- Nuritas Ltd., Dublin, Ireland
Scientific research consistently demonstrates that diseases may be delayed, treated, or even prevented and, thereby, health may be maintained with health-promoting functional food ingredients (FFIs). Consumers are increasingly demanding sound information about food, nutrition, nutrients, and their associated health benefits. Consequently, a nutrition industry is being formed around natural foods and FFIs, the economic growth of which is increasingly driven by consumer decisions. Information technology, in particular artificial intelligence (AI), is primed to vastly expand the pool of characterised and annotated FFIs available to consumers, by systematically discovering and characterising natural, efficacious, and safe bioactive ingredients (bioactives) that address specific health needs. However, FFI-producing companies are lagging in adopting AI technology for their ingredient development pipelines for several reasons, resulting in a lack of efficient means for large-scale and high-throughput molecular and functional ingredient characterisation. The arrival of the AI-led technological revolution allows for the comprehensive characterisation and understanding of the universe of FFI molecules, enabling the mining of the food and natural product space in an unprecedented manner. In turn, this expansion of bioactives dramatically increases the repertoire of FFIs available to the consumer, ultimately resulting in bioactives being specifically developed to target unmet health needs.
Functional Food Ingredients
Consumer Demand for Science-Backed Healthy Ingredients and Natural Foods
With the recent exponential surge in information technology advancements, today’s consumers demand instant access to deeper product understanding unlike any generation before. For the food and nutrition market, this means that the next wave of economic growth will be driven by nutrition-based technology that empowers consumers to take better control of their own health by accessing validated information about beneficial effects of natural ingredients and supplements for various conditions (Doub et al., 2015; Chen et al., 2018; Littlejohn et al., 2018). Market trends are demonstrating that consumers are 1) increasingly expecting robust scientific evidence for nutritional claims (Talati et al., 2016; Lin, Shih, and Lin 2017); 2) educating themselves to understand the meaning and validity of Functional Food Ingredients (FFIs) and their associated health claims (A. Zhang et al., 2020; Hartmann et al., 2018); 3) becoming confident to express their opinion regarding the safety and benefits of food products (Savchenko et al., 2019); and 4) identifying which food characteristics, such as food naturalness and sustainability, are important to them (Román, Sánchez-Siles, and Siegrist 2017). In particular, there is a need for standardisation and transparency when it comes to health claims for additives produced by food and nutrition companies. For example, E-numbers (codes used to describe substances used as food additives throughout the European Union) are a set of standardised codes brought in to ensure uniformity in additive description and as studies are showing consumers prefer simpler health claims (Lynam, 2011), an approach should be developed to standardise health claims, similar to E-numbers, which is already an active area of research (Gallagher et al., 2011). Ultimately, consumers are currently pushing for more natural products, as some of the additives used in food processing and preservation have raised health concerns (Winkler et al., 2016; Younes et al., 2018; Partridge et al., 2019) and triggered negative consumer perception (Buchler et al., 2010; Bearth et al., 2014; Bärebring et al., 2020; Jansen et al., 2020).
Functional Food Ingredients and Health
Lifestyle-associated complex diseases, especially metabolic conditions such as diabetes and obesity (Baboota et al., 2013; Rebello et al., 2014; Gentile et al., 2018), do not conform to the traditional “one disease one drug target” paradigm (Hopkins 2008). Moreover, these conditions are typically chronic (Bailes 2002; Bray, Kim et al., 2017), and are amenable to dietary therapy (Barnard et al., 2009; Sami et al., 2017; Zou et al., 2018; Castellana et al., 2019; Estruch and Ros 2020). They cannot be sustainably addressed by pharmaceutical means only, i.e., through “repair after onset”; rather, prevention and nutritional intervention are necessary complementary strategies to alleviate the public health burden of lifestyle-associated chronic disease (Mattei et al., 2015; S.; Sharma et al., 2010; Turner-McGrievy et al., 2017; Schwarz et al., 2008). Large-scale clinical studies repeatedly demonstrate that foods affect the risk of incidence and mortality from common chronic diseases that are rapidly rising in society (L. Wang et al., 2018; De Koning et al., 2012; Kenfield et al., 2014; Tasevska et al., 2014; Salas-Salvadó et al., 2014; Esposito et al., 2017; Dicker et al., 2018; Muraki et al., 2013), thus bearing the potential to improve global population health in a cost-effective manner (Eussen et al., 2011; Yang et al., 2018; J.; Wang et al., 2020). For example, FFIs can mitigate the effects of chronic, unresolved inflammation (Rein et al., 2019; K.; Kennedy et al., 2020a) that can cause damage to healthy cells, tissues and organs, and FFIs can actually maintain and restore homeostatic balance (Stampfer et al., 2000; Muraki et al., 2013; Valls-Pedret et al., 2015; Alkhatib et al., 2017). While pharmacological compounds typically act on disease states after onset, FFIs exert multiple, subtle, long-term effects in a concerted fashion that delay or prevent such disease states. The benefits of FFIs become evident upon chronic consumption of a blend of bioactives, where the (food) matrix matters for both bioavailability and bioefficacy (Kussmann et al., 2007; Holst and Williamson 2008).
So which factors are impeding a more rapid and systematic progress in translational nutrition studies for health maintenance and disease prevention?
a) Study design: One important explanation is inconclusive and at times contradictory scientific findings as to the health effects of various functional foods and ingredients (Ferreira et al., 2016; Hungin et al., 2018). These partly result from poor comparability of nutritional intervention studies, which are in turn due to lack of standards for diets and study designs (Kaput et al., 2015). Therefore, standardised studies performed across different ethnicities with biochemically defined diets and ingredients are required (Kaput et al., 2015).
b) FFIs discovery and characterisation: a further key limitation in translational nutritional studies is the incomplete biochemical and biological characterisation of active ingredients and the serendipitous discovery process for natural ingredients with health benefits.
This second issue can be effectively addressed with the adoption of AI into the discovery and characterisation pipeline which is the focus of this paper.
Characterisation of Functional Food Ingredients—For a Healthier and More Sustainable Food Chain
Customarily, characterisation comes after the identification of a FFI and encompasses the determination and description of biochemical, biophysical, and biological properties of the active molecule(s) with all of these, and especially the latter, allowing for assignment of health benefits to FFIs. Such characterisation is usually performed with bioanalytical methods in the “wet laboratory” and provided information about key properties of FFIs when administered as a dietary component or as a supplement. These properties concern safety profile, stability after (oral) ingestion and across gastro-intestinal digestion, amenability to active or passive transport from the gut lumen across the brush border membrane into the blood stream (systemic bioavailability), and availability in the target tissue (local bioavailability). Ultimately, this information provides a comprehensive understanding of mechanism-of-action, overall bioavailability, bioefficacy and therefore dosage of FFIs. This fundamental understanding of the ingredient’s molecular properties and functions informs on:
- Reproducible analytical and production methods.
-Application of the ingredient within a diet or as a supplement.
- An efficient route to clinical trials.
- Regulatory requirements.
- Further scientific research on the ingredient’s health effects.
In addition to the discovery and validation of new health-beneficial FFIs or the assignment of new benefits to existing FFIs, the above described systematic characterisation also enables many of the risk-associated food product additives to be replaced by natural food-derived compounds, in particular peptides, resulting in a safer food chain (Scotter 2011; Schweiggert 2018). Such food additives typically confer stability for longer shelf-life, and sensorial attributes like flavour, texture, and colour to the food product (Parmar, Angad, and Gupta Phutela 2015; Solymosi et al., 2015; Taghvaei, 2015). Both a strong consumer demand and compelling scientific reasons have triggered the replacement of artificial, synthetic food additives by natural, typically plant-derived, alternatives and, thereby, reduced the necessity for potentially harmful food additives that are typically the source of consumer concern (Van Gunst and Roodenburg, 2019; Poortvliet and Hartemink, 2013).
Given the obvious scientific advantages and consumer needs, why has FFI characterisation science not progressed at speed? Notably, there are a number of challenges in characterising FFIs.
First, from an analytical perspective, characterisation of bioactive food ingredients requires expensive and specialized instrumentation and methods to identify, quantify and biochemically characterise FFIs and, therefore, quality control standards need to be established. Numerous compound-specific bioanalytical methods and assays have been developed for the micronutrient classes of vitamins (Mitić et al., 2011; Nannapaneni et al., 2017; Y.; Zhang et al., 2019), essential fatty (Ichihara et al., 1996; Birjandi et al., 2014; Dołowy and Pyka, 2015) and amino acids (Fonseca et al., 2018; Virág et al., 2020) and minerals (trace elements) (Carapelli et al., 2020; Tempesta et al., 2020). While those methods have proven to be precise, they typically represent “one-off” methods, and are neither versatile nor high-throughput (Höller et al., 2018). These traditional methods typically rely on multi-step fractionation, separation and isolation of bioactive ingredients via size exclusion (X. Wang et al., 2017; Gallego et al., 2018), ion exchange (Mukherjee et al., 2016; Acquah et al., 2019), or reversed-phase high-performance liquid chromatography (Bah et al., 2016; Liu et al., 2018), coupled to compound detection, typically by ultraviolet (UV) absorption (Brodbelt, 2016). Due to the lack of compound-specific detection when using UV, the final confirmation of bioactive compound identity relies on the employment of internal or external standards, adding cost and time to each analysis (Schnatbaum et al., 2020). Mass spectrometry coupled with liquid chromatography (LC-MS/MS) has replaced or is replacing most of those methods because of its universal applicability to virtually any kind of biochemical compound, the possibility of analyte multiplexing in one run, speed of operation, and the capability of de novo identification and structure elucidation of biochemical compounds, largely enabled by MS/MS fragmentation of the molecule of interest (Tribalat et al., 2006). LC-MS/MS is particularly suited for the analysis of bioactive food peptides, because of its high-throughput capability of sequencing and quantifying peptides (Bronsema et al., 2013; “Simplification of Complex Peptide Mixtures for Proteomic Analysis: Reversible Biotinylation of Cysteinyl Peptides—Spahr et al., 2000—ELECTROPHORESIS—Wiley Online Library,” n.d.). Hence, peptidomics, and more specifically food peptidomics, have evolved within the overarching mother discipline of proteomics as the platform for comprehensive characterisation of the protein and peptide complement in both food raw material and products (Panchaud et al., 2012). Similarly, high-throughput and paralleled LC-MS/MS methods have recently been developed for the comprehensive profiling of essential nutrients such as vitamins (Nagy et al., 2007), essential fatty (Hewawasam et al., 2017; Serafim et al., 2019) and amino acids (Le et al., 2014; Kambhampati et al., 2019). The task of identifying and quantifying minerals (or trace elements) as a further complement of essential nutrients, requires even more sophisticated analysis with inductively-coupled plasma mass spectrometry having more recently been established as the state-of-the-art for such purpose (Konz et al., 2017).
Second, from a biochemical and biological perspective, the molecular food characterisation process involves the disentanglement of millions of compounds present at different concentrations in the natural source, which essentially makes the process akin to space exploration at molecular level. For example, curcumin is a polyphenol found in Curcuma species. Such species are considered functional food that exhibit moderate anti-oxidant and anti-inflammatory effects in vitro and yet, due to the complexity of the curcuma compound class, individual molecules responsible for these activities have not yet been identified (Schaffer et al., 2015; Hewlings and Douglas, 2017). Conversely, even if a potential bioactive within a functional ingredient is identified, assessing stability and bioavailability, attributing functionality, and validating effects in vitro/in vivo has proven to be difficult (Corrochano et al., 2019; Feng et al., 2019). The latter challenge of assigning a specific effect to a molecule is characteristic for food bioactives, because—unlike pharmaceutical compounds—food bioactives exert multiple, subtle, long-term effects in a concerted fashion, rather than a single, strong, immediate effect conferred by a single molecule (Cal et al., 2020; Corrochano et al., 2021). In addition, the food matrix adds to the mode-of-action of food bioactives (Udenigwe and Fogliano, 2017; Sun et al., 2020). Consequently, the attempt to isolate both the active principle and its effect “in the same fraction” often fails because the “purer” the compound the smaller the effect (Papetti 2012).
Traditional Ingredient Discovery and Characterisation
Health-augmenting bioactive components of natural products have traditionally been discovered serendipitously, in an ad hoc, hazardous fashion. For example, the discovery and establishment of essential nutrients such as vitamins (from “vital amines”, i.e. initially thought of as amine molecules essential to sustain life) took decades over the 20th century (DeLuca 2016; Jones, 2018; Cerullo et al., 2020) and, in turn, excessive financial resources had to be invested to proceed from discovery via validation to production (Acevedo-Rocha et al., 2019). Often, the absence of an essential nutrient has been observed to be associated with a disease and this disease has then been causally related to a deficiency syndrome. For example, the high occurrence of scurvy among sailing seafarers could be attributed to lack of vitamin C over long periods of time, which was due to the absence of fruits from their diets (Magiorkinis et al.2011). Conversely, the presence of certain dietary components has been correlated with health benefits. For example, Mediterranean diets are typically rich in olive oil and, therefore, in monounsaturated fatty acids, and a causal relationship with reduced incidence of cardiovascular disease in Mediterranean populations has been postulated (Lorgeril and Salen, 2007; Massaro et al., 2010; De). Notably, many of these correlational observations have to date still not been solidified into causality between nutrient (un)availability and health/disease condition—simply because of lacking directional relationships between biochemically characterised diets and bioactive ingredients on the one hand and health outcomes in humans on the other hand (Kussmann et al., 2007). Another, much more recent example of an initially serendipitous functional ingredient discovery, is human milk oligosaccharides (HMO) that were first discovered ∼1930 when a human milk carbohydrate fraction named “gynolactose” was identified [the discovery process is reviewed in Bode (2012)]. This discovery established the foundation for a century of research (Kunz, 2012), but it is only in recent years that this effort has delivered decisive data describing the benefits of HMOs in the modification of intestinal microbiota (LoCascio et al., 2007; Asakuma et al., 2011; Hoeflinger et al., 2015; Garrido et al., 2016), the anti-adhesive effects against pathogens (Idänpään-Heikkilä et al., 1997; Ruiz-Palacios et al., 2003; Angeloni et al., 2005; Morrow et al., 2005; Yu et al., 2016), the modulation of the intestinal epithelial cell response (Kuntz et al., 2009; Holscher et al., 2014), and the development of the immune system (Castillo-Courtade et al., 2015; Goehring et al., 2016; He et al., 2016; Comstock et al., 2017; Donovan and Comstock 2017; Dicker et al., 2018). In turn, driven by increased consumer interest in improving gut health and in the consumption of health-promoting dietary supplements (“Human Milk Oligosaccharides Market Size | Industry Report, 2027, 2021”), the emerging global HMO market value is expected to increase five-fold in the present decade (“Global Human Milk Oligosaccharides Market Expected to Grow with a CAGR of 21% During the Forecast Period, 2018–2027—ResearchAndMarkets.Com | Business Wire, 2020”). Further exponential growth in the HMO market is not being hindered by lack of demand, but limited primarily by lack of technology for large-scale production, in addition to high R&D cost and stringent government regulations (“Human Milk Oligosaccharides Market Size | Industry Report, 2027, 2021”).
These examples of serendipitous ingredient discovery demonstrate the urgent need for a more efficient technology-aided functional food ingredient characterisation pipeline. As discussed above, the first big technological revolution in bioactive compound—and also functional ingredient—discovery and characterisation came with the advent of high-throughput (HTP) screening. With the richness of biofunctionalities of compounds present in the natural product and food space and the resulting potential for therapeutic effects in mind, these platforms were built to enable high-throughput fractionation, identification, and quantification of (expected to be novel) natural bioactives. The development was catalysed by a combination of technological breakthroughs in miniaturised and multiplexed separation (Chu et al., 2006), automated liquid handling (robotics) (Chow et al., 2018), more sensitive and versatile detection techniques, such as MS, and advanced bioinformatics and in silico techniques to interpret MS data (Li-Chan and Eunice, 2015). Despite notable successes, especially in drug discovery (Chan et al., 2010; Takebe et al., 2018), the yield of new bioactive food compounds and functional ingredients has been limited, because of the particular challenge with food bioactives acting together and within the food matrix, as discussed above.
The integration of these new “wet laboratory” technologies such as mass spectrometry and HTP screening into traditional ingredient discovery and characterisation has certainly advanced the field (Figure 1). However, due to still high costs for equipment and analysis execution, this traditional approach of “food material fractionation → bioactivity screening → identification of active fraction(s) → ingredient identification within active fraction → functional ingredient characterisation → benefit assignment” has not delivered on rapid expansion of the pool of truly novel, disease-modifying and health-maintaining molecules available to consumers: it is simply not viable to experimentally unravel and confirm the effect of all molecules within natural products for multiple therapeutic benefits. Moreover, employing this traditional approach, health claims are assigned only after bioactivity has been established: this approach does not start with a health benefit and consumer need to be addressed, rather it searches for an application once the fortuitous new activities of the bioactives have been identified. As a result, to date only a limited number of bioactive ingredients with true life-changing properties, such as the essential micronutrients vitamins (Jacobson and Jacobson, 2018; Maqbool and Aslam, 2018), omega-3 and -6 fatty acids (Thomas et al., 2009; Watanabe and Tatsuno, 2017; Shahidi and Ambigaipalan, 2018) or phytonutrients such as polyphenols (Nagy et al., 2009) and anthocyanins (Speer et al., 2020; Tena et al., 2020), with the latter two not being classified as “nutrients”, have been characterised and successfully marketed. In view of this, identifying a malleable target in relation to a health claim may be a more beneficial approach to discovery, and one where advanced computational techniques offer the greatest opportunity.
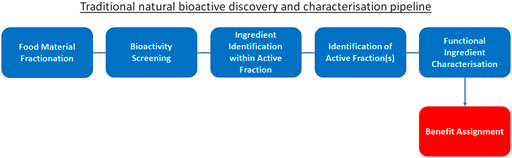
FIGURE 1. Traditional natural bioactive discovery and characterisation pipeline. Traditional process (blue) to discovery and characterisation of functional ingredients. This process begins with food material fractionation, followed by bioactivity screening, identification of active fraction(s), ingredient identification within active fraction and functional ingredient characterisation. Finally, the benefit is assigned to the bioactive (red) based on the screening results.
Artificial Intelligence in Functional Food Ingredient Characterisation
Advancing From Classical Bioinformatics to Predicting Novel Bioactives
As discussed in Traditional Ingredient Discovery and Characterisation, combining in silico bioinformatics, in vitro, and HTP screening approaches has greatly advanced the characterisation of FFIs (Li-Chan and Eunice, 2015). For example, bioinformatic tools that implement sequence similarity searches, such as the Basic Local Alignment Search Tool (BLAST) (Altschul et al., 1990) can be used to in silico mine the proteomes that contain specific proteins of interest, and to identify molecular pathways, functions and diseases in which these proteins are involved (Kanehisa and Goto, 2000; Harris et al., 2004; Carvalho-Silva et al., 2019). However, these processes typically analyse and extrapolate from known data and cannot de novo predict molecular entities and functions. Such de novo computational identification of bioactives and their functions could and should be expedited by the incorporation of artificial intelligence (AI) methods, in particular deep learning approaches, providing true innovation in areas such as functional food and small molecules at an unprecedented rate, resulting in novel insights for a range of questions (Kaur and Kumari 2019; Morley et al., 2020; Zorn et al., 2021; A. K.; Sharma et al., 2017; Kathy; Kennedy et al., 2020b; K.; Kennedy et al., 2020a; Chauhan et al., 2021; Corrochano et al., 2021; Cal et al., 2020). With a focus on peptides, we argue here for complementing, if not replacing, the traditional sequence of FFI characterisation (Figure 1) with an AI-powered alternative (“benefit definition → bioactive prediction → food source identification → bioactive release → bioactive validation”; Figure 2) by placing artificial intelligence upfront in the entire process. In other words: AI changes the paradigm from screening and retrospective benefit assignment to design according to predefined benefit.

FIGURE 2. Integrating AI into the natural bioactive discovery and characterisation pipeline. Specific health benefits or replacement needs (red) are targeted at the beginning of the process. AI integration allows for prediction of bioactives with pre-specified health benefits and subsequent selection of a most suitable source, which contains an abundance of these bioactives, plus bioactivity confirmation (blue).
