- Department of Pathology, Ruian People’s Hospital, The Third Affiliated Hospital of Wenzhou Medical University, Wenzhou, China
The tumor microenvironment (TME) has been shown to be involved in angiogenesis, tumor metastasis, and immune response, thereby affecting the treatment and prognosis of patients. This study aims to identify genes that are dysregulated in the TME of patients with colon adenocarcinoma (COAD) and to evaluate their prognostic value based on RNA omics data. We obtained 512 COAD samples from the Cancer Genome Atlas (TCGA) database and 579 COAD patients from the independent dataset (GSE39582) in the Gene Expression Omnibus (GEO) database. The immune/stromal/ESTIMATE score of each patient based on their gene expression was calculated using the ESTIMATE algorithm. Kaplan–Meier survival analysis, Cox regression analysis, gene functional enrichment analysis, and protein–protein interaction (PPI) network analysis were performed. We found that immune and stromal scores were significantly correlated with COAD patients’ overall survival (log rank p < 0.05). By comparing the high immune/stromal score group with the low score group, we identified 688 intersection differentially expressed genes (DEGs) from the TCGA dataset (663 upregulated and 25 downregulated). The functional enrichment analysis of intersection DEGs showed that they were mainly enriched in the immune process, cell migration, cell motility, Toll-like receptor signaling pathway, and PI3K-Akt signaling pathway. The hub genes were revealed by PPI network analysis. Through Kaplan–Meier and Cox analysis, four TME-related genes that were significantly related to the prognosis of COAD patients were verified in GSE39582. In addition, we uncovered the relationship between the four prognostic genes and immune cells in COAD. In conclusion, based on the RNA expression profiles of 1091 COAD patients, we screened four genes that can predict prognosis from the TME, which may serve as candidate prognostic biomarkers for COAD.
Introduction
Colorectal cancer (CRC) is a common malignant gastrointestinal tumor worldwide (Siegel et al., 2017; Siegel et al., 2020). Colon adenocarcinoma (COAD) is the most common histological type of CRC (Barresi et al., 2015). According to GLOBOCAN 2018, CRC is the malignant tumor with the third highest incidence and the second highest mortality. It is estimated that there were more than 1.8 million new cases and 881,000 deaths caused by CRC in 2018 (Bray et al., 2018). In addition, the incidence of CRC among young adults is increasing (Benson et al., 2017), which brings a huge health burden to human beings worldwide. The prognosis of CRC varies in different countries around the world. The 5-year relative survival rate of CRC in high-income countries is close to 65%, while in low-income countries, it is less than 50% (Brenner et al., 2014). Despite the continuous development of treatment methods such as operation, chemotherapy agents, and radiotherapy, the prognosis of CRC has not been significantly improved. Recently, immunotherapy has become a promising therapeutic method for CRC patients. Unfortunately, current clinical trials show that only a few people can benefit from immunotherapy; thus, finding biomarkers that can indicate treatment response and prognosis has become an urgent problem (Piawah and Venook, 2019).
The tumor microenvironment (TME) has been proven to be involved in angiogenesis, tumor metastasis, and immune response, thereby affecting the treatment and prognosis of patients (Qi and Wu, 2019). The TME is composed of immune cells (T cells, macrophages, etc.), stromal cells (endothelial cells, etc.), and extracellular components (cytokines, hormones, etc.). Immune and stromal cells are reported to be the key carriers for the tumor microenvironment to perform multiple biological functions. For CRC, researchers have confirmed the prognostic role of tumor-infiltrating immune cells in the TME (Galon et al., 2006). Immune and stromal classification of CRC has been found to be associated with molecular subtypes and precision immunotherapy (Becht et al., 2016; Micke et al., 2021). Therefore, understanding the immune status of the TME is greatly significant for improving the treatment and prognosis of COAD.
Based on the gene expression value in the TME, Yoshihara et al. (2013) constructed a new algorithm and called it “ESTIMATE” to evaluate the proportion of stromal and immune cells in tumor tissues. Through this method, researchers have discovered diagnostic or prognostic markers of glioblastoma, cervical squamous cell carcinoma, bladder cancer, gastric cancer, etc. (Jia et al., 2018; Luo et al., 2019; Pan et al., 2019; Wang et al., 2019), as well as tumor immune-related therapeutic targets. However, the prognostic value of the TME of COAD has not yet been elucidated. In this study, we used the COAD gene expression profile data from TCGA to calculate the immune/stromal scores of COAD patients using ESTIMATE and explored the correlation of these scores with the clinical characters and overall survival of COAD patients. Subsequently, we investigated the potential prognostic genes in the TME of COAD.
Materials and Methods
Patients and Gene Expression Data
We collected the RNA expression data of 512 COAD patients from the TCGA database (https://cancergenome.nih.gov/). Clinical and pathological characteristics, including gender, age, and pathological tumor staging of all 512 COAD patients, are listed in Table 1. The log2 transformed FPKM values were used for gene expression analysis. Due to the lack of survival information of 25 patients, we used the expression and survival data of 487 patients for further analysis. In order to verify the prognostic value of genes in COAD and the relationship between genes and immune cell behavior, we obtained another group of 587 COAD patients with RNA expression profiles and clinical characters (GSE39582) from the Gene Expression Omnibus (GEO) database.
