- 1Department of Gynecology and Obstetrics, the People’s Hospital of China Three Gorges University/The First People’s Hospital of Yichang, Yichang, China
- 2Emergency Services Department, HanYang Hospital Affiliated of Wuhan University of Science and Technology, Wuhan, China
Background: Emerging pieces of evidence demonstrated that the solute carrier family 39 (SLC39A) members are critical for the oncogenic and immune infiltrating targets in multiple types of tumors. However, the precise relationship between the SLC39A family genes and clinical prognosis as well as the pan-cancer tumor cell infiltration has not been fully elucidated.
Methods: In this study, the pan-cancer expression profile, genetic mutation, prognostic effect, functional enrichment, immune infiltrating, and potential therapeutic targets of the SLC39A family members were investigated by analyzing multiple public databases such as the Oncomine, TIMER, GEPIA, cBioPortal, KM-plotter, PrognoScan, GeneMANIA, STRING, DAVID, TIMER 2.0, and CellMiner databases.
Results: The expression levels of most SLC39 family genes in the tumor tissues were found to be significantly upregulated compared to the normal group. In mutation analysis, the mutation frequencies of SLC39A4 and SLC39A1 were found to be higher among all the members (6 and 4%, respectively). Moreover, the overall mutation frequency of the SLC39A family genes ranged from 0.8 to 6% pan-cancer. Also, the function of the SLC39A highly related genes was found to be enriched in functions such as zinc II ion transport across the membrane, steroid hormone biosynthesis, and chemical carcinogenesis. In immune infiltration analysis, the expression level of the SLC39A family genes was found to be notably related to the immune infiltration levels of six types of immune cells in specific types of tumors. In addition, the SLC39A family genes were significantly related to the sensitivity or resistance of 63 antitumor drugs in a variety of tumor cell lines.
Conclusion: These results indicate that the SLC39 family genes are significant for determining cancer progression, immune infiltration, and drug sensitivity in multiple cancers. This study, therefore, provides novel insights into the pan-cancer potential targets of the SLC39 family genes.
Introduction
Cancer has gradually emerged as the leading threat to public health worldwide, as estimated by GLOBOCAN 2020, stating 19.3 million newly confirmed cancer cases and nearly 10 million cancer-related deaths (Ferlay et al., 2021; Sung et al., 2021). Indeed, efforts for cancer prevention, screening, diagnosis, and comprehensive treatment have met with tremendous success in various tumors. However, studies on the clinical outcome of most cancers need further improvisation (Chen et al., 2021). The current promising targeted therapy particularly confirms that exploring the mechanism of pan-cancer initiation, maintenance, and development will unfurl new avenues for fighting various malignant tumors (Loomans-Kropp and Umar, 2019). Therefore, identification of the hub tumor-related genes is very urgent and necessary to develop new diagnostic and prognostic biomarkers and therapeutic targets. Presently, massive high-throughput data and multiple available big data online public databases are greatly helpful for finding the tumorigenic genes and conducting pan-cancer studies in multi-omics (Loomans-Kropp and Umar, 2019; Xiao et al., 2021).
The SLC39A family genes encode a family of proteins belonging to the Zrt- and Irt-like protein (ZIP) transport proteins, having 14 family members (SLC39A1-14). It controls the transportation and influx of zinc, with important roles in multiple signaling pathways and physiological processes, like gene transcription, endocrine regulation, cell growth, cell differentiation, and the immune response process (Kimura and Kambe, 2016; Baltaci and Yuce, 2018). Emerging pieces of evidence indicate that the mutation or functional change in the SLC39A family genes leads to the development and progression of multiple malignancies, such as colorectal cancer, breast cancer, esophageal cancer, hepatocellular carcinoma, pancreas cancer, gastric cancer, prostate cancer, and lung cancer (Hoang et al., 2016; To et al., 2020; Prasad, 2012). Besides, recent multi-omics studies have confirmed certain SLC39A family genes to have differential expression and prognostic value in breast, gastric, and lung cancers, acting as potentially promising clinical markers for these cancers (Liu et al., 2020; Zhou et al., 2021; Ding et al., 2019). Some basic studies have demonstrated the targeted regulation of the SLC39A family genes to be capable of changing the biological characteristics of some tumor cells. For example, Jin et al. found that knockdown of SLC39A5 expression significantly inhibits the invasion, proliferation, and migration of esophageal tumor cells (Jin et al., 2015). In addition, Zhu et al. demonstrated the knockdown of SLC39A11 to attenuate the cellular proliferation of the pancreatic cancer Capan-1 with decreased activation of the ERK1/2 pathway (Zhu et al., 2021). Fan et al. have found SLC39A4 gene knockout to inhibit the malignant behavior of the ovarian tumor cells both in vitro and in vivo (Fan et al., 2017). More importantly, the growing studies have shown that SLC transporters not only directly bring the anticancer drugs into cancer cells but also serve as a medium for the uptake of essential nutrients for the growth and survival of the tumor, thereby regulating the sensitivity and resistance of the chemotherapeutic drugs (Li and Shu, 2014). Nevertheless, the underlying mechanism and biological functions of the SLC39A family genes in the tumor progression and as the potential therapeutic target have not been fully elucidated.
This study systematically performed an in-depth analysis on the expression of the SLC39A family genes and their impact on the prognosis, to explore the relationship between the SLC39A family genes and pan-cancer immune cell infiltration. In addition to utilizing the multi-omics and large sample data analysis, the genetic mutation, function enrichment, and drug sensitivity of the SLC39A family genes were investigated across different cancer types. These analyses could provide a new direction for a promising biomarker and potential targeted therapy for treating cancer.
Materials and Methods
Expression Profiles Analysis
Three online databases (Oncomine, TIMER, and GEPIA) were applied to investigate the differential expression profiles of the SLC39A family genes between the normal and the tumor tissues in various cancer types. The website of the Oncomine online platform is www.oncomine.org (Rhodes et al., 2007), the website of the TIMER online platform is https://cistrome.shinyapps.io/timer/ (Li et al., 2017), and the website of the GEPIA online platform is http://gepia.cancer-pku.cn/ (Tang et al., 2017). Among them, the p-value was set to 0.01; fold change was set to 1.5; the gene level was set to all, and data type was set to mRNA in the Oncomine database, and the relevant parameters of the TIMER and GEPIA databases were set by default.
Mutation Profiles Analysis
The cBioPortal (http://www.cbioportal.org) (Gao et al., 2013) was exploited for detecting the mutation landscape (amplification, deep deletion, and missense mutations) and general mutation count of the SLC39A family genes in 33 types of tumors from the TCGA database. In addition, the impact of gene mutations in the SLC39A family on the clinical outcomes was surveyed using the cBioPortal database.
Survival Analysis
The relationship between the expression of the SLC39A family gene and the overall survival (OS) and progression-free survival (PFS) in the pan-cancer patients was investigated using the pan-cancer module of the KM-plotter database (http://www.kmplot.com/) (Nagy et al., 2021). In addition, the PrognoScan database (http://dna00.bio.kyutech.ac.jp/PrognoScan/index.html) (Mizuno et al., 2009) was further utilized to confirm the relationship between the expression of the SLC39A family genes and clinical outcome in the different cohorts. Multiple types of survival parameters, including OS, PFS, relapse-free survival (RFS), disease-free survival (DFS), distant recurrence–free survival (DRFS), distant metastasis–free survival (DMFS), and disease-specific survival (DSS) were represented in the current analysis. The hazard ratio (HR), log-rank p-value, and 95% confidence interval were directly displayed on the online platform, and the p-value cut-off value was set to 0.05.
Enrichment Analysis
To seek out the highly related genes of SLC39A family genes, the GeneMANIA database (http://www.genemania.org) (Warde-Farley et al., 2010) and the STRING database (https://string-db.org) (Szklarczyk et al., 2019) were exploited. Then, the DAVID database (Database for Annotation, Visualization, and Integrated Discovery, https://david.ncifcrf.gov) (Huang et al., 2009) was used to conduct the GO (gene ontology) annotation and KEGG (Kyoto Encyclopedia of Genes and Genomes) enrichment analysis of the SLC39A family highly related genes.
Immune Infiltration Analysis
The TIMER 2.0 (http://cistrome.shinyapps.io/timer) (Li et al., 2020) was used to evaluate the relationship between the SLC39A family gene expression levels and the infiltration of six common immune cells, including the B cells, CD4+ T cells, CD8+ T cells, Treg T cells, macrophages, and neutrophils.
Drug Sensitivity Analysis
The CellMiner database (https://discover.nci.nih.gov/cellminer/) (Shankavaram et al., 2009) was exploited to evaluate the relationship between the SLC39A family gene expression levels and the compound sensitivity or resistance through the NCI-60 analyses tools. Data processing and Pearson correlation analysis visualization used the limma and ggplot2 package, and the scatter plot showed significant correlations sorted by p-value from small to large, and the p-value cut-off value was set to 0.05.
Results
The Expression Profiles of the SLC39A Family Genes in Pan-Cancer
Subsequently, the expression profiles of the SLC39A family genes were explored in various cancer types. The Oncomine, GEPIA, and TIMER databases were exploited to examine and verify the expression levels of the SLC39A family genes in the tumor tissues and the corresponding non-tumor tissues. The Oncomine database reported an increase in the mRNA expression level of other SLC39 family genes in the tumor tissues compared to the normal control group, except for SLC39A8 (Figure 1A). The median expression of the SLC39A family genes in the tumor tissues of all types of tumors was further compared, revealing that most of the SLC39A family genes show relatively high expression in the specific tumor types, such as lymphoid neoplasm diffuse large B-cell lymphoma (DLBC), esophageal carcinoma (ESCA), glioblastoma multiforme (GBM), head and neck squamous cell carcinoma (HNSC), rectum adenocarcinoma (READ), and thymoma (THYM) (Figure 1B). In addition, the expression level of the SLC39A family genes was detected in 33 tumors, and their normal controls match TCGA normal and GTEx data using GEPIA. Similar to the results of the other studies, the SLC39A family genes have notably increased the expression in most tumors compared to the normal controls (Figure 1C and Supplementary Table S1). As shown in Figure 2, the TIMER2.0 database results demonstrated that the transcriptional expression levels of the SLC39A family genes are inconsistent between the tumor tissues and corresponding normal tissues, and most SLC39A families were over-regulated in the tumor tissues, extremely so in the bladder urothelial carcinoma (BLCA), breast invasive carcinoma (BRCA), cholangiocarcinoma (CHOL), colon adenocarcinoma (COAD), HNSC, kidney renal papillary cell carcinoma (KIRP), lung adenocarcinoma (LUAD), stomach adenocarcinoma (STAD), and thyroid carcinoma (THCA) tumor types.