Advancing From Screening to Design: The AI-Powered Discovery and Subsequent Validation Workflow
An ideal starting point and guiding principle to FFI characterisation that would address the current ad hoc discovery pipeline limitations would be to first understand and define the nutritionally actionable health needs of consumers and food chain-relevant replacement requirements. This should be followed by design and development of a commercially viable approach to identify, source, unlock and characterise a safe bioactive food peptide solution that is specific and effective and addresses needs in a cost-effective manner. For an integrated AI workflow for ingredient discovery and characterisation, once a health benefit is targeted, data in the public domain are curated from structured and unstructured data sources; these sources include scientific literature, patents and public databases that are searched for known peptide sequences with relevant bioactivity. Of note, the increased availability of food and plant molecular data has resulted in the improvement of relevant curated datasets (Westerman et al., 2020; Jensen et al., 2015; Rutz et al., 2021). These data are combined with information collected from different mass spectrometry-based peptidomics studies of plant sources and FFIs (discussed in AI Integration With Peptidomics) and in-house bioactivity validation data (discussed in Circular Science: The Iterative Feedback Loop). All these data are manually curated to ensure robust standards required for building training datasets. Typically, training datasets are compiled consisting of positive bioactive datasets, where peptide sequences have been shown to be efficacious for a specific activity either in published literature, databases or in-house assays, as well as negative datasets, where a peptide sequence did not exhibit the specified bioactivity (Kurczab et al., 2014). If there are only positive datasets available, random sequences may be used as the negative component for training purposes. The positive and negative datasets are used as input to build the algorithmic architecture that will predict peptides for a given bioactivity. These datasets essentially “train” the predictive architecture, in an iterative process, which results in improved accuracy of peptide prediction.
AI Integration With Peptidomics
The power of AI-guided discovery and characterisation becomes particularly evident in the case of peptide-containing food protein hydrolysates, which represent a large source of FFIs with virtually unlimited benefit potential. Protein hydrolysates are widely available in the food market, for example as infant formulae based on cow’s milk; soy, or rice protein hydrolysates; and fermented foods such as yoghurt or kefir (Schaafsma, 2009). They contain large complex networks of interacting bioactive peptides with attributable health benefits (Hernández-Ledesma et al., 2014; Nasri 2017). To untangle these networks and characterise key bioactives and functions within, an integrated peptidomics-AI platform has recently been proven to be successful by our laboratories (Corrochano et al., 2021; Casey et al., 2021; Kathy Kennedy et al., 2020b; K. Kennedy et al., 2020a; Chauhan et al., 2021). As previously discussed, mass spectrometry-based peptidomics of food protein hydrolysates is a key input to the AI platform: the process begins with LC-MS/MS-based peptidomics that identifies and quantifies peptides in their native state, as released by mild hydrolysis from parent proteins in the natural food source, which is not limited to a single type of food or plant source. Physicochemical characteristics such as molecular weight, charge, length, and hydrophobicity can be assigned to these peptides, thus creating libraries of invaluable parsed data from a wide range of sources, including food-by products, ready to be leveraged by the AI architecture, as described in Advancing From Classical Bioinformatics to Predicting Novel Bioactives. This approach creates millions of entries from natural source-derived in-house peptidomic data, which are then combined with the manually curated peptide data sourced from the public domain, and results from subsequent in-house validation of peptide efficacy in relevant bioactivity assays. Leveraging this data pool, these peptide libraries can be classified into various structural and functional categories using deep learning approaches, parameters such as toxicity, solubility, size, polarity and binding dynamics and many less definable albeit important characteristics are inferred from the AI architecture. Ultimately, any number of these different deep learning approaches are chosen depending on their relevance to the area of interest and have been shown to be successful in the identification of peptides with anti-microbial (Mohan et al., 2019), anti-aging (Kathy Kennedy et al., 2020b; Kathy Kennedy et al., 2020a), or anti-inflammatory (Rein et al., 2019) activity.
Circular Science: The Iterative Feedback Loop
In addition to the hydrolysate-derived and peptidomics-analysed peptide networks, individual predicted bioactive peptides are synthesised chemically and validated for efficacy in vitro, thereby elucidating mechanisms-of-action and creating a sophisticated real-time feedback loop of positive and negative data (Figure 3). This feedback constantly refines the deep learning algorithms resulting in ever-improving accuracy of bioactive peptide prediction. Once positive bioactive peptides are identified, AI is used to search natural plant and food proteomes for the presence of those positive peptides within the protein complement of a plant or food source. Once such a suitable source proteome is identified, AI informs the design of the enzymatic hydrolysis process (selection of food-grade enzymes) to be applied to unlock the targeted peptide and generate a hydrolysate with the desired peptide profile. Like the predicted and synthesised individual peptides, produced FFIs are also validated for efficacy in vitro, creating more valuable positive and negative data feedback for the AI platform. In addition to bioactivity feedback, the safety and toxicity of predicted peptides and FFIs as well as peptide stability across oral ingestion and gastro-intestinal digestion are also assessed, using cell viability assays (Cal et al., 2020) and in vitro digestion models (Corrochano et al., 2021) that are incorporated as part of the iterative learning process (Figure 3). Resulting from this AI approach, the end product FFI exhibits high bioefficacy, has a good safety profile and is characterised with a pre-approved list of key constituent peptides that have been synthesised and validated in vitro for a predicted activity.
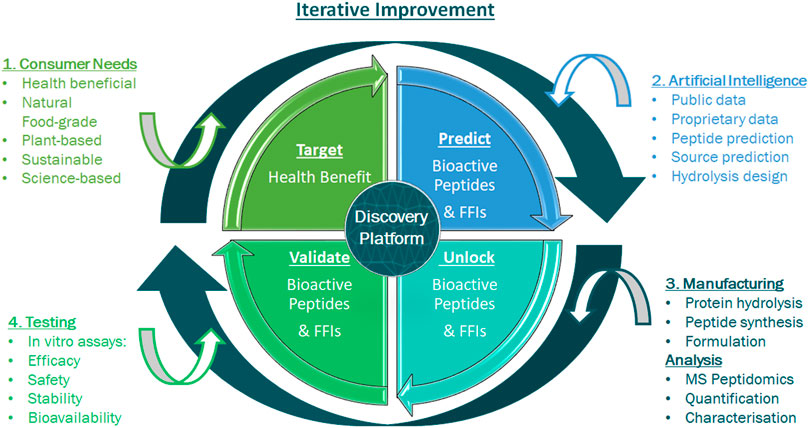
FIGURE 3. Iterative feedback loop for an integrated discovery and validation of efficacy platform. This consists of different technologies to explore the molecular diversity in plants and foods and create characterised natural, safe and effective health promoting bioactives for consumers. A number of processes are integrated in creating and characterising a functional food ingredient. 1) A consumer-driven health benefit is targeted. 2) The Artificial Intelligence (AI) process begins with data curation, predicts key bioactives and selects preferred ingredients. 3) Predicted bioactive peptides and ingredients are unlocked and produced. LC-MS/MS based-peptidomics identifies and characterises the ingredients’ peptide profile. 4) Predicted peptides and ingredients are assessed for relevant bioactivity, safety and toxicity, as well as for bioavailability using simulated gastric intestinal digestion (SGID) and investigation of stability in a relevant human matrix, i.e. blood plasma. The output of this integrated discovery platform is a characterised functional food ingredient that addresses an unmet health benefit.
For building an ingredient dossier and substantiating health claims, it is not only important to characterise key bioactivities of ingredients within a FFI but to also assess the ingredient’s stability across the gastro-intestinal digestion, its transport properties from the gut lumen to blood plasma, and its bioavailability at systemic and target tissue level. Of note, current AI architecture has the ability to predict peptides with gastro-intestinal resistance (Corrochano et al., 2021). Therefore, further simulated gastrointestinal digestion and stability experiments in vitro and ex vivo using FFIs bears the potential for future AI applications to identify key latent features, and therefore, predict the bioavailability profile of bioactive peptides and FFIs.
Case studies of AI-powered natural bioactive peptide discovery and characterisation
Anti-Inflammatory FFI
An example of a successful AI-powered benefit-driven bioactive FFI discovery is the identification and characterisation of novel anti-inflammatory bioactive peptides to address chronic low-grade inflammation. This study applied an ensemble of deep learning models to unstructured (publications, patents) and structured (data repositories) public data, as well as to proprietary peptidomic data, molecular docking simulations and phenotypic data accrued from internal experimental screening, to predict novel peptides with immunomodulatory potential from a large input set of peptide sequences from plant origins. Using an untargeted predictive approach, Asian rice was shown to be a candidate source that contained the novel predicted immunomodulatory peptides encrypted in Asian rice proteins. A hydrolysate was designed from the Asian rice bulk protein complement to create a novel FFI, rice Natural Peptide Network (NPN) (Rein et al., 2019). This FFI contained seven key constituent bioactive peptides that were physicochemically characterised and shown to exert immuno-modulatory effects in vitro. In proof-of-principle human feeding trial and a kinetic human trial, rice NPN reduced circulating TNF-α and improved physical performance in a series of challenges (such as a chair stand test) in an elderly population showing “inflammaging”, i.e. immune-senescence (K. Kennedy et al., 2020a; Rein et al., 2019). Of note, in this case of rice NPN, the time from discovery to commercial launch was approximately 2 years (Nuritas 2018), highlighting the speed at which a consumer need can be addressed using an AI-driven bioactive food peptide discovery and characterisation process.
FFI for Muscle Health
Complementary to starting with a targeted health benefit, (“from benefit to bioactive”), AI can be leveraged to characterise an already known FFI or plant/food source in terms of constituent bioactives with a targeted functionality (“from source to benefit”). This approach was adopted to characterise a hydrolysate derived from Vicia faba, NPN_1, that was previously identified to prevent muscle atrophy in vivo (Cal et al., 2020). Here, two constituent peptides were predicted to increase protein synthesis and decrease inflammation and subsequently showed positive in vitro effects on ribosomal protein (S6) phosphorylation and reduction of TNF-α, respectively (see Corrochano et al., 2021 for in depth detail on predictive architecture methods utilised) (Corrochano et al., 2021). S6 phosphorylation induces the translation of mRNA transcripts for ribosomal proteins and elongation factors that induce muscle protein synthesis (Peterson and Schreiber, 1998; Gordon et al., 2013), while TNF-α is responsible for producing chronic inflammation, which is implicated in skeletal muscle dysfunction (Londhe and Guttridge, 2015). As discussed in Characterisation of Functional Food Ingredients—For a Healthier and More Sustainable Food Chain, assessing the bioavailability of an FFI within a diet is key to characterising the bioefficacy of the ingredient. Considering this, Corrochano et al. (2021) demonstrated in vitro that both of the peptides with predicted activity within NPN_1 from Vicia faba survived simulated gastrointestinal digestion, were transported across the intestinal barrier, and exhibited notable stability in human plasma (Corrochano et al., 2021).
These two examples illustrate the game-changing impact of AI in discovery and characterisation of peptide-based FFIs. When integrated into the process, AI can:
- Extend the discovery space to the entire plant and food kingdom because peptides are the largest class of genome-encoded nutrients and are therefore amenable to prediction of function and source.
