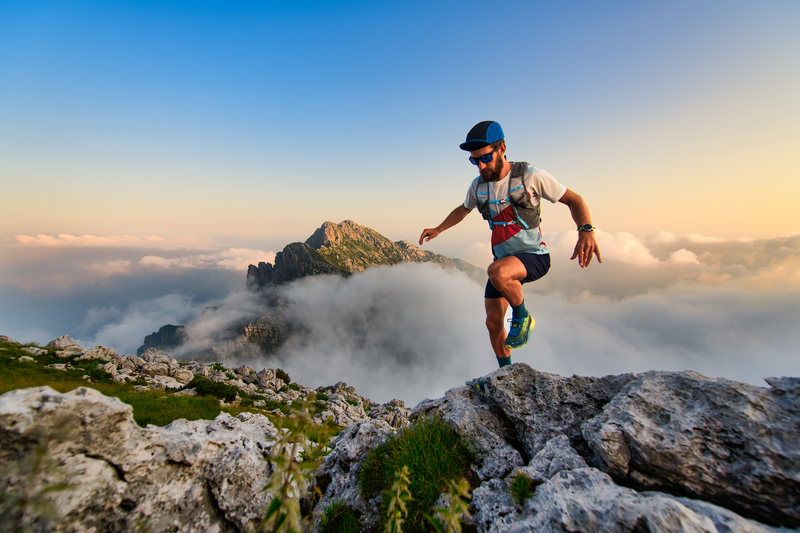
94% of researchers rate our articles as excellent or good
Learn more about the work of our research integrity team to safeguard the quality of each article we publish.
Find out more
ORIGINAL RESEARCH article
Front. Genet. , 23 December 2021
Sec. Computational Genomics
Volume 12 - 2021 | https://doi.org/10.3389/fgene.2021.784775
Pan-cancer strategy, an integrative analysis of different cancer types, can be used to explain oncogenesis and identify biomarkers using a larger statistical power and robustness. Fine-mapping defines the casual loci, whereas genome-wide association studies (GWASs) typically identify thousands of cancer-related loci and not necessarily have a fine-mapping component. In this study, we develop a novel strategy to identify the causal loci using a pan-cancer and fine-mapping assumption, constructing the CAusal Pan-cancER gene (CAPER) score and validating its performance using internal and external validation on 1,287 individuals and 985 cell lines. Summary statistics of 15 cancer types were used to define 54 causal loci in 15 potential genes. Using the Cancer Genome Atlas (TCGA) training set, we constructed the CAPER score and divided cancer patients into two groups. Using the three validation sets, we found that 19 cancer-related variables were statistically significant between the two CAPER score groups and that 81 drugs had significantly different drug sensitivity between the two CAPER score groups. We hope that our strategies for selecting causal genes and for constructing CAPER score would provide valuable clues for guiding the management of different types of cancers.
Cancer is a major cause of mortality in both developed and developing countries, resulting in more than 8 million deaths each year worldwide (Tarver, 2012; Bray et al., 2013; Rodriguez-Martin et al., 2020). Since the causal factors and regulatory mechanisms are complex and remain largely unknown, there could be an increasing trend of morbidity and mortality attributed to cancer in the future (Nakagawa and Fujita, 2018). Based on multi-omics technologies, it has been demonstrated that cancers in different tissues and organs may share common features, whereas those in the same or similar organ may have distinct characteristics (Ciriello et al., 2013; Kandoth et al., 2013; Peng et al., 2021). These findings indicate that a pan-cancer strategy, which takes into account commonalities across cancer types, can be used to identify molecular abnormalities that transcend particular lineages, may explain oncogenesis, and make a large contribution towards the personal management of cancer (Vargas and Harris, 2016). In addition, pan-cancer analysis improves the statistical power used to identify cancer-related molecular dysregulation and avoids poor reproducibility in the characterization of rare subtypes (Priestley et al., 2019). Programs, such as the Cancer Genome Atlas (TCGA), which coordinate multi-omics sequencing and the clinical annotation of approximately 10,000 samples across over 30 cancer types, provide a great opportunity to identify pan-cancer biomarkers (Chang et al., 2013; Nawy, 2018).
Although genome-wide association studies (GWASs) have identified thousands of cancer-related loci (De Los Campos et al., 2018), there are still some unsolved issues. First, the majority of GWAS have identified variants located in non-coding regions and with small effect sizes, making it difficult to interpret functional and biological mechanisms that underlie the associations (Maurano et al., 2012; Visscher et al., 2017; Zeng et al., 2021). Second, complex linkage disequilibrium (LD) may obscure causal variants that drive the associations. Therefore, significant associations identified by GWASs are more about disease-related genomic regions than individual variants (Gallagher and Chen-Plotkin, 2018; Tam et al., 2019). Third, the most statistically significant variants may not be causal.
In general, genetic variants cause complex diseases by regulating gene expression, the abundance of one or multiple downstream proteins (Lappalainen et al., 2013; Westra et al., 2013; Albert and Kruglyak, 2015; Gusev et al., 2016). Gene pathway analysis and enrichment analysis have been widely applied to explore potential cancer-related mechanisms and have supplied plenty of valuable clues for the development of intervention targets (Shukla et al., 2016; Bao et al., 2019; Demircioğlu et al., 2019; Peng et al., 2019; Kim et al., 2020). Although previous studies have also leveraged data from TCGA to identify specific genes and signaling pathways involved in oncogenesis and development from a pan-cancer perspective (Ballot et al., 2020; Frost et al., 2020; Liu et al., 2020), these candidate genes or pathway-specific strategies are all based on prior knowledge, resulting in the loss of potential causal associations (Deng et al., 2014; Liu et al., 2014; Zhang et al., 2018). In addition, many of the associated genes identified may be the outcome rather than the cause of the disease (Gusev et al., 2016). Consequently, transcriptome-wide association studies (TWASs) were proposed to integrate GWASs with expression quantitative trait locus (eQTL) reference panels constructed from external genome-wide gene expression and genotype data to identify predicted gene–trait associations (Xu et al., 2017; Barbeira et al., 2018). Since a large sample size is used, the performance and statistical power of a TWAS is superior to that of traditional transcriptome analysis (Gusev et al., 2016; Xu et al., 2017; Barbeira et al., 2018). However, TWASs tend to identify multiple significant genes per region but fail to define the causal gene due to LD confounding (Mancuso et al., 2019; Wainberg et al., 2019; Wu and Pan, 2020). To overcome this drawback, fine-mapping methods were used to identify causal variants responsible for complex traits by accounting for the patterns of LD among the SNPs within a region associated with the target disease and assuming that at least one causal variant exists (Schaid et al., 2018).
In this study, we developed a novel strategy to define causal genes with the assumption of pan-cancer, to construct the CAusal Pan-cancER gene (CAPER) score, and to validate its performance using internal and external validation (Figure 1). Based on the workflow, we identified causal genes related to multiple cancers, used the same gene panel to differentiate cancer patients, and validated the efficiency of the gene panel in external validation sets (Li et al., 2021).
We obtained 15 GWAS summary statistics of common cancers, including bladder cancer (BLCA, Prev. = 23.0/100,000), breast cancer (BRCA, Prev. = 125.2/100,000), cervical squamous cell carcinoma and endocervical adenocarcinoma (CESC, Prev. = 12.4/100,000), colorectal cancer (COADREAD, Prev. = 55.9/100,000), esophageal or stomach adenocarcinoma (ESCASTAD, Prev. = 15.1/100,000), kidney cancer (KC, Prev. = 14.5/100,000), lung cancer (LC, Prev. = 35.7/100,000), lymphocytic leukemia (LL, Prev. = 10.1/100,000), melanoma (MM, Prev. = 18.3/100,000), oral cavity and pharyngeal cancer (OCPC, Prev. = 3.3/100,000), ovarian cancer (OV, Prev. = 13.5/100,000), pancreatic adenocarcinoma (PAAD, Prev. = 7.9/100,000), prostate cancer (PRAD, Prev. = 120.1/100,000), thyroid carcinoma (THCA, Prev. = 10.2/100,000), and uterine corpus endometrial cancer (UCEC, Prev. = 29.4/100,000) (Li et al., 2012; Leo et al., 2017; Phelan et al., 2017; O’Mara et al., 2018; Schumacher et al., 2018; Zhou et al., 2018; Rashkin et al., 2020). The average number of SNPs was 1,144,365 (median = 1,293,959, ranging from 269,795 to 1,298,901) and the average sample size was 273,861 (median = 399,215, ranging from 7,785 to 417,127). In addition, we used Linkage Disequilibrium SCore regression (LDSC) (Bulik-Sullivan et al., 2015) to estimate the observed and liability heritability of each cancer and the genetic correlation between each pair of cancer types. We identified 28 significant pairs of cancer types (p < 0.05), among which OV and UCEC presented the lowest p-value (p = 3.69E-7) (Supplementary Figure S1). Since all the GWASs had been performed on patients with European ancestry, we used the LD scores of the European population of the 1000 Genome Project (1 KGP) to estimate the heritability of each cancer (Consortium, 2015). Details on the 15 summary statistics are provided in Table 1.