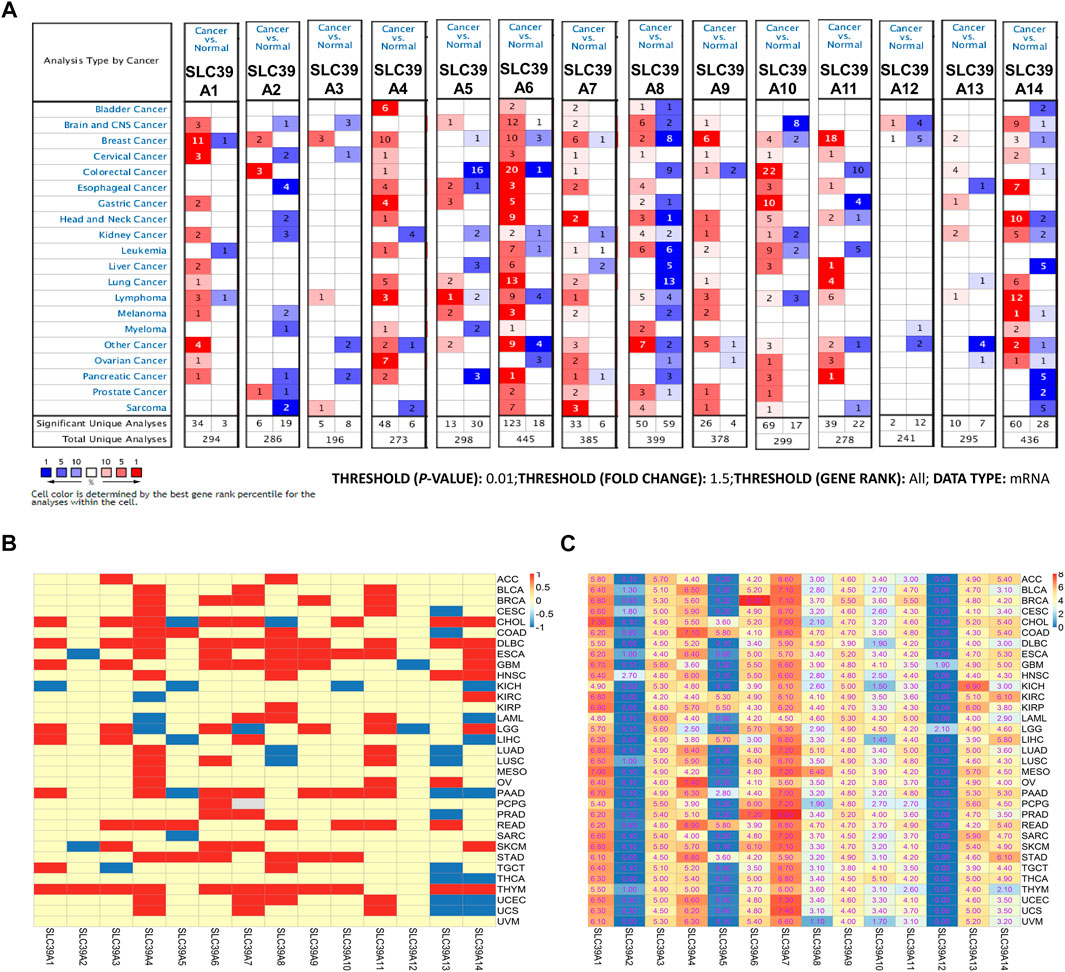
FIGURE 1. mRNA expression profiles of the SLC39A family genes in pan-cancer. (A) Transcriptome expression profile of SLC39A family members in pan-cancer was explored in the Oncomine database. In the graph, red represents statistically significant mRNA overexpression of SLC39A family gene mRNA between the tumor and the corresponding normal tissue, blue represents down-expression, and the number represents the number of data sets. p value is set to 0.01; fold change is set to 1.5; gene level is set to all; and data type is set to mRNA in the Oncomine database. (B) Transcriptome expression profiles of SLC39A family genes in pan-cancer were explored in the GEPIA database. The red boxes represent higher SLC39A family gene expression in tumor tissues, while the green boxes represent the lower SLC39A family gene expression in tumor tissues. The inspection standard is set to p-value < 0.05. (C) Expression level of SLC39A family genes in 33 tumors and their normal controls in the match TCGA normal and GTEx data using GEPIA. The data in the figure represents average mRNA expression of SLC39A family genes in different tumors. The colors from blue to red represent the range of values in the figure.
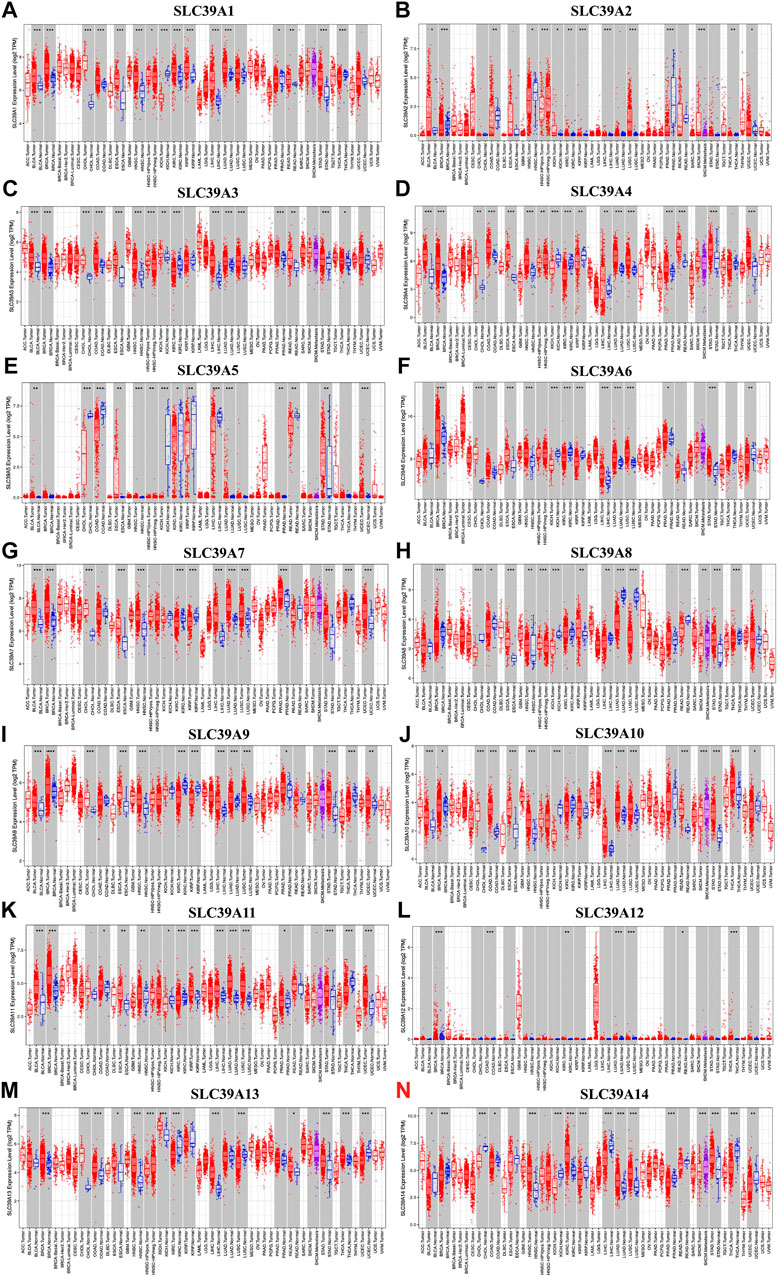
FIGURE 2. Differential expression of the SLC39A family genes in pan-cancer and corresponding normal tissues. (A) Transcriptome expression of SLC39A1 was explored in the TIMER database. (B) Transcriptome expression of SLC39A2 was explored in the TIMER database. (C) Transcriptome expression of SLC39A3 was explored in the TIMER database. (D) Transcriptome expression of SLC39A4 was explored in the TIMER database. (E) Transcriptome expression of SLC39A5 was explored in the TIMER database. (F) Transcriptome expression of SLC39A6 was explored in the TIMER database. (G) Transcriptome expression of SLC39A7 was explored in the TIMER database. (H) Expression of SLC39A8 was explored in the TIMER database. (I) Transcriptome expression of SLC39A 9 was explored in the TIMER database. (J) Transcriptome expression of SLC39A10 was explored in the TIMER database. (K) Transcriptome expression of SLC39A11 was explored in the TIMER database. (L) Transcriptome expression of SLC39A12 was explored in the TIMER database. (M) Transcriptome expression of SLC39A13 was explored in the TIMER database. (N) Transcriptome expression of SLC39A14 was explored in the TIMER database. (*p < 0.05, **p < 0.01, ***p < 0.001. The red box and the green box represent tumor tissue and normal control tissue, respectively. The middle line of the box represents the median and the lower and upper bounds represent the 25th and 75th percentiles, respectively.)
The Genetic Mutation of the SLC39A Family Genes in Pan-Cancer
The cBioPortal and TCGA database was employed to probe the mutation status of the SLC39A family genes in 10,967 samples in 32 studies of the pan-cancer atlas. Results showed that the mutation frequencies of SLC39A4 and SLC39A1 were higher than those of all the other members, 6 and 4%, respectively, and the overall mutation frequency of the SLC39A family genes ranged from 0.8 to 6% (Figure 3A). As shown in Figure 3B, the mutation frequency of the SLC39A family genes in ovarian serous cystadenocarcinoma (OV), liver hepatocellular carcinoma (LIHC), ESCA, uterine corpus endometrial carcinoma (UCEC), LUAD, skin cutaneous melanoma (SKCM), BLCA, uterine carcinosarcoma (UCS), lung squamous cell carcinoma (LUSC), STAD, and BRCA was relatively higher by more than 30%, and the other types of tumors all exhibited a very low alteration in mutation (<30%). In addition, the Kaplan–Meier plotter results demonstrated that the combined mutation of the SLC39A family genes has no significant effect on OS (p-values, 0.0664) (Figure 3C). However, there are statistical differences in the DSS, PFS, and DFS between the mutation group and the non-mutation group of the SLC39A family genes (p-values, 0.0308, 4.11e-5, and 7.94e-11, respectively) (Figures 3D–F).
