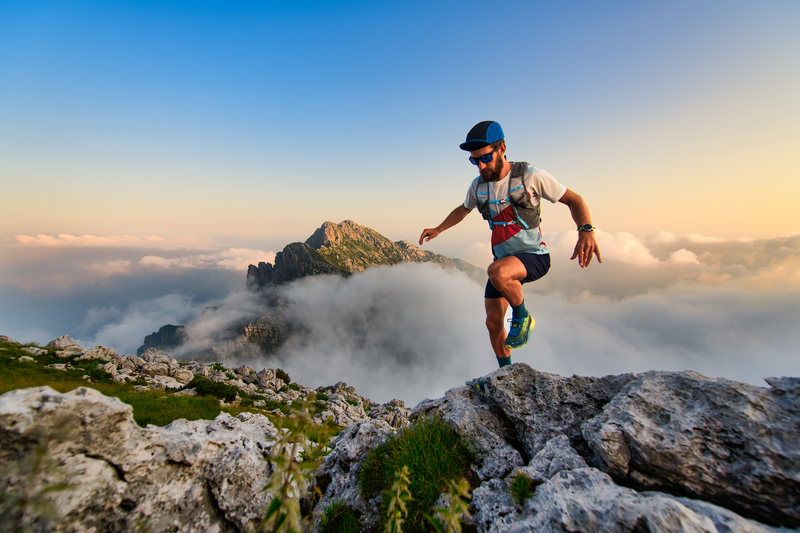
95% of researchers rate our articles as excellent or good
Learn more about the work of our research integrity team to safeguard the quality of each article we publish.
Find out more
ORIGINAL RESEARCH article
Front. Genet. , 26 November 2021
Sec. Livestock Genomics
Volume 12 - 2021 | https://doi.org/10.3389/fgene.2021.752424
This article is part of the Research Topic Multi-Layered Genome-Wide Association/Prediction in Animals View all 10 articles
The importance of meat and carcass quality is growing in beef cattle production to meet both producer and consumer demands. Primal cut yields, which reflect the body compositions of carcass, could determine the carcass grade and, consequently, command premium prices. Despite its importance, there have been few genome-wide association studies on these traits. This study aimed to identify genomic regions and putative candidate genes related to 10 primal cut traits, including tenderloin, sirloin, striploin, chuck, brisket, top round, bottom round, shank, flank, and rib in Hanwoo cattle using a single-step Bayesian regression (ssBR) approach. After genomic data quality control, 43,987 SNPs from 3,745 genotyped animals were available, of which 3,467 had phenotypic records for the analyzed traits. A total of 16 significant genomic regions (1-Mb window) were identified, of which five large-effect quantitative trait loci (QTLs) located on chromosomes 6 at 38–39 Mb, 11 at 21–22 Mb, 14 at 6–7 Mb and 26–27 Mb, and 19 at 26–27 Mb were associated with more than one trait, while the remaining 11 QTLs were trait-specific. These significant regions were harbored by 154 genes, among which TOX, FAM184B, SPP1, IBSP, PKD2, SDCBP, PIGY, LCORL, NCAPG, and ABCG2 were noteworthy. Enrichment analysis revealed biological processes and functional terms involved in growth and lipid metabolism, such as growth (GO:0040007), muscle structure development (GO:0061061), skeletal system development (GO:0001501), animal organ development (GO:0048513), lipid metabolic process (GO:0006629), response to lipid (GO:0033993), metabolic pathways (bta01100), focal adhesion (bta04510), ECM–receptor interaction (bta04512), fat digestion and absorption (bta04975), and Rap1 signaling pathway (bta04015) being the most significant for the carcass primal cut traits. Thus, identification of quantitative trait loci regions and plausible candidate genes will aid in a better understanding of the genetic and biological mechanisms regulating carcass primal cut yields.
Hanwoo is an indigenous and popular meat-type cattle in Korea, and is particularly renowned for its rapid growth rate and quality attributes such as juiciness, tenderness, characteristic flavor, and extensive marbling of its beef (Jo et al., 2012). In recent years, both carcass and meat quality traits in Hanwoo have been extensively studied because of their economic relevance for optimizing the profitability of the beef industry. The current selection index in Hanwoo focuses on the improvement of carcass traits, such as backfat thickness (BFT), carcass weight (CW), eye muscle area (EMA), and marbling score (MS) as major selection criteria for breeding programs (Kim et al., 2017). However, other important traits such as carcass primal cuts have received inadequate attention in the Hanwoo breeding program, which affects both the quantity and quality of meat, and consequently, command premium prices. To meet consumer demand, the importance of primal cut yields is growing in the beef industry of developed countries because of its market value. Hence, cattle breeders need to address these traits, which determine selection decisions to increase carcass cut-out value and consumer acceptance of meat. Meanwhile, the existence of genetic variation and moderate to high heritability in the yield of primal cuts has been reported (Choi et al., 2015). In this sense, the improvement of primal cut yields requires knowledge of the underlying genetic background influencing these invaluable traits.
Over the last decade, with the development of high-throughput single nucleotide polymorphism (SNP) genotyping technologies, genome-wide association studies (GWAS) have become an affordable and powerful tool for detecting and localizing candidate genes and causal mutations associated with quantitative traits in different species (Matukumalli et al., 2009). Several statistical methods to conduct GWAS have been developed and applied, among which a simple regression model has been widely used, where one marker is tested at a time for significance (Meyer and Tier, 2012). However, this method was challenged by false positives and overestimation of quantitative trait loci (QTL) effects. Therefore, the marker effect models in the Bayesian approaches have been proposed for GWAS analysis (Habier et al., 2011; Wang et al., 2012; Moser et al., 2015; Wang et al., 2016) as they offer methods to overcome these challenges (Strömberg, 2009; Peters et al., 2012). One such method could have a higher power to detect SNPs with moderate effects on a trait of interest. In addition, Bayesian methods are flexible in accounting for the uncertainties of variables and parameters and allow for inferences to be made by finding their marginal posterior distributions (Yi and Shriner, 2008; Blasco and Blasco, 2017). Recently, Fernando et al. (2014; 2016) developed a class of single-step Bayesian regression methods (ssBR), which not only combines all available information as single-step genomic best linear unbiased prediction [ssGBLUP; (Misztal et al., 2009)] does, but also accommodates Bayesian models. This method can also be extended to GWAS and controls the proportion of false positives by computing the posterior probability of association of a trait with each SNP or each window of consecutive SNPs. Numerous association studies have been carried out on growth and carcass traits in beef cattle using different GWAS approaches (Peters et al., 2012; Lee et al., 2013; Magalhaes et al., 2016; Weng et al., 2016; Roberts, 2018; Bedhane et al., 2019; Naserkheil et al., 2020). However, GWAS have not yet been conducted to identify significant genomic regions for carcass primal cut traits, which are highