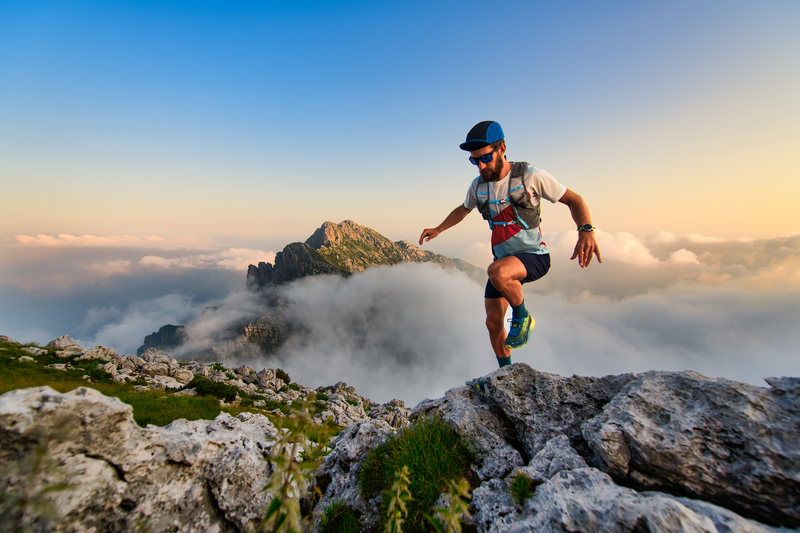
94% of researchers rate our articles as excellent or good
Learn more about the work of our research integrity team to safeguard the quality of each article we publish.
Find out more
ORIGINAL RESEARCH article
Front. Genet. , 04 November 2021
Sec. Statistical Genetics and Methodology
Volume 12 - 2021 | https://doi.org/10.3389/fgene.2021.782005
This article is part of the Research Topic System Biology Methods and Tools for Integrating Omics Data, Volume II View all 15 articles
Systemic lupus erythematosus (SLE) is a complex and heterogeneous autoimmune disease that the immune system attacks healthy cells and tissues. SLE is difficult to get a correct and timely diagnosis, which makes its morbidity and mortality rate very high. The pathogenesis of SLE remains to be elucidated. To clarify the potential pathogenic mechanism of SLE, we performed an integrated analysis of two RNA-seq datasets of SLE. Differential expression analysis revealed that there were 4,713 and 2,473 differentially expressed genes, respectively, most of which were up-regulated. After integrating differentially expressed genes, we identified 790 common differentially expressed genes (DEGs). Gene functional enrichment analysis was performed and found that common differentially expressed genes were significantly enriched in some important immune-related biological processes and pathways. Our analysis provides new insights into a better understanding of the pathogenic mechanisms and potential candidate markers for systemic lupus erythematosus.
Systemic lupus erythematosus is a chronic autoimmune disease (Beccastrini et al., 2013; Davies et al., 2021). Its clinical manifestations are heterogeneous and involve one or more organs such as skin, kidney, joints, and nervous system (Von Feldt, 1995; Adinolfi et al., 2016; Ronco et al., 2021). The latest data from the US Lupus Registry and published studies around the world can more accurately estimate the incidence and prevalence of SLE. It is estimated that the incidence of 23.2 cases per 100,000 people in North America is the highest in the world (Tsokos, 2011; Rees et al., 2017). SLE is a heterogeneous rheumatic systemic disease with extremely diverse clinical manifestations and diverse pathogenesis (Wu et al., 2021). In addition, it is one of the most varied diseases in its epidemiology and etiology, with different types of immune dysfunction (Oku and Atsumi, 2018). SLE patients’ immune system activation is characterized by exaggerated B cells and T cells responses (Tsokos, 2011). The health-related quality of life of SLE patients is significantly impaired (Di Battista et al., 2018). To obtain a better diagnosis and treatment method, it is necessary to explore the pathogenesis of SLE.
Since the successful application of high-throughput technology, it has been widely used in almost all biological research fields (Hess et al., 2020). With the development of high-throughput technology (Hess et al., 2020), biological research has been transformed from a single gene level to a full transcriptome level, which has greatly advanced many research fields in biology (Wang et al., 2009; McDermaid et al., 2019). Cheng. et al. based on the genome-wide expression data of peripheral blood mononuclear cells (PBMC) of SLE patients found a novel marker of SLE (Cheng et al., 2021). Jiang. et al. discovered a new type of lncRNA that plays an important role in the pathogenesis of SLE based on the whole transcriptome data of PBMC of SLE patients (Jiang et al., 2021). However, these studies were only conducted on a single dataset, and there was heterogeneity between different datasets. Therefore, through a comprehensive analysis of multiple datasets, more robust results will be obtained.