- Direct the discovery process according to predefined, unmet consumer needs, be they health care- or food chain-related.
- Accelerate the discovery and subsequent validation process by rapid development and improvement of predictors and an efficient prediction-experiment feedback loop.
- Reduce the number of candidates to be characterised biochemically and biologically.
- Inform on clinical trial design and FFI health claims.
- Efficiently build a peptide-based FFI knowledge base that informs both producers and consumers, fuelling food innovation and augmenting science-backed nutritional health benefits.
Conclusion
Given the widespread consumer acceptance of technology as a facet of their healthcare regime and the potential importance of FFI in preventive and therapeutic interventions for many chronic disorders, it is becoming clear that we are standing on the precipice of the next age of nutrition technology. The vision of health care, enabled by both nutrition and medicine (with an increasing overlap between the two) is changing from being disease-focussed in a medical setting, to a prevention-oriented practice, guided by health knowledge-empowered consumers. Ultimately, what does this next age of nutrition technology look like for the consumer? At present, most FFIs are developed in extrapolation from a limited number of ingredients with known health effects, in an inefficient, unfocussed, costly, and exceptionally slow manner. There is a vast reservoir of health-promoting and disease-defeating molecules in nature and the use of AI will enable the most efficient discovery and characterisation of innovative physiochemically and biologically characterised FFI products. These nutritional interventions will have the capability to be developed to address unmet consumer needs in the future at the levels of both individual and population health and a safe and sustainable food system. As food and nutrition become increasingly perceived as a knowledge-based health care and industry sector, we believe that integrating AI into FFIs discovery and development will greatly enhance the delivery of safe and cost-effective solutions for improved human and animal health as well as a safer and more sustainable food chain, over the decades to come.
Author Contributions
Conceptualization, NK and MK; data curation, AD; writing—original draft preparation, AD, AW and MK; writing—review and editing, AD, AW, MK and NK; visualization, MK and AW; supervision, NK; project administration, AW.
Conflict of Interest
This study received funding from Nuritas Ltd. The funder had the following involvement with the study: study design, interpretation of data, writing of this article and the decision to submit it for publication.
Authors AD, AW, NK, and MK were employed by the company Nuritas Ltd.
Publisher’s Note
All claims expressed in this article are solely those of the authors and do not necessarily represent those of their affiliated organizations, or those of the publisher, the editors and the reviewers. Any product that may be evaluated in this article, or claim that may be made by its manufacturer, is not guaranteed or endorsed by the publisher.
Acknowledgments
The authors would like to thank Dr Alberto Corrochano (Nuritas Limited) for useful discussion.
References
Acevedo-Rocha, C. G., Gronenberg, L. S., Mack, M., Commichau, F. M., Genee, H. J., and Genee, Hans. J. 2019. “Microbial Cell Factories for the Sustainable Manufacturing of B Vitamins.” Curr. Opin. Biotechnol. 56: 18–29. doi:10.1016/j.copbio.2018.07.006
Acquah, C., Chan, Y. W., Pan, S., Agyei, D., and Udenigwe, C. C. (2019). Structure-informed Separation of Bioactive Peptides. J. Food Biochem. 43 (1), e12765–10. doi:10.1111/jfbc.12765
Alkhatib, A., Tsang, C., Tiss, A., Bahorun, T., Arefanian, H., Barake, R., et al. (2017). Functional Foods and Lifestyle Approaches for Diabetes Prevention and Management. Nutrients 9 (12), 1310. doi:10.3390/nu9121310
Altschul, S. F., Gish, W., Miller, W., Myers, E. W., and Lipman, D. J. (1990). Basic Local Alignment Search Tool. J. Mol. Biol. 215 (3), 403–410. doi:10.1016/S0022-2836(05)80360-2
Angeloni, S., Ridet, J. L., Kusy, N., Gao, H., Crevoisier, F., Guinchard, S., et al. (2005). Glycoprofiling with Micro-arrays of Glycoconjugates and Lectins. Glycobiology 15 (1), 31–41. doi:10.1093/glycob/cwh143
Asakuma, S., Hatakeyama, E., Urashima, T., Yoshida, E., Katayama, T., Yamamoto, K., et al. (2011). Physiology of Consumption of Human Milk Oligosaccharides by Infant Gut-Associated Bifidobacteria. J. Biol. Chem. 286 (40), 34583–34592. doi:10.1074/jbc.M111.248138
Baboota, R. K., Bishnoi, M., Ambalam, P., Kondepudi, K. K., Sarma, S. M., Boparai, R. K., et al. (2013). Functional Food Ingredients for the Management of Obesity and Associated Co-morbidities - A Review. J. Funct. Foods 5, 997–1012. doi:10.1016/j.jff.2013.04.014
Bah, C. S. F., Bekhit, A. E.-D. A., McConnell, M. A., and Carne, A. (2016). Generation of Bioactive Peptide Hydrolysates from Cattle Plasma Using Plant and Fungal Proteases. Food Chem. 213 (December), 98–107. doi:10.1016/j.foodchem.2016.06.065
Bailes, B. K. (2002). Diabetes Mellitus and its Chronic Complications. AORN J. 76 (2), 265–282. doi:10.1016/S0001-2092(06)61065-X
Bärebring, L., Palmqvist, M., Winkvist, A., and Augustin, H. (2020). Gender Differences in Perceived Food Healthiness and Food Avoidance in a Swedish Population-Based Survey: A Cross Sectional Study. Nutr. J. 19 (1), 1–8. doi:10.1186/s12937-020-00659-0
Barnard, N. D., Cohen, J., Jenkins, D. J., Turner-McGrievy, G., Gloede, L., Green, A., et al. (2009). A Low-Fat Vegan Diet and a Conventional Diabetes Diet in the Treatment of Type 2 Diabetes: A Randomized, Controlled, 74-Wk Clinical Trial. Am. J. Clin. Nutr. 89 (5), 1588S–1596S. doi:10.3945/AJCN.2009.26736H
Bearth, A., Cousin, M.-E., and Siegrist, M. (2014). The Consumer's Perception of Artificial Food Additives: Influences on Acceptance, Risk and Benefit Perceptions. Food Qual. Preference 38 (December), 14–23. doi:10.1016/j.foodqual.2014.05.008
Birjandi, A. P., Mirnaghi, F. S., Bojko, B., Wąsowicz, M., and Pawliszyn, J. (2014). Application of Solid Phase Microextraction for Quantitation of Polyunsaturated Fatty Acids in Biological Fluids. Anal. Chem. 86 (24), 12022–12029. doi:10.1021/ac502627w
Bode, L. (2012). Human Milk Oligosaccharides: Every Baby Needs a Sugar Mama” Glycobiology, 22. 1147–1162. doi:10.1093/glycob/cws074
Bray, G. A., Kim, K. K., and Wilding, J. P. H. (2017). Obesity: A Chronic Relapsing Progressive Disease Process. A Position Statement of the World Obesity Federation. Obes. Rev. 18 (7), 715–723. doi:10.1111/OBR.12551
Brodbelt, J. S. (2016). Ion Activation Methods for Peptides and Proteins. Anal. Chem. 88, 30–51. doi:10.1021/acs.analchem.5b04563
Bronsema, K. J., Bischoff, R., and Van De Merbel, N. C. (2013). High-Sensitivity LC-MS/MS Quantification of Peptides and Proteins in Complex Biological Samples: The Impact of Enzymatic Digestion and Internal Standard Selection on Method Performance. Anal. Chem. 85 (20), 9528–9535. doi:10.1021/ac4015116
Buchler, S., Smith, K., and Lawrence, G. (2010). Food Risks, Old and New. J. Sociol. 46 (4), 353–374. doi:10.1177/1440783310384449
Cal, R., Davis, H., Kerr, A., Wall, A., Molloy, B., Chauhan, S., et al. (2020). Preclinical Evaluation of a Food-Derived Functional Ingredient to Address Skeletal Muscle Atrophy. Nutrients 12 (8), 2274. doi:10.3390/nu12082274
Carapelli, R., de Oliveira, A. F., Giglioti, R., Tullio, R. R., Nassu, R. T., Oliveira, M. C. S., et al. (2020). Calcium, Fe, Cu, Zn, and Mg Fractionation in in Natura and Aged Beef Samples by Bioanalytical Methods. Food Anal. Methods 13 (1), 186–194. doi:10.1007/s12161-019-01560-1
Carvalho-Silva, D., Pierleoni, A., Pignatelli, M., Ong, C., Fumis, L., Karamanis, N., et al. (2019). Open Targets Platform: New Developments and Updates Two Years on. Nucleic Acids Res. 47 (D1), D1056–D1065. doi:10.1093/nar/gky1133
Casey, R., Adelfio, A., Connolly, M., Wall, A., Holyer, I., and Khaldi, N. (2021). Discovery through Machine Learning and Preclinical Validation of Novel Anti-diabetic Peptides. Biomedicines 9, 276. doi:10.3390/biomedicines9030276
Castellana, M., Conte, E., Cignarelli, A., Perrini, S., Giustina, A., Giovanella, L., et al. (20192019). Efficacy and Safety of Very Low Calorie Ketogenic Diet (VLCKD) in Patients with Overweight and Obesity: A Systematic Review and Meta-Analysis. Rev. Endocr. Metab. Disord. 211 21 (1), 5–16. doi:10.1007/S11154-019-09514-Y
Castillo-Courtade, L., Han, S., Lee, S., Mian, F. M., Buck, R., and Forsythe, P. (2015). Attenuation of Food Allergy Symptoms Following Treatment with Human Milk Oligosaccharides in a Mouse Model. Allergy 70 (9), 1091–1102. doi:10.1111/all.12650
Cerullo, G., Negro, M., Parimbelli, M., Pecoraro, M., Perna, S., Liguori, G., et al. (2020). The Long History of Vitamin C: From Prevention of the Common Cold to Potential Aid in the Treatment of COVID-19. Front. Immunol. 11. doi:10.3389/fimmu.2020.574029
Chan, J. N. Y., Nislow, C., and Emili, A. 2010. “Recent Advances and Method Development for Drug Target Identification.” Trends Pharmacol. Sci. 31 (2): 82–88. doi:10.1016/j.tips.2009.11.002
Chauhan, S., Kerr, A., Keogh, B., Nolan, S., Casey, R., Adelfio, A., et al. (2021). An Artificial-Intelligence-Discovered Functional Ingredient, NRT_N0G5IJ, Derived from Pisum Sativum, Decreases HbA1c in a Prediabetic Population. Nutrients 13, 1635. doi:10.3390/nu13051635
Chen, J., Gemming, L., Hanning, R., and au, M. (2018). Smartphone Apps and the Nutrition Care Process: Current Perspectives and Future Considerations. Patient Educ. Couns. 101, 750–757. doi:10.1016/j.pec.2017.11.011
Chow, S., Liver, S., and Nelson, A. (2018). Streamlining Bioactive Molecular Discovery through Integration and Automation. Nat. Rev. Chem. 2 (8), 174–183. doi:10.1038/s41570-018-0025-7
Chu, Q., Guan, Y., Geng, C., and Ye, J. (2006). Miniaturized Capillary Electrophoresis with Amperometric Detection: Fast Separation and Detection of Bioactive Amines. Anal. Lett. 39 (4), 729–740. doi:10.1080/00032710600611129
Comstock, S. S., Li, M., Wang, M., Monaco, M. H., Kuhlenschmidt, T. B., Kuhlenschmidt, M. S., et al. (2017). Dietary Human Milk Oligosaccharides but Not Prebiotic Oligosaccharides Increase Circulating Natural Killer Cell and Mesenteric Lymph Node Memory T Cell Populations in Noninfected and Rotavirus-Infected Neonatal Piglets. J. Nutr. 147 (6), 1041–1047. doi:10.3945/jn.116.243774
Corrochano, A. R., Ferraretto, A., Arranz, E., Stuknytė, M., Bottani, M., O'Connor, P. M., et al. (2019). Bovine Whey Peptides Transit the Intestinal Barrier to Reduce Oxidative Stress in Muscle Cells. Food Chem. 288 (October 2018), 306–314. doi:10.1016/j.foodchem.2019.03.009
Corrochano, A. R., Cal, R., Kennedy, K., Wall, A., Murphy, N., Trajkovic, S., et al. (2021). “Characterising the Efficacy and Bioavailability of Bioactive Peptides Identified for Attenuating Muscle Atrophy within a Vicia Faba-Derived Functional Ingredient.” Curr. Res. Food Sci., 4, 224, 232. doi:10.1016/j.crfs.2021.03.008
de Koning, L., Malik, V. S., Kellogg, M. D., Rimm, E. B., Willett, W. C., and Hu, F. B. (2012). Sweetened Beverage Consumption, Incident Coronary Heart Disease, and Biomarkers of Risk in Men. Circulation 125 (14), 1735–1741. doi:10.1161/CIRCULATIONAHA.111.067017
de Lorgeril, M., and Salen, P. (2007)., 8. Lippincott Williams and Wilkins, S38–S41. doi:10.2459/01.JCM.0000289268.90482.7bMediterranean Diet and N-3 Fatty Acids in the Prevention and Treatment of Cardiovascular DiseaseJ. Cardiovasc. Med.