Calculating Immune/Stromal Scores and Survival Analysis
Based on the ESTIMATE algorithm in the R program (3.5.3), we obtained the immune/stromal/ESTIMATE score of each sample (Yoshihara et al., 2013). Subsequently, the degree of infiltration of immune cells was quantified by Single Sample Gene Set Enrichment Analysis (ssGSEA) (Hänzelmann et al., 2013; Xiao et al., 2020). In order to determine the optimal cut-off value of the immune/stromal score to classify participants into high-/low-score groups, R packages including “maxstat” and “survival” were used (Hothorn and Zeileis, 2008). Kaplan–Meier (KM) analysis was performed to explore the prognostic performance of the immune/stromal/ESTIMATE score, and the log rank p value was computed and showed on the survival curves. To understand the correlation of the tumor stage with the immune/stromal score, one-way ANOVA was used to test differences. The differentially expressed genes (DEGs) with a |fold change| > 1.5 and a p value <0.05 were found by SAM test, which was a statistical technique based on a t-test in R software (Tusher et al., 2001). KM and COX regression analysis were used to further evaluate the relationship between the DEGs and over survival of COAD in the TCGA and GSE39582 datasets.
Functional Prediction and PPI Network Analysis
ClueGo of the Cytosccape plug-in (Bindea et al., 2009) was performed to predict the biological function of DEGs, which could cluster genes using Gene Ontology (GO) and the Kyoto Encyclopedia of Genes and Genomes (KEGG). The protein–protein interaction (PPI) network was constructed through the STRING database (von Mering et al., 2005), and these selected genes required a confidence score ≥ 0.4 and a maximum number of interactors = 0. The visualization analysis of PPI was completed using Cytoscape software (Shannon et al., 2003). The Network Analyzer plug-in of Cytoscape was used to analyze the degree distribution of genes.
Results
Prognostic Correlation Analysis of Immune/Stromal Scores in COAD
As shown in Table 1, the median age of the 512 COAD patients in TCGA was 69, males outnumbered females, and patients without lymph node metastasis and distant metastasis (stage I and II) accounted for the majority. A total of 487 patients with complete survival information and gene expression data were studied. From the gene expression profiles, we identified 17,590 expressed genes in the 487 COAD samples. We acquired the immune, stromal, and ESTIMATE scores of each COAD patient using ESTIMATE (Supplementary Table S1). The immune scores of the 487 COAD patients ranged from −1,262.3 to 2,598.7, the stromal scores ranged from −2,543.4 to 1,622.9, and the ESTIMATE scores ranged from −3,579.2 to 3,689.2. To investigate the potential correlation between the prognosis of COAD and the immune/stromal/ESTIMATE score, we divided patients into low-score or high-score groups by the cut-off value selected by maximally selected rank statistics in the R maxstat package. Kaplan–Meier analysis revealed that the high immune score group with a score higher than −202.9 was significantly correlated with a better prognosis than the low immune score group (median survival 8.33 vs. 5.49 years, log rank p = 0.03, Figure 1A). Based on −382.6/49.5 as the selected cut-off value, the high-score groups of the stromal/ESTIMATE scores had a shorter survival (stromal: median survival 5.23 vs. 7.73 years; ESTIMATE: median survival 5.85 vs. 7.73 years, log rank p < 0.05, Figures 1B,C).
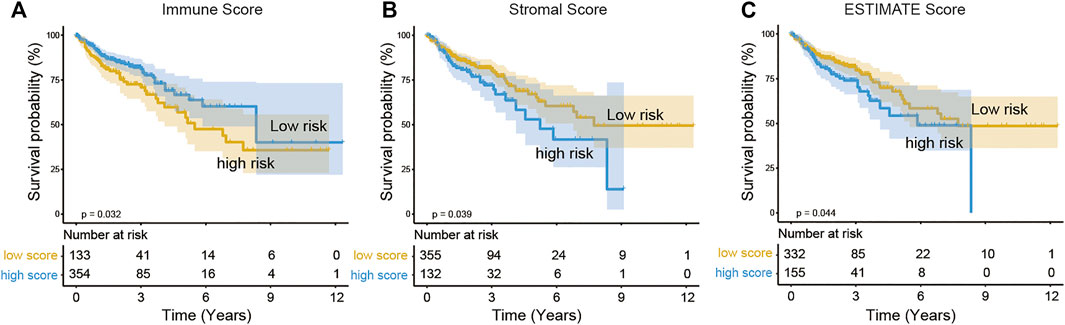
FIGURE 1. Association of immune scores (A), stromal scores (B), and ESTIMATE scores (C) with COAD overall survival. The COAD cases were divided into two groups based on their immune scores or stromal scores or ESTIMATE scores. Kaplan–Meier survival curve of OS between high and low immune/stromal/ESTIMATE score groups.
Subsequently, we investigated the association of immune/stromal/ESTIMATE scores with the COAD tumor stage and pathologic T, N, and M stages by one-way ANOVA test. As shown in Figure 2 and Supplementary Figure S1, the immune scores were significantly associated with the tumor stage and pathologic M stage (p < 0.05, Figure 2A, Supplementary Figure S1G). But the stromal scores or ESTIMATE scores were not correlated with that of COAD (p > 0.05, Figures 2B,C, Supplementary Figure S1). Then we ran Tukey’s HSD test to compare the scores between different tumor stages (Figures 2D–F) and found out that the group means of immune scores owned a significantly different value between tumor stages IV and II (p < 0.05, Figure 2D).