Furthermore, we used the GENCODE database (v25) to map the SNPs to gene positions. In total, we retained 19,201 protein-coding genes selected from 60,252 transcripts in autosomes. Then, the SNPs located 100 kb upstream and downstream of a specific gene were selected to perform fine-mapping. After mapping to the gene and intersecting using the reference panel, 1,403,668 SNPs remained. We used PLINK (v1.9b6.22) to estimate the LD matrix of each gene.
We used SuSiE, a fine-mapping method, to identify the causal SNPs in the 15 GWAS summary datasets with the aid of the susieR package (v0.11.42) (Wang et al., 2020a) in R software. We also used 1 KGP EUR samples as the LD reference panel. According to SuSiE manual, we set the maximum number of causal variants in the region to 10. Based on the results of previous studies (Fadista et al., 2016; Schaid et al., 2018), we set the significant level to 1E-5. A specific gene with causal SNPs was defined as a potential causal gene. We repeated the fine-mapping procedure for each type of cancer. Finally, the potential causal gene set consisted of genes regarded as casual genes of at least three types of cancers.
We also used the Molecular Signatures Database (MSD) to evaluate the overlapping of our candidate genes with regard to common processes, pathways, and underlying biological themes, while considering an FDR q-value of less than 0.05 and a minimum gene set size of two as statistically significant (Subramanian et al., 2005; Liberzon et al., 2011; Liberzon et al., 2015).
To verify the clinical translation of the potential causal gene set, we downloaded two types of TCGA data: (1) molecular data, which included gene expression (HTSeq-FPKM) (log2(FPKM+1)) and DNA methylation data; and (2) clinical data, which included age, sex, and survival time. These data were downloaded from the University of California Santa Cruz (UCSC) Xena browser (Goldman et al., 2018). We used data on all 11,057 samples and 60,483 transcripts available on 33 different types of cancer. We transformed the Ensembl IDs to symbols, using the biomaRt package (v.2.46.3) (Durinck et al., 2009). Specifically, we used the average level to represent the gene with multiple Ensembl ID mapping with a single symbol. We obtained the sum of the genes from the 33 datasets and calculated the average expression level for genes that contained more than one transcript. We also filtered out the samples that were (1) non-European ancestry and (2) missing tumor stage data. Genes that were not expressed (FPKM = 0) in more than 50% of samples were excluded from the expression data of our causal gene set. After quality control, we obtained 38,596 genes, including 12 CAPER genes, and 4,842 individuals with 21 different types of cancer.
First, we divided the TCGA sample into two parts: the training set (80%), used to construct the CAPER score, and the test set (20%), used to perform internal validation. Of the 4,842 individuals, 3,873 were allocated to the training set and 969 were allocated to the test set (Supplementary Table S1). The 21 types of cancer included were adrenocortical carcinoma (ACC), BLCA, BRCA, cholangiocarcinoma (CHOL), colon adenocarcinoma (COAD), esophageal carcinoma (ESCA), head and neck squamous cell carcinoma (HNSC), kidney chromophobe (KICH), kidney renal clear cell carcinoma (KIRC), kidney renal papillary cell carcinoma (KIRP), liver hepatocellular carcinoma (LIHC), lung adenocarcinoma (LUAD), lung squamous cell carcinoma (LUSC), mesothelioma (MESO), PAAD, rectum adenocarcinoma (READ), skin cutaneous melanoma (SKCM), stomach adenocarcinoma (STAD), testicular germ cell tumors (TGCT), THCA, and uveal melanoma (UVM). The training set was used to perform Cox regression on each gene after adjusting for age, sex, and tumor stage. We used the survival package (v3.2–11) to fit the Cox regression data, and the survminer package (v0.4.9) was used to perform survival analysis and visualization. We obtained the association between each gene and the disease-specific overall survival rate.
Second, we used a causal gene set to fit the multivariable Cox regression adjusted for age, sex, and tumor stage. The model used was as follows:
where
In addition, we used the TCGA test set to perform three types of sensitivity analyses. (1) To show the performance of the CAPER score for a shorter survival time, we selected all samples with survival <5 years. (2) To show the performance of the CAPER score in a smaller sample size, we randomly selected 80 samples. (3) To show the effectiveness and accuracy of the CAPER score, we randomly selected 12 genes, namely, DGFRL, GLRX5, KCNJ14, SMARCAL1, FTH1P16, CDK5, WDFY1, TMEM266, RAD21, NAA16, AGPS, and FBXO39, to construct the random CAPER score. We used the log-rank test for all three analyses.
We performed a series of analyses to investigate the clinical translation of the CAPER score, using the TCGA test set, IMvigor210 cohort, and Genomics of Drug Sensitivity in Cancer (GDSC) (Yang et al., 2012; Tomczak et al., 2015; Mariathasan et al., 2018).
First, using the TCGA test set, we defined the association between CAPER score and TNM staging, tumor histological grade, and vascular tumor cell types. Specifically, the pathological stages of the primary tumor (T) were divided into two groups: (1) Tis (tumor in situ) and T1, and (2) T2 or larger. The staging of distant metastasis (M) is defined as M0 and M1. In addition, we detected the association between the CAPER score and immune subtypes, including C1 (wound healing), C2 (IFN-γ dominant), C3 (inflammatory), C4 (lymphocyte depleted), C5 (immunologically quiet), and C6 (TGF-β dominant), which were filtered because the sample size was below 40. These immune subtypes were proven to be associated with prognosis, genetic, and immune-modulatory alterations. These factors may shape the specific types of immune environments that we observed and indicate response to therapy or prognosis (Thorsson et al., 2018). We also showed the association between the high-CAPER group and the low-CAPER group, as well as the immune subtype of each gene.
Second, we used the TCGA test set to define the association between the CAPER score and the tumor microenvironment. On the one hand, we estimated the immune score, stromal score, and tumor purity using the limma (v3.46.0) (Ritchie et al., 2015) and the estimate (v1.0.13) packages (Yoshihara et al., 2013). ESTIMATE is a method that uses gene expression signatures to infer the fraction of stromal and immune cells in the tumor samples. We estimated the correlation between the CAPER score, the three metrics, and identified differences between the two scores and tumor purity of the high- and low-CAPER group. After filtering out data on 3 cancer types (CHOL, KICH, and UVM) with a sample size below 10 (low sample size cancers), we also estimated the Spearman correlation between single CAPER gene expression and three metrics in each cancer. On the other hand, we used the support vector regression (SVR) on CIBERSORTx (Steen et al., 2020) to deconvolve RNA admixtures to the abundance of 22 types of immune cells in TCGA samples to further observe the tumor microenvironment (TME) (Chen et al., 2018). Following the CIBERSORTx manual, we set the number of permutations to 100. We uploaded RNA-seq FPKM data and set quantile normalization to discern the recommended setting (Craven et al., 2021). We filtered out immune cell types with an average proportion lower than 2%, and 14 types of immune cells were included into the final analysis. We identified differences in the immune cell abundance between the high- and low-CAPER groups. After filtering out 3 types of cancers with a small sample size, we estimated the Spearman correlation between the CAPER score and the abundance of 14 immune cells in 18 types of cancers.