DeLuca, H. F. (2016). Vitamins and Hormones, 100. Academic Press, 1–20. doi:10.1016/bs.vh.2015.11.001Vitamin D
Dicker, Daniel., Nguyen, Grant., Abate, Degu., Abay, Solomon. M., Abbafati, Cristiana., Abbasi, Nooshin., et al. (2018). Global, Regional, and National Age-sex-specific Mortality and Life Expectancy, 1950-2017: A Systematic Analysis for the Global Burden of Disease Study 2017. The Lancet 392 (10159), 1684–1735. doi:10.1016/S0140-6736(18)31891-9
Dołowy, M., and Pyka, A. (2015). Chromatographic Methods in the Separation of Long-Chain Mono- and Polyunsaturated Fatty Acids. J. Chem. 2015, 1–20. Hindawi Limited. doi:10.1155/2015/120830
Donovan, S. M., and Comstock, S. S. (2017). Human Milk Oligosaccharides Influence Neonatal Mucosal and Systemic Immunity. Ann. Nutr. Metab. 69, 41–51. doi:10.1159/000452818
Doub, A. E., Levin, A., Heath, C. E., and LeVangie, K. (2015). Mobile App-Etite: Consumer Attitudes towards and Use of Mobile Technology in the Context of Eating Behaviour. J. Direct Data Digit Mark Pract. 17 (2), 114–129. doi:10.1057/dddmp.2015.44
Esposito, K., Maiorino, M. I., Bellastella, G., Panagiotakos, D. B., and Giugliano, D. (2017). Mediterranean Diet for Type 2 Diabetes: Cardiometabolic Benefits. Endocrine 56 (1), 27–32. doi:10.1007/s12020-016-1018-2
Estruch, R., and Ros, E. (20202020). The Role of the Mediterranean Diet on Weight Loss and Obesity-Related Diseases. Rev. Endocr. Metab. Disord. 213 21 (3), 315–327. doi:10.1007/S11154-020-09579-0
Eussen, S. R. B. M., Feenstra, T. L., Toxopeus, I. B., Hoekstra, J., Klungel, O. H., Verhagen, H., et al. (2011). Costs and Health Effects of Adding Functional Foods Containing Phytosterols/-Stanols to Statin Therapy in the Prevention of Cardiovascular Disease. Eur. J. PharmacologyEur J Pharmacol 668, S91–S100. doi:10.1016/j.ejphar.2011.05.081
Feng, L., Peng, F., Wang, X., Li, M., Lei, H., and Xu, H. (2019). Identification and Characterization of Antioxidative Peptides Derived from Simulated In Vitro Gastrointestinal Digestion of Walnut Meal Proteins. Food Res. Int. 116, 518–526. doi:10.1016/j.foodres.2018.08.068
Ferreira, M. A., Silva, D. M., de Morais, A. C., Mota, J. F., and Botelho, P. B. (2016). Therapeutic Potential of Green Tea on Risk Factors for Type 2 Diabetes in Obese Adults - a Review. Obes. Rev. 17 (12), 1316–1328. doi:10.1111/obr.12452
Fonseca, B. M., Cristóvão, A. C., and Alves, G. (2018). An Easy-To-Use Liquid Chromatography Method with Fluorescence Detection for the Simultaneous Determination of Five Neuroactive Amino Acids in Different Regions of Rat Brain. J. Pharmacol. Toxicol. Methods 91 (May), 72–79. doi:10.1016/j.vascn.2018.02.002
Gallagher, A. M., Meijer, G. W., Richardson, D. P., Rondeau, V., Skarp, M., Stasse-Wolthuis, M., et al. (2011). A Standardised Approach towards PROving the Efficacy of Foods and Food Constituents for Health CLAIMs (PROCLAIM): Providing Guidance. Br. J. Nutr. 106 (Suppl. 2), S16–S28. doi:10.1017/S0007114511003618
Gallego, M., Mora, L., Escudero, E., and Toldrá, F. (2018). Bioactive Peptides and Free Amino Acids Profiles in Different Types of European Dry-Fermented Sausages. Int. J. Food Microbiol. 276 (July), 71–78. doi:10.1016/j.ijfoodmicro.2018.04.009
Garrido, D., Ruiz-Moyano, S., Kirmiz, N., Davis, J. C., Totten, S. M., Lemay, D. G., et al. (2016). A Novel Gene Cluster Allows Preferential Utilization of Fucosylated Milk Oligosaccharides in Bifidobacterium Longum Subsp. Longum SC596. Sci. Rep. 6 (October). doi:10.1038/srep35045
Gene Ontology Consortium, M. A., Clark, J., Ireland, A., Lomax, J., Ashburner, M., Foulger, R., et al. (2004). The Gene Ontology (GO) Database and Informatics Resource. Nucleic Acids Res. 32 (DATABASE ISS.), 258D–261D. doi:10.1093/nar/gkh036
Gentile, D., Fornai, M., Pellegrini, C., Colucci, R., Blandizzi, C., and Antonioli, L. (2018). Dietary Flavonoids as a Potential Intervention to Improve Redox Balance in Obesity and Related Co-morbidities: A Review. Nutr. Res. Rev. 31 (2), 239–247. doi:10.1017/S0954422418000082
Global Human Milk Oligosaccharides Market Expected to Grow with a CAGR of 21% During the Forecast Period, 2018-2027 - ResearchAndMarkets.Com | Business Wire“Global Human Milk Oligosaccharides Market Expected to Grow with a CAGR of 21% during the Forecast Period, 2018-2027 - ResearchAndMarkets.Com | Business Wire.”2020.