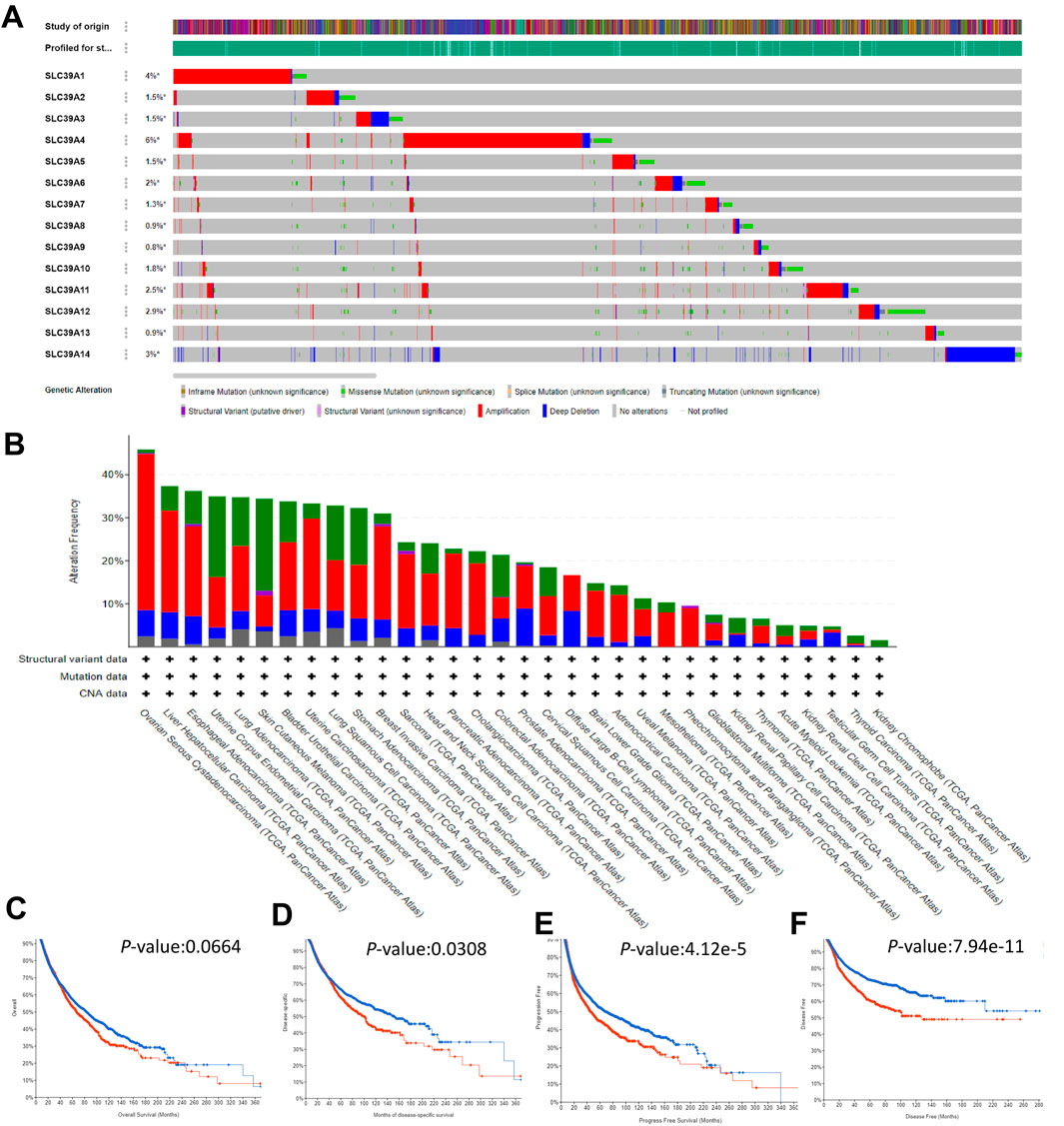
FIGURE 3. Mutation landscape of the SLC39A family genes in pan-cancer derived from the cBioPortal platform. (A) OncoPrint summary of alteration on SLC39A family genes in 10,967 numbers of samples in 32 studies of the pan-cancer atlas. The mutation frequency was shown as green for mutations, red for fusions, blue for sions, and black for multiple mutations. (B) Rectangular graph of the general mutation counts of SLC39A family genes in the pan-cancer atlas. The X- and Y-axis represent the mutation frequency of SLC39A family genes and cancer type, respectively. It was shown as green for missense mutations, violet for fusions, deep blue for truncating, and blue for no mutations. (C) Kaplan–Meier chart of OS of pan-cancer with and without SLC39A family gene mutation. (D) Kaplan–Meier chart of DDS of pan-cancer with and without SLC39A family gene mutation. (E) Kaplan–Meier chart of PFS of pan-cancer with and without SLC39A family gene mutation. (F) Kaplan–Meier chart of DFS of pan-cancer with and without SLC39A family gene mutation. The red line and the blue line represent high and low expression, respectively. The Cox p-value cut-off value was set to 0.05.
The Prognostic Value of SLC39A Family Genes in Pan-Cancer
The association between the mRNA expression of the SLC39A family genes and the clinical outcomes in pan-cancer patients were analyzed using the KM-plotter and PrognoScan databases. As shown in Figure 4, the KM-plotter database revealed that the expression of the SLC39A family genes was significantly related to the OS and RFS in some tumor types. Among them, the high expression of most of the SLC39A family genes presents the risk factor for the OS of BLCA, CESC, HNSC, LIHC, LUAD, LUSC, and PAAD, as well as for the protection factors for OS of BRCA, ESCA, OV, PAAD, STAD, TGCT, and THCA. Similarly, for RFS, the high expression of most SLC39A family genes was significantly related to the inferior survival of BLCA, CESC, KIRP, LUAD, LUSC, PAAD, and TGCT, as well as the better prognosis of BRCA, OV, PCPG, and STAD (Figures 4A,B and Supplementary Tables S2, S3). As shown in Figures 4C–R, the increase in the expression of SLC39A1, SLC39A 3, SLC39A 5, SLC39A 8, SLC39A 10, SLC39A 13, and SLC39A 14 was associated with poor OS, and the upregulation of SLC39A1, SLC39A 4, SLC39A 7, SLC39A 9, and SLC39A 10 was found to lead to poor RFS in the patients with CESC.
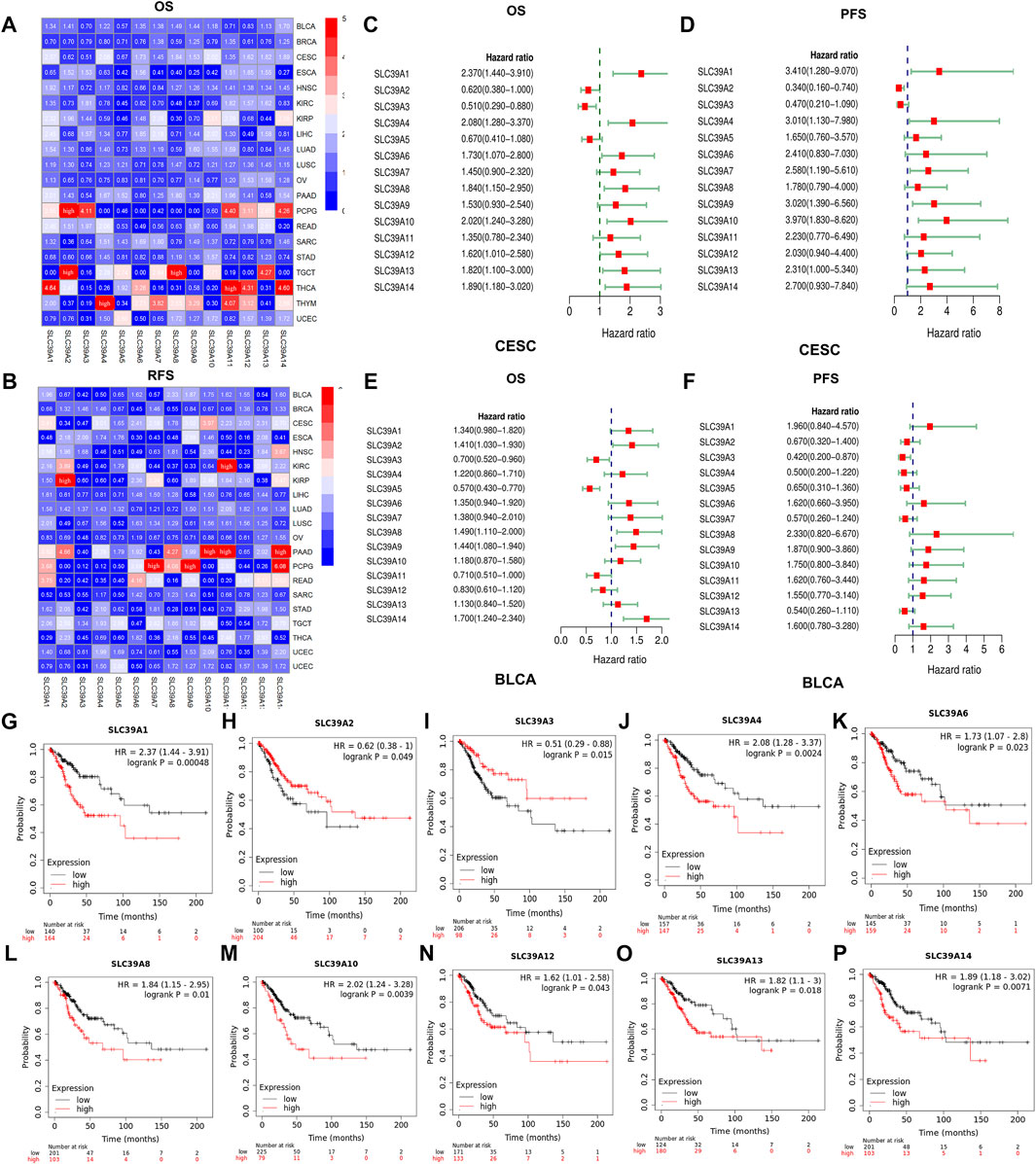
FIGURE 4. Survival analysis of the prognostic value of the SLC39A family genes in pan-cancer derived from the KM-plotter dataset. (A) Heat map shows the hazard ratio (HR) value of the OS in pan-cancer calculated using the KM-plotter database. (B) Heat map shows the hazard ratio (HR) value of the RFS in pan-cancer calculated using the KM-plotter database. (The colors from blue to red represent the range of HR values in the figure. HRs over 5 were replaced with “high.”) (C) Forest plot quantitatively synthesizes the HR and 95% confidence interval of the OS of the SLC39A gene family in CESC. (D) Forest plot quantitatively synthesizes the HR and 95% confidence interval of the PFS of the SLC39A gene family in CESC. (E) Forest plot quantitatively synthesizes the HR and 95% confidence interval of the OS of the SLC39A gene family in BLCA. (F) Forest plot quantitatively synthesizes the HR and 95% confidence interval of the PFS of the SLC39A gene family in BLCA. (G) Survival curve of SLC39A1 on the OS in CESC. (H) Survival curve of SLC39A2 on the OS in CESC. (I) Survival curve of SLC39A3 on the OS in CESC. (J) Survival curve of SLC39A4 on the OS in CESC. (K) Survival curve of SLC39A6 on the OS in CESC. (L) Survival curve of SLC39A8 on the OS in CESC. (M) Survival curve of SLC39A10 on the OS in CESC. (N) Survival curve of SLC39A12 on the OS in CESC. (O) Survival curve of SLC39A13 on the OS in CESC. (P) Survival curve of SLC39A14 on the OS in CESC. The red line and the blue line represent high and low expression, respectively. The Cox p-value cut-off value was set to 0.05.
Then, the PrognoScan platform was used to further assess and verify the prognostic value of the SLC39A family genes in pan-cancer, based on public datasets. The results of PrognoScan indicated that the expression level of the SLC39A family genes was significantly related to the clinical survival of 12 types of tumors, such as colorectal cancer, breast cancer, bladder cancer, lung cancer, ovarian cancer, blood cancer, brain cancer, skin cancer, eye cancer, soft tissue cancer, prostate cancer, and head and neck cancer (Supplementary Table S4). Interestingly, most of the studies and data sets were previously focused on breast, colorectal, lung, and ovarian cancer. The results of the quantitative synthesis of related studies showed that higher expression of the SLC39A family genes indicated a worse survival prognosis for RFS ([HR] = 1.30, 95% confidence interval [CI] = 1.10 to 1.53) and DSS (HR = 1.60, 95% CI = 1.27 to 2.02) in breast cancer (Figures 5A,B). However, the higher expression of the SLC39A family genes was associated with a better prognosis for OS (HR = 0.62, 95% CI = 0.44 to 0.88) and DFS (HR = 0.75, 95% CI = 0.62 to 0.90) in colorectal cancer (Figures 5C,D). In addition, the upregulation of the SLC39A family gene expression was significantly associated with poor OS (HR = 2.12, 95% CI = 1.45 to 3.05) and RFS (HR = 1.79, 95% CI = 1.26 to 2.54) in lung cancer.