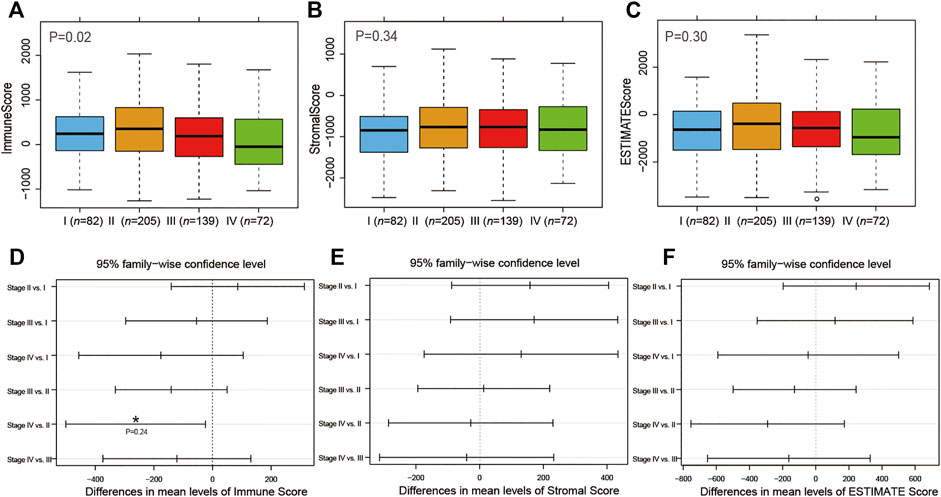
FIGURE 2. Association of tumor stage with immune (A), stromal (B), and ESTIMATE (C) scores. Tukey’s HSD test to compare the differences between different tumor stages with immune (D), stromal (E), and ESTIMATE (F) scores.
Differentially Expressed Gene Analysis in the TME of COAD
After obtaining the immune scores, we performed differentially expressed gene analysis based on the high- (n = 354) and low-score (n = 133) groups. A total of 953 DEGs were identified, of which 892 DEGs were upregulated genes and 61 DEGs were downregulated genes (Figure 3A). Meanwhile, there were 1,090 upregulated and 160 downregulated DEGs according to the comparison between high stromal score (n = 132) and low stromal score (n = 355) groups (Figure 3B). Venn diagrams indicated 663 overlapping upregulated genes and 25 overlapping downregulated genes in both immune and stromal groups (Figures 3C,D). Further analysis focused on the common DEGs.
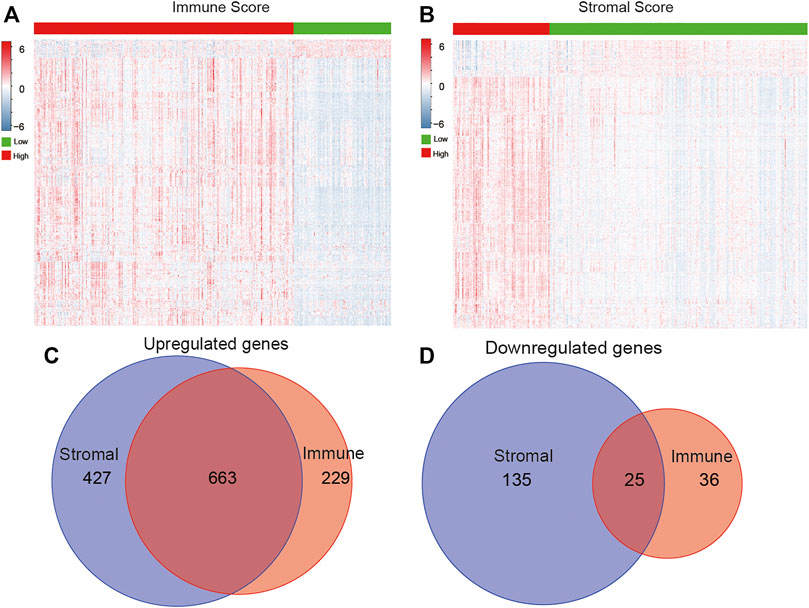
FIGURE 3. Identification of DEGs based on immune/stromal scores. Heatmap of DEGs from the low vs. high immune (A)/stromal (B) score groups (|fold change| >1.5, p < 0.05). Venn diagrams showed the number of overlapped up- or downregulated DEGs in immune score (C) and stromal score (D) groups.
Through the ClueGO annotation in Cytoscape software, we conducted GO and KEGG analysis to predict the function of the 688 intersection DEGs and found that these genes were mainly clustered in 922 GO terms and 44 KEGG pathways (Supplementary Table S2). From the aspects of biological processes (BPs), we found that these intersection DEGs were mainly enriched in cell migration, cell motility, and regulation of the immune system process. From the aspects of the cellular component (CC), these DEGs were primarily clustered in the extracellular space and the extracellular matrix. At the level of molecular function (MF), they were mainly associated with glycosaminoglycan binding, growth factor binding, and heparin binding (Figure 4A). The KEGG pathway analysis result suggested that these DEGs were mainly enriched in the toll-like receptor signaling pathway, cell adhesion molecules (CAMs), and the PI3K-Akt signaling pathway (Figure 4B).
PPI Network Construction of DEGs
The PPI network of these 688 DEGs was constructed based on the STRING database, and a total of 664 nodes and 10,015 interactions were detected (Supplementary Figure S2). We further analyzed the node degree in PPI and found that they obeyed the power-law distribution, indicating that the network was scale-free, similar to most biological networks (Figure 5A). In addition, we calculated the average path length of the PPI network, which showed that the characteristic path length of the network was much longer than that of the random network (1,000 times that of the random network, p = 0.002, Figure 5B). The most highly connected intersection DEGs were identified. Among these, IL6, FN1, PTPRC, ITGAM, CXCL8, ITGB2, CD86, MMP9, TLR2, and TYROBP were the top ten with 205, 190, 189, 177, 149, 147, 146, 142, 138, and 136 nodes, respectively (Figure 5C). So we grabbed the subnetwork of the 10 genes and found that most of them were interactive and are highly expressed in the high immune score group (Figure 5D).