In this study, we conducted a systematic analysis of two gene expression datasets of SLE. First, differential expression analysis was performed to obtain differentially expressed genes (DEGs) in each dataset. To obtain robust results, we intersected the DEGs s of the two datasets. We found that 790 genes were differentially expressed in both datasets. Finally, gene function enrichment analysis showed that common DEGs were enriched in immune-related biological pathways. Overall, our research provided new insight into the molecular mechanism of SLE.
“Systemic Lupus Erythematosus” and “RNA-seq” were used as the keywords for searching the GEO database. The gene expression datasets of PBMC from freshly isolated healthy controls and SLE patients were downloaded from the GEO database (GSE162828 and GSE169080), the platforms used were GPL24676, and GPL20795. GSE162828 included 10 samples of peripheral blood mononuclear cells and was divided into the SLE group (5 samples) and healthy controls group (5 samples). GSE169080 included seven samples of peripheral blood mononuclear cells and was divided into SLE group (4 samples) and healthy controls group (3 samples) (Clough and Barrett, 2016; Cheng et al., 2021; Jiang et al., 2021).
R package DESeq2 (1.26.0) was used for the analysis of the original datasets (Love et al., 2014). |log FC| > 1 and p. adj <0.05 were defined as the cutoff values for further analysis of DEGs. Volcano and heatmap were constructed by R package ggplot2. Venn plot (http://bioinformatics.psb.ugent.be/webtools/Venn/) was used to draw the intersection of two databases.
Protein-protein interaction (PPI) network analysis helps to study the molecular mechanism of diseases from a systematic perspective and discover new drug targets (Wu et al., 2019). STRING (https://string-db.org/) is a database covering more than 5,000 organisms with known and predicted protein-protein interactions, providing direct (physical) and indirect (functional) associations (Szklarczyk et al., 2017). We used String (https://string-db.org/) to generate biological networks for proteins, and the results were analyzed by Cytoscape (Shannon et al., 2003; Szklarczyk et al., 2017).
Gene Ontology (GO) is an ontology widely used in the field of bioinformatics, which covers three aspects of biology: biological process (BP), cellular component (CC), and molecular function (MF) (Thomas, 2017). Kyoto Encyclopedia of Genes and Genomes (KEGG) is a biological system advanced function and utility database based on molecular-level information from genome sequencing and other high-throughput experimental technologies (Kanehisa et al., 2017). In this study, R package clusterProfiler was used to perform GO functional annotation and KEGG pathway enrichment analysis for DEGs (Yu et al., 2012).
To obtain abnormally expressed genes in SLE patients, we separately analyzed the differential expression of two GEO datasets (GSE162828 and GSE169080). As shown in Figure 1A, there were 4,713 DEGs, including 2,717 up-regulated and 1,996 down-regulated in the GSE162828 dataset. In the GSE169080 dataset, there were 2,473 DEGs, including 1,552 up-regulated and 921 down-regulated (Figure 1B). In both datasets, the number of up-regulated DEGs was more than the number of down-regulated DEGs (Figure 1C). In the GSE162828 dataset, the up-regulated DEGs accounted for 56.7% of all DEGs. At the same time, the up-regulated DEGs accounted for 62.8% of all DEGs in the GSE169080 dataset. The trends in the two datasets were roughly the same.
FIGURE 1. Analysis of differentially expressed genes (DEGs) between SLE patients and healthy controls. (A,B) The volcano plots exhibited the differentially expressed genes in SLE patients groups compared to healthy controls groups. Each dot in the figure represented one gene. The green dots indicated the differentially expressed genes, while the red dots denoted no significant difference. (C) Barplot showed the number of DEGs whose expression levels were up-regulated (green) and down-regulated (red) in the two datasets. (D, E) Hierarchy Clustering Analysis. Repeated samples are clustered together, indicating the repeatability of samples and the differences between samples.
In addition, the heatmap showed that DEGs can group samples by sample type, namely SLE patients (SLE) and healthy controls (Norm) (Figures 1D,E). These genes were highly concordant within groups. The expression level of these genes between SLE patients and healthy controls exhibited a large difference in both databases.