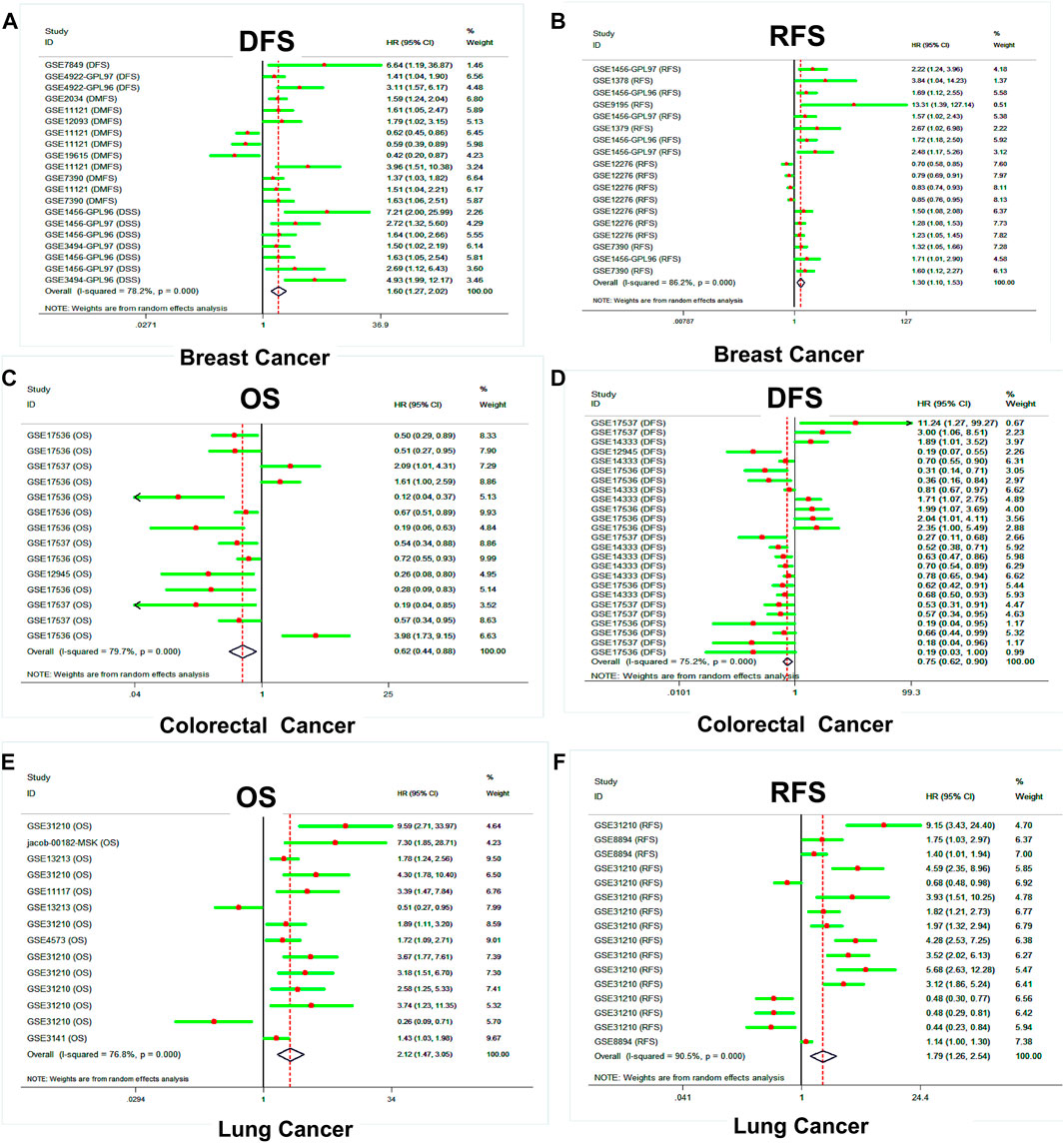
FIGURE 5. Survival analysis of the prognostic value of the SLC39A family genes in pan-cancer derived from the PrognoScan dataset. (A) Forest plot of DDS shows the effect of SLC39A family gene expression on the clinical prognosis of breast cancer. (B) Forest plot of RFS shows the effect of SLC39A family gene expression on the clinical prognosis of breast cancer. (C) Forest plot of OS shows the effect of SLC39A family gene expression on the clinical prognosis of colorectal cancer. (D) Forest plot of DFS shows the effect of SLC39A family gene expression on the clinical prognosis of colorectal cancer. (E) Forest plot of OS shows the effect of SLC39A family gene expression on the clinical prognosis of lung cancer. (F) Forest plot of DFS shows the effect of SLC39A family gene expression on the clinical prognosis of lung cancer.
The Function Enrichment of the SLC39A Family Genes in Pan-Cancer
To investigate the potential mechanism of the SLC39A family genes affecting the prognosis and progression of tumors, the protein–protein interactions (PPIs) of the SLC39A family highly related genes were conducted by using the STRING and GeneMANIA platforms. The STRING web was used to conduct the protein–protein interaction (PPI) network analysis of the SLC39A family genes. As expected, 54 nodes and 410 edges were obtained in the PPI network, and the 10 top-ranked node genes were CBR4, MCAT, CYP17A1, HSD17B6, HEPH, SRD5A1, HSD17B3, CYP11B2, CYP11B1, and CYP19A1 (Figure 6A; Supplementary Table S5 and Supplementary Figure S1). In addition, the GeneMANIA results revealed that a total of 34 genes (including the SLC39A family genes) are associated with co-expression, genetic interactions, physical interactions, and shared protein domains. Among them, relationships of co-expression were predicted between SLC39A1 and SLC39A13, SLC39A1 and SLC39A7, SLC39A4 and SLC39A14, SLC39A5 and SLC39A14, SLC39A5 and SLC39A4, SLC39A6 and SLC39A10, and SLC39A8 and SLC39A14. Genetic interactions were predicted between SLC39A9 and SLC39A8, SLC39A9 and SLC39A3, SLC39A11 and SLC39A13, and SLC39A11 and SLC39A14. Moreover, SLC39A1 and SLC39A2, SLC39A5 and SLC39A10, SLC39A5 and SLC39A6, and SLC39A9 and SLC39A2 were found to share physical interactions. Most of the SLC39A family genes were found to share protein domains (Figure 6B).
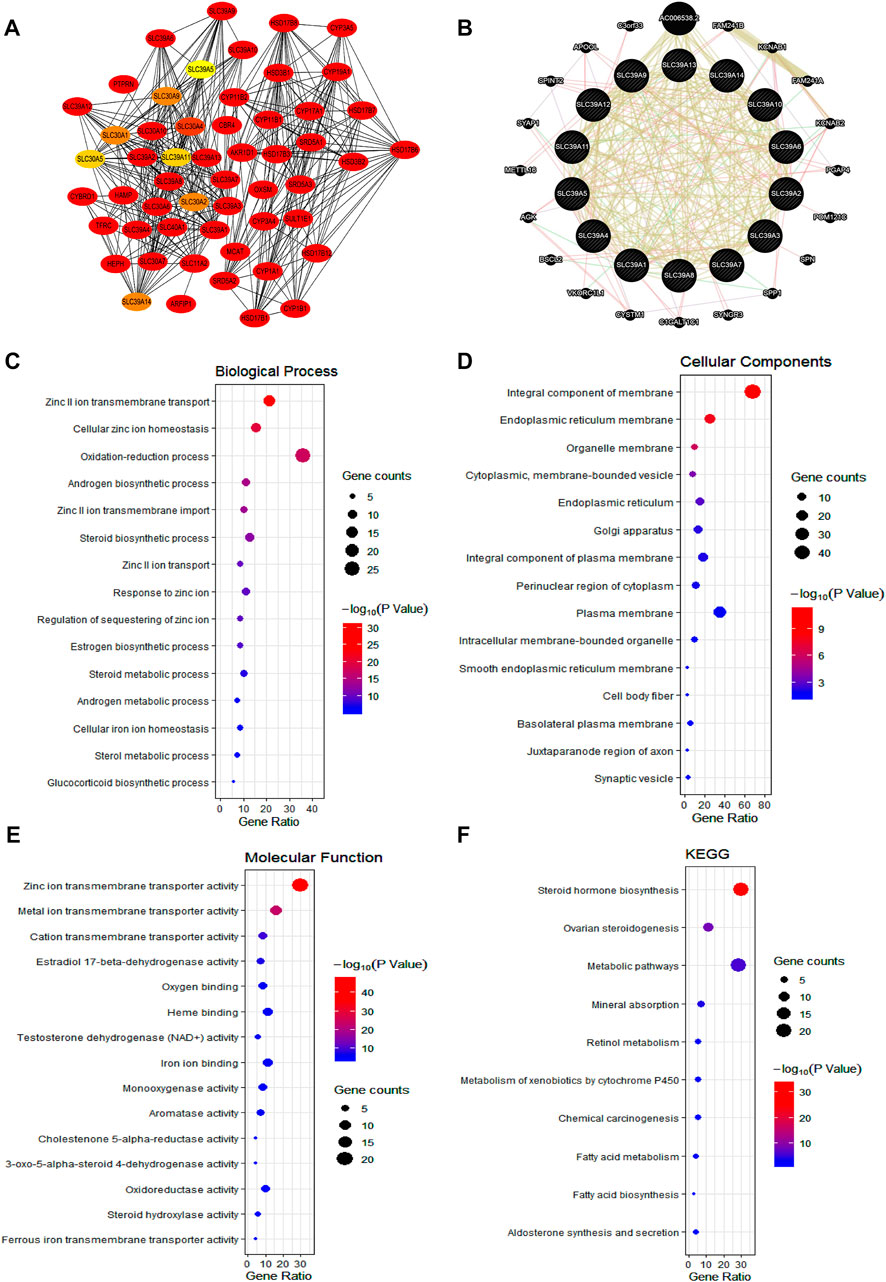
FIGURE 6. Function enrichment of the SLC39A family genes in pan-cancer. (A) Protein–protein interaction of SLC39A family in the STRING dataset. (B) Gene–gene interaction network among SLC39A family members in the GeneMANIA dataset. (C) Bubble chart showing the BP of SLC39A family highly correlated genes. (D) Bubble chart showing the CC of SLC39A family highly correlated genes. (E) Bubble chart showing the MF of SLC39A family highly correlated genes. (H) Bubble chart showing the KEGG of SLC39A family highly correlated genes.
The functional enrichment of the SLC39A family’s highly related genes was predicted by analyzing the GO annotation and KEGG pathway via the DAVID platform. According to the results (Figures 6C–F and Table 1), the function of the SLC39A highly related genes was enriched in the zinc II ion transmembrane transport, cellular zinc ion homeostasis, oxidation-reduction process, androgen biosynthetic process, and zinc II ion transmembrane import in biological processes (BPs). As for the cellular component (CC), the SLC39A family highly related genes were enriched in the integral components of the membrane, endoplasmic reticulum membrane, organelle membrane, cytoplasmic, membrane-bounded vesicle, and endoplasmic reticulum. Moreover, the SLC39A family influenced molecular functions through histone methyltransferase binding. With respect to the molecular function (MF), the SLC39A family of highly related genes was enriched in the zinc ion transmembrane transporter activity, metal ion transmembrane transporter activity, cation transmembrane transporter activity, estradiol 17-beta-dehydrogenase activity, and oxygen binding. Meanwhile, in the KEGG analysis, 10 pathways were significantly enriched, including the steroid hormone biosynthesis, ovarian steroidogenesis, metabolic pathways, mineral absorption, retinol metabolism, metabolism of xenobiotics by cytochrome P450, chemical carcinogenesis, fatty acid metabolism, fatty acid biosynthesis, and aldosterone synthesis and secretion.