Goehring, K. C., Marriage, B. J., Oliver, J. S., Wilder, J. A., Barrett, E. G., and Buck, R. H. (2016). Similar to Those Who Are Breastfed, Infants Fed a Formula Containing 2′-Fucosyllactose Have Lower Inflammatory Cytokines in a Randomized Controlled Trial. J. Nutr. 146 (12), 2559–2566. doi:10.3945/jn.116.236919
Gordon, B. S., Kelleher, A. R., and Kimball, S. R. (2013). Regulation of Muscle Protein Synthesis and the Effects of Catabolic States. Int. J. Biochem. Cel Biol. 45 (10), 2147–2157. doi:10.1016/j.biocel.2013.05.039
Höller, U., Bakker, S. J. L., Düsterloh, A., Frei, B., Köhrle, J., Konz, T., et al. 2018. “Micronutrient Status Assessment in Humans: Current Methods of Analysis and Future Trends.” Trac Trends Anal. Chem. 102: 110–122. doi:10.1016/j.trac.2018.02.001
Hartmann, C., Hieke, S., Taper, C., and Siegrist, M. (2018). European Consumer Healthiness Evaluation of 'Free-From' Labelled Food Products. Food Qual. Preference 68 (September), 377–388. doi:10.1016/j.foodqual.2017.12.009
He, Y., Liu, S., Kling, D. E., Leone, S., Lawlor, N. T., Huang, Y., et al. (2016). The Human Milk Oligosaccharide 2′-fucosyllactose Modulates CD14 Expression in Human Enterocytes, Thereby Attenuating LPS-Induced Inflammation. Gut 65 (1), 33–46. doi:10.1136/gutjnl-2014-307544
Hernández-Ledesma, B., García-Nebot, M. J., Fernández-Tomé, S., Amigo, L., and Recio, I. 2014. “Dairy Protein Hydrolysates: Peptides for Health Benefits.” Int. Dairy J. 38 (2): 82–100. doi:10.1016/j.idairyj.2013.11.004
Hewawasam, E., Liu, G., Jeffery, D. W., Muhlhausler, B. S., and Gibson, R. A. (2017). A Validated Method for Analyzing Polyunsaturated Free Fatty Acids from Dried Blood Spots Using LC-MS/MS. Prostaglandins, Leukot. Essent. Fatty Acids 125 (October), 1–7. doi:10.1016/j.plefa.2017.08.010
Hewlings, S., and Kalman, D. (2017). Curcumin: A Review of its Effects on Human Health. Foods 6 (10), 92. doi:10.3390/foods6100092
Hoeflinger, J. L., Davis, S. R., Chow, Jo. May., Chow, J., and Miller, M. J. (2015). In Vitro Impact of Human Milk Oligosaccharides on Enterobacteriaceae Growth. J. Agric. Food Chem. 63 (12), 3295–3302. doi:10.1021/jf505721p
Holscher, H. D., Davis, S. R., and Tappenden, K. A. (2014). Human Milk Oligosaccharides Influence Maturation of Human Intestinal Caco-2Bbe and HT-29 Cell Lines. J. Nutr. 144 (5), 586–591. doi:10.3945/jn.113.189704
Holst, B., and Williamson, G. (2008). Nutrients and Phytochemicals: From Bioavailability to Bioefficacy beyond Antioxidants. Curr. Opin. Biotechnol. 19 (2), 73–82. doi:10.1016/j.copbio.2008.03.003
Hopkins, A. L. (2008). Network Pharmacology: The Next Paradigm in Drug Discovery. Nat. Chem. Biol. 4, 682–690. doi:10.1038/nchembio.118
Hungin, A. P. S., Mitchell, C. R., Whorwell, P., Mulligan, C., Cole, O., Agréus, L., et al. (2018). Systematic Review: Probiotics in the Management of Lower Gastrointestinal Symptoms - an Updated Evidence-Based International Consensus. Aliment. Pharmacol. Ther. 47 (8), 1054–1070. doi:10.1111/apt.14539
Ichihara, K. i., Shibahara, A., Yamamoto, K., and Nakayama, T. (1996). An Improved Method for Rapid Analysis of the Fatty Acids of Glycerolipids. Lipids 31 (5), 535–539. doi:10.1007/BF02522648
Idänpään‐Heikkilä, I., Simon, P. M., Zopf, D., Vullo, T., Cahill, P., Sokol, K., et al. (1997). Oligosaccharides Interfere with the Establishment and Progression of Experimental Pneumococcal Pneumonia. J. Infect. Dis. 176 (3), 704–712. doi:10.1086/514094
Jacobson, M. K., and Jacobson, E. L. (2018). Vitamin B3 in Health and Disease: Toward the Second Century of Discovery. Methods Mol. Biol. 1813, 3–8. doi:10.1007/978-1-4939-8588-3_1
Jansen, T., Claassen, L., van Kamp, I., Timmermans, D. R. M., and Timmermans, M. (2020). 'All Chemical Substances Are harmful.' Public Appraisal of Uncertain Risks of Food Additives and Contaminants. Food Chem. Toxicol. 136 (February), 110959. doi:10.1016/j.fct.2019.110959
Jensen, K., Panagiotou, G., and Kouskoumvekaki, I. (2015). NutriChem: A Systems Chemical Biology Resource to Explore the Medicinal Value of Plant-Based Foods. Nucleic Acids Res. 43 (D1), D940–D945. doi:10.1093/NAR/GKU724
Jones, G. (2018). The Discovery and Synthesis of the Nutritional Factor Vitamin D. Int. J. Paleopathology 23, 96–99. doi:10.1016/j.ijpp.2018.01.002
Kambhampati, S., Li, J., Evans, B. S., and Allen, D. K. (2019). Accurate and Efficient Amino Acid Analysis for Protein Quantification Using Hydrophilic Interaction Chromatography Coupled Tandem Mass Spectrometry. Plant Methods 15 (1), 1–12. doi:10.1186/s13007-019-0430-z
Kanehisa, M., and Goto, Susumu. (2000). ” Nucleic Acids Research, 28. Oxford University Press, 27–30. doi:10.1093/nar/28.1.27KEGG: Kyoto Encyclopedia of Genes and Genomes
Kaput, J., Kussmann, M., Radonjic, M., Virgili, F., and Perozzi, G. (2015). Human Nutrition, Environment, and Health. Genes Nutr. 10 (5), 489. doi:10.1007/s12263-015-0489-8
Kaur, H., and Kumari, V. (2020). Predictive Modelling and Analytics for Diabetes Using a Machine Learning Approach. Aci ahead-of-print. doi:10.1016/j.aci.2018.12.004
Kenfield, S. A., Dupre, N., Richman, E. L., Stampfer, M. J., Chan, J. M., and Giovannucci, E. L. (2014). Mediterranean Diet and Prostate Cancer Risk and Mortality in the Health Professionals Follow-Up Study. Eur. Urol. 65 (5), 887–894. doi:10.1016/j.eururo.2013.08.009
Kennedy, K., Cal, R., Casey, R., Lopez, C., Adelfio, A., Molloy, B., et al. (2020b). The Anti‐ageing Effects of a Natural Peptide Discovered by Artificial Intelligence. Int. J. Cosmet. Sci. 42 (May, ics), 388–398. doi:10.1111/ics.12635
Kennedy, K., Keogh, B., Lopez, C., Adelfio, A., Molloy, B., Kerr, A., et al. (2020a). Foods publicatio.An Artificial Intelligence Characterized Functional Ingredient, Derived from Rice, Inhibits TNF-α and Significantly Improves Physical Strength in an Inflammaging Population
Konz, T., Migliavacca, E., Dayon, L., Bowman, G., Oikonomidi, A., Popp, J., et al. (2017). ICP-MS/MS-Based Ionomics: A Validated Methodology to Investigate the Biological Variability of the Human Ionome. J. Proteome Res. 16 (5), 2080–2090. doi:10.1021/acs.jproteome.7b00055
Kuntz, S., Kunz, C., and Rudloff, S. (2009). Oligosaccharides from Human Milk Induce Growth Arrest via G2/M by Influencing Growth-Related Cell Cycle Genes in Intestinal Epithelial Cells. Bjn 101 (9), 1306–1315. doi:10.1017/S0007114508079622
Kunz, C. (2012). Historical Aspects of Human Milk Oligosaccharides. Adv. Nutr. 3 (3), 430S–439S. doi:10.3945/an.111.001776
Kurczab, R., Smusz, S., and Bojarski, A. J. (2014). The Influence of Negative Training Set Size on Machine Learning-Based Virtual Screening. J. Cheminform 6 (1 61), 1–9. doi:10.1186/1758-2946-6-32
Kussmann, M., Affolter, M., Nagy, K., Holst, B., and Fay, L. B. 2007. “Mass Spectrometry in Nutrition: Understanding Dietary Health Effects at the Molecular Level.” Mass. Spectrom. Rev. 26 (6): 727–750. doi:10.1002/mas.20147
Le, A., Ng, A., Kwan, T., Cusmano-Ozog, K., and Cowan, T. M. (2014). A Rapid, Sensitive Method for Quantitative Analysis of Underivatized Amino Acids by Liquid Chromatography-Tandem Mass Spectrometry (LC-MS/MS). J. Chromatogr. B 944 (January), 166–174. doi:10.1016/j.jchromb.2013.11.017
Li-Chan, E. C., and Eunice, C. Y. (2015). Bioactive Peptides and Protein Hydrolysates: Research Trends and Challenges for Application as Nutraceuticals and Functional Food Ingredients. Curr. Opin. Food Sci. 1 (1), 28–37. doi:10.1016/j.cofs.2014.09.005
Lin, H.-C., Shih, L.-C., and Lin, H.-M. (2017). The Influence of Consumers' Self-Perceived Health Status and Need for Cognition on Food-Product Evaluation. Bfj 119 (2), 242–252. doi:10.1108/BFJ-05-2016-0217
Littlejohn, P., Cop, I., Brown, E., Afroze, R., and Davison, K. M. (2018). Comparison of Nutrigenomics Technology Interface Tools for Consumers and Health Professionals: Protocol for a Mixed-Methods Study. JMIR Res. Protoc. 7 (6), e115. doi:10.2196/resprot.9846
Liu, G., Sun, S., Guo, B., Miao, B., Luo, Z., Xia, Z., et al. (2018). Bioactive Peptide Isolated from Casein Phosphopeptides Promotes Calcium Uptake In Vitro and In Vivo. Food Funct. 9 (4), 2251–2260. doi:10.1039/c7fo01709j
LoCascio, R. G., Ninonuevo, M. R., Freeman, S. L., Lebrilla, David. A., Sela, D. A., Grimm, R., et al. (2007). Glycoprofiling of Bifidobacterial Consumption of Human Milk Oligosaccharides Demonstrates Strain Specific, Preferential Consumption of Small Chain Glycans Secreted in Early Human Lactation. J. Agric. Food Chem. 55 (22), 8914–8919. doi:10.1021/jf0710480
Londhe, P., and Guttridge, D. C. (2015). Inflammation Induced Loss of Skeletal Muscle. Bone 80 (November), 131–142. doi:10.1016/j.bone.2015.03.015
Lynam, A.-M., McKevitt, A., and Gibney, M. J. (2011). Irish Consumers' Use and Perception of Nutrition and Health claimsIrish Consumers’ Use and Perception of Nutrition and Health Claims. Public Health Nutr. 14 (12), 2213–2219. doi:10.1017/S1368980011000723
Magiorkinis, E., Beloukas, A., and Diamantis, A. (2011). Scurvy: Past, Present and Future. Eur. J. Intern. Med. 22, 147–152. doi:10.1016/j.ejim.2010.10.006
Maqbool, Muhammad. Amir., and Aslam, Muhammad. 2018, n.d. “Biological Importance of Vitamins for Human Health: A Review Breeding of White Maize View Project Measurement of Cell Membrane Thermo-Stability and Leaf Temperature for Heat Tolerance in Maize (Zea Mays L): Genotypic Variability and Inheritance Pattern Vi.”
