformatics analysis, we successfully identified 13 large deletions (62.0%), 6 duplications (28.6%), and 2 point mutations (9.5%) at the DMD gene locus (Table 1). Specifically, 10 (76.9%) of the identified deletions were located at the region of exons 44–55, while five (83.3%) of the duplications were located in exons 8–12 (Supplementary Figures S2, S3). These two regions are hotspots for DMD gene variants. With targeted deep sequencing, the variants in the probands were validated and the mothers were defined as carriers. For families with deletions or duplications, the carrier mother had a different read depth when compared to the baseline read depth outside the deleted region, after normalization to reference datasets. Furthermore, all the identified variants were detected in both the proband and mother. The results indicated that it is an accurate method for DMD carrier screening.
According to the autosomal loci homologous in both parents but with different genotypes, fetal fraction in maternal cfDNA was calculated, which ranged from 1.87 to 11.68% with a median of 6.21% (Table 1). However, because of low fetal fraction (0.86%) in family P5 after the first blood collection, which was lower than the quality control threshold (f ≥ 1%), we suggested another blood draw from the mother after 2 weeks to ensure detection accuracy.
Besides fetal fraction, gender determination is another critical factor for the NIPD of X-linked diseases. We used the ratio between the average depth of chromosome Y loci and 213 autosomal SNP loci (chrY_ratio) to predict fetal gender. In our dataset, we observed a significant difference in chrY_ratio between male and female fetuses, which were all <0.15% for female fetuses and >1% for male fetuses, indicating high accuracy in fetal gender determination (Figure 4A). Finally, we found 8 male fetuses and 13 female fetuses in all the enrolled families.
FIGURE 4. The relationship between fetal fraction,
The average depth of gDNA ranged from 98× to 563×, the average on-target rate for gDNA was 65.38% (43.39–85.55%), and the average coverage (≥30×) of the target region was 91.86% (75.37–97.87%) (Supplementary Table S1). For each sample of maternal plasma cfDNA, the mean depth was 290× (range 165×–490×), and the average on-target rate was 62.71% (Supplementary Table S1). All samples met the depth quality control requirements and none of these required re-sequencing.
Based on family-based strategy and fetal gender, we further classified Hap 1 and Hap 2 into Type 1 and Type 2 as described in the methods section. The average number of Type 1 and Type 2 alleles was 310 (range: 97–687) and 303 (range: 108–687) (Table 1), respectively. The inherited allele was overrepresented in the maternal plasma. To evaluate the imbalance of fetal alleles, the Bayes factor (BF) was used to predict whether the fetal inherited maternal haplotype was pathogenic. The BF values (log10-transformation) showed a positive correlation with fetal fractions for fetuses inheriting Hap 1 (Pearson correlation coefficient r = 0.63, p = 0.021), while it presented a negative correlation for fetuses inheriting Hap 2 (r = -0.77, p = 0.024) (Figure 4B).
BF showed significant accuracy in predicting fetal genotypes. For example, family P1 had a male fetus, whereas family P2 had a female fetus. The BFs of these two families were far more than 10 (2.7
FIGURE 5. The RHDO results of families P1 and P2. (A) Scatter plot of the dosage change (DC) of each allele. The X-axis is the genomic coordinate, and the Y-axis represents DC. Red dots denote the DC of the Type 1 allele (over-represented when the fetus inherited maternal Hap1, which carries the pathogenic variant), whereas blue dots are the DC of the Type 2 allele (overrepresented if the fetus inherited maternal Hap 2, which carries the wild-type DMD gene). The red and blue dashed lines indicate the expected value of DC for Type 1 and Type 2 alleles under the assumption that the fetus inherits the maternal pathogenic and wild-type haplotype. The red and blue horizontal line is the center of DC returned by the CBS algorithm. When recombination occurs, both lines will cross at the switch site. Gray rectangles indicate the range of pathogenic deletion, and the gray vertical dashed line marks the position of DMD exons. (B) Violin plot of DC. The shape around each box demonstrates the distribution of DC. The red and blue dashed lines indicate the expected value of DC for Type 1 and Type 2 alleles under the assumption that the fetus inherits the maternal pathogenic and wild-type haplotypes. The Bayes factor is labeled at the top: BF ≥ 10 indicates a positive test result, favoring the assumption that the fetus inherits the maternal pathogenic variant, while a BF ≤ 0.1 reveals a negative test result, where the fetus inherits the wild-type haplotype.