Third, we used the stemness score based on DNA methylation (DNAss) and RNA expression (RNAss) obtained from UCSC. The stemness score is defined as the quantification of stemness and is associated with tumor progression, therapeutic resistance, and recurrence. DNAss indicates epigenetic features while RNAss indicates gene expression (Malta et al., 2018; Pei et al., 2020). We estimated the Spearman correlation between single CAPER gene expression and the stemness score in 18 types of cancer. For the CAPER score, we estimated the Spearman correlation between the CAPER score and the stemness score and conducted a Wilcoxon rank-sum test to compare the statistical significance of the stemness score between high- and low-CAPER groups.
Fourth, we used the IMvigor210 cohort to verify the robustness and efficiency of the CAPER score. Data were downloaded using the Imvigor210CoreBiologies package (v1.0.0). The cohort data included immune phenotypes (immune inflamed, immune excluded, and immune desert), Lund molecular subtypes, IC-Level (level of immunohistochemistry-assessed PD-L1 staining on immune cells), and TC-Level (level of immunohistochemistry-assessed PD-L1 staining on tumor cells). We used the coefficient estimated using the training set in TCGA to construct the CAPER score for the Imvigor210 cohort and investigated the difference in variables in the high- and low-CAPER groups.
Finally, using GDSC, the largest free public database of information on drug sensitivity in cancer cells and molecular markers of drug response (Yang et al., 2012), we identified the association between the CAPER score and drug sensitivity. We constructed the CAPER score for each cell line sample and conducted a Spearman correlation analysis between the CAPER score and IC50 value, the half maximal inhibitory concentration, which is an established measurement of drug efficacy (Aykul and Martinez-Hackert, 2016).
Using SuSiE (Wang et al., 2020a), we identified 54 causal SNPs in 15 genes (ATP6V1G2, ATP6V1G2-DDX39B, CLPTM1L, DDX39B, MCCD1, MICA, MICB, MYC, MYEOV, NFKBIL1, POU5F1B, SLC6A18, SLC6A19, SLC6A3, and TERT). Detailed information of the causal SNPs and potential causal genes is shown in Table 2 and Figure 2, and Supplementary Table S2. MDB showed that 13 were significantly enriched in breast tumor, hepatocellular carcinoma, substance transport-related, and certain other pathways, while 12 were located in the cytogenic region of 6p21 or 5p15 (FDR q-value < 0.05, Supplementary Table S3).
FIGURE 2. Summary of the CAPER genes. (A) Ideogram of the 15 CAPER genes (the color of each chromosome indicates gene density across the human genome). (B) The bubble plot shows the minimum p-value of each causal gene in each cancer dataset.
The training data of TCGA was used to select 12 CAPER genes, investigate their pan-cancer association, and construct the CAPER score. Through univariate Cox regression, we defined 6 significant causal genes with an average p-value of 0.01 (median = 1.87E-06, ranging from 4.62E-09 to 0.04) by adjusting for age, sex, and tumor size. For example, from among the 12 CAPER genes, MYEOV was the gene with the greatest risk (HR = 1.09, 95% CI: 1.06–1.13), while ATP6V1G2 was the gene that offered the largest protection effect (HR = 0.68, 95% CI: 0.59–0.78). Details of the univariate analysis are presented in Table 3 and Figure 3A.
TABLE 3. Summary of the univariable Cox regression analysis conducted on 12 CAPER genes in the TCGA training set *.
FIGURE 3. Summary of the CAPER score. (A) Heatmap showing the p-value of each CAPER gene in the univariate Cox regression performed on each type of cancer. (B) ROC of the multivariate Cox regression conducted on the TCGA test set. We estimated the AUC of 1-year (green), 3-year (Red), and 5-year (Blue) survival. (C) K-M curve of the high- and low-CAPER score groups. The HR of the CAPER score was statistically significant to the survival time with a p-value of <0.001 and was adjusted for age, sex, and tumor stage.
Furthermore, we performed a multivariate Cox regression to construct the CAPER score using the TCGA training set (Table 4). We estimated the time-dependent ROC curves for multivariate Cox regression for 1-, 3-, and 5-year survival (AUC = 0.704, 0.717, and 0.710, respectively) using TCGA test data (Figure 3B). The time-dependent ROC curves in the TCGA training set are provided in Supplementary Figure S2. The formula used to construct the CAPER score is as follows:
TABLE 4. Summary of the multivariable Cox regression conducted on 12 CAPER genes in the TCGA training set *.
The HR for the CAPER score is 3.75 (95% CI: 2.65–5.32). In addition, Kaplan–Meier curves were used to detect differences in survival between the high- and low-CAPER groups, which was found to be significant (log-rank test, p < 0.0001), with the median survival time of the high-CAPER group being shorter (Figure 3C).
In addition, sensitivity analyses indicated the effective construction procedure and accurate prognosis performance. When we used 5 years as the cutoff, the difference in survival between high- and low-CAPER groups was found to be significant (log-rank test; p < 0.0001) (Supplementary Figure S3A). When a small sample size was used (i.e., 80 individuals), the difference in survival between the high- and low-CAPER groups was also significant (log-rank test; p = 0.011) (Supplementary Figure S3B). A random gene set was used, and although three genes showed significant differences, the difference between high- and low-CAPER groups was not significant (log-rank test, p = 0.54) (Supplementary Figure S4 and Supplementary Table S4).
TCGA test data were used to explore the clinical translation of the CAPER score and its potential application for therapeutic and prognostic purposes in cancer management. We used the CAPER score to classify patients into high- and low-CAPER groups and tested differences in clinical metrics, TME, and the stemness score between the two groups.
First, we analyzed the association between CAPER score and immune subtypes and TNM stage (Figure 4 and Table 5). The Kruskal–Wallis test found the difference in each immune subtype to be significant (
FIGURE 4. Summary of the differences between the three tumor-related variables in the different CAPER groups using the TCGA test set. (A) immune subtypes; (B) the pathological stage of the primary tumor (T); (C) the pathological stage of the lymph nodes (N).
TABLE 5. Summary of the association between clinical categorical variables and the CAPER score in TCGA.
FIGURE 5. Summary of the correlation between TME and CAPER score or CAPER gene expression using the TCGA test set. (A) Differences in the immune score between the CAPER score groups. (B) Differences in the stromal score between the CAPER score groups. (C) Differences in tumor purity between the CAPER score groups. (D) The bubble plot shows the p-value of Spearman correlation tests conducted on 18 types of cancer. (E) The boxplot cellular abundance differential score between the high- and low-CAPER score groups (*p < 0.05, **p < 0.01, and ***p < 0.001). (F) The autocorrelation plot of cellular abundance.
Finally, we analyzed the correlation between the CAPER score and the stemness score constructed using gene expression and DNA methylation data (Supplementary Figure S7). The RNA stemness score (Spearman correlation test, ρ = 0.29, p < 2.2E-16) and the DNA stemness score were both positively correlated to the CAPER score (Spearman correlation test, ρ = 0.32, p < 2.2E-16). The high-CAPER group had a higher DNA (Wilcoxon rank-sum test, p = 6.9E-15) and RNA (Wilcoxon rank-sum test, p = 1.5E-13) stemness score, indicating stronger tumor stem cell activity and a lower degree of tumor differentiation.
The IMvigor210 cohort was analyzed to verify the robustness and efficiency of the CAPER score; we observed that the CAPER score was significantly associated with the three types of clinical metrics in IMvigor210 (Table 6 and Figure 6). For the clinical phenotypes, differences in the CAPER score between patients with different TC levels were statistically significant (Kruskal–Wallis test, p = 5.2E-05). The CAPER score of TC2+ patients was higher than that of TC0 patients (Wilcoxon rank-sum test, p = 1.1E-05). The differences in CAPER score between patients with different IC levels were statistically significant (Kruskal–Wallis test, p = 0.015). The CAPER score of IC2+ patients was higher than that in IC0 patients (Wilcoxon rank-sum test, p = 3.9E-03). In addition, we analyzed CAPER score differences between immune phenotypes using IMvigor210. The differences in CAPER score between patients with different immune phenotypes were statistically significant (Kruskal–Wallis test, p = 0.003). We observed that the “immune inflamed” type had a higher CAPER score than the “desert” type (Wilcoxon rank-sum test, p = 1.2E-03). The difference in Lund molecular subtypes was significant in different CAPER groups (Kruskal–Wallis test, p = 3.2E-14). The CAPER score of the “Basal/SCC-like” type was higher than that of the “Infiltrated” (Wilcoxon rank-sum test, p = 1.5E-11), and “UroA” (Wilcoxon rank-sum test, p = 7.2E-13) types. As expected, differences between the high- and low-CAPER groups were also significant for the three tumor immunity-related variables indicated above (TC levels, p = 2.53E-03, immune subtypes, p = 0.01, and Lund molecular subtypes, p = 7.28E-09, respectively).