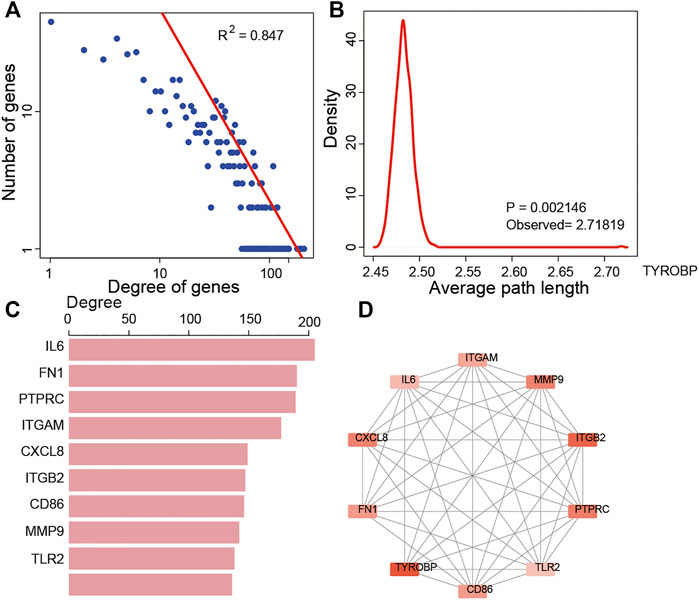
FIGURE 5. Topological features of DEGs in the PPI network. (A) View of the PPI network. (A) Degree distributions of the PPI network. (B) All degrees followed a power-law distribution and average path length distributions of the real network and 1,000 times random networks. (C) Key DEGs in the PPI network with top 10 degree distributions. (D) Subnetwork of the top 10 genes.
Prognostic Value of DEGs in COAD
The association of intersection DEGs with OS of COAD was evaluated by Kaplan–Meier and Cox regression analysis in the 487 COAD cases. Among 668 intersection DEGs, a total of 38 genes were associated with the OS (p < 0.05, Supplementary Table S3). Among the 38 prognostic DEGs, patients with high expression of CPA3, MMP12, MMP1, CXCL8, and TSPAN11 were associated with longer OS than those with low expression, while the upregulated expression of other genes were associated with unfavorable outcomes.
To further validate above results, an independent dataset including 579 patients from the GEO database (accession number GSE39582) were used to verify the prognostic genes of COAD. As a result, we found that four genes out of a total of 38 identified genes from the TCGA were significantly associated with COAD survival. These four prognostic genes were VIM, SIGLEC1, ARL4C, and CPA3. From Figure 6, we observed that high expression of VIM, SIGLEC1, and ARL4C and low expression of CPA3 were associated with poor prognosis.
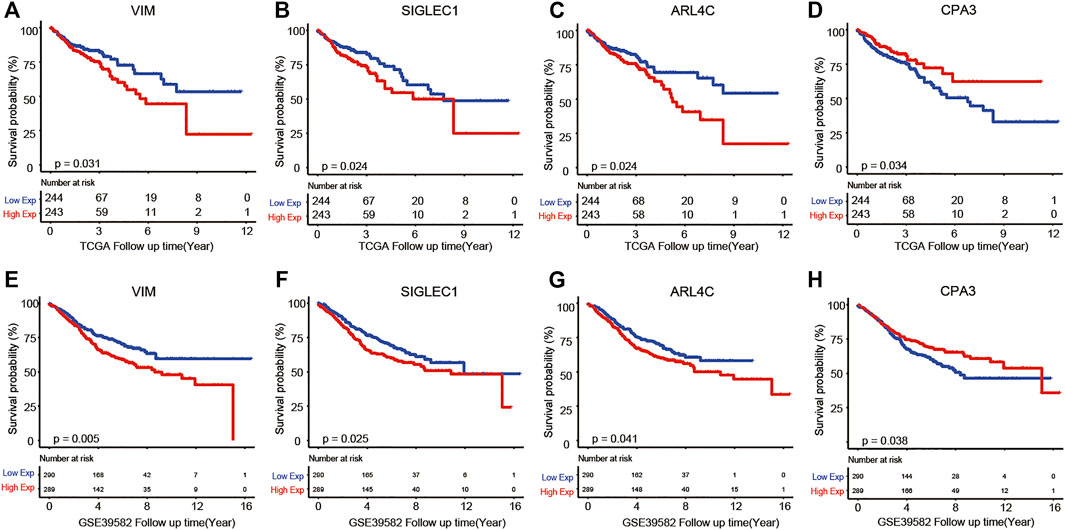
FIGURE 6. Kaplan–Meier analysis results of VIM (A,E), SIGLEC1 (B,F), ARL4C (C,G), and CPA3 (D,H) in TCGA and GSE39582 datasets.