The recombination event largely influences the prediction accuracy of NIPD. In this study, recombination events downstream of the related variants were detected in two fetuses (P16, P18) by the CBS algorithm (Supplementary Figure S5). The recombination points were about 1.30 M (P16) and 0.59 M (P18) away from the related variants in these two families. The number of SNP linkages to variant positions was sufficient in generating precise NIPD results for the two families.
To validate our NIPD results, we performed CVS for high-risk families and amniocentesis for low-risk families (Supplementary Figure S6). The results of all invasive diagnoses were similar to those generated by NIPD.
In our study, NIPD was successfully utilized to assess 21 fetuses at risk for DMD, with an average gestational age of 10+3 weeks. Fetal genotypes were detected by RHDO in maternal plasma DNA and subsequently confirmed by invasive prenatal diagnosis with 100% concordance. To our best knowledge, this is the largest dataset to date for the NIPD of DMD. Furthermore, it is also the first real-world dataset generated from clinical practice.
Earlier prenatal testing for DMD can provide families with more options to prepare, as well as more time for genetic therapy (Birnkrant et al., 2018; Iftikhar et al., 2021). The minimum gestational age in our study was 7+3 weeks (Family P11), which is 5 weeks earlier than CVS and 9 weeks earlier than amniocentesis. However, early gestational age often suggests a lower fetal fraction, which requires higher assay sensitivity (Lo et al., 2010). The minimum fetal fraction in our study was 1.87% (Family P10: 8+0 weeks), and the Bayes factor of that sample was still high enough (1.3
Instead of the straightforward detection of allelic mutations, we used linkage analysis for constructing the haplotype of the DMD region. By expanding the capture probe coverage from exonic regions to their adjacent intronic regions, combined with common SNPs in deep intronic regions, we successfully identified disease-causing variants in all proband and maternal gDNA samples in parallel with SNP genotyping, including exonic deletions and duplications. Of note, we even found the exact breakpoint in two families with an exonic deletion (data not shown). Our data indicated that target capture design could also be a powerful tool for the molecular diagnosis of DMD. For haplotype-based NIPD, the error risk caused by recombination within the DMD gene should be fully considered. Yoo et al. emphasized that recombination events within the DMD gene would greatly affect dosage imbalance analysis with false predictions, and deduced one recombination case in a duplication DMD family (Yoo et al., 2015). We utilized the circular binary segmentation (CBS) algorithm to predict the recombination event, which is widely used in detecting copy number variations. We found recombination in 2 of the 21 fetuses (P16 with a male fetus and P18 with a female fetus), and the observed frequency (11%) was concordant with the findings of previous reports (6–10%) (Abbs et al., 1990; Nobile et al., 1995; Shashi et al., 1996; Giliberto et al., 2011; Ling et al., 2020). Observed recombination sites of these two fetuses were both far away from the disease-causing region and did not interfere with inferring fetal genotypes. Subsequent invasive diagnosis confirmed the NIPD results.
A complete pedigree that includes parents and probands is necessary for prenatal diagnosis and genetic counseling (Cole et al., 1978; Posch et al., 2012; Slomp et al., 2018; Gilstrop Thompson et al., 2020). Nevertheless, in clinical practice, the situation of incomplete pedigrees occurs occasionally. In our dataset, two families (P12 and P18) lacked a proband, and six families (P1, P9, P13, P17, P19, and P21) lacked paternal samples. In families in which the proband was absent, we required normal offspring for haplotype phasing. Type 1 and Type 2 SNPs were swapped after haplotype phasing to predict the inheritance of the pathogenic haplotype. In families that lacked the paternal sample, the SNP classification between a male patient (P1) and a normal male fetus (P17, P21) was not influenced because paternal alleles did not act on haplotype dosage. However, if the fetus was a female, then dosage changes were not as expected for the portion of Type 1 and Type 2 SNPs in which paternal alleles differed from proband alleles, thereby decreasing the assay performance of distinguishing female carriers (P13, P19) and normal female fetuses (P9). However, we still reported the NIPD result in case of a definite Bayesian factor. We informed patients of the above information during pre-test genetic counseling.