Massaro, M., Scoditti, E., Carluccio, M. A., and De Caterina, R. (2010). Nutraceuticals and Prevention of Atherosclerosis: Focus on ω-3 Polyunsaturated Fatty Acids and Mediterranean Diet Polyphenols. Cardiovasc. Ther. 28, e13–e19. doi:10.1111/j.1755-5922.2010.00211.x
Mattei, J., Malik, Vasanti., Malik, V., Wedick, N. M., Hu, F. B., Spiegelman, D., et al. (20152015). Reducing the Global Burden of Type 2 Diabetes by Improving the Quality of Staple Foods: The Global Nutrition and Epidemiologic Transition Initiative. Glob. Health 11 (1 111), 1–20. doi:10.1186/S12992-015-0109-9
Mitic, S., Kostic, D., Nasković-Dokić, D., and Mitic, M. (2011). Rapid and Reliable HPLC Method for the Determination of Vitamin C in Pharmaceutical Samples. Trop. J. Pharm. Res. 10 (1), 105–111. doi:10.4314/tjpr.v10i1.66549
Mohan, Niamh. Maire., Amine, Zorgani., Jalowicki, Gael., Kerr, Alish., Khaldi, Nora., and Martins, Marta. (2019). Unlocking NuriPep 1653 from Common Pea Protein: A Potent Antimicrobial Peptide to Tackle a Pan-Drug Resistant Acinetobacter Baumannii. Front. Microbiol. 10, 2086. doi:10.3389/fmicb.2019.02086
Morley, J., Machado, C. C. V., Burr, C., Cowls, J., Joshi, I., Taddeo, M., et al. (2020). The Ethics of AI in Health Care: A Mapping Review. Soc. Sci. Med. 260, 113172. doi:10.1016/j.socscimed.2020.113172
Morrow, A. L., Ruiz-Palacios, G. M., Jiang, X., and Newburg, D. S. (2005). Human-Milk Glycans that Inhibit Pathogen Binding Protect Breast-Feeding Infants against Infectious Diarrhea. J. Nutr. 135, 1304–1307. doi:10.1093/jn/135.5.1304
Mukherjee, R. P., Beitle, R., Jayanthi, S., Kumar, T. K. S., and McNabb, D. S. (2016). Production of an Anti-candidapeptide via Fed Batch and Ion Exchange Chromatography. Biotechnol. Prog. 32 (4), 865–871. doi:10.1002/btpr.2296
Muraki, I., Imamura, F., Manson, J. E., Hu, F. B., Willett, W. C., van Dam, R. M., et al. (2013). Fruit Consumption and Risk of Type 2 Diabetes: Results from Three Prospective Longitudinal Cohort Studies. Bmj 347 (7923), f5001. doi:10.1136/bmj.f5001
Nagy, K., Courtet-Compondu, M.-C., Holst, B., and Kussmann, M. (2007). Comprehensive Analysis of Vitamin E Constituents in Human Plasma by Liquid Chromatography−Mass Spectrometry. Anal. Chem. 79 (18), 7087–7096. doi:10.1021/ac0708689
Nagy, K., Redeuil, K., Bertholet, R., Steiling, H., and Kussmann, M. (2009). Quantification of Anthocyanins and Flavonols in Milk-Based Food Products by Ultra Performance Liquid Chromatography−Tandem Mass Spectrometry. Anal. Chem. 81 (15), 6347–6356. doi:10.1021/ac900608g
Nannapaneni, N. K., Jalalpure, S. S., Muppavarapu, R., and Sirigiri, S. K. (2017). A Sensitive and Rapid UFLC-APCI-MS/MS Bioanalytical Method for Quantification of Endogenous and Exogenous Vitamin K1 Isomers in Human Plasma: Development, Validation and First Application to a Pharmacokinetic Study. Talanta 164 (March), 233–243. doi:10.1016/j.talanta.2016.11.056
Nasri, M. 2017. “Protein Hydrolysates and Biopeptides.” In , edited by Fidel B T - Advances in Food and Nutrition Research Toldrá, 81:109–159. Academic Press. doi:10.1016/bs.afnr.2016.10.003
Nuritas (2018). Nuritas Announces Launch of the World’s First Healthcare Ingredient Delivered through Artificial Intelligence.” Globe Newswire. 2018. Avaialble at: https://www.globenewswire.com/news-release/2018/11/08/1647949/0/en/Nuritas-Announces-Launch-of-the-World-s-First-Healthcare-Ingredient-Delivered-Through-Artificial-Intelligence.html.
Panchaud, A., Affolter, M., and Kussmann, M. (2012). Mass Spectrometry for Nutritional Peptidomics: How to Analyze Food Bioactives and Their Health Effects. J. Proteomics 75, 3546–3559. doi:10.1016/j.jprot.2011.12.022
Papetti, A. 2012. “Isolation and Characterization of Antimicrobial Food Components.” Curr. Opin. Biotechnol. 23 (2): 168–173. doi:10.1016/j.copbio.2011.09.001
Parmar, Manisha., Guru, Angad., and Gupta, Phutela. (2015). The New Generation Additives Article in. Int. J. Curr. Microbiol. Appl. Sci. 4.
Partridge, D., Lloyd, K. A., Rhodes, J. M., Walker, A. W., Johnstone, A. M., and Campbell, B. J. (2019). Food Additives: Assessing the Impact of Exposure to Permitted Emulsifiers on Bowel and Metabolic Health - Introducing the FADiets Study. Nutr. Bull. 44 (4), 329–349. doi:10.1111/nbu.12408
Peterson, R. T., and Schreiber, S. L. 1998. “Translation Control: Connecting Mitogens and the Ribosome.” Curr. Biol. 8 (7): R248–R250. doi:10.1016/S0960-9822(98)70152-6
Rebello, C. J., Greenway, F. L., and Finley, J. W. (2014). A Review of the Nutritional Value of Legumes and Their Effects on Obesity and its Related Co-morbidities. Obes. Rev. 15 (5), 392–407. doi:10.1111/obr.12144
Rein, D., Ternes, P., Demin, R., Gierke, J., Helgason, T., and Schön, C. (2019). Artificial Intelligence Identified Peptides Modulate Inflammation in Healthy Adults. Food Funct. 10 (9), 6030–6041. doi:10.1039/c9fo01398a
Román, S., Sánchez-Siles, L. M., and Siegrist, M. (2017). The Importance of Food Naturalness for Consumers: Results of a Systematic Review. Trends Food Sci. Techn. 67, 44–57. doi:10.1016/j.tifs.2017.06.010
Ruiz-Palacios, G. M., Cervantes, L. E., Ramos, P., Chavez-Munguia, B., and Newburg, D. S. (2003). Campylobacter Jejuni Binds Intestinal H(O) Antigen (Fucα1, 2Galβ1, 4GlcNAc), and Fucosyloligosaccharides of Human Milk Inhibit its Binding and Infection. J. Biol. Chem. 278 (16), 14112–14120. doi:10.1074/jbc.M207744200
Rutz, A., Sorokina, M., Galgonek, J., Mietchen, D., Willighagen, E., Gaudry, A., et al. (2021). The LOTUS Initiative for Open Natural Products Research: Knowledge Management through Wikidata, 02. BioRxiv 28, 433265. doi:10.1101/2021.02.28.433265
Salas-Salvadó, J., Bulló, M., Estruch, R., Ros, E., Covas, M.-I., Ibarrola-Jurado, N., et al. (2014). Prevention of Diabetes with Mediterranean Diets. Ann. Intern. Med. 160 (1), 1–10. doi:10.7326/M13-1725
Sami, W., Ansari, T., Butt, N. S., and Hamid, M. R. A. (2017). Effect of Diet on Type 2 Diabetes Mellitus: A Review. Int. J. Health Sci. (Qassim) 11 (2), 65–71.
Savchenko, O. M., Li, T., Kecinski, M., and Messer, K. D. (2019). Does Food Processing Mitigate Consumers' Concerns about Crops Grown with Recycled Water? Food Policy 88 (October), 101748. doi:10.1016/j.foodpol.2019.101748
Schaafsma, G. (2009). Safety of Protein Hydrolysates, Fractions Thereof and Bioactive Peptides in Human Nutrition. Eur. J. Clin. Nutr. 63 (10), 1161–1168. doi:10.1038/ejcn.2009.56
Schaffer, M., Schaffer, P. M., and Bar-Sela, G. (2015). An Update on Curcuma as a Functional Food in the Control of Cancer and Inflammation. Curr. Opin. Clin. Nutr. Metab. Care 18 (6), 605–611. doi:10.1097/MCO.0000000000000227
Schnatbaum, K., Solis‐Mezarino, V., Pokrovsky, D., Schäfer, F., Nagl, D., Hornberger, L., et al. (2020). New Approaches for Absolute Quantification of Stable‐Isotope‐Labeled Peptide Standards for Targeted Proteomics Based on a UV Active Tag. Proteomics 20 (10), 2000007. doi:10.1002/pmic.202000007
Schwarz, P., Lindström, J., Kissimova-Scarbeck, K., Szybinski, Z., Barengo, N., Peltonen, M., et al. (2008). J. Tuomilehto, and Null on Behalf of the DE-PLAN projectThe European Perspective of Type 2 Diabetes Prevention: Diabetes in Europe - Prevention Using Lifestyle, Physical Activity and Nutritional Intervention (DE-PLAN) Project. Exp. Clin. Endocrinol. Diabetes 116 (03), 167–172. doi:10.1055/S-2007-992115
Schweiggert, R. M. (2018). Perspective on the Ongoing Replacement of Artificial and Animal-Based Dyes with Alternative Natural Pigments in Foods and Beverages. J. Agric. Food Chem. 66 (12), 3074–3081. doi:10.1021/acs.jafc.7b05930
Scotter, M. J. (2011). Emerging and Persistent Issues with Artificial Food Colours: Natural Colour Additives as Alternatives to Synthetic Colours in Food and Drink. Qual. Assur. Saf. Crops Foods 3 (1), 28–39. doi:10.1111/j.1757-837X.2010.00087.x
Serafim, V., Tiugan, D.-A., Andreescu, N., Mihailescu, A., Paul, C., Velea, I., et al. (2019). Development and Validation of a LC-MS/MS-Based Assay for Quantification of Free and Total Omega 3 and 6 Fatty Acids from Human Plasma. Molecules 24 (2), 360. doi:10.3390/molecules24020360
Shahidi, F., and Ambigaipalan, P. (2018). Omega-3 Polyunsaturated Fatty Acids and Their Health Benefits. Annu. Rev. Food Sci. Technol. 9 (1), 345–381. doi:10.1146/annurev-food-111317-095850
Sharma, A. K., Srivastava, G. N., Roy, A., and Sharma, V. K. (2017). ToxiM: A Toxicity Prediction Tool for Small Molecules Developed Using Machine Learning and Chemoinformatics Approaches. Front. Pharmacol. 8 (NOV), 880. doi:10.3389/FPHAR.2017.00880
Sharma, S., Gittelsohn, J., Rosol, R., and Beck, L. (2010). Addressing the Public Health Burden Caused by the Nutrition Transition through the Healthy Foods North Nutrition and Lifestyle Intervention Programme. J. Hum. Nutr. Diet. 23 (Suppl. 1), 120–127. doi:10.1111/J.1365-277X.2010.01107.X