Due to the heterogeneity between different datasets, the analysis results of different datasets may have certain differences (Ying et al., 2020). The gene expression in different samples may be different (Bao et al., 2021). To avoid this problem, integrating multiple datasets and a large number of samples help obtain more solid results (Kou et al., 2020). In this study, we integrated DEGs from two datasets to obtain common DEGs.
The Venn diagram showed that 790 DEGs were shared between the two datasets (Figure 2A). They accounted for 16.8% (GSE162828) and 31.9% (GSE169080) of the two datasets, respectively. There were 3,923 DEGs only in the GSE162828 dataset, and 1,683 DEGs only in GSE169080 dataset. This may be caused by different sequencing technologies and sample heterogeneity.
FIGURE 2. Common differentially expressed genes. (A) Venn plot showed the intersection of DEGs in two datasets (B) The histogram showed the distribution of DEGs on chromosomes.
We defined these 790 DEGs as common DEGs. To further explore the distribution of common DEGs on the chromosomes, we had made statistics on the chromosomal locations of these genes. As shown in Figure 2B, we found that these genes were distributed on every chromosome. Most of these genes were distributed on chromosome 19. On the contrary, they were only 6 DEGs on chromosome 13.
Proteins usually perform biological functions in concert. It has been shown that there is a close relationship between Protein-Protein Interaction (PPI) and the biological functions of gene/protein clusters (Li H. et al., 2019). To further analyze the correlation between common DEGs, STRING and Cytoscape were used to construct the PPI network (Figure 3). Part of common DEGs was predicted to have a strong association with other genes. The size and color of the node depending on the degree, the larger the degree, the larger the node.
FIGURE 3. Protein-Protein Interaction network of common DEGs. The size and color of the node depending on the degree, the larger the degree, the larger the node.
Especially, CCNB2, CDCA8, AURKB, BUB1B, RRM2, BIRC5, and UBE2C had the largest degree. CCNB2 is an essential component of the cell cycle regulatory machinery (Takashima et al., 2014; Li R. et al., 2019). CDCA8 is an essential regulator of mitosis and cell division (Zhang et al., 2020). AURKB participates in the regulation of alignment and segregation of chromosomes during mitosis and meiosis through association with microtubules (Ahmed et al., 2021). BUB1B encodes a kinase involved in the spindle checkpoint function (Zhang et al., 2021). RRM2 encodes one of two non-identical subunits for ribonucleotide reductase (Mazzu et al., 2020). BIRC5 encodes negative regulatory proteins that prevent apoptotic cell death (Adamopoulos et al., 2021). UBE2C is required for the destruction of mitotic cyclins and cell cycle progression (Jin et al., 2020).
To investigate the biological function of common DEGs, we used clusterProfiler to perform Functional enrichment analysis. Biological Process (BP) enrichment showed that the common DEGs were enriched in neutrophil mediated immunity, neutrophil degranulation, neutrophil activation involved in immune response, neutrophil activation and regulation of inflammatory response (Figure 4A). Cellular Component (CC) enrichment showed that the common DEGs were mainly enriched in secretory granule lumen, cytoplasmic vesicle lumen, vesicle lumen, secretory granule membrane and vacuolar membrane (Figure 4B). Molecular Function (MF) enrichment showed that the common DEGs were significantly enriched in tubulin binding, microtubule binding, carbohydrate binding, cargo receptor activity and immunoglobulin binding (Figure 4C).
FIGURE 4. Functional Enrichment Analysis of common DEGs. (A) Biological process analysis of common DEGs. (B) Cellular component analysis of common DEGs. (C) Molecular function analysis of common DEGs. (D) KEGG analysis of common DEGs.
KEGG pathway analysis provided a potential functional cluster of common DEGs, indicating that the common DEGs were clustered in Herpes simplex virus one infection, Human T−cell leukemia virus one infection, Cell cycle, Transcriptional misregulation in cancer and Epstein−Barr virus infection (Figure 4D).
SLE is a multi-system autoimmune inflammation that can affect multiple organs and cause extensive and severe clinical manifestations (Wu et al., 2021). The current understanding of the pathogenesis of SLE is not comprehensive. The key driving factors involved in the occurrence and development of SLE remain to be determined. In this study, we provided new insights into the transcriptome of SLE based on RNA-seq data.
The results showed that compared with the normal healthy control groups, a large number of genes in SLE patients were abnormally expressed. Through integrated analysis, we found that there were 790 shared DEGs in the two databases. The results indicated that these common DEGs may lead to the occurrence and development of SLE. Previous studies had shown that lncRNA and circRNA are important factors leading to the occurrence of SLE (Cheng et al., 2021; Jiang et al., 2021). We found that the differential expression of these common DEGs might play an important role in this process.