Relationships Between the Four Prognostic Genes and Immune Cell Behavior in COAD
Next, to uncover the potential role of genes in tumor immunology, ssGSEA analysis unearthed the ratio of immune cells, and then we analyzed the correlation between the expression of these genes and immune cells by Pearson test and presented it with heatmaps based on TCGA (Figure 7A) and GSE39582 (Figure 7B) datasets. Interestingly, we observed that CPA3 was highly correlated only with mast cells (Figures 7C,D), while VIM, SIGLEC1, and ARL4C were highly correlated with macrophage, natural killer cell, regulatory T cell, T follicular helper cell, and Type 1 T helper cell in TCGA (Supplementary Figure S3) and GSE39582 (Supplementary Figure S4), simultaneously (Pearson coefficient>0.6, p < 0.001). These results suggested that the four prognostic genes may participate in cancer progression by regulating the level of immune cells in COAD (Pearson coefficient>0.6, p < 0.001).
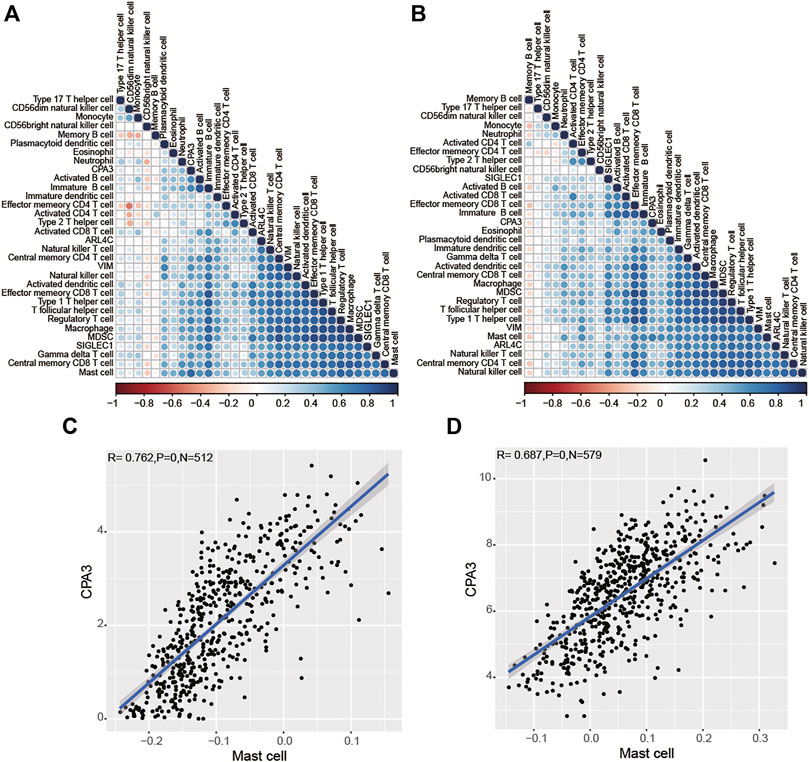
FIGURE 7. Relationships between the four prognostic genes and immune cells based on TCGA (A) and GSE39582 (B) dataset analysis. CPA3 was highly correlated with Mast cells in TCGA (C) and GSE39582 (D) datasets.
Discussion
COAD is a heterogeneous malignant tumor with widely variable prognosis (Barresi et al., 2015). Therefore, new prognostic biomarkers and theraputic methods are needed. Recently, immunotherapy has brought great hope to COAD patients, but its limited effectiveness and drug resistance are still major challenges. The TME has been reported to be implicated in the development of various tumors (Balkwill et al., 2012; Hui and Chen, 2015) and affect the treatment and prognosis of patients, but research on the TME of COAD is rare. In this study, we identified the correlation of the immune/stromal scores with the survival of COAD. Moreover, we screened out a total of 688 DEGs from high vs. low immune/stromal score groups and found four genes with prognostic value in the TME, which have the potential ability to serve as molecular biomarkers of COAD.
After analyzing the profiles of 487 COAD patients through the ESTIMATE algorithm, we found that a high immune score was correlated with a high overall survival rate of COAD, which was identical to the results reported in other tumors such as hepatocellular carcinoma (Pan et al., 2020), prostate cancer (Sun et al., 2020), and endometrial cancer (Chen et al., 2020). This correlation result indicates that immune cell infiltration is beneficial to the prognosis of COAD. Similarly, Michael J et al. found that high tumor-associated macrophage infiltration in CRC was associated with better prognosis (Cavnar et al., 2017). Franck et al. discovered that cytotoxic (CD8) and memory (CD45RO) T cells could predict better clinical outcomes of CRC patients (Pagès et al., 2009). Other researchers have confirmed that immune cells are prognostic factors for CRC (Galon et al., 2006). On the other hand, our study also discovered that the high stromal/ESTIMATE scores were correlated with poor prognosis, indicating that the stromal cells in the TME are indicators of unfavorable clinical outcome for CRC. Consistent with our findings, the high expression of cancer-associated fibroblasts (CAFs, one group of stromal cells) was found to be associated with the poor prognosis of untreated CRC (Isella et al., 2015). Moreover, some researchers have found that stromal cells in the TME of colon cancer have a key role in inhibiting tumor immune response and enhancing tumor malignant progression (O’Malley et al., 2018).
When analyzing the correlation between clinical parameters and immune/stromal scores, we found significant differences in the immune scores of COAD patients with different tumor stages. Then, we obtained 688 DEGs through analyzing DEGs that appear in both groups with high and low immune/stromal scores. Function analysis found that these intersection DEGs were enriched in the immune system process, cell migration, cell motility, growth factor binding and extracellular matrix, Toll-like receptor signaling pathway, NF-kappa B signaling pathway, and PI3K-Akt signaling pathway, which were closely related to tumor metastasis. Moreover, we identified that the most highly connected intersection DEGs in the TME of COAD were IL6. IL6 (Interleukin-6), one of the major cytokines in the TME, has been reported to promote tumor progression including apoptosis, proliferation, invasiveness, and metastasis via regulating multiple key cell signaling pathways (Kumari et al., 2016). All the above findings indicated that the TME of COAD had an important role in tumor progress and outcome.