TABLE 6. Summary of the association between four tumor immunity-related variables and CAPER score in the IMvigor210 cohort.
FIGURE 6. Summary of the differences between the four tumor phenotypes in different CAPER groups using IMvigor210. (A) TC levels; (B) IC levels; (C) immune phenotypes; (D) Lund molecular subtypes.
We analyzed the correlation between the CAPER score and drug sensitivity in the GDSC using Spearman correlation and identified 81 significantly correlated drugs between the CAPER score and drug sensitivity (Supplementary Table S5). For example, the CAPER score was negatively correlated with the sensitivity of Afatinib (ρ = −0.28, p = 6.71E-17), Trametinib (ρ = −0.23, p = 2.12E-12), Selumetinib (ρ = −0.22, p = 1.36E-11), and Refametinib (ρ = −0.21, p = 2.22E-10), which indicated a lower CAPER score against their higher level of drug sensitivity. The CAPER score was positively correlated to the sensitivity of Axitinib (ρ = 0.14, p = 2.56E-05), SB52334 (ρ = 0.13, p = 5.61E-05), GSK269962A (ρ = 0.12, p = 4.2E-04), and Quizartinib (ρ = 0.10, p = 3.0E-03). Furthermore, we found that drugs with sensitivity that was negatively correlated with the CAPER score mostly targeted EGFR signaling and the ERK MAPK signaling pathway, while drugs with sensitivity that was positively correlated with the CAPER score mostly targeted RTK signaling and cytoskeleton pathways.
In our study, using GWAS summary statistics of 15 cancers and the SuSiE package, we identified causal SNPs and 12 CAPER genes. SuSiE is a newly developed approach for genetic fine-mapping that quantifies the uncertainty of causal variables (Wang et al., 2020a). Compared with existing fine-mapping methods, such as CAVIAR (Hormozdiari et al., 2014), FINEMAP (Benner et al., 2016), and DAP-G (Wen et al., 2016; Lee et al., 2018), SuSiE has been demonstrated to have a fair degree of accuracy for computing PIPs and a much higher computation speed and higher power in distinguishing between causal variables and non-causal variables (Wang et al., 2020a). Then, we used the expression level of the CAPER genes in the TCGA training set to construct the CAPER score. We performed internal and external validation of the CAPER score using three validation sets, including the TCGA test set, IMvigor210, and GDSC. The results demonstrated the potential application of the CAPER score for therapeutic and prognostic purposes in cancer management.
Among the 54 causal SNPs obtained through fine-mapping, 29 were shown to have a significant eQTL effect on 8 potential causal genes across different tissues. A total of 324 eQTL pairs were extracted from GTEx and are listed in Supplementary Table S6. As expected, the association between all 12 CAPER genes and cancers have been demonstrated using a series of basic experimental and population-based studies. For example, ATP6V1G2 is a ferroptosis-related gene that plays a primary role in metabolism and oxidative stress and is defined as a colorectal cancer prognosis biomarker (Huang et al., 2021; Shao et al., 2021). DDX39B is involved in the regulation of pre-mRNA splicing, nuclear export of mRNAs, and pre-ribosomal RNA translation, and may promote the genesis, development, and metastasis of multiple cancer types by regulating cell proliferation (Awasthi et al., 2018; Gu et al., 2020; Xu et al., 2020; He et al., 2021). MYC and MYEOV are two well-known oncogenes (Specht et al., 2004; Paglia et al., 2020). MYC can contribute to oncogenesis and immune evasion through various mechanisms, including the promotion of autonomous cell growth and proliferation, modulation of tumor–stroma interactions, and regulation of the host immune system (Dang, 2012; Paglia et al., 2020; Dhanasekaran et al., 2021; Lourenco et al., 2021), while the mechanism of action that underlies the function of MYEOV in cancer development and metastasis may enhance SOX9 transcriptional activity (Lawlor et al., 2010; Fang et al., 2019; Liang et al., 2020). Thus, both MYC and MYEOV have also been identified as potential immunotherapy targets (Fang et al., 2019; Duffy et al., 2021). POU5F1B can promote cancer oncogenesis by cooperating with MYC and is associated with poor prognosis in pancreatic ductal adenocarcinoma patients (Hayashi et al., 2015; Amantini et al., 2019). It may also promote HCC proliferation by activating AKT (Pan et al., 2018). SLC6A19 has also been reported to be a potential biomarker that has a significantly low level of expression in patients with renal cancer (Zamora-Fuentes et al., 2020). In addition, a series of genetic variants in MICA, MICB, NFKBIL1, SLC6A3, CLPTM1L, and TERT have been found to be associated with the susceptibility and prognosis of different cancer types (Rafnar et al., 2009; Wang et al., 2009; Turnbull et al., 2010; Miki et al., 2011; Baek et al., 2018; Toledo-Stuardo et al., 2021). Meanwhile, using summary statistics of GETx data, we performed a colocalization analysis using the coloc package (v.5.1.0) (Giambartolomei et al., 2014). Using default settings, we found that the posterior probabilities of hypothesis 4 (PPH4: both the expression of CLPTM1L and MM are associated and share a single causal variant) are 0.986 and 0.985 for skin exposed to the Sun (lower leg) and skin not exposed to the Sun (suprapubic), respectively, while rs31490 had the largest PPH4 values of 0.541 and 0.624, respectively.
However, some well-known cancer-related genes, such as TP53, are not among the CAPER genes. For example, all108 SNPs of TP53 were not significant in the 15 cancer summary statistics of the 15 types of cancer. There may be two probable reasons for this. First, the dysfunction of familiar genes may not have been caused by genetic variation. For example, the methylation of TP53 has been regarded as a causal factor of leukemia (Saeed et al., 2019). Second, the limitation of sample size in the GWASs and its weak signal may result in SNPs from which common genes have been filtered out.
Furthermore, to verify the robustness and efficiency of the CAPER score, we applied it to TCGA test samples and the IMvigor210 cohort. As expected, patients in the high-risk group tended to have shorter survival and a worse TN stage, which together indicate a poor prognosis. The results also showed that samples in the high-risk group had a higher degree of immune infiltration and a lower differentiation ability, with a higher immune score and stemness score. The stemness score usually indicates the differentiation potential, and a loss of a differentiation ability and gain of stem-cell-like were reported to be the main signs of tumor progression (Seguin et al., 2015; Prasetyanti and Medema, 2017; Zhang et al., 2020); while immune infiltration was also reported to be correlated with the malignancy and prognosis of different types of cancer (Wang et al., 2020b; Wu et al., 2020; Zhang et al., 2020). In addition to the degree of infiltration, the composition of the infiltrating immune cell types in the high-risk and low-risk groups were also different, indicating a more complex difference in the tumor microenvironment between the two groups. Taken together, validation using the TCGA test samples and the IMvigor210 cohort indicated consistency of the CAPER score with clinical prognosis, proving its value in clinical translation.
Interestingly, some associations between genes and certain cancer types were not taken into account during the selection procedure but were detected during the validation procedure. For example, MICB is defined as the casual gene of CESC, PRAD, and UCEC (Table 2), but showed a high correlation with the tumor purity in four cancer types (KIRC, KIPP, LUAD, and MESO), which were not included in the selection step (Figures 5A,E). In addition, while THCA was not included in the selection step, its stemness showed a high level of correlation with the expression of MICA, MYEOV, POU5F1B, and TRET (Supplementary Figures S7A,C). This indicates the potential value of a pan-cancer analysis to identify novel associations.
In addition, we also found a link between the CAPER score and drug sensitivity using the GDSC database, which indicated the potentially extensible application of the CAPER score for the therapeutic and prognostic management of cancer. In particular, 81 drugs were found to be significantly correlated with the CAPER score, among which 67 showed a negative correlation with CAPER score, while only 16 showed higher sensitivity in the group with high CAPER score, indicating a limited selection of drugs available for the high-risk group.