Quality control is indispensable for the clinical application of NIPD. We performed quality control of each sequencing sample. The primary quality control metrics were fetal fraction, the number of informative SNPs, and sequencing depth. Besides setting up a lower limit for individual parameters, secondary quality control evaluated assay performance using an in silico model by considering all these parameters together. Supplementary Figure S7 demonstrates that the 3D surface comprises predicted assay performance (sensitivity and specificity), the number of informative SNPs, and sequencing depth at 1, 2, 4, and 8% fetal fraction. It was shown that even when the fetal fraction was as low as 1%, the predicted assay sensitivity could still be over 95% when the number of informative SNPs was larger than 200 and the sequencing depth was more than 500×. The in silico model provides clinicians a direct and simple tool for evaluating result reliability, therefore minimizing the chances of false positive NIPD results.
Additionally, testing costs and turnaround time are critical issues for widespread clinical applications. To optimize costs, we utilized a strategy for panel design with the selective enrichment of gene and spanning Single-Nucleotide Polymorphisms, which only targets essential genomic regions for NIPD. Using this approach, we narrowed down the panel size to only 288.612 kb, which was the minimum among previously reported panels dedicated to the NIPD of DMD (Xu et al., 2015; Yoo et al., 2015; Parks et al., 2016; Chen et al., 2019). Only an average of 5 M sequencing reads for maternal plasma DNA and 2 M sequencing reads for each family member’s gDNA were required, indicating that the required total number of reads per family is only 11 M. In addition, we mixed gDNA libraries from the same family together in the same proportion before target DNA capture to further reduce the cost for capture probes and hybridization reagents. As a result, experimental expense per family was controlled below $500, which is comparable to other routine genetic tests, such as family-based whole-exome sequencing (Fan et al., 2012; Kitzman et al., 2012; Rabinowitz et al., 2019). In terms of time effectiveness, blood samples were processed immediately after collection, followed by library construction. Probe hybridization was performed overnight. Post-capture libraries of NIPD shared one sequencing chip with other routine genetic tests such as noninvasive fetal aneuploidy testing (NIPT) or carrier screening to accelerate queuing time for next-generation sequencing. We developed a visual web application that integrates sample analysis, management, and report generation. This application liberates clinicians from complex bioinformatic analysis and saves manpower. By utilizing the strategies described above, the minimum turnaround time is shortened to 3 days. In most cases, test reports can be sent to patients within a week, which meets a typical genetic test requirement.
The datasets for this article are not publicly available due to concerns regarding participant/patient anonymity. Requests to access the datasets should be directed to the corresponding authors.
The project passed the review of the ethics committee by the Ethics Committee for Scientific Research and Clinical Trials of the First Affiliated Hospital of Zhengzhou University. All patients and their family members signed informed consent.
Conceptualization: LS, XK, SJL, HZ and DW; Data curation: SJL, JF and LK; Formal Analysis: LK and ZZ; Funding acquisition: XK and LS; Investigation: LK; Resources: LK, ZZ, WT, SQL, and XH; Software: SJL and JF; Validation: LL. Visualization: SJL, JF, LK and DW; Writing—original draft: LK, SJL, JF, and DW; Writing—review and editing: XK, LS, HZ, DW, and GC. All authors contributed to the article and approved the submitted version.
Funding support was given to XK by the National Key Research and Development Program of China (2018YFC1002203) and Key Scientific Research Projects in Colleges and Universities of Henan Province (22A320075), LS by the National Key R&D Program of China (2018YFC1002900) and National Natural Science Foundation of China (82071656).
Authors SJL, JF, WT, SQL, FL, XH, DW and HZ are employed by Celula (China) Medical Technology Co., Ltd.
The remaining authors declare that the research was conducted in the absence of any commercial or financial relationships that could be construed as a potential conflict of interest.
All claims expressed in this article are solely those of the authors and do not necessarily represent those of their affiliated organizations, or those of the publisher, the editors and the reviewers. Any product that may be evaluated in this article, or claim that may be made by its manufacturer, is not guaranteed or endorsed by the publisher.