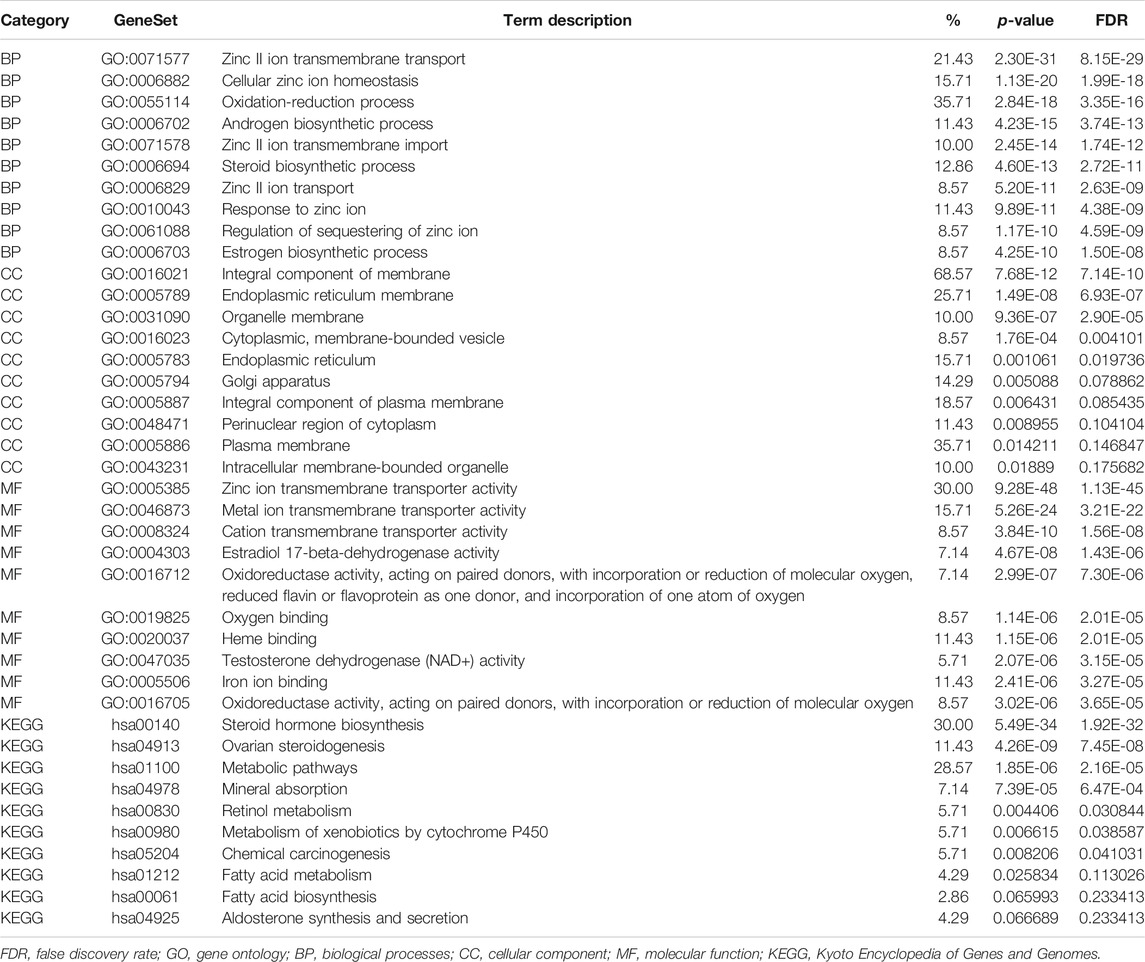
TABLE 1. Top 10 GO and KEGG functional enrichment of SLC39A family highly related genes in pan-cancer derived from STRING, GENEMAIN, and DAVID datasets.
The Immune Infiltration of the SLC39A Family of Genes in Pan-Cancer
To explore whether the SLC39A family genes affect the tumor immune infiltrating and microenvironment in pan-cancer, the TIMER 2.0 was used to evaluate the relationship between the SLC39A family gene expression levels and the infiltration of six common immune cells, including B cells, CD4+ T cells, CD8+ T cells, Treg T cells, macrophages, and neutrophils. The results confirmed a positive correlation between B-cell infiltration in ACC, KICH, KIRP, LIHC, PCPG, and PRAD but found a negative relation to B-cell immunity in COAD, DLBC, HNSC, KIRC, OV, SKCM, TGCT, TKYM, UCEC, and UVM (Figure 7A). For the CD4+ T cell, most of the SLC39A family members were positively related to immune infiltration in ESCA, GBM, HNSC, LIHC, and TCGT, while most of the SLC39A family genes were negatively correlated with the immune infiltration in BRCA and THYM (Figure 7B). For the CD8+ T cell, most of the SLC39A family genes were positively correlated with immune infiltration in the ACC, BLCA, DLBC, KICH, PRAD, and UVM, while most of the SLC39A family genes were negatively correlated with the immune infiltration in the HNSC, THYM, and UCEC (Figure 7C). Besides, a positive correlation was observed in most SLC39A family genes and Treg cell infiltration in TGCT and UVM, and a negative correlation was observed in the DLBC and THYM (Figure 7D). Particularly, a negative correlation was observed in most SLC39A family genes and macrophage cell infiltration in the BRCA, DLBC, UCEC, and UVM (Figure 7E). In addition, a positive correlation was observed in most of the SLC39A family genes and neutrophil infiltration (Figure 7F). It is noteworthy that the SLC39A2, SLC39A3, SLC39A3, and SLC39A5 showed a significant negative correlation with the macrophages and neutrophil cell infiltration in most tumor types.
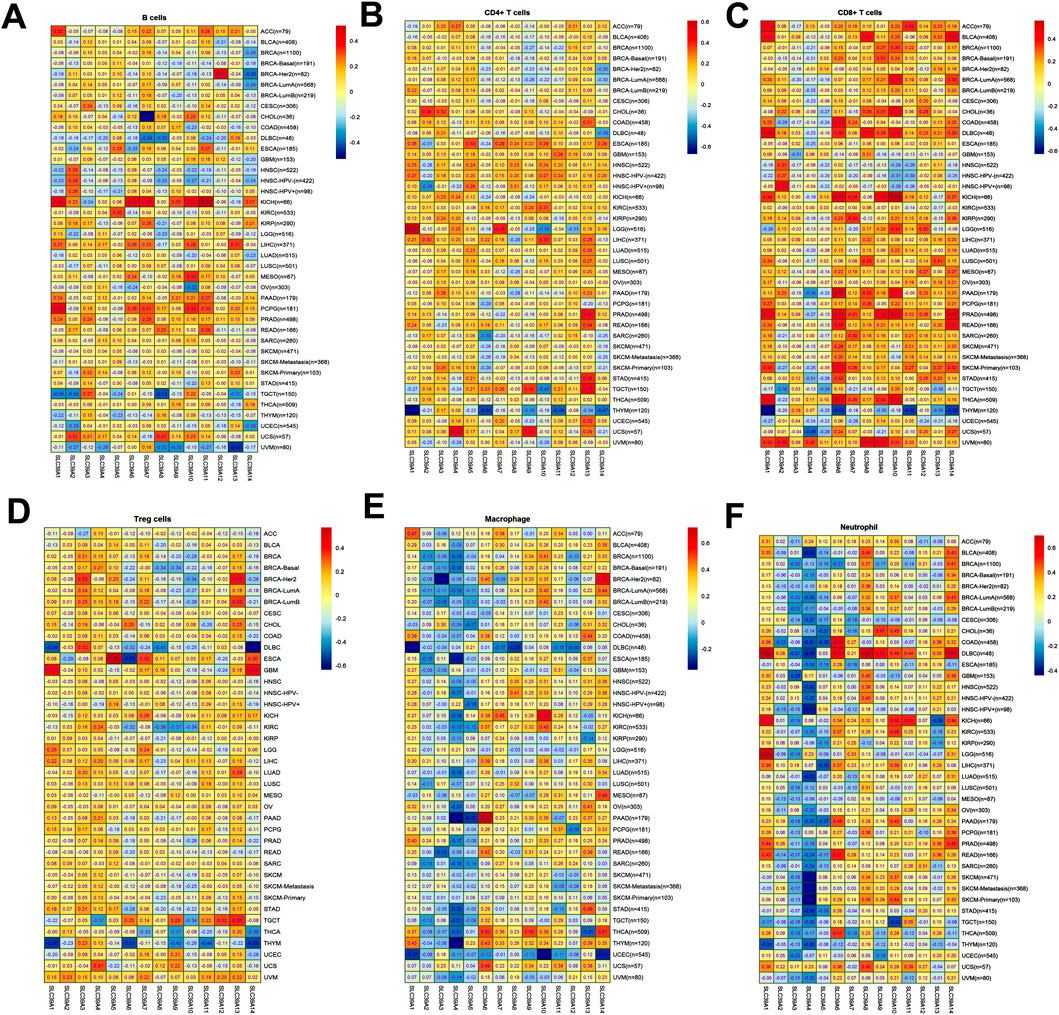
FIGURE 7. Immune cell infiltration of the SLC39A family genes in pan-cancer derived from the TIMER2.0 dataset. (A) Correlation coefficient between SLC39A family gene expression and B-cell infiltration score in pan-cancer. (B) Correlation coefficient between SLC39A family gene expression and CD4+ T-cell infiltration score in pan-cancer. (C) Correlation coefficient between SLC39A family gene expression and CD8+ T-cell infiltration score in pan-cancer. (D) Correlation coefficient between SLC39A family gene expression and Treg T-cell infiltration score in pan-cancer. (E) Correlation coefficient between SLC39A family gene expression and macrophage cell infiltration score in pan-cancer. (F) Correlation coefficient between SLC39A family gene expression and neutrophil cell infiltration score in pan-cancer. The association was generated with tumor purification adjusted.
Drug Sensitivity Analysis of the SLC39A Family Genes in Pan-Cancer
To explore the potential sensitization or the effects of drug resistance of the SLC39A family genes on the drug response of different human cancer cell lines, a Pearson correlation analysis was performed between the mRNA expression of the SLC39A family genes in the NCI-60 cancer cell line and the drug activity of 263 antitumor drugs (Figure 8; Table 2; and Supplementary Figure S2). The results demonstrated the upregulation of SLC39A1 expression to reduce the drug sensitivity of imisone, oxaliplatin, ifosfamide, eribulin mesylate, palbociclib, and paclitaxel but enhanced the drug sensitivity of Irofulven. An increase in the SLC39A2 expression enhanced the drug sensitivity of Isotretinoin, and the sensitivity of cladribine was found to increase by SLC39A. Various tumor cells with high expression of SLC39A4 were found to be more resistant to the okadaic acid and are more sensitive to 8-chloroadenosine and allopurinol. An increase in the SLC39A5 expression enhanced the drug sensitivity of tegafur, fluorouracil, and BML-277. Notably, the upregulation of SLC39A6 expression was found to increase the sensitivity of raloxifene and fulvestrant. High SLC39A7 expression was found to increase the drug resistance of oxaliplatin, palbociclib, dexrazoxane, entinostat, carfilzomib, epirubicin, and teniposide. However, an elevation in the SLC39A8 gene expression was found to enhance the drug sensitivity of nelarabine, fluphenazine, chelerythrine, fenretinide, imexon, hydroxyurea, cyclophosphamide, and pipobroman. In addition, the SLC39A10 gene expression was found to increase the drug sensitivity of gefitinib, afatinib, erlotinib, lapatinib, vandetanib, ibrutinib, and bosutinib and also increased the tolerance of cell lines to elesclomol, paclitaxel, tyrothricin, and vinorelbine. The SLC39A12 gene expression increased the drug sensitivity of PD-98059, vemurafenib, selumetinib, hypothemycin, and dabrafenib and also increased the tolerance of the cell lines to dasatinib. The expression of SLC39A13 was found to reduce the drug sensitivity of the by-product of CUDC-305, vinorelbine, eribulin mesilate, paclitaxel, oxaliplatin, actinomycin D, nilotinib, homoharringtonine, LDK-378, vinblastine, dolastatin 10, tamoxifen, imatinib, AFP464, tanespimycin, crizotinib, palbociclib, and carfilzomib and enhance the drug sensitivity of simvastatin. At last, the expression of the SLC39A14 gene was also found to increase the resistance of multiple drugs, including AFP464, panobinostat, cyclophosphamide, palbociclib, lificguat, and fulvestrant. On the other hand, the SLC39A14 gene expression was found to increase the drug sensitivity of entinostat.
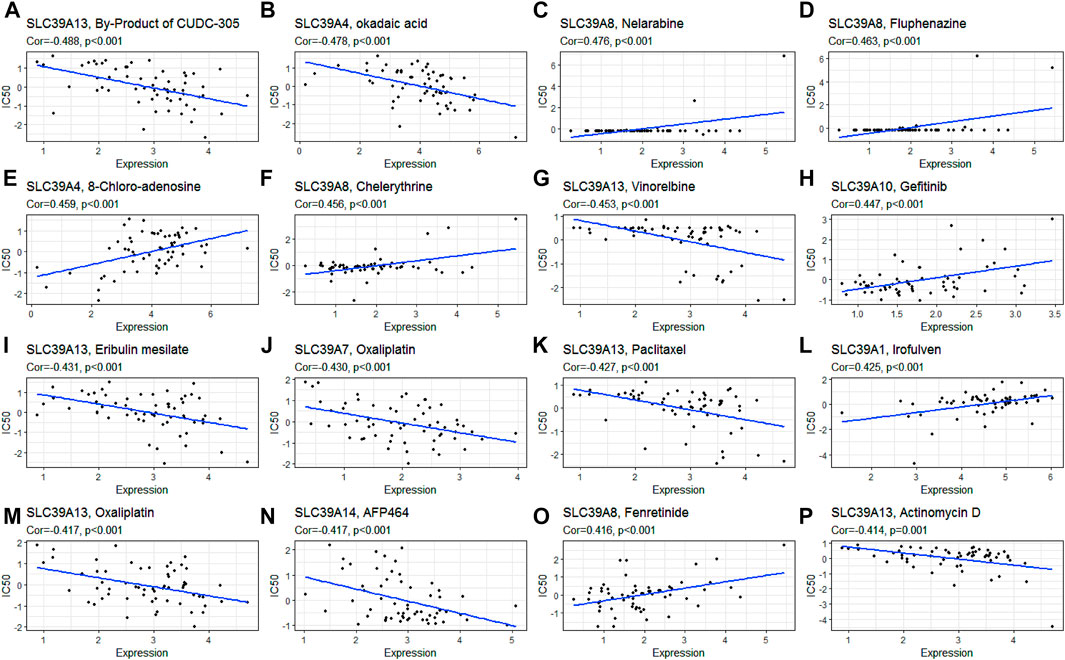
FIGURE 8. Association of the SLC39A family gene expression with the drug sensitivity derived from the NCI-60 cell line data. (A) Scatter plot of negative correlation between SLC39A13 expression and the sensitivity of the by-product of CUDC-305. (B) Scatter plot of negative correlation between SLC39A4 expression and the sensitivity of okadaic acid. (C) Scatter plot of positive correlation between SLC39A8 expression and the sensitivity of nelarabine. (D) Scatter plot of positive correlation between SLC39A8 expression and the sensitivity of fluphenazine. (E) Scatter plot of positive correlation between SLC39A4 expression and the sensitivity of 8-chloro-adenosine. (F) Scatter plot of negative correlation between SLC39A13 expression and the sensitivity of chelerythrine. (G) Scatter plot of positive correlation between SLC39A4 expression and the sensitivity of vinorelbine. (H) Scatter plot of positive correlation between SLC39A10 expression and the sensitivity of gefitinib. (I) Scatter plot of negative correlation between SLC39A13 expression and the sensitivity of eribulin mesilate. (J) Scatter plot of negative correlation between SLC39A7 expression and the sensitivity of oxaliplatin. (K) Scatter plot of negative correlation between SLC39A13 expression and the sensitivity of paclitaxel. (L) Scatter plot of positive correlation between SLC39A1 expression and the sensitivity of Irofulven. (M) Scatter plot of negative correlation between SLC39A13 expression and the sensitivity of oxaliplatin. (N) Scatter plot of negative correlation between SLC39A14 expression and the sensitivity of AFP464. (O) Scatter plot of positive correlation between SLC39A8 expression and the sensitivity of fenretinide. (P) Scatter plot of negative correlation between SLC39A13 expression and the sensitivity of actinomycin D. Z-score from test by Pearson’s correlation using NCI-60 cell line data.
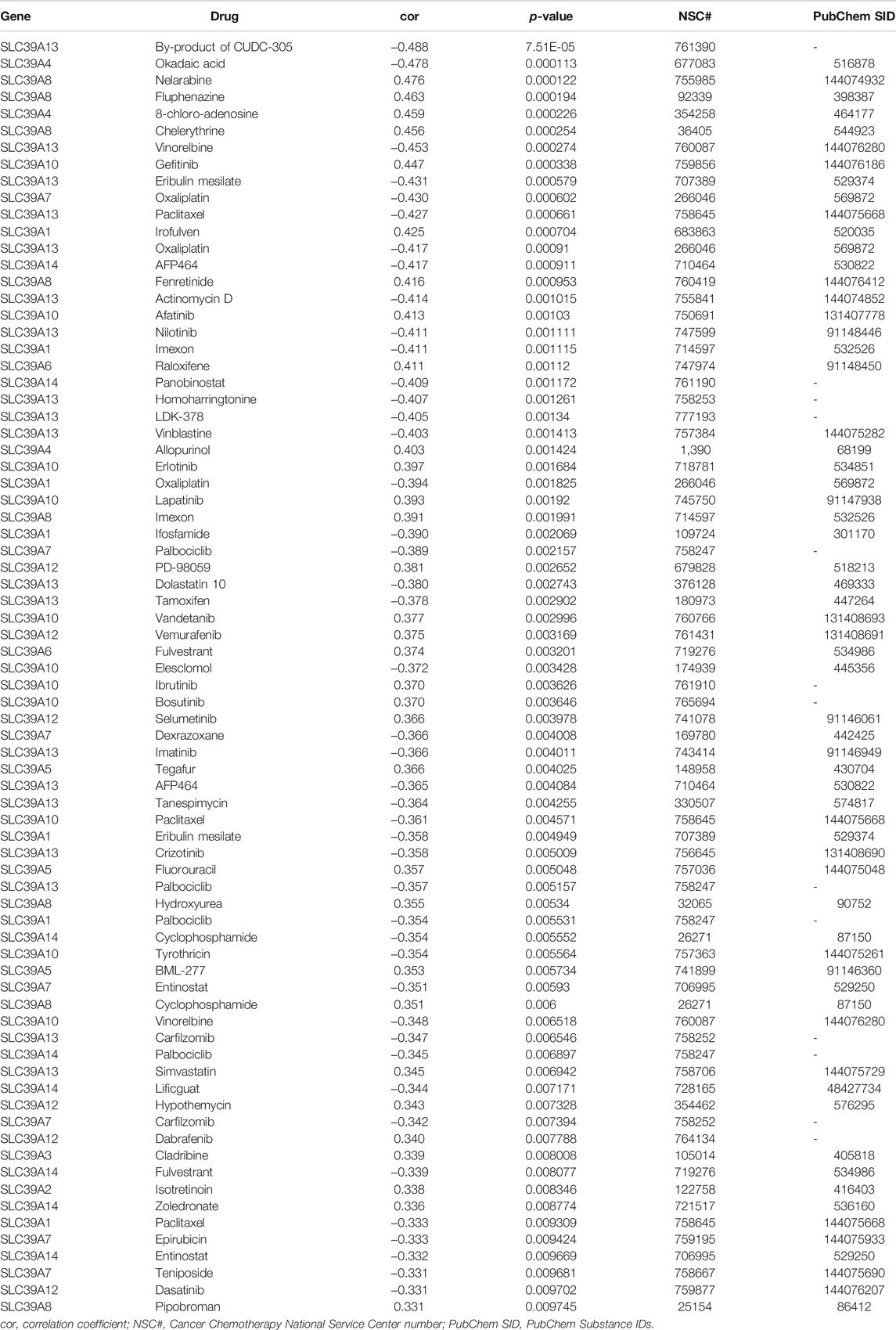
TABLE 2. Relationship between SLC39A family gene expression and drug sensitivity based on the NCI-60 cell line.
Discussion
Zinc, as an essential trace element, participates in various physiological events, such as growth, differentiation, development, immunity, apoptosis, and other physiological processes (Kimura and Kambe, 2016). Previous studies have reported that zinc is required for over 300 enzymes’ activity and 2,000 transcription factors to work (Prasad, 2012). Thus, zinc metabolism and homeostasis regulate the normal cell functions in a complex manner. Aberrant Zn transporters have been reported to contribute to specific diseases, including endocrine diseases, neurodegenerative diseases, metabolic diseases, cardiovascular diseases, immune deficiencies, and cancers (Prasad, 2012; Kambe et al., 2014). In particular, current evidence suggests that zinc deficiency and dysregulation of the zinc metabolism are risk factors for tumorigenesis, and zinc is considered a tumor-suppressive agent and a potential tumor treatment target (Grattan and Freake, 2012; Pan et al., 2017; Zhang et al., 2021). In addition, the two groups of zinc transporters, the ZnT transporter (SLC30A) and the ZIP channel (SLC39A), exert strict control over the concentration of zinc in cells, and the SLC39A are known to operate in the influx of zinc across the cytoplasm from the extracellular environment into the cytosol (Hojyo and Fukada, 2016; Pan et al., 2017; Brito et al., 2020). The available data suggest that the mutation or functional change of the SLC39A family of genes develops various diseases such as the tumors of the digestive system, urinary system, and reproductive tract (Prasad, 2012; Hoang et al., 2016; To et al., 2020). Interestingly, the SLC39A family gene knockout animals have revealed many unique phenotypes and the possibility of the clinical targeted application and the possibility of discovery and development of the SLC39A family inhibitors as anticancer drugs and modulators regulating the sensitivity or resistance of chemotherapeutic drugs (Geng et al., 2018; Hu, 2020; Mohammadinejad et al., 2020; Cheng et al., 2021). However, the precise function of the SLC39A family genes in pan-cancer has not been comprehensively determined.