In summary, we developed a CAPER score using a novel strategy based on fine-mapping. An extensive validation procedure was followed to confirm the robustness and efficiency of the CAPER score. Considering its potential usage in prognosis prediction and the identification of novel associations, we expect that this score may provide valuable information that can be used to better understand oncogenesis to guide management from a pan-cancer perspective.
The original contributions presented in the study are included in the article/Supplementary Material. Further inquiries can be directed to the corresponding author.
SY designed the study. ST and ZP performed the datasets’ quality control. ST and XY performed the data analysis. ST, XY, LP, and MF interpreted the analysis results. XY, ST, and LP wrote the draft manuscript. SY and PH revised the article. All authors accepted the final manuscript.
This research was funded by Nanjing Medical University (https://www.njmu.edu.cn/).
The authors declare that the research was conducted in the absence of any commercial or financial relationships that could be construed as a potential conflict of interest.
All claims expressed in this article are solely those of the authors and do not necessarily represent those of their affiliated organizations, or those of the publisher, the editors, and the reviewers. Any product that may be evaluated in this article, or claim that may be made by its manufacturer, is not guaranteed or endorsed by the publisher.
We acknowledge the participants and investigators of GWAS-ALTAS and GWAS-Catalog for making the summary data publicly available for us.
The Supplementary Material for this article can be found online at: https://www.frontiersin.org/articles/10.3389/fgene.2021.784775/full#supplementary-material
Albert, F. W., and Kruglyak, L. (2015). The Role of Regulatory Variation in Complex Traits and Disease. Nat. Rev. Genet. 16, 197–212. doi:10.1038/nrg3891
Amantini, C., Morelli, M. B., Nabissi, M., Piva, F., Marinelli, O., Maggi, F., et al. (2019). Expression Profiling of Circulating Tumor Cells in Pancreatic Ductal Adenocarcinoma Patients: Biomarkers Predicting Overall Survival. Front. Oncol. 9, 874. doi:10.3389/fonc.2019.00874
Awasthi, S., Chakrapani, B., Mahesh, A., Chavali, P. L., Chavali, S., and Dhayalan, A. (2018). DDX39B Promotes Translation through Regulation of Pre-ribosomal RNA Levels. RNA Biol. 15, 1157–1166. doi:10.1080/15476286.2018.1517011
Aykul, S., and Martinez-Hackert, E. (2016). Determination of Half-Maximal Inhibitory Concentration Using Biosensor-Based Protein Interaction Analysis. Anal. Biochem. 508, 97–103. doi:10.1016/j.ab.2016.06.025
Baek, I.-C., Shin, D.-H., Choi, E.-J., Kim, H.-J., Yoon, J.-H., Cho, B.-S., et al. (2018). Association of MICA and MICB Polymorphisms with the Susceptibility of Leukemia in Korean Patients. Blood Cancer J. 8, 58. doi:10.1038/s41408-018-0092-5
Ballot, E., Ladoire, S., Routy, B., Truntzer, C., and Ghiringhelli, F. (2020). Tumor Infiltrating Lymphocytes Signature as a New Pan-Cancer Predictive Biomarker of Anti PD-1/pd-L1 Efficacy. Cancers 12, 2418. doi:10.3390/cancers12092418
Bao, Y., Wang, L., Shi, L., Yun, F., Liu, X., Chen, Y., et al. (2019). Transcriptome Profiling Revealed Multiple Genes and ECM-Receptor Interaction Pathways that May Be Associated with Breast Cancer. Cell Mol Biol Lett 24, 38. doi:10.1186/s11658-019-0162-0
Barbeira, A. N., Dickinson, S. P., Dickinson, S. P., Bonazzola, R., Zheng, J., Wheeler, H. E., et al. (2018). Exploring the Phenotypic Consequences of Tissue Specific Gene Expression Variation Inferred from GWAS Summary Statistics. Nat. Commun. 9, 1825. doi:10.1038/s41467-018-03621-1
Benner, C., Spencer, C. C. A., Havulinna, A. S., Salomaa, V., Ripatti, S., and Pirinen, M. (2016). FINEMAP: Efficient Variable Selection Using Summary Data from Genome-wide Association Studies. Bioinformatics 32, 1493–1501. doi:10.1093/bioinformatics/btw018
Bray, F., Ren, J.-S., Masuyer, E., and Ferlay, J. (2013). Global Estimates of Cancer Prevalence for 27 Sites in the Adult Population in 2008. Int. J. Cancer 132, 1133–1145. doi:10.1002/ijc.27711
Bulik-Sullivan, B. K., Loh, P.-R., Loh, P.-R., Finucane, H. K., Ripke, S., Yang, J., et al. (2015). LD Score Regression Distinguishes Confounding from Polygenicity in Genome-wide Association Studies. Nat. Genet. 47, 291–295. doi:10.1038/ng.3211
Chang, K., Weinstein, J. N., Collisson, E. A., Mills, G. B., Shaw, K. R., Ozenberger, B. A., et al. (2013). The Cancer Genome Atlas Pan-Cancer Analysis Project. Nat. Genet. 45, 1113–1120. doi:10.1038/ng.2764
Chen, B., Khodadoust, M. S., Liu, C. L., Newman, A. M., and Alizadeh, A. A. (2018). Profiling Tumor Infiltrating Immune Cells with CIBERSORT. Methods Mol. Biol. (Clifton, NJ) 1711, 243–259. doi:10.1007/978-1-4939-7493-1_12
Ciriello, G., Miller, M. L., Aksoy, B. A., Senbabaoglu, Y., Schultz, N., and Sander, C. (2013). Emerging Landscape of Oncogenic Signatures across Human Cancers. Nat. Genet. 45, 1127–1133. doi:10.1038/ng.2762
Consortium, G. P., Auton, A., Brooks, L. D., Durbin, R. M., Garrison, E. P., Kang, H. M., et al. (2015). A Global Reference for Human Genetic Variation. Nature 526, 68–74. doi:10.1038/nature15393
Craven, K. E., Gökmen-Polar, Y., and Badve, S. S. (2021). CIBERSORT Analysis of TCGA and METABRIC Identifies Subgroups with Better Outcomes in Triple Negative Breast Cancer. Sci. Rep. 11 (1), 1–19. doi:10.1038/s41598-021-83913-7
De Los Campos, G., Vazquez, A. I., Hsu, S., and Lello, L. (2018). Complex-Trait Prediction in the Era of Big Data. Trends Genet. 34, 746–754. doi:10.1016/j.tig.2018.07.004
Demircioğlu, D., Cukuroglu, E., Kindermans, M., Nandi, T., Calabrese, C., Fonseca, N. A., et al. (2019). A Pan-Cancer Transcriptome Analysis Reveals Pervasive Regulation through Alternative Promoters. Cell 178, 1465–e17. e1417. doi:10.1016/j.cell.2019.08.018
Deng, Y., Huang, Z., Xu, Y., Jin, J., Zhuo, W., Zhang, C., et al. (2014). MiR-215 Modulates Gastric Cancer Cell Proliferation by Targeting RB1. Cancer Lett. 342, 27–35. doi:10.1016/j.canlet.2013.08.033
Dhanasekaran, R., Deutzmann, A., Mahauad-Fernandez, W. D., Hansen, A. S., Gouw, A. M., and Felsher, D. W. (2021). The MYC Oncogene-The Grand Orchestrator of Cancer Growth and Immune Evasion. Nat. Rev. Clin. Oncol. 2021, 1–14. doi:10.1038/s41571-021-00549-2
Duffy, M. J., O'grady, S., Tang, M., and Crown, J. (2021). MYC as a Target for Cancer Treatment. Cancer Treat. Rev. 94, 102154. doi:10.1016/j.ctrv.2021.102154