The Supplementary Material for this article can be found online at: https://www.frontiersin.org/articles/10.3389/fgene.2021.791856/full#supplementary-material
Abbs, S., Roberts, R. G., Mathew, C. G., Bentley, D. R., and Bobrow, M. (1990). Accurate Assessment of Intragenic Recombination Frequency within the Duchenne Muscular Dystrophy Gene. Genomics 7 (4), 602–606. doi:10.1016/0888-7543(90)90205-9
Agarwal, K., and Alfirevic, Z. (2012). Pregnancy Loss after Chorionic Villus Sampling and Genetic Amniocentesis in Twin Pregnancies: a Systematic Review. Ultrasound Obstet. Gynecol. 40 (2), 128–134. doi:10.1002/uog.10152
Bakker, M., Birnie, E., Robles de Medina, P., Sollie, K. M., Pajkrt, E., and Bilardo, C. M. (2017). Total Pregnancy Loss after Chorionic Villus Sampling and Amniocentesis: a Cohort Study. Ultrasound Obstet. Gynecol. 49 (5), 599–606. doi:10.1002/uog.15986
Birnkrant, D. J., Bushby, K., Bann, C. M., Apkon, S. D., Blackwell, A., Brumbaugh, D., et al. (2018). Diagnosis and Management of Duchenne Muscular Dystrophy, Part 1: Diagnosis, and Neuromuscular, Rehabilitation, Endocrine, and Gastrointestinal and Nutritional Management. Lancet Neurol. 17 (3), 251–267. doi:10.1016/s1474-4422(18)30024-3
Chen, Y., Yu, Q., Mao, X., Lei, W., He, M., and Lu, W. (2019). Noninvasive Prenatal Testing for Chromosome Aneuploidies and Subchromosomal Microdeletions/microduplications in a Cohort of 42,910 Single Pregnancies with Different Clinical Features. Hum. Genomics 13 (1), 60. doi:10.1186/s40246-019-0250-2
Chiu, R. W., Lau, T. K., Cheung, P. T., Gong, Z. Q., Leung, T. N., and Lo, Y. D. (2002). Noninvasive Prenatal Exclusion of Congenital Adrenal Hyperplasia by Maternal Plasma Analysis: a Feasibility Study. Clin. Chem. 48 (5), 778–780. doi:10.1093/clinchem/48.5.778
Cole, J., Conneally, P. M., Hodes, M. E., and Merritt, A. D. (1978). Genetic Family History Questionnaire. J. Med. Genet. 15 (1), 10–18. doi:10.1136/jmg.15.1.10
Coote, D. J., Davis, M. R., Cabrera, M., Needham, M., Laing, N. G., and Nowak, K. J. (2018). CUGC for Duchenne Muscular Dystrophy (DMD). Eur. J. Hum. Genet. 26 (5), 749–757. doi:10.1038/s41431-017-0013-2
Di Mascio, D., Khalil, A., Rizzo, G., Buca, D., Liberati, M., Martellucci, C. A., et al. (2020). Risk of Fetal Loss Following Amniocentesis or Chorionic Villus Sampling in Twin Pregnancy: Systematic Review and Meta‐analysis. Ultrasound Obstet. Gynecol. 56 (5), 647–655. doi:10.1002/uog.22143
Fan, H. C., Gu, W., Wang, J., Blumenfeld, Y. J., El-Sayed, Y. Y., and Quake, S. R. (2012). Non-invasive Prenatal Measurement of the Fetal Genome. Nature 487 (7407), 320–324. doi:10.1038/nature11251
Fox, H., Millington, L., Mahabeer, I., and van Ruiten, H. (2020). Duchenne Muscular Dystrophy. Bmj 368, l7012. doi:10.1136/bmj.l7012
Giliberto, F., Ferreiro, V., Massot, F., Ferrer, M., Francipane, L., and Szijan, I. (2011). Prenatal Diagnosis of Duchenne/Becker Muscular Dystrophy by Short Tandem Repeat Segregation Analysis in Argentine Families. Muscle Nerve 43 (4), 510–517. doi:10.1002/mus.21904
Gilstrop Thompson, M., Corsetti, S., Jain, V., Ruhstaller, K., and Sciscione, A. (2020). Accuracy of Routine Prenatal Genetic Screening in Patients Referred for Genetic Counseling. Am. J. Perinatol. 37 (3), 271–276. doi:10.1055/s-0039-1678533