Subsequently, Kaplan–Meier and COX analysis found that 38 TME-related DEGs were significantly correlated with the OS of COAD patients from TCGA database and validated four genes (VIM, SIGLEC1, ARL4C, and CPA3) in the GEO dataset. VIM (vimentin) gene encodes type III intermediate filament protein and is expressed in most cell types, particularly tumor cells. VIM has an important function of regulating cell migration (Battaglia et al., 2018). It has been reported that the abnormally high expression of vimentin in various epithelial cancers including prostate cancer, gastrointestinal tumors, and breast cancer is closely related to tumor growth, invasion, and poor prognosis (Satelli and Li, 2011). In CRC cells, researchers found that siRNA knockdown of VIM expression could reduce cell migration and invasiveness (McInroy and Määttä, 2007). Consistent with the results of the above studies, our study found that high expression of VIM indicated poor prognosis for patients with COAD. SIGLEC1, also known as CD169, encodes a type I transmembrane protein expressed on macrophages. Studies have shown that CD169+ macrophages are a favorable prognostic indicator for bladder cancer (Asano et al., 2018) and hepatocellular carcinoma (Zhang et al., 2016). These results in other tumors are contrary to this article, so it is necessary to further clarify the prognostic significance and specific mechanisms of SIGLEC1. ARL4C (ADP-ribosylation factor-like protein 4C) is a target gene for both Wnt/β-catenin and epidermal growth factor/Ras signaling, and its expression is reported to promote cellular migration and proliferation, thereby indicating its involvement in tumorigenesis. It has been found that ARL4C is overexpressed in colorectal cancers and plays a pivotal role in the progression of CRC (Fujii et al., 2015; Chen et al., 2016). CPA3 (carboxypeptidase A3) is a member of the metallocarboxypeptidase family and can be expressed in many cell types, especially basophils and mast cells. There are few studies on the expression of CPA3 in tumors and its prognostic significance. Our study found that CPA3 was a protective factor of COAD, and high expression of CPA3 was associated with better survival rates.
In conclusion, this study provides an attempt at understanding the role of immune/stromal cells and genes in the TME of COAD and confirms that the composition of TME affects the clinical outcomes of COAD patients. Moreover, four TME-related genes have been identified, which could be used as new prognostic biomarkers and targets for immunotherapy.
Data Availability Statement
Publicly available datasets were analyzed in this study. The data can be found here. Publicly available datasets can be found here: https://gdc-hub.s3.us-east-1.amazonaws.com/download/TCGA-COAD.htseq_fpkm-uq.tsv.gz; https://ftp.ncbi.nlm.nih.gov/geo/series/GSE39nnn/GSE39582/matrix/GSE39582_series_matrix.txt.gz.
Author Contributions
The authors contributed in the following way: SP: data collection, data analysis, interpretation, and drafting; SY: study design, study supervision, and final approval of the manuscript; TT, YW, LZ, and ZS: technical support and critical revision of the manuscript. All authors read and approved the final manuscript.
Funding
This work was supported by the Wenzhou Basic Scientific Research Project (grant number: Y2020956).
Conflict of Interest
The authors declare that the research was conducted in the absence of any commercial or financial relationships that could be construed as a potential conflict of interest.
Publisher’s Note
All claims expressed in this article are solely those of the authors and do not necessarily represent those of their affiliated organizations, or those of the publisher, the editors, and the reviewers. Any product that may be evaluated in this article, or claim that may be made by its manufacturer, is not guaranteed or endorsed by the publisher.
Supplementary Material
The Supplementary Material for this article can be found online at: https://www.frontiersin.org/articles/10.3389/fgene.2021.778153/full#supplementary-material
Abbreviations
COAD, colon adenocarcinoma; KM, Kaplan–Meier; TME, tumor microenvironment; TCGA, the Cancer Genome Atlas; PPI, protein–protein interaction network.