Durinck, S., Spellman, P. T., Birney, E., and Huber, W. (2009). Mapping Identifiers for the Integration of Genomic Datasets with the R/Bioconductor Package biomaRt. Nat. Protoc. 4, 1184–1191. doi:10.1038/nprot.2009.97
Fadista, J., Manning, A. K., Florez, J. C., and Groop, L. (2016). The (In)famous GWAS P-Value Threshold Revisited and Updated for Low-Frequency Variants. Eur. J. Hum. Genet. 24, 1202–1205. doi:10.1038/ejhg.2015.269
Fang, L., Wu, S., Zhu, X., Cai, J., Wu, J., He, Z., et al. (2019). MYEOV Functions as an Amplified Competing Endogenous RNA in Promoting Metastasis by Activating TGF-β Pathway in NSCLC. Oncogene 38, 896–912. doi:10.1038/s41388-018-0484-9
Frost, F. G., Cherukuri, P. F., Milanovich, S., and Boerkoel, C. F. (2020). Pan‐cancer RNA‐seq Data Stratifies Tumours by Some Hallmarks of Cancer. J. Cel Mol Med 24, 418–430. doi:10.1111/jcmm.14746
Gallagher, M. D., and Chen-Plotkin, A. S. (2018). The Post-GWAS Era: From Association to Function. Am. J. Hum. Genet. 102, 717–730. doi:10.1016/j.ajhg.2018.04.002
Giambartolomei, C., Vukcevic, D., Schadt, E. E., Franke, L., Hingorani, A. D., Wallace, C., et al. (2014). Bayesian Test for Colocalisation between Pairs of Genetic Association Studies Using Summary Statistics. Plos Genet. 10, e1004383. doi:10.1371/journal.pgen.1004383
Goldman, M., Craft, B., Brooks, A., Zhu, J., and Haussler, D. (2018). The UCSC Xena Platform for Cancer Genomics Data Visualization and Interpretation. biorxiv 2018, 326470. doi:10.1101/326470
Gu, H.-Y., Zhang, C., Guo, J., Yang, M., Zhong, H.-C., Jin, W., et al. (2020). Risk Score Based on Expression of Five Novel Genes Predicts Survival in Soft Tissue Sarcoma. Aging 12, 3807–3827. doi:10.18632/aging.102847
Gusev, A., Ko, A., Shi, H., Bhatia, G., Chung, W., Penninx, B. W. J. H., et al. (2016). Integrative Approaches for Large-Scale Transcriptome-wide Association Studies. Nat. Genet. 48, 245–252. doi:10.1038/ng.3506
Hayashi, H., Arao, T., Togashi, Y., Kato, H., Fujita, Y., De Velasco, M. A., et al. (2015). The OCT4 Pseudogene POU5F1B Is Amplified and Promotes an Aggressive Phenotype in Gastric Cancer. Oncogene 34, 199–208. doi:10.1038/onc.2013.547
He, C., Li, A., Lai, Q., Ding, J., Yan, Q., Liu, S., et al. (2021). The DDX39B/FUT3/TGFβR-I axis Promotes Tumor Metastasis and EMT in Colorectal Cancer. Cell Death Dis 12, 74. doi:10.1038/s41419-020-03360-6
Hormozdiari, F., Kostem, E., Kang, E. Y., Pasaniuc, B., and Eskin, E. (2014). Identifying Causal Variants at Loci with Multiple Signals of Association. Genetics 198, 497–508. doi:10.1534/genetics.114.167908
Huang, C., Zhao, J., and Zhu, Z. (2021). Prognostic Nomogram of Prognosis-Related Genes and Clinicopathological Characteristics to Predict the 5-Year Survival Rate of Colon Cancer Patients. Front. Surg. 8. doi:10.3389/fsurg.2021.681721
Kandoth, C., Mclellan, M. D., Vandin, F., Ye, K., Niu, B., Lu, C., et al. (2013). Mutational Landscape and Significance across 12 Major Cancer Types. Nature 502, 333–339. doi:10.1038/nature12634
Kim, S.-K., Kim, H.-J., Park, J.-L., Heo, H., Kim, S.-Y., Lee, S.-I., et al. (2020). Identification of a Molecular Signature of Prognostic Subtypes in Diffuse-type Gastric Cancer. Gastric Cancer 23, 473–482. doi:10.1007/s10120-019-01029-4
Lappalainen, T., Sammeth, M., Sammeth, M., Friedländer, M. R., ‘t Hoen, P. A. C., Monlong, J., et al. (2013). Transcriptome and Genome Sequencing Uncovers Functional Variation in Humans. Nature 501, 506–511. doi:10.1038/nature12531
Lawlor, G., Doran, P. P., Macmathuna, P., and Murray, D. W. (2010). MYEOV (Myeloma Overexpressed Gene) Drives colon Cancer Cell Migration and Is Regulated by PGE2. J. Exp. Clin. Cancer Res. 29, 81–85. doi:10.1186/1756-9966-29-81
Lee, Y., Luca, F., Pique-Regi, R., and Wen, X. (2018). Bayesian Multi-SNP Genetic Association Analysis: Control of FDR and Use of Summary Statistics. bioRxiv 2018, 316471. doi:10.1371/journal.pgen.1007856
Leo, P. J., Madeleine, M. M., Wang, S., Schwartz, S. M., Newell, F., Pettersson-Kymmer, U., et al. (2017). Defining the Genetic Susceptibility to Cervical Neoplasia-A Genome-wide Association Study. Plos Genet. 13, e1006866. doi:10.1371/journal.pgen.1006866
Li, D., Duell, E. J., Yu, K., Risch, H. A., Olson, S. H., Kooperberg, C., et al. (2012). Pathway Analysis of Genome-wide Association Study Data Highlights Pancreatic Development Genes as Susceptibility Factors for Pancreatic Cancer. Carcinogenesis 33, 1384–1390. doi:10.1093/carcin/bgs151
Li, R., Yin, Y.-H., Ji, X.-L., Liu, X., Li, J.-P., and Qu, Y.-Q. (2021). Pan-Cancer Prognostic, Immunity, Stemness, and Anticancer Drug Sensitivity Characterization of N6-Methyladenosine RNA Modification Regulators in Human Cancers. Front. Mol. Biosciences 8, 644620. doi:10.3389/fmolb.2021.644620
Liang, E., Lu, Y., Shi, Y., Zhou, Q., and Zhi, F. (2020). MYEOV Increases HES1 Expression and Promotes Pancreatic Cancer Progression by Enhancing SOX9 Transactivity. Oncogene 39, 6437–6450. doi:10.1038/s41388-020-01443-4
Liberzon, A., Birger, C., Thorvaldsdóttir, H., Ghandi, M., Mesirov, J. P., and Tamayo, P. (2015). The Molecular Signatures Database Hallmark Gene Set Collection. Cel Syst. 1, 417–425. doi:10.1016/j.cels.2015.12.004
Liberzon, A., Subramanian, A., Pinchback, R., Thorvaldsdóttir, H., Tamayo, P., and Mesirov, J. P. (2011). Molecular Signatures Database (MSigDB) 3.0. Bioinformatics 27, 1739–1740. doi:10.1093/bioinformatics/btr260
Liu, J., Mccleland, M., Stawiski, E. W., Gnad, F., Mayba, O., Haverty, P. M., et al. (2014). Integrated Exome and Transcriptome Sequencing Reveals ZAK Isoform Usage in Gastric Cancer. Nat. Commun. 5, 3830. doi:10.1038/ncomms4830
Liu, J. N., Kong, X. S., Huang, T., Wang, R., Li, W., and Chen, Q. F. (2020). Clinical Implications of Aberrant PD-1 and CTLA4 Expression for Cancer Immunity and Prognosis: A Pan-Cancer Study. Front. Immunol. 11, 2048. doi:10.3389/fimmu.2020.02048
Lourenco, C., Resetca, D., Redel, C., Lin, P., Macdonald, A. S., Ciaccio, R., et al. (2021). MYC Protein Interactors in Gene Transcription and Cancer. Nat. Rev. Cancer 21, 579–591. doi:10.1038/s41568-021-00367-9
Malta, T. M., Sokolov, A., Gentles, A. J., Burzykowski, T., Poisson, L., Weinstein, J. N., et al. (2018). Machine Learning Identifies Stemness Features Associated with Oncogenic Dedifferentiation. Cell 173, 338–e15. e315. doi:10.1016/j.cell.2018.03.034
Mancuso, N., Freund, M. K., Johnson, R., Shi, H., Kichaev, G., Gusev, A., et al. (2019). Probabilistic fine-mapping of Transcriptome-wide Association Studies. Nat. Genet. 51, 675–682. doi:10.1038/s41588-019-0367-1