Solymosi, K., Latruffe, N., Morant-Manceau, A., and Schoefs, B. (2015). Colour Additives For Foods And Beverages, 4–34. Elsevier, 3–34. doi:10.1016/B978-1-78242-011-8.00001-5Food Colour Additives of Natural Origin
Spahr, C. S., Susin, S. A., Bures, E. J., Robinson, J. H., Davis, M. T., and McGinley, M. D. (2000). , 200021, 1635–1650. doi:10.1002/(SICI)1522-2683(20000501)21:9<1635:AID-ELPS1635>3.0.CO;2-1Simplification of Complex Peptide Mixtures for Proteomic Analysis: Reversible Biotinylation of Cysteinyl Peptides - SpahrELECTROPHORESIS9
Speer, H., D’Cunha, N. M., Alexopoulos, N. I., McKune, A. J., and Naumovski, N. (2020). Anthocyanins and Human Health-A Focus on Oxidative Stress, Inflammation and Disease. Antioxidants 9, 366. doi:10.3390/antiox9050366
Stampfer, M. J., Hu, F. B., Hu, J. E., Manson, J. E., Rimm, E. B., and Willett, W. C. (2000). Primary Prevention of Coronary Heart Disease in Women through Diet and Lifestyle. N. Engl. J. Med. 343 (1), 16–22. doi:10.1056/NEJM200007063430103
Sun, X., Acquah, C., Aluko, R. E., and Udenigwe, C. C. (2020). Considering Food Matrix and Gastrointestinal Effects in Enhancing Bioactive Peptide Absorption and Bioavailability. J. Funct. Foods 64 (November), 103680. doi:10.1016/j.jff.2019.103680
Taghvaei, M., and Jafari, S. M. (2015). Application and Stability of Natural Antioxidants in Edible Oils in Order to Substitute Synthetic Additives. J. Food Sci. Technol. 52, 1272–1282. doi:10.1007/s13197-013-1080-1
Takebe, T., Imai, R., and Ono, S. (2018). The Current Status of Drug Discovery and Development as Originated in United States Academia: The Influence of Industrial and Academic Collaboration on Drug Discovery and Development. Clin. Translational Sci. 11 (6), 597–606. doi:10.1111/cts.12577
Talati, Z., Pettigrew, S., Hughes, C., Dixon, H., Kelly, B., Ball, K., et al. (2016). The Combined Effect of Front-Of-Pack Nutrition Labels and Health Claims on Consumers' Evaluation of Food Products. Food Qual. Preference 53 (October), 57–65. doi:10.1016/j.foodqual.2016.05.016
Tasevska, N., Park, Y., Jiao, L., Hollenbeck, A., Subar, A. F., and Potischman, N. (2014). Sugars and Risk of Mortality in the NIH-AARP Diet and Health Study. Am. J. Clin. Nutr. 99 (5), 1077–1088. doi:10.3945/ajcn.113.069369
Tempesta, G., Bosi, F., and Agrosì, G. (2020). Crystal Chemical Characterisation of Red Beryl by 'Standardless' Laser‐Induced Breakdown Spectroscopy and Single‐Crystal Refinement by X‐Ray Diffraction: An Example of Validation of an Innovative Method for the Chemical Analysis of Minerals. Geostand Geoanal Res. 44 (4), 685–693. doi:10.1111/ggr.12346
Tena, N., Martín, J., and Asuero, A. G. (2020). State of the Art of Anthocyanins: Antioxidant Activity, Sources, Bioavailability, and Therapeutic Effect in Human Health. Antioxidants 9, 451. doi:10.3390/antiox9050451
Thomas, S., Karnik, S., Barai, R. S., Jayaraman, V. K., and Idicula-Thomas, S. (2009). Jayaraman, and Susan Idicula-ThomasCAMP: A Useful Resource for Research on Antimicrobial Peptides. Nucleic Acids Res. 38 (Suppl. 1), D774–D780. doi:10.1093/nar/gkp1021
Tribalat, L., Paisse, O., Dessalces, G., and Grenier-Loustalot, M.-F. (2006). Advantages of LC-MS-MS Compared to LC-MS for the Determination of Nitrofuran Residues in Honey. Anal. Bioanal. Chem. 386 (7–8), 2161–2168. doi:10.1007/s00216-006-0878-3
Turner-McGrievy, G., Mandes, T., and Crimarco, A. (2017). A Plant-Based Diet for Overweight and Obesity Prevention and Treatment. J. Geriatr. Cardiol. 14 (5), 369–374. doi:10.11909/J.ISSN.1671-5411.2017.05.002
Udenigwe, C. C., and Fogliano., V. (2017). Food Matrix Interaction and Bioavailability of Bioactive Peptides: Two Faces of the Same Coin? J. Funct. Foods 35, 9–12. doi:10.1016/j.jff.2017.05.029
Valls-Pedret, C., Sala-Vila, A., Serra-Mir, M., Corella, D., de la Torre, R., Martínez-González, M. Á., et al. (2015). Mediterranean Diet and Age-Related Cognitive Decline. JAMA Intern. Med. 175 (7), 1094–1103. doi:10.1001/jamainternmed.2015.1668
van Gunst, A., and Roodenburg, A. J. C. (2019). Consumer Distrust about E-Numbers: A Qualitative Study Among Food Experts. Foods 8 (5), 178. doi:10.3390/foods8050178
Virág, D., Király, M., Drahos, L., Édes, A. E., Gecse, K., Bagdy, G., et al. (2020). Development, Validation and Application of LC-MS/MS Method for Quantification of Amino Acids, Kynurenine and Serotonin in Human Plasma. J. Pharm. Biomed. Anal. 180 (February), 113018. doi:10.1016/j.jpba.2019.113018
Wang, J., Lee Bravatti, M. A., Johnson, E. J., Raman, G., and Raman, Gowri. (2020). Daily Almond Consumption in Cardiovascular Disease Prevention via LDL-C Change in the U.S. Population: A Cost-Effectiveness Analysis. BMC Public Health 20 (1), 1–10. doi:10.1186/s12889-020-08642-4
Wang, L., Du, S., Wang, H., and Popkin, B. M. (2018). Influence of Dietary Fat Intake on Bodyweight and Risk of Obesity Among Chinese Adults, 1991-2015: a Prospective Cohort Study. The Lancet 392 (October), S20. doi:10.1016/s0140-6736(18)32649-7
Wang, X., Yu, H., Xing, R., and Li, P. (2017). Characterization, Preparation, and Purification of Marine Bioactive Peptides. Biomed. Res. Int. 2017, 1–16. doi:10.1155/2017/9746720
Watanabe, Y., and Tatsuno, I. (2017). Omega-3 Polyunsaturated Fatty Acids for Cardiovascular Diseases: Present, Past and Future. Expert Rev. Clin. Pharmacol. 10, 865–873. doi:10.1080/17512433.2017.1333902
Westerman, K. E., Harrington, S., Ordovas, J. M., and Parnell, L. D. (20202020). PhyteByte: Identification of Foods Containing Compounds with Specific Pharmacological Properties. BMC Bioinformatics 21 (1 211), 1–8. doi:10.1186/S12859-020-03582-7
Winkler, H. C., Suter, M., and Naegeli, H. (2016). Critical Review of the Safety Assessment of Nano-Structured Silica Additives in Food. J. Nanobiotechnol 14. BioMed Central Ltd. doi:10.1186/s12951-016-0189-6
Yang, W., Gage, H., Jackson, D., and Raats, M. (2018). The Effectiveness and Cost-Effectiveness of Plant Sterol or Stanol-Enriched Functional Foods as a Primary Prevention Strategy for People with Cardiovascular Disease Risk in England: A Modeling Study. Eur. J. Health Econ. 19 (7), 909–922. doi:10.1007/s10198-017-0934-2
Younes, Maged., Younes, M., Aggett, P., Aguilar, F., Crebelli, R., Dusemund, B., et al. (2018). Re‐evaluation of Silicon Dioxide (E 551) as a Food Additive. Efs2 16 (1), e05088. doi:10.2903/j.efsa.2018.5088
Yu, Z.-T., Nanthakumar, N., and Newburg, D. S. (2016). The Human Milk Oligosaccharide 2′-Fucosyllactose Quenches Campylobacter Jejuni-Induced Inflammation in Human Epithelial Cells HEp-2 and HT-29 and in Mouse Intestinal Mucosa. J. Nutr. 146 (10), 1980–1990. doi:10.3945/jn.116.230706
Zhang, A., Mankad, A., and Ariyawardana, A. (2020). Establishing Confidence in Food Safety: Is Traceability a Solution in Consumers' Eyes? J. Consum Prot. Food Saf. 15 (2), 99–107. doi:10.1007/s00003-020-01277-y
Zhang, Y., Bala, V., Mao, Z., Chhonker, Y. S., and Murry, D. J. (2019). A Concise Review of Quantification Methods for Determination of Vitamin K in Various Biological Matrices. J. Pharm. Biomed. Anal. 169, 133–141. doi:10.1016/j.jpba.2019.03.006
Zorn, K. M., Sun, S., Chen, K., Daniel, H., McConnon, C. L., Ma, K., et al. (2021). A Machine Learning Strategy for Drug Discovery Identifies Anti-schistosomal Small Molecules. ACS Infect. Dis. 7 (2), 406–420. doi:10.1021/ACSINFECDIS.0C00754
Keywords: bioactive, food ingredient, functional food, artificial intelligence, characterisation, mass spectrometry, high-throughput screening, discovery
Citation: Doherty A, Wall A, Khaldi N and Kussmann M (2021) Artificial Intelligence in Functional Food Ingredient Discovery and Characterisation: A Focus on Bioactive Plant and Food Peptides. Front. Genet. 12:768979. doi: 10.3389/fgene.2021.768979
Received: 01 September 2021; Accepted: 28 October 2021;
Published: 19 November 2021.
Edited by:
Rosita Gabbianelli, University of Camerino, ItalyReviewed by:
Larry Parnell, Jean Mayer USDA Human Nutrition Research Center on Aging at Tufts University, United StatesBibiana Garcia-Bailo, University of Toronto, Canada
Copyright © 2021 Doherty, Wall, Khaldi and Kussmann. This is an open-access article distributed under the terms of the Creative Commons Attribution License (CC BY). The use, distribution or reproduction in other forums is permitted, provided the original author(s) and the copyright owner(s) are credited and that the original publication in this journal is cited, in accordance with accepted academic practice. No use, distribution or reproduction is permitted which does not comply with these terms.
*Correspondence: Audrey Wall, d2FsbC5hdWRyZXlAbnVyaXRhcy5jb20=
†These authors share first authorship