Through further analysis, we found that the DEGs tended to up-regulated in the two datasets. Through protein-protein interaction network analysis of commonly dysregulated genes, we found that there was a strong correlation between these genes. These PPI networks may have affected the occurrence and development of SLE. Pathway enrichment results showed that common DEGs were significantly enriched in immune-related pathways such as neutrophil mediated immunity, neutrophil degranulation, neutrophil activation involved in the immune response.
In summary, we integrated and analyzed high-throughput sequencing RNA-seq datasets to uncover potential molecular mechanisms of SLE. Our findings provide new clues for possible targeted therapy of SLE. Further studies on the functions of those common DEGs hoped to better understand SLE by integrating more data.
Publicly available datasets were analyzed in this study. This data can be found here: https://www.ncbi.nlm.nih.gov/geo/ GSE162828 and GSE169080
ZZ designed the experiments. HZ obtained the data from GEO. YW, JF, SW, YW, and WK analyzed the data. HZ and ZZ wrote the manuscript. All authors read and approved the manuscript.
The authors declare that the research was conducted in the absence of any commercial or financial relationships that could be construed as a potential conflict of interest.
All claims expressed in this article are solely those of the authors and do not necessarily represent those of their affiliated organizations, or those of the publisher, the editors and the reviewers. Any product that may be evaluated in this article, or claim that may be made by its manufacturer, is not guaranteed or endorsed by the publisher.
Adamopoulos, P. G., Tsiakanikas, P., Adam, E. E., and Scorilas, A. (2021). Unraveling Novel Survivin mRNA Transcripts in Cancer Cells Using an In-House Developed Targeted High-Throughput Sequencing Approach. Genomics 113 (1 Pt 2), 573–581. doi:10.1016/j.ygeno.2020.09.053
Adinolfi, A., Valentini, E., Calabresi, E., Tesei, G., Signorini, V., Barsotti, S., et al. (2016). One Year in Review 2016: Systemic Lupus Erythematosus. Clin. Exp. Rheumatol. 34 (4), 569–574.
Ahmed, A., Shamsi, A., Mohammad, T., Hasan, G. M., Islam, A., and Hassan, M. I. (2021). Aurora B Kinase: a Potential Drug Target for Cancer Therapy. J. Cancer Res. Clin. Oncol. 147 (8), 2187–2198. doi:10.1007/s00432-021-03669-5
Bao, X., Shi, R., Zhao, T., Wang, Y., Anastasov, N., Rosemann, M., et al. (2021). Integrated Analysis of Single-Cell RNA-Seq and Bulk RNA-Seq Unravels Tumour Heterogeneity Plus M2-like Tumour-Associated Macrophage Infiltration and Aggressiveness in TNBC. Cancer Immunol. Immunother. 70 (1), 189–202. doi:10.1007/s00262-020-02669-7
Beccastrini, E., D'Elios, M. M., Emmi, G., Silvestri, E., Squatrito, D., Prisco, D., et al. (2013). Systemic Lupus Erythematosus: Immunopathogenesis and Novel Therapeutic Targets. Int. J. Immunopathol Pharmacol. 26 (3), 585–596. doi:10.1177/039463201302600302
Cheng, Q., Chen, M., Chen, X., Chen, X., Jiang, H., Wu, H., et al. (2021). Novel Long Non-coding RNA Expression Profile of Peripheral Blood Mononuclear Cells Reveals Potential Biomarkers and Regulatory Mechanisms in Systemic Lupus Erythematosus. Front. Cel Dev. Biol. 9, 639321. doi:10.3389/fcell.2021.639321
Clough, E., and Barrett, T. (2016). The Gene Expression Omnibus Database. Methods Mol. Biol. 1418, 93–110. doi:10.1007/978-1-4939-3578-9_5
Davies, K., Dures, E., and Ng, W.-F. (2021). Fatigue in Inflammatory Rheumatic Diseases: Current Knowledge and Areas for Future Research. Nat. Rev. Rheumatol. 17, 651–664. doi:10.1038/s41584-021-00692-1
Di Battista, M., Marcucci, E., Elefante, E., Tripoli, A., Governato, G., Zucchi, D., et al. (2018). One Year in Review 2018: Systemic Lupus Erythematosus. Clin. Exp. Rheumatol. 36 (5), 763–777.