Iftikhar, M., Frey, J., Shohan, M. J., Malek, S., and Mousa, S. A. (2021). Current and Emerging Therapies for Duchenne Muscular Dystrophy and Spinal Muscular Atrophy. Pharmacol. Ther. 220, 107719. doi:10.1016/j.pharmthera.2020.107719
Kitzman, J. O., Snyder, M. W., Ventura, M., Lewis, A. P., Qiu, R., Simmons, L. E., et al. (2012). Noninvasive Whole-Genome Sequencing of a Human Fetus. Sci. Transl. Med. 4 (137), 137ra176. doi:10.1126/scitranslmed.3004323
Lalic, T., Vossen, R. H. A. M., Coffa, J., Schouten, J. P., Guc-Scekic, M., Radivojevic, D., et al. (2005). Deletion and Duplication Screening in the DMD Gene Using MLPA. Eur. J. Hum. Genet. 13 (11), 1231–1234. doi:10.1038/sj.ejhg.5201465
Ling, C., Dai, Y., Fang, L., Yao, F., Liu, Z., Qiu, Z., et al. (2020). Exonic Rearrangements in DMD in Chinese Han Individuals Affected with Duchenne and Becker Muscular Dystrophies. Hum. Mutat. 41 (3), 668–677. doi:10.1002/humu.23953
Lo, Y. M. D., Corbetta, N., Chamberlain, P. F., Rai, V., Sargent, I. L., Redman, C. W., et al. (1997). Presence of Fetal DNA in Maternal Plasma and Serum. Lancet 350 (9076), 485–487. doi:10.1016/s0140-6736(97)02174-0
Lo, Y. M. D., Chan, K. C. A., Sun, H., Chen, E. Z., Jiang, P., Lun, F. M. F., et al. (2010). Maternal Plasma DNA Sequencing Reveals the Genome-wide Genetic and Mutational Profile of the Fetus. Sci. Transl. Med. 2 (61), 61ra91. doi:10.1126/scitranslmed.3001720
Nobile, C., Galvagni, F., Marchi, J., Roberts, R., and Vitiello, L. (1995). Genomic Organization of the Human Dystrophin Gene across the Major Deletion Hot Spot and the 3′ Region. Genomics 28 (1), 97–100. doi:10.1006/geno.1995.1111
Olshen, A. B., Bengtsson, H., Neuvial, P., Spellman, P. T., Olshen, R. A., and Seshan, V. E. (2011). Parent-specific Copy Number in Paired Tumor-normal Studies Using Circular Binary Segmentation. Bioinformatics 27 (15), 2038–2046. doi:10.1093/bioinformatics/btr329
Parks, M., Court, S., Cleary, S., Clokie, S., Hewitt, J., Williams, D., et al. (2016). Non-invasive Prenatal Diagnosis of Duchenne and Becker Muscular Dystrophies by Relative Haplotype Dosage. Prenat Diagn. 36 (4), 312–320. doi:10.1002/pd.4781
Posch, A., Springer, S., Langer, M., Blaicher, W., Streubel, B., and Schmid, M. (2012). Prenatal Genetic Counseling and Consanguinity. Prenat Diagn. 32 (12), 1133–1138. doi:10.1002/pd.3971
Rabinowitz, T., Polsky, A., Golan, D., Danilevsky, A., Shapira, G., Raff, C., et al. (2019). Bayesian-based Noninvasive Prenatal Diagnosis of Single-Gene Disorders. Genome Res. 29 (3), 428–438. doi:10.1101/gr.235796.118
Salomon, L. J., Sotiriadis, A., Wulff, C. B., Odibo, A., and Akolekar, R. (2019). Risk of Miscarriage Following Amniocentesis or Chorionic Villus Sampling: Systematic Review of Literature and Updated Meta‐analysis. Ultrasound Obstet. Gynecol. 54 (4), 442–451. doi:10.1002/uog.20353
Shashi, V., Golden, W. L., Allinson, P. S., Blanton, S. H., von Kap-Herr, C., and Kelly, T. E. (1996). Molecular Analysis of Recombination in a Family with Duchenne Muscular Dystrophy and a Large Pericentric X Chromosome Inversion. Am. J. Hum. Genet. 58 (6), 1231–1238.