Mariathasan, S., Turley, S. J., Nickles, D., Castiglioni, A., Yuen, K., Wang, Y., et al. (2018). TGFβ Attenuates Tumour Response to PD-L1 Blockade by Contributing to Exclusion of T Cells. Nature 554, 544–548. doi:10.1038/nature25501
Maurano, M. T., Humbert, R., Rynes, E., Thurman, R. E., Haugen, E., Wang, H., et al. (2012). Systematic Localization of Common Disease-Associated Variation in Regulatory DNA. Science 337, 1190–1195. doi:10.1126/science.1222794
Miki, D., Ochi, H., Hayes, C. N., Abe, H., Yoshima, T., Aikata, H., et al. (2011). Variation in the DEPDC5 Locus Is Associated with Progression to Hepatocellular Carcinoma in Chronic Hepatitis C Virus Carriers. Nat. Genet. 43, 797–800. doi:10.1038/ng.876
Nakagawa, H., and Fujita, M. (2018). Whole Genome Sequencing Analysis for Cancer Genomics and Precision Medicine. Cancer Sci. 109, 513–522. doi:10.1111/cas.13505
O'Mara, T. A., Glubb, D. M., Amant, F., Annibali, D., Ashton, K., Attia, J., et al. (2018). Identification of Nine New Susceptibility Loci for Endometrial Cancer. Nat. Commun. 9 (1), 1–12. doi:10.1038/s41467-018-05427-7
Paglia, S., Sollazzo, M., Di Giacomo, S., Strocchi, S., and Grifoni, D. (2020). Exploring MYC Relevance to Cancer Biology from the Perspective of Cell Competition. Semin. Cancer Biol. 63, 49–59. doi:10.1016/j.semcancer.2019.05.009
Pan, Y., Zhan, L., Chen, L., Zhang, H., Sun, C., and Xing, C. (2018). POU5F1B Promotes Hepatocellular Carcinoma Proliferation by Activating AKT. Biomed. Pharmacother. 100, 374–380. doi:10.1016/j.biopha.2018.02.023
Pei, J., Wang, Y., and Li, Y. (2020). Identification of Key Genes Controlling Breast Cancer Stem Cell Characteristics via Stemness Indices Analysis. J. Transl Med. 18, 74–15. doi:10.1186/s12967-020-02260-9
Peng, J., Guan, J., Hui, W., and Shang, X. (2021). A Novel Subnetwork Representation Learning Method for Uncovering Disease-Disease Relationships. Methods 192, 77–84. doi:10.1016/j.ymeth.2020.09.002
Peng, J., Hui, W., Li, Q., Chen, B., Hao, J., Jiang, Q., et al. (2019). A Learning-Based Framework for miRNA-Disease Association Identification Using Neural Networks. Bioinformatics 35, 4364–4371. doi:10.1093/bioinformatics/btz254
Phelan, C. M., Kuchenbaecker, K. B., Tyrer, J. P., Kar, S. P., Lawrenson, K., Winham, S. J., et al. (2017). Identification of 12 New Susceptibility Loci for Different Histotypes of Epithelial Ovarian Cancer. Nat. Genet. 49, 680–691. doi:10.1038/ng.3826
Prasetyanti, P. R., and Medema, J. P. (2017). Intra-tumor Heterogeneity from a Cancer Stem Cell Perspective. Mol. Cancer 16, 41. doi:10.1186/s12943-017-0600-4
Priestley, P., Baber, J., Lolkema, M. P., Steeghs, N., De Bruijn, E., Shale, C., et al. (2019). Pan-cancer Whole-Genome Analyses of Metastatic Solid Tumours. Nature 575, 210–216. doi:10.1038/s41586-019-1689-y
Rafnar, T., Sulem, P., Stacey, S. N., Geller, F., Gudmundsson, J., Sigurdsson, A., et al. (2009). Sequence Variants at the TERT-Clptm1l Locus Associate with many Cancer Types. Nat. Genet. 41, 221–227. doi:10.1038/ng.296
Rashkin, S. R., Graff, R. E., Kachuri, L., Thai, K. K., Alexeeff, S. E., Blatchins, M. A., et al. (2020). Pan-cancer Study Detects Genetic Risk Variants and Shared Genetic Basis in Two Large Cohorts. Nat. Commun. 11 (1), 1–14. doi:10.1038/s41467-020-18246-6
Ritchie, M. E., Phipson, B., Wu, D., Hu, Y., Law, C. W., Shi, W., et al. (2015). Limma powers Differential Expression Analyses for RNA-Sequencing and Microarray Studies. Nucleic Acids Res. 43, e47. doi:10.1093/nar/gkv007
Rodriguez-Martin, B., Alvarez, E. G., Baez-Ortega, A., Zamora, J., Supek, F., Demeulemeester, J., et al. (2020). Pan-cancer Analysis of Whole Genomes Identifies Driver Rearrangements Promoted by LINE-1 Retrotransposition. Nat. Genet. 52, 306–319. doi:10.1038/s41588-019-0562-0
Saeed, W. H., Eissa, A. A., and Al-Doski, A. A. (2019). Impact of TP53 Gene Promoter Methylation on Chronic Lymphocytic Leukemia Pathogenesis and Progression. Jbm Vol. 10, 399–404. doi:10.2147/jbm.s221707
Schaid, D. J., Chen, W., and Larson, N. B. (2018). From Genome-wide Associations to Candidate Causal Variants by Statistical fine-mapping. Nat. Rev. Genet. 19, 491–504. doi:10.1038/s41576-018-0016-z
Schumacher, F. R., Al Olama, A. A., Berndt, S. I., Benlloch, S., Ahmed, M., Saunders, E. J., et al. (2018). Association Analyses of More Than 140,000 Men Identify 63 New Prostate Cancer Susceptibility Loci. Nat. Genet. 50, 928–936. doi:10.1038/s41588-018-0142-8
Seguin, L., Desgrosellier, J. S., Weis, S. M., and Cheresh, D. A. (2015). Integrins and Cancer: Regulators of Cancer Stemness, Metastasis, and Drug Resistance. Trends Cel Biol. 25, 234–240. doi:10.1016/j.tcb.2014.12.006
Shao, Y., Jia, H., Huang, L., Li, S., Wang, C., Aikemu, B., et al. (2021). An Original Ferroptosis-Related Gene Signature Effectively Predicts the Prognosis and Clinical Status for Colorectal Cancer Patients. Front. Oncol. 11, 711776. doi:10.3389/fonc.2021.711776
Shukla, S., Evans, J. R., Malik, R., Feng, F. Y., Dhanasekaran, S. M., Cao, X., et al. (2016). Development of a RNA-Seq Based Prognostic Signature in Lung Adenocarcinoma. J. Natl. Cancer Inst. Natl. Cancer Inst. 109, djw200. doi:10.1093/jnci/djw200
Specht, K., Haralambieva, E., Bink, K., Kremer, M., Mandl-Weber, S., Koch, I., et al. (2004). Different Mechanisms of Cyclin D1 Overexpression in Multiple Myeloma Revealed by Fluorescence In Situ Hybridization and Quantitative Analysis of mRNA Levels. Blood 104, 1120–1126. doi:10.1182/blood-2003-11-3837
Steen, C. B., Liu, C. L., Alizadeh, A. A., and Newman, A. M. (2020). “Profiling Cell Type Abundance and Expression in Bulk Tissues with CIBERSORTx,” in Stem Cell Transcriptional Networks. Editor B. L. Kidder (New York, United States: Springer), 135–157. doi:10.1007/978-1-0716-0301-7_7
Subramanian, A., Tamayo, P., Mootha, V. K., Mukherjee, S., Ebert, B. L., Gillette, M. A., et al. (2005). Gene Set Enrichment Analysis: A Knowledge-Based Approach for Interpreting Genome-wide Expression Profiles. Proc. Natl. Acad. Sci. 102, 15545–15550. doi:10.1073/pnas.0506580102
Tam, V., Patel, N., Turcotte, M., Bossé, Y., Paré, G., and Meyre, D. (2019). Benefits and Limitations of Genome-wide Association Studies. Nat. Rev. Genet. 20, 467–484. doi:10.1038/s41576-019-0127-1
Tarver, T. (2012). Cancer Facts & Figures 2012. American Cancer Society (ACS). J. Consumer Health Internet 16, 366–367. doi:10.1080/15398285.2012.701177
Thorsson, V., Gibbs, D. L., Brown, S. D., Wolf, D., Bortone, D. S., Ou Yang, T. H., et al. (2018). The Immune Landscape of Cancer. Immunity 48, 812–e14. e814. doi:10.1016/j.immuni.2018.03.023