References
Asano, T., Ohnishi, K., Shiota, T., Motoshima, T., Sugiyama, Y., Yatsuda, J., et al. (2018). CD 169‐positive Sinus Macrophages in the Lymph Nodes Determine Bladder Cancer Prognosis. Cancer Sci. 109, 1723–1730. doi:10.1111/cas.13565
Balkwill, F. R., Capasso, M., and Hagemann, T. (2012). The Tumor Microenvironment at a Glance. J. Cel. Sci. 125, 5591–5596. doi:10.1242/jcs.116392
Barresi, V., Reggiani Bonetti, L., Ieni, A., Caruso, R. A., and Tuccari, G. (2015). Histological Grading in Colorectal Cancer: New Insights and Perspectives. Histol. Histopathol 30, 1059–1067. doi:10.14670/HH-11-633
Battaglia, R. A., Delic, S., Herrmann, H., and Snider, N. T. (2018). Vimentin on the Move: New Developments in Cell Migration. F1000Res 7, F1000Res. doi:10.12688/f1000research.15967.1
Becht, E., de Reyniès, A., Giraldo, N. A., Pilati, C., Buttard, B., Lacroix, L., et al. (2016). Immune and Stromal Classification of Colorectal Cancer Is Associated with Molecular Subtypes and Relevant for Precision Immunotherapy. Clin. Cancer Res. 22, 4057–4066. doi:10.1158/1078-0432.ccr-15-2879
Benson, A. B., Venook, A. P., Cederquist, L., Chan, E., Chen, Y.-J., Cooper, H. S., et al. (2017). Colon Cancer, Version 1.2017, NCCN Clinical Practice Guidelines in Oncology. J. Natl. Compr. Canc Netw. 15, 370–398. doi:10.6004/jnccn.2017.0036
Bindea, G., Mlecnik, B., Hackl, H., Charoentong, P., Tosolini, M., Kirilovsky, A., et al. (2009). ClueGO: a Cytoscape Plug-In to Decipher Functionally Grouped Gene Ontology and Pathway Annotation Networks. Bioinformatics 25, 1091–1093. doi:10.1093/bioinformatics/btp101
Bray, F., Ferlay, J., Soerjomataram, I., Siegel, R. L., Torre, L. A., and Jemal, A. (2018). Global Cancer Statistics 2018: GLOBOCAN Estimates of Incidence and Mortality Worldwide for 36 Cancers in 185 Countries. CA: a Cancer J. clinicians 68, 394–424. doi:10.3322/caac.21492
Brenner, H., Kloor, M., and Pox, C. P. (2014). Colorectal Cancer. The Lancet 383, 1490–1502. doi:10.1016/s0140-6736(13)61649-9
Cavnar, M. J., Turcotte, S., Katz, S. C., Kuk, D., Gönen, M., Shia, J., et al. (2017). Tumor-Associated Macrophage Infiltration in Colorectal Cancer Liver Metastases Is Associated with Better Outcome. Ann. Surg. Oncol. 24, 1835–1842. doi:10.1245/s10434-017-5812-8
Chen, P., Yang, Y., Zhang, Y., Jiang, S., Li, X., and Wan, J. (2020). Identification of Prognostic Immune-Related Genes in the Tumor Microenvironment of Endometrial Cancer. Aging 12, 3371–3387. doi:10.18632/aging.102817
Chen, X., Su, Z., Wang, S., and Xu, H. (2016). Clinical and Prognostic Significance of Arl4c Expression in Colorectal Cancer. Cbm 16, 253–257. doi:10.3233/cbm-150562
Fujii, S., Matsumoto, S., Nojima, S., Morii, E., and Kikuchi, A. (2015). Arl4c Expression in Colorectal and Lung Cancers Promotes Tumorigenesis and May Represent a Novel Therapeutic Target. Oncogene 34, 4834–4844. doi:10.1038/onc.2014.402
Galon, J., Costes, A., Sanchez-Cabo, F., Kirilovsky, A., Mlecnik, B., Lagorce-Pagès, C., et al. (2006). Type, Density, and Location of Immune Cells within Human Colorectal Tumors Predict Clinical Outcome. Science 313, 1960–1964. doi:10.1126/science.1129139
Hänzelmann, S., Castelo, R., and Guinney, J. (2013). GSVA: Gene Set Variation Analysis for Microarray and RNA-Seq Data. BMC bioinformatics 14, 7. doi:10.1186/1471-2105-14-7
Hothorn, T., and Zeileis, A. (2008). Generalized Maximally Selected Statistics. Biometrics 64, 1263–1269. doi:10.1111/j.1541-0420.2008.00995.x
Hui, L., and Chen, Y. (2015). Tumor Microenvironment: Sanctuary of the Devil. Cancer Lett. 368, 7–13. doi:10.1016/j.canlet.2015.07.039
Isella, C., Terrasi, A., Bellomo, S. E., Petti, C., Galatola, G., Muratore, A., et al. (2015). Stromal Contribution to the Colorectal Cancer Transcriptome. Nat. Genet. 47, 312–319. doi:10.1038/ng.3224
Jia, D., Li, S., Li, D., Xue, H., Yang, D., and Liu, Y. (2018). Mining TCGA Database for Genes of Prognostic Value in Glioblastoma Microenvironment. Aging 10, 592–605. doi:10.18632/aging.101415
Kumari, N., Dwarakanath, B. S., Das, A., and Bhatt, A. N. (2016). Role of Interleukin-6 in Cancer Progression and Therapeutic Resistance. Tumor Biol. 37, 11553–11572. doi:10.1007/s13277-016-5098-7
Luo, Y., Zeng, G., and Wu, S. (2019). Identification of Microenvironment-Related Prognostic Genes in Bladder Cancer Based on Gene Expression Profile. Front. Genet. 10, 1187. doi:10.3389/fgene.2019.01187
McInroy, L., and Määttä, A. (2007). Down-regulation of Vimentin Expression Inhibits Carcinoma Cell Migration and Adhesion. Biochem. Biophysical Res. Commun. 360, 109–114. doi:10.1016/j.bbrc.2007.06.036
Micke, P., Strell, C., Mattsson, J., Martín-Bernabé, A., Brunnström, H., Huvila, J., et al. (2021). The Prognostic Impact of the Tumour Stroma Fraction: A Machine Learning-Based Analysis in 16 Human Solid Tumour Types. EBioMedicine 65, 103269. doi:10.1016/j.ebiom.2021.103269