Slomp, C., Morris, E., Inglis, A., Lehman, A., and Austin, J. (2018). Patient Outcomes of Genetic Counseling: Assessing the Impact of Different Approaches to Family History Collection. Clin. Genet. 93 (4), 830–836. doi:10.1111/cge.13176
Talevich, E., Shain, A. H., Botton, T., and Bastian, B. C. (2016). CNVkit: Genome-wide Copy Number Detection and Visualization from Targeted DNA Sequencing. Plos Comput. Biol. 12 (4), e1004873. doi:10.1371/journal.pcbi.1004873
van Deutekom, J. C. T., and van Ommen, G.-J. B. (2003). Advances in Duchenne Muscular Dystrophy Gene Therapy. Nat. Rev. Genet. 4 (10), 774–783. doi:10.1038/nrg1180
Verhaart, I. E. C., and Aartsma-Rus, A. (2019). Therapeutic Developments for Duchenne Muscular Dystrophy. Nat. Rev. Neurol. 15 (7), 373–386. doi:10.1038/s41582-019-0203-3
Walter, M. C., and Reilich, P. (2017). Recent Developments in Duchenne Muscular Dystrophy: Facts and Numbers. J. Cachexia, Sarcopenia Muscle 8 (5), 681–685. doi:10.1002/jcsm.12245
Wang, K., Li, M., and Hakonarson, H. (2010). ANNOVAR: Functional Annotation of Genetic Variants from High-Throughput Sequencing Data. Nucleic Acids Res. 38 (16), e164. doi:10.1093/nar/gkq603
Xu, Y., Li, X., Ge, H.-j., Xiao, B., Zhang, Y.-Y., Ying, X.-M., et al. (2015). Haplotype-based Approach for Noninvasive Prenatal Tests of Duchenne Muscular Dystrophy Using Cell-free Fetal DNA in Maternal Plasma. Genet. Med. 17 (11), 889–896. doi:10.1038/gim.2014.207
Keywords: cell-free DNA, duchenne muscular dystrophy, noninvasive prenatal diagnosis, haplotype, Bayes factor
Citation: Kong L, Li S, Zhao Z, Feng J, Chen G, Liu L, Tang W, Li S, Li F, Han X, Wu D, Zhang H, Sun L and Kong X (2021) Haplotype-Based Noninvasive Prenatal Diagnosis of 21 Families With Duchenne Muscular Dystrophy: Real-World Clinical Data in China. Front. Genet. 12:791856. doi: 10.3389/fgene.2021.791856
Received: 09 October 2021; Accepted: 18 November 2021;
Published: 14 December 2021.
Edited by:
Desheng Liang, Central South University, ChinaReviewed by:
Qifei Li, Boston Children’s Hospital and Harvard Medical School, United StatesCopyright © 2021 Kong, Li, Zhao, Feng, Chen, Liu, Tang, Li, Li, Han, Wu, Zhang, Sun and Kong. This is an open-access article distributed under the terms of the Creative Commons Attribution License (CC BY). The use, distribution or reproduction in other forums is permitted, provided the original author(s) and the copyright owner(s) are credited and that the original publication in this journal is cited, in accordance with accepted academic practice. No use, distribution or reproduction is permitted which does not comply with these terms.
*Correspondence: Xiangdong Kong, a29uZ3hkQHp6dS5lZHUuY24=; Luming Sun, THVtaW5nX3N1bkAxNjMuY29t; Haichuan Zhang, aHpoYW5nQG5lb3NlcWx0ZC5jb20=; Di Wu, amFtZXMud3VAY2VsdWxhLWNoaW5hLmNvbQ==
†These authors share first authorship
‡These authors share senior authorship
Disclaimer: All claims expressed in this article are solely those of the authors and do not necessarily represent those of their affiliated organizations, or those of the publisher, the editors and the reviewers. Any product that may be evaluated in this article or claim that may be made by its manufacturer is not guaranteed or endorsed by the publisher.
Research integrity at Frontiers
Learn more about the work of our research integrity team to safeguard the quality of each article we publish.