Hess, J. F., Kohl, T. A., Kotrová, M., Rönsch, K., Paprotka, T., Mohr, V., et al. (2020). Library Preparation for Next Generation Sequencing: A Review of Automation Strategies. Biotechnol. Adv. 41, 107537. doi:10.1016/j.biotechadv.2020.107537
Jiang, Z., Zhong, Z., Miao, Q., Zhang, Y., Ni, B., Zhang, M., et al. (2021). circPTPN22 as a Novel Biomarker and ceRNA in Peripheral Blood Mononuclear Cells of Rheumatoid Arthritis. Mol. Med. Rep. 24 (2), 617. doi:10.3892/mmr.2021.12256
Jin, Z., Zhao, X., Cui, L., Xu, X., Zhao, Y., Younai, F., et al. (2020). UBE2C Promotes the Progression of Head and Neck Squamous Cell Carcinoma. Biochem. Biophysical Res. Commun. 523 (2), 389–397. doi:10.1016/j.bbrc.2019.12.064
Kanehisa, M., Furumichi, M., Tanabe, M., Sato, Y., and Morishima, K. (2017). KEGG: New Perspectives on Genomes, Pathways, Diseases and Drugs. Nucleic Acids Res. 45 (D1), D353–D361. doi:10.1093/nar/gkw1092
Kou, N., Zhou, W., He, Y., Ying, X., Chai, S., Fei, T., et al. (2020). A Mendelian Randomization Analysis to Expose the Causal Effect of IL-18 on Osteoporosis Based on Genome-wide Association Study Data. Front. Bioeng. Biotechnol. 8, 201. doi:10.3389/fbioe.2020.00201
Li, H., Long, J., Xie, F., Kang, K., Shi, Y., Xu, W., et al. (2019a). Transcriptomic Analysis and Identification of Prognostic Biomarkers in Cholangiocarcinoma. Oncol. Rep. 42 (5), 1833–1842. doi:10.3892/or.2019.7318
Li, R., Jiang, X., Zhang, Y., Wang, S., Chen, X., Yu, X., et al. (2019b). Cyclin B2 Overexpression in Human Hepatocellular Carcinoma Is Associated with Poor Prognosis. Arch. Med. Res. 50 (1), 10–17. doi:10.1016/j.arcmed.2019.03.003
Love, M. I., Huber, W., and Anders, S. (2014). Moderated Estimation of Fold Change and Dispersion for RNA-Seq Data with DESeq2. Genome Biol. 15 (12), 550. doi:10.1186/s13059-014-0550-8
Mazzu, Y. Z., Armenia, J., Nandakumar, S., Chakraborty, G., Yoshikawa, Y., Jehane, L. E., et al. (2020). Ribonucleotide Reductase Small Subunit M2 Is a Master Driver of Aggressive Prostate Cancer. Mol. Oncol. 14 (8), 1881–1897. doi:10.1002/1878-0261.12706
McDermaid, A., Monier, B., Zhao, J., Liu, B., and Ma, Q. (2019). Interpretation of Differential Gene Expression Results of RNA-Seq Data: Review and Integration. Brief Bioinform 20 (6), 2044–2054. doi:10.1093/bib/bby067
Oku, K., and Atsumi, T. (2018). Systemic Lupus Erythematosus: Nothing Stale Her Infinite Variety. Mod. Rheumatol. 28 (5), 758–765. doi:10.1080/14397595.2018.1494239
Rees, F., Doherty, M., Grainge, M. J., Lanyon, P., and Zhang, W. (2017). The Worldwide Incidence and Prevalence of Systemic Lupus Erythematosus: a Systematic Review of Epidemiological Studies. Rheumatology (Oxford) 56 (11), 1945–1961. doi:10.1093/rheumatology/kex260
Ronco, P., Beck, L., Debiec, H., Fervenza, F. C., Hou, F. F., Jha, V., et al. (2021). Membranous Nephropathy. Nat. Rev. Dis. Primers 7 (1), 69. doi:10.1038/s41572-021-00303-z
Shannon, P., Markiel, A., Ozier, O., Baliga, N. S., Wang, J. T., Ramage, D., et al. (2003). Cytoscape: a Software Environment for Integrated Models of Biomolecular Interaction Networks. Genome Res. 13 (11), 2498–2504. doi:10.1101/gr.1239303
Szklarczyk, D., Morris, J. H., Cook, H., Kuhn, M., Wyder, S., Simonovic, M., et al. (2017). The STRING Database in 2017: Quality-Controlled Protein-Protein Association Networks, Made Broadly Accessible. Nucleic Acids Res. 45 (D1), D362–D368. doi:10.1093/nar/gkw937