O'Malley, G., Treacy, O., Lynch, K., Naicker, S. D., Leonard, N. A., Lohan, P., et al. (2018). Stromal Cell PD-L1 Inhibits CD8+ T-Cell Antitumor Immune Responses and Promotes Colon Cancer. Cancer Immunol. Res. 6, 1426–1441. doi:10.1158/2326-6066.cir-17-0443
Pagès, F., Kirilovsky, A., Mlecnik, B., Asslaber, M., Tosolini, M., Bindea, G., et al. (2009). In Situ cytotoxic and Memory T Cells Predict Outcome in Patients with Early-Stage Colorectal Cancer. Jco 27, 5944–5951. doi:10.1200/jco.2008.19.6147
Pan, L., Fang, J., Chen, M.-Y., Zhai, S.-T., Zhang, B., Jiang, Z.-Y., et al. (2020). Promising Key Genes Associated with Tumor Microenvironments and Prognosis of Hepatocellular Carcinoma. Wjg 26, 789–803. doi:10.3748/wjg.v26.i8.789
Pan, X.-B., Lu, Y., Huang, J.-L., Long, Y., and Yao, D.-S. (2019). Prognostic Genes in the Tumor Microenvironment in Cervical Squamous Cell Carcinoma. Aging 11, 10154–10166. doi:10.18632/aging.102429
Piawah, S., and Venook, A. P. (2019). Targeted Therapy for Colorectal Cancer Metastases: A Review of Current Methods of Molecularly Targeted Therapy and the Use of Tumor Biomarkers in the Treatment of Metastatic Colorectal Cancer. Cancer 125, 4139–4147. doi:10.1002/cncr.32163
Qi, D., and Wu, E. (2019). Cancer Prognosis: Considering Tumor and its Microenvironment as a Whole. EBioMedicine 43, 28–29. doi:10.1016/j.ebiom.2019.04.031
Satelli, A., and Li, S. (2011). Vimentin in Cancer and its Potential as a Molecular Target for Cancer Therapy. Cell. Mol. Life Sci. 68, 3033–3046. doi:10.1007/s00018-011-0735-1
Shannon, P., Markiel, A., Ozier, O., Baliga, N. S., Wang, J. T., Ramage, D., et al. (2003). Cytoscape: a Software Environment for Integrated Models of Biomolecular Interaction Networks. Genome Res. 13, 2498–2504. doi:10.1101/gr.1239303
Siegel, R. L., Miller, K. D., Fedewa, S. A., Ahnen, D. J., Meester, R. G. S., Barzi, A., et al. (2017). Colorectal Cancer Statistics, 2017. CA: a Cancer J. clinicians 67, 177–193. doi:10.3322/caac.21395
Siegel, R. L., Miller, K. D., Goding Sauer, A., Fedewa, S. A., Butterly, L. F., Anderson, J. C., et al. (2020). Colorectal Cancer Statistics. Hoboken: CA: a cancer journal for clinicians.
Sun, W., Shi, H., Yuan, Z., Xia, L., Xiang, X., Quan, X., et al. (2020). Prognostic Value of Genes and Immune Infiltration in Prostate Tumor Microenvironment. Front. Oncol. 10, 584055. doi:10.3389/fonc.2020.584055
Tusher, V. G., Tibshirani, R., and Chu, G. (2001). Significance Analysis of Microarrays Applied to the Ionizing Radiation Response. Proc. Natl. Acad. Sci. 98, 5116–5121. doi:10.1073/pnas.091062498
von Mering, C., Jensen, L. J., Snel, B., Hooper, S. D., Krupp, M., Foglierini, M., et al. (2005). STRING: Known and Predicted Protein-Protein Associations, Integrated and Transferred across Organisms. Nucleic Acids Res. 33, D433–D437. doi:10.1093/nar/gki005
Wang, H., Wu, X., and Chen, Y. (2019). Stromal-Immune Score-Based Gene Signature: A Prognosis Stratification Tool in Gastric Cancer. Front. Oncol. 9, 1212. doi:10.3389/fonc.2019.01212
Xiao, B., Liu, L., Li, A., Xiang, C., Wang, P., Li, H., et al. (2020). Identification and Verification of Immune-Related Gene Prognostic Signature Based on ssGSEA for Osteosarcoma. Front. Oncol. 10, 607622. doi:10.3389/fonc.2020.607622
Yoshihara, K., Shahmoradgoli, M., Martínez, E., Vegesna, R., Kim, H., Torres-Garcia, W., et al. (2013). Inferring Tumour Purity and Stromal and Immune Cell Admixture from Expression Data. Nat. Commun. 4, 2612. doi:10.1038/ncomms3612
Keywords: tumor microenvironment, immune/stromal scores, colon adenocarcinoma, protein–protein interaction network, OMICS data
Citation: Pan S, Tang T, Wu Y, Zhang L, Song Z and Yu S (2022) Identification and Validation of Immune-Related Prognostic Genes in the Tumor Microenvironment of Colon Adenocarcinoma. Front. Genet. 12:778153. doi: 10.3389/fgene.2021.778153
Received: 16 September 2021; Accepted: 07 December 2021;
Published: 03 January 2022.
Edited by:
Luis Zapata, Institute of Cancer Research (ICR), United KingdomReviewed by:
Xiaoxiao Sun, University of Arizona, United StatesEleonora Lusito, San Raffaele Telethon Institute for Gene Therapy (SR-Tiget), Italy
Ahmet Acar, Middle East Technical University, Turkey
Copyright © 2022 Pan, Tang, Wu, Zhang, Song and Yu. This is an open-access article distributed under the terms of the Creative Commons Attribution License (CC BY). The use, distribution or reproduction in other forums is permitted, provided the original author(s) and the copyright owner(s) are credited and that the original publication in this journal is cited, in accordance with accepted academic practice. No use, distribution or reproduction is permitted which does not comply with these terms.
*Correspondence: Sisi Yu, zsy1737@126.com