Toledo-Stuardo, K., Ribeiro, C. H., Canals, A., Morales, M., Gárate, V., Rodríguez-Siza, J., et al. (2021). Major Histocompatibility Complex Class I-Related Chain A (MICA) Allelic Variants Associate with Susceptibility and Prognosis of Gastric Cancer. Front. Immunol. 12, 645528. doi:10.3389/fimmu.2021.645528
Tomczak, K., Czerwińska, P., and Wiznerowicz, M. (2015). The Cancer Genome Atlas (TCGA): an Immeasurable Source of Knowledge. Contemp. Oncol. (Pozn) 19, A68–A77. doi:10.5114/wo.2014.47136
Turnbull, C., Rapley, E. A., Rapley, E. A., Seal, S., Pernet, D., Renwick, A., et al. (2010). Variants Near DMRT1, TERT and ATF7IP Are Associated with Testicular Germ Cell Cancer. Nat. Genet. 42, 604–607. doi:10.1038/ng.607
Vargas, A. J., and Harris, C. C. (2016). Biomarker Development in the Precision Medicine Era: Lung Cancer as a Case Study. Nat. Rev. Cancer 16, 525–537. doi:10.1038/nrc.2016.56
Visscher, P. M., Wray, N. R., Zhang, Q., Sklar, P., Mccarthy, M. I., Brown, M. A., et al. (2017). 10 Years of GWAS Discovery: Biology, Function, and Translation. Am. J. Hum. Genet. 101, 5–22. doi:10.1016/j.ajhg.2017.06.005
Wainberg, M., Sinnott-Armstrong, N., Mancuso, N., Barbeira, A. N., Knowles, D. A., Golan, D., et al. (2019). Opportunities and Challenges for Transcriptome-wide Association Studies. Nat. Genet. 51, 592–599. doi:10.1038/s41588-019-0385-z
Wang, G., Sarkar, A., Carbonetto, P., and Stephens, M. (2020a). A Simple New Approach to Variable Selection in Regression, with Application to Genetic fine Mapping. J. R. Stat. Soc. B 82, 1273–1300. doi:10.1111/rssb.12388
Wang, S., Xiong, Y., Zhang, Q., Su, D., Yu, C., Cao, Y., et al. (2020b). Clinical Significance and Immunogenomic Landscape Analyses of the Immune Cell Signature Based Prognostic Model for Patients with Breast Cancer. Brief Bioinform 22. doi:10.1093/bib/bbaa311
Wang, S. S., Purdue, M. P., Cerhan, J. R., Zheng, T., Menashe, I., Armstrong, B. K., et al. (2009). Common Gene Variants in the Tumor Necrosis Factor (TNF) and TNF Receptor Superfamilies and NF-kB Transcription Factors and Non-hodgkin Lymphoma Risk. PLOS ONE 4, e5360. doi:10.1371/journal.pone.0005360
Wen, X., Lee, Y., Luca, F., and Pique-Regi, R. (2016). Efficient Integrative Multi-SNP Association Analysis via Deterministic Approximation of Posteriors. Am. J. Hum. Genet. 98, 1114–1129. doi:10.1016/j.ajhg.2016.03.029
Westra, H.-J., Peters, M. J., Esko, T., Yaghootkar, H., Schurmann, C., Kettunen, J., et al. (2013). Systematic Identification of Trans eQTLs as Putative Drivers of Known Disease Associations. Nat. Genet. 45, 1238–1243. doi:10.1038/ng.2756
Wu, C., and Pan, W. (2020). A Powerful fine-mapping Method for Transcriptome-wide Association Studies. Hum. Genet. 139, 199–213. doi:10.1007/s00439-019-02098-2
Wu, F., Wang, Z. L., Wang, K. Y., Li, G. Z., Chai, R. C., Liu, Y. Q., et al. (2020). Classification of Diffuse Lower‐grade Glioma Based on Immunological Profiling. Mol. Oncol. 14, 2081–2095. doi:10.1002/1878-0261.12707
Xu, Z., Li, X., Li, H., Nie, C., Liu, W., Li, S., et al. (2020). Suppression of DDX39B Sensitizes Ovarian Cancer Cells to DNA-Damaging Chemotherapeutic Agents via Destabilizing BRCA1 mRNA. Oncogene 39, 7051–7062. doi:10.1038/s41388-020-01482-x
Xu, Z., Wu, C., Wei, P., and Pan, W. (2017). A Powerful Framework for Integrating eQTL and GWAS Summary Data. Genetics 207, 893–902. doi:10.1534/genetics.117.300270
Yang, W., Soares, J., Greninger, P., Edelman, E. J., Lightfoot, H., Forbes, S., et al. (2012). Genomics of Drug Sensitivity in Cancer (GDSC): a Resource for Therapeutic Biomarker Discovery in Cancer Cells. Nucleic Acids Res. 41, D955–D961. doi:10.1093/nar/gks1111
Yoshihara, K., Shahmoradgoli, M., Martínez, E., Vegesna, R., Kim, H., Torres-Garcia, W., et al. (2013). Inferring Tumour Purity and Stromal and Immune Cell Admixture from Expression Data. Nat. Commun. 4 (1), 1–11. doi:10.1038/ncomms3612
Zamora-Fuentes, J. M., Hernández-Lemus, E., and Espinal-Enríquez, J. (2020). Gene Expression and Co-expression Networks Are Strongly Altered through Stages in Clear Cell Renal Carcinoma. Front. Genet. 11, 578679. doi:10.3389/fgene.2020.578679
Zeng, P., Dai, J., Jin, S., and Zhou, X. (2021). Aggregating Multiple Expression Prediction Models Improves the Power of Transcriptome-wide Association Studies. Hum. Mol. Genet. 30, 939–951. doi:10.1093/hmg/ddab056
Zhang, C., Chen, T., Li, Z., Liu, A., Xu, Y., Gao, Y., et al. (2020). Depiction of Tumor Stemlike Features and Underlying Relationships with hazard Immune Infiltrations Based on Large Prostate Cancer Cohorts. Brief Bioinform 22. doi:10.1093/bib/bbaa211
Zhang, W., Bouchard, G., Yu, A., Shafiq, M., Jamali, M., Shrager, J. B., et al. (2018). GFPT2-Expressing Cancer-Associated Fibroblasts Mediate Metabolic Reprogramming in Human Lung Adenocarcinoma. Cancer Res. 78, 3445–3457. doi:10.1158/0008-5472.CAN-17-2928
Keywords: pan-cancer, risk estimation, genome-wide association study, summary statistics, fine-mapping
Citation: Tao S, Ye X, Pan L, Fu M, Huang P, Peng Z and Yang S (2021) Construction and Clinical Translation of Causal Pan-Cancer Gene Score Across Cancer Types. Front. Genet. 12:784775. doi: 10.3389/fgene.2021.784775
Received: 28 September 2021; Accepted: 24 November 2021;
Published: 23 December 2021.
Edited by:
Jing Hua Zhao, University of Cambridge, United KingdomReviewed by:
Wenlong Ren, Nantong University, ChinaCopyright © 2021 Tao, Ye, Pan, Fu, Huang, Peng and Yang. This is an open-access article distributed under the terms of the Creative Commons Attribution License (CC BY). The use, distribution or reproduction in other forums is permitted, provided the original author(s) and the copyright owner(s) are credited and that the original publication in this journal is cited, in accordance with accepted academic practice. No use, distribution or reproduction is permitted which does not comply with these terms.
*Correspondence: Sheng Yang, eWFuZ3NoZW5nQG5qbXUuZWR1LmNu
†These authors have contributed equally to this work and share first authorship
Disclaimer: All claims expressed in this article are solely those of the authors and do not necessarily represent those of their affiliated organizations, or those of the publisher, the editors and the reviewers. Any product that may be evaluated in this article or claim that may be made by its manufacturer is not guaranteed or endorsed by the publisher.
Research integrity at Frontiers
Learn more about the work of our research integrity team to safeguard the quality of each article we publish.