Takashima, S., Saito, H., Takahashi, N., Imai, K., Kudo, S., Atari, M., et al. (2014). Strong Expression of Cyclin B2 mRNA Correlates with a Poor Prognosis in Patients with Non-small Cell Lung Cancer. Tumor Biol. 35 (5), 4257–4265. doi:10.1007/s13277-013-1556-7
Thomas, P. D. (2017). The Gene Ontology and the Meaning of Biological Function. Methods Mol. Biol. 1446, 15–24. doi:10.1007/978-1-4939-3743-1_2
Tsokos, G. C. (2011). Systemic Lupus Erythematosus. N. Engl. J. Med. 365 (22), 2110–2121. doi:10.1056/NEJMra1100359
Von Feldt, J. M. (1995). Systemic Lupus Erythematosus. Postgrad. Med. 97 (483), 7986–7994. doi:10.1080/00325481.1995.11945982
Wang, Z., Gerstein, M., and Snyder, M. (2009). RNA-seq: a Revolutionary Tool for Transcriptomics. Nat. Rev. Genet. 10 (1), 57–63. doi:10.1038/nrg2484
Wu, D., Ai, L., Sun, Y., Yang, B., Chen, S., Wang, Q., et al. (2021). Role of NLRP3 Inflammasome in Lupus Nephritis and Therapeutic Targeting by Phytochemicals. Front. Pharmacol. 12, 621300. doi:10.3389/fphar.2021.621300
Wu, H.-T., Chen, W.-T., Li, G.-W., Shen, J.-X., Ye, Q.-Q., Zhang, M.-L., et al. (2019). Analysis of the Differentially Expressed Genes Induced by Cisplatin Resistance in Oral Squamous Cell Carcinomas and Their Interaction. Front. Genet. 10, 1328. doi:10.3389/fgene.2019.01328
Ying, X., Jin, X., Wang, P., He, Y., Zhang, H., Ren, X., et al. (2020). Integrative Analysis for Elucidating Transcriptomics Landscapes of Glucocorticoid-Induced Osteoporosis. Front. Cel Dev. Biol. 8, 252. doi:10.3389/fcell.2020.00252
Yu, G., Wang, L.-G., Han, Y., and He, Q.-Y. (2012). clusterProfiler: an R Package for Comparing Biological Themes Among Gene Clusters. OMICS: A J. Integr. Biol. 16 (5), 284–287. doi:10.1089/omi.2011.0118
Zhang, C., Zhao, L., Leng, L., Zhou, Q., Zhang, S., Gong, F., et al. (2020). CDCA8 Regulates Meiotic Spindle Assembly and Chromosome Segregation during Human Oocyte Meiosis. Gene 741, 144495. doi:10.1016/j.gene.2020.144495
Keywords: systemic lupus erythematosus, differential expression analysis, gene functional enrichment analysis, RNA-seq, protein-protein interaction
Citation: Zhang H, Wang Y, Feng J, Wang S, Wang Y, Kong W and Zhang Z (2021) Integrative Analysis for Elucidating Transcriptomics Landscapes of Systemic Lupus Erythematosus. Front. Genet. 12:782005. doi: 10.3389/fgene.2021.782005
Received: 23 September 2021; Accepted: 20 October 2021;
Published: 04 November 2021.
Edited by:
Lei Deng, Central South University, ChinaReviewed by:
Chen Qingfeng, Guangxi University, ChinaCopyright © 2021 Zhang, Wang, Feng, Wang, Wang, Kong and Zhang. This is an open-access article distributed under the terms of the Creative Commons Attribution License (CC BY). The use, distribution or reproduction in other forums is permitted, provided the original author(s) and the copyright owner(s) are credited and that the original publication in this journal is cited, in accordance with accepted academic practice. No use, distribution or reproduction is permitted which does not comply with these terms.
*Correspondence: Zhiyi Zhang, emhhbmd6aGl5aTIwMTRAMTYzLmNvbQ==
Disclaimer: All claims expressed in this article are solely those of the authors and do not necessarily represent those of their affiliated organizations, or those of the publisher, the editors and the reviewers. Any product that may be evaluated in this article or claim that may be made by its manufacturer is not guaranteed or endorsed by the publisher.
Research integrity at Frontiers
Learn more about the work of our research integrity team to safeguard the quality of each article we publish.