In the current study, compared to the normal control group, the expression levels of most of the SLC39 family genes in the tumor tissues were found to be significantly upregulated. The high expression of SLC39A6 is a dependable marker for breast cancer (luminal A subtype), and elevated SLC39A10 mRNA levels were evident in the cancer cell lines of the highly aggressive breast cancer (Kagara et al., 2007; Hogstrand et al., 2013). Cheng et al. (2017) have found the ESCC tissues to possess an increased mRNA expression level of SLC39A6 compared to the non-tumor tissues. Studies by Li et al. (2007) reported that compared to the human pancreatic ductal epithelium (HPDE) cells, the expression of the SLC39A4 mRNA was significantly increased in the human pancreatic cancer cells. In addition, a bioinformatics study also found increased expression levels of the SLC39A family genes with significant upregulation in the breast cancer, gastric cancer, and lung cancer tissues compared to the normal breast tissues (Ding et al., 2019; Liu et al., 2020; Zhou et al., 2021). There are not many studies on the genetic mutations of the SLC39A family in tumor tissues. Our study reported that the mutation frequencies of the SLC39A4 and SLC39A1 were the highest among all the members and were 6 and 4%, respectively. The overall mutation frequency of the SLC39A family genes was in the general range of 0.8–6.0% in pan-cancer. Moreover, the mutations in the SLC39A family genes were found to have a significant impact on the DSS, PFS, and DFS of the malignant tumor.
For prognostic analysis, the expression of SLC39A family genes was found to be significantly related to the OS and RFS in multiple types using the KM-plotter database. Most SLC39A family genes showed protective effects in BLCA, CESC, HNSC, BRCA, ESCA, and OV. Simultaneously, the PrognoScan results indicated that the SLC39A family gene expression levels were significantly correlated with the prognosis of the colorectal, breast, bladder, lung, ovarian, blood, brain, skin, eye, soft tissue, prostate, and head and neck cancers. Our findings were consistent with those of the previous study, in that high mRNA expression levels of SLC39A6 and SLC39A14 indicated favorable OS, but upregulated SLC39A2-5, SLC39A7, and SLC39A12-13 were associated with poor OS in the patients with breast carcinoma (Liu et al., 2020). Higher expression of SLC39A1, 5–7, and 9 indicated better OS, FPS, and PPS, and increased SLC39A2–4, 8, and SLC39A10 expression indicated poor OS, FP, and PPS in the patients with gastric cancer (Ding et al., 2019). In addition, an increase in the SLC39A7 expression was related to better OS, while the upregulated level of SLC39A3 and SLC39A4 were associated with inferior OS in patients with LUSC (Zhou et al., 2021). Consistent with previous research, the GO function enrichment indicated the SLC39A family genes and their highly related genes to contribute to zinc transport– and homeostasis-related biological processes, such as zinc II ion transmembrane transport, cellular zinc ion homeostasis, oxidation-reduction, androgen biosynthesis, and zinc II ion transmembrane import. The KEGG analysis showed SLC39A family genes to be involved in the hormone regulation, metabolic pathways, mineral absorption, and chemical carcinogenesis pathways (Ding et al., 2019; Zhou et al., 2021). Therefore, the prognostic effect of the SLC39A family genes can be speculated to be closely related to zinc transfer, metabolism, and function (Guo and He, 2020).
Increasing studies have shown that zinc is involved in a variety of important functions of immune cell activation and initiation of immune response in the process of innate immunity and adaptive immunity; thus, zinc deficiency can lead to immune dysfunction (Bin et al., 2018). Given the close relationship between the SLC39A family and zinc transport, the regulatory relationship between the SLC39A family genes and immune infiltration deserves more attention. SLC39A6 and SLC39A10 are the first zinc transporters reported to regulate immune cell functions in mammals. Subsequent studies have confirmed the role of SLC39A8 in regulating various immune cells and playing an irreplaceable role in the process of innate immunity (Kitamura et al., 2006; Liu et al., 2013). The study of Hojyo et al. (2014) reported the SLC39A10 expression to be upregulated in pro-B lymphocytes and SLC39A10 to participate in B-cell immunity by leading the homeostasis and the function of the B cells. Our findings suggested a significant correlation between the SLC39A family gene expression and B-cell infiltration in broad cancer types and CD4+ T cells, CD8+ T cells, Treg T cells, macrophages, and neutrophils in specific tumors. These results provide new possibilities for immunotherapy to improve the prognosis by modulating the SLC39A family genes on the tumors or immune cells. Presently, there have been a few attempts of transformational application research using the SLC39A family to treat or alleviate diseases in animals or cell models. One study showed that in an in vivo xenograft model, the overexpression of the SLC39A1 leads to an increased zinc uptake, reducing the tumor growth (Golovine et al., 2008). Utilizing the characteristics of the zinc SLC39A6 transporter widely expressed in all breast cancer subtypes, Seattle Genetics has designed and constructed a new antibody–drug conjugate called SGN-LIV1A to treat metastatic breast cancer through the targeted regulation of SLC39A6 (Sun et al., 2011). In our study, the Pearson correlation analysis was performed between the mRNA expression of the SLC39A family genes in the NCI-60 cancer cell line and the drug activity of 263 antitumor drugs. The results showed that SLC39A family genes are significantly related to the sensitivity or resistance of 63 antitumor drugs in a variety of tumor cell lines. Among them, SLC39A13 and the by-product of the CUDC-305, SLC39A4, okadaic acid, SLC39A8, and nelarabine are the three most likely connections. Based on these results, the detection and targeted regulation of the expression of the SLC39A family gene have been found to have special potential value for the clinical selection of antitumor drugs.
Despite being the first one to perform a multidimensional and multi-omics analysis of the SLC39A family genes in pan-cancer, this study has some shortcomings worth considering. First, the bioinformatics analysis was carried out through multiple online big data databases, and further in vitro and in vivo experiments are required to verify the prediction results. Second, multiple databases are not completely consistent in the expression and survival prognosis of the SLC39A family genes in certain tumors. Large samples, different populations, and multicenter clinical studies need further clarification. Third, although we have confirmed that the SLC39A family gene expression was significantly related to the immune infiltration and survival outcome of a variety of tumors, the causal relationship between the immune infiltration and prognosis remains elusive. Fourth, analyzing the level of immune infiltrating cells at the tumor tissue level may be error-prone, and hence, single-cell sequencing may be required for further exploration.
Conclusion
This pan-cancer study performed a comprehensive and systematic investigation of the expression patterns, genetic mutation, prognostic value, function enrichment, immune infiltrating, and potential therapeutic targets of the SLC39A family of genes. Our results proved that most of the SLC39 family genes’ expression was significantly increased in the tumor tissues and was associated with clinical prognosis in pan-cancer. Moreover, the SLC39A family gene expression was significantly related to the immune cell infiltration levels of six types of immune cells and contributed to the sensitivity or resistance of the drugs in specific types of tumors. Thus, we concluded that the SLC39A of family genes may be crucial for tumorigenesis, the tumor microenvironment, and drug sensitivity, providing novel ideas to develop new targeted therapy for malignant tumors.
Data Availability Statement
The datasets presented in this study can be found in online repositories. The names of the repository/repositories and accession number(s) can be found in the article/Supplementary Material.
Author Contributions
QZ conceptualized and designed this study. Y-YQ, R-YG, and M-LL performed the data collection and analysis. YYQ and M-LL wrote the manuscript. Y-YQ and QZ participated in manuscript revision. All authors contributed to the article and approved the submitted version.
Conflict of Interest
The authors declare that the research was conducted in the absence of any commercial or financial relationships that could be construed as a potential conflict of interest.
Publisher’s Note
All claims expressed in this article are solely those of the authors and do not necessarily represent those of their affiliated organizations, or those of the publisher, the editors, and the reviewers. Any product that may be evaluated in this article, or claim that may be made by its manufacturer, is not guaranteed or endorsed by the publisher.
Supplementary Material
The Supplementary Material for this article can be found online at: https://www.frontiersin.org/articles/10.3389/fgene.2021.757582/full#supplementary-material
References
Baltaci, A. K., and Yuce, K. (2018). Zinc Transporter Proteins. Neurochem. Res. 43 (3), 517–530. doi:10.1007/s11064-017-2454-y
Bin, B. H., Seo, J., and Kim, S. T. (2018). Function, Structure, and Transport Aspects of ZIP and ZnT Zinc Transporters in Immune Cells. J. Immunol. Res. 2018, 9365747. doi:10.1155/2018/9365747
Brito, S., Lee, M. G., Bin, B. H., and Lee, J. S. (2020). Zinc and its Transporters in Epigenetics. Mol. Cell 43 (4), 323–330. doi:10.14348/molcells.2020.0026
Chen, W., Sun, Z., and Lu, L. (2021). Targeted Engineering of Medicinal Chemistry for Cancer Therapy: Recent Advances and Perspectives. Angew. Chem. Int. Ed. 60 (11), 5626–5643. doi:10.1002/anie.201914511
Cheng, X., Wang, J., Liu, C., Jiang, T., Yang, N., Liu, D., et al. (2021). Zinc Transporter SLC39A13/ZIP13 Facilitates the Metastasis of Human Ovarian Cancer Cells via Activating Src/FAK Signaling Pathway. J. Exp. Clin. Cancer Res. 40 (1), 199. doi:10.1186/s13046-021-01999-3
Cheng, X., Wei, L., Huang, X., Zheng, J., Shao, M., Feng, T., et al. (2017). Solute Carrier Family 39 Member 6 Gene Promotes Aggressiveness of Esophageal Carcinoma Cells by Increasing Intracellular Levels of Zinc, Activating Phosphatidylinositol 3-Kinase Signaling, and Up-Regulating Genes that Regulate Metastasis. Gastroenterology 152 (8), 1985–1997. doi:10.1053/j.gastro.2017.02.006
Ding, B., Lou, W., Xu, L., Li, R., and Fan, W. (2019). Analysis the Prognostic Values of Solute Carrier (SLC) Family 39 Genes in Gastric Cancer. Am. J. Transl Res. 11 (1), 486–498.
Fan, Q., Cai, Q., Li, P., Wang, W., Wang, J., Gerry, E., et al. (2017). The Novel ZIP4 Regulation and its Role in Ovarian Cancer. Oncotarget 8 (52), 90090–90107. doi:10.18632/oncotarget.21435
Ferlay, J., Colombet, M., Soerjomataram, I., Parkin, D. M., Piñeros, M., Znaor, A., et al. (2021). Cancer Statistics for the Year 2020: An Overview. Int. J. Cancer. doi:10.1002/ijc.33588
Gao, J., Aksoy, B. A., Dogrusoz, U., Dresdner, G., Gross, B., Sumer, S. O., et al. (2013). Integrative Analysis of Complex Cancer Genomics and Clinical Profiles Using the cBioPortal. Sci. Signal. 6 (269), pl1. doi:10.1126/scisignal.2004088
Geng, X., Liu, L., Banes-Berceli, A., Yang, Z., Kang, P., Shen, J., et al. (2018). Role of ZIP8 in Regulating Cell Morphology and NF-κB/Snail2 Signaling. Metallomics 10 (7), 953–964. doi:10.1039/c8mt00079d
Golovine, K., Makhov, P., Uzzo, R. G., Shaw, T., Kunkle, D., and Kolenko, V. M. (2008). Overexpression of the Zinc Uptake Transporter hZIP1 Inhibits Nuclear Factor-Κb and Reduces the Malignant Potential of Prostate Cancer Cells In Vitro and In Vivo. Clin. Cancer Res. 14 (17), 5376–5384. doi:10.1158/1078-0432.ccr-08-0455
Grattan, B. J., and Freake, H. C. (2012). Zinc and Cancer: Implications for LIV-1 in Breast Cancer. Nutrients 4 (7), 648–675. doi:10.3390/nu4070648
Guo, Y., and He, Y. (2020). Comprehensive Analysis of the Expression of SLC30A Family Genes and Prognosis in Human Gastric Cancer. Sci. Rep. 10 (1), 18352. doi:10.1038/s41598-020-75012-w
Hoang, B. X., Han, B., Shaw, D. G., and Nimni, M. (2016). Zinc as a Possible Preventive and Therapeutic Agent in Pancreatic, Prostate, and Breast Cancer. Eur. J. Cancer Prev. 25 (5), 457–461. doi:10.1097/cej.0000000000000194
Hogstrand, C., Kille, P., Ackland, M. L., Hiscox, S., and Taylor, K. M. (2013). A Mechanism for Epithelial-Mesenchymal Transition and Anoikis Resistance in Breast Cancer Triggered by Zinc Channel ZIP6 and STAT3 (Signal Transducer and Activator of Transcription 3). Biochem. J. 455 (2), 229–237. doi:10.1042/bj20130483
Hojyo, S., and Fukada, T. (2016). Zinc Transporters and Signaling in Physiology and Pathogenesis. Arch. Biochem. Biophys. 611, 43–50. doi:10.1016/j.abb.2016.06.020
Hojyo, S., Miyai, T., Fujishiro, H., Kawamura, M., Yasuda, T., Hijikata, A., et al. (2014). Zinc Transporter SLC39A10/ZIP10 Controls Humoral Immunity by Modulating B-Cell Receptor Signal Strength. Proc. Natl. Acad. Sci. 111 (32), 11786–11791. doi:10.1073/pnas.1323557111
Hu, J. (2020). Toward Unzipping the ZIP Metal Transporters: Structure, Evolution, and Implications on Drug Discovery against Cancer. FEBS J. 288, 5805–5825. doi:10.1111/febs.15658
Huang, D. W., Sherman, B. T., and Lempicki, R. A. (2009). Systematic and Integrative Analysis of Large Gene Lists Using DAVID Bioinformatics Resources. Nat. Protoc. 4 (1), 44–57. doi:10.1038/nprot.2008.211
Jin, J., Li, Z., Liu, J., Wu, Y., Gao, X., and He, Y. (2015). Knockdown of Zinc Transporter ZIP5 (SLC39A5) Expression Significantly Inhibits Human Esophageal Cancer Progression. Oncol. Rep. 34 (3), 1431–1439. doi:10.3892/or.2015.4097
Kagara, N., Tanaka, N., Noguchi, S., and Hirano, T. (2007). Zinc and its Transporter ZIP10 Are Involved in Invasive Behavior of Breast Cancer Cells. Cancer Sci. 98 (5), 692–697. doi:10.1111/j.1349-7006.2007.00446.x
Kambe, T., Hashimoto, A., and Fujimoto, S. (2014). Current Understanding of ZIP and ZnT Zinc Transporters in Human Health and Diseases. Cell. Mol. Life Sci. 71 (17), 3281–3295. doi:10.1007/s00018-014-1617-0
Kimura, T., and Kambe, T. (2016). The Functions of Metallothionein and ZIP and ZnT Transporters: An Overview and Perspective. Ijms 17 (3), 336. doi:10.3390/ijms17030336
Kitamura, H., Morikawa, H., Kamon, H., Iguchi, M., Hojyo, S., Fukada, T., et al. (2006). Toll-like Receptor-Mediated Regulation of Zinc Homeostasis Influences Dendritic Cell Function. Nat. Immunol. 7 (9), 971–977. doi:10.1038/ni1373
Li, M., Zhang, Y., Liu, Z., Bharadwaj, U., Wang, H., Wang, X., et al. (2007). Aberrant Expression of Zinc Transporter ZIP4 (SLC39A4) Significantly Contributes to Human Pancreatic Cancer Pathogenesis and Progression. Proc. Natl. Acad. Sci. 104 (47), 18636–18641. doi:10.1073/pnas.0709307104
Li, Q., and Shu, Y. (2014). Role of Solute Carriers in Response to Anticancer Drugs. Mol. Cell Therapies 2, 15. doi:10.1186/2052-8426-2-15
Li, T., Fan, J., Wang, B., Traugh, N., Chen, Q., Liu, J. S., et al. (2017). TIMER: A Web Server for Comprehensive Analysis of Tumor-Infiltrating Immune Cells. Cancer Res. 77 (21), e108–e110. doi:10.1158/0008-5472.can-17-0307
Li, T., Fu, J., Zeng, Z., Cohen, D., Li, J., Chen, Q., et al. (2020). TIMER2.0 for Analysis of Tumor-Infiltrating Immune Cells. Nucleic Acids Res. 48 (W1), W509–W514. doi:10.1093/nar/gkaa407
Liu, L., Yang, J., and Wang, C. (2020). Analysis of the Prognostic Significance of Solute Carrier (SLC) Family 39 Genes in Breast Cancer. Biosci. Rep. 40 (8). doi:10.1042/BSR20200764
Liu, M.-J., Bao, S., Gálvez-Peralta, M., Pyle, C. J., Rudawsky, A. C., Pavlovicz, R. E., et al. (2013). ZIP8 Regulates Host Defense through Zinc-Mediated Inhibition of NF-Κb. Cell Rep. 3 (2), 386–400. doi:10.1016/j.celrep.2013.01.009
Loomans-Kropp, H. A., and Umar, A. (2019). Cancer Prevention and Screening: the Next Step in the Era of Precision Medicine. npj Precision Onc 3, 3. doi:10.1038/s41698-018-0075-9
Mizuno, H., Kitada, K., Nakai, K., and Sarai, A. (2009). PrognoScan: a New Database for Meta-Analysis of the Prognostic Value of Genes. BMC Med. Genomics 2, 18. doi:10.1186/1755-8794-2-18
Mohammadinejad, R., Sassan, H., Pardakhty, A., Hashemabadi, M., Ashrafizadeh, M., Dehshahri, A., et al. (2020). ZEB1 and ZEB2 Gene Editing Mediated by CRISPR/Cas9 in A549 Cell Line. Bratisl Lek Listy 121 (1), 31–36. doi:10.4149/BLL_2020_005
Nagy, Á., Munkácsy, G., and Győrffy, B. (2021). Pancancer Survival Analysis of Cancer Hallmark Genes. Sci. Rep. 11 (1), 6047. doi:10.1038/s41598-021-84787-5
Pan, Z., Choi, S., Ouadid-Ahidouch, H., Yang, J. M., Beattie, J. H., and Korichneva, I. (2017). Zinc Transporters and Dysregulated Channels in Cancers. Front. Biosci. 22, 623–643. doi:10.2741/4507
Prasad, A. S. (2012). Discovery of Human Zinc Deficiency: 50 Years Later. J. Trace Elem. Med. Biol. 26 (2-3), 66–69. doi:10.1016/j.jtemb.2012.04.004
Rhodes, D. R., Kalyana-Sundaram, S., Mahavisno, V., Varambally, R., Yu, J., Briggs, B. B., et al. (2007). Oncomine 3.0: Genes, Pathways, and Networks in a Collection of 18,000 Cancer Gene Expression Profiles. Neoplasia 9 (2), 166–180. doi:10.1593/neo.07112
Shankavaram, U. T., Varma, S., Kane, D., Sunshine, M., Chary, K. K., Reinhold, W. C., et al. (2009). CellMiner: a Relational Database and Query Tool for the NCI-60 Cancer Cell Lines. BMC genomics 10, 277. doi:10.1186/1471-2164-10-277
Sun, J., Liu, J., Pan, X., Quimby, D., Zanesi, N., Druck, T., et al. (2011). Effect of Zinc Supplementation on N-Nitrosomethylbenzylamine-Induced Forestomach Tumor Development and Progression in Tumor Suppressor-Deficient Mouse Strains. Carcinogenesis 32 (3), 351–358. doi:10.1093/carcin/bgq251
Sung, H., Ferlay, J., Siegel, R. L., Laversanne, M., Soerjomataram, I., Jemal, A., et al. (2021). Global Cancer Statistics 2020: GLOBOCAN Estimates of Incidence and Mortality Worldwide for 36 Cancers in 185 Countries. CA A. Cancer J. Clin. 71 (3), 209–249. doi:10.3322/caac.21660
Szklarczyk, D., Gable, A. L., Lyon, D., Junge, A., Wyder, S., Huerta-Cepas, J., et al. (2019). STRING V11: Protein-Protein Association Networks with Increased Coverage, Supporting Functional Discovery in Genome-wide Experimental Datasets. Nucleic Acids Res. 47 (D1), D607–D613. doi:10.1093/nar/gky1131
Tang, Z., Li, C., Kang, B., Gao, G., Li, C., and Zhang, Z. (2017). GEPIA: a Web Server for Cancer and normal Gene Expression Profiling and Interactive Analyses. Nucleic Acids Res. 45 (W1), W98–W102. doi:10.1093/nar/gkx247
To, P. K., Do, M. H., Cho, J. H., and Jung, C. (2020). Growth Modulatory Role of Zinc in Prostate Cancer and Application to Cancer Therapeutics. Int. J. Mol. Sci. 21 (8). doi:10.3390/ijms21082991
Warde-Farley, D., Donaldson, S. L., Comes, O., Zuberi, K., Badrawi, R., Chao, P., et al. (2010). The GeneMANIA Prediction Server: Biological Network Integration for Gene Prioritization and Predicting Gene Function. Nucleic Acids Res. 38, W214–W220. doi:10.1093/nar/gkq537
Xiao, Q., Zhang, F., Xu, L., Yue, L., Kon, O. L., Zhu, Y., et al. (2021). High-throughput Proteomics and AI for Cancer Biomarker Discovery. Adv. Drug Deliv. Rev. 176, 113844. doi:10.1016/j.addr.2021.113844
Zhang, Y., Tian, Y., Zhang, H., Xu, B., and Chen, H. (2021). Potential Pathways of Zinc Deficiency-Promoted Tumorigenesis. Biomed. Pharmacother. 133, 110983. doi:10.1016/j.biopha.2020.110983
Zhou, H., Zhu, Y., Qi, H., Liang, L., Wu, H., Yuan, J., et al. (2021). Evaluation of the Prognostic Values of Solute Carrier (SLC) Family 39 Genes for Patients with Lung Adenocarcinoma. Aging 13 (4), 5312–5331. doi:10.18632/aging.202452
Keywords: SLC39 family genes, biomarker, pan-cancer, prognosis, immune infiltration, drug sensitivity
Citation: Qu Y-Y, Guo R-Y, Luo M-L and Zhou Q (2021) Pan-Cancer Analysis of the Solute Carrier Family 39 Genes in Relation to Oncogenic, Immune Infiltrating, and Therapeutic Targets. Front. Genet. 12:757582. doi: 10.3389/fgene.2021.757582
Received: 12 August 2021; Accepted: 12 November 2021;
Published: 02 December 2021.
Edited by:
Liang Zhao, University of São Paulo Ribeirão Preto, BrazilReviewed by:
Luca Zammataro, Kiromic Biopharma Inc., Houston, United StatesShailender Kumar Verma, Central University of Himachal Pradesh, India
Copyright © 2021 Qu, Guo, Luo and Zhou. This is an open-access article distributed under the terms of the Creative Commons Attribution License (CC BY). The use, distribution or reproduction in other forums is permitted, provided the original author(s) and the copyright owner(s) are credited and that the original publication in this journal is cited, in accordance with accepted academic practice. No use, distribution or reproduction is permitted which does not comply with these terms.
*Correspondence: Quan Zhou, zhouquan8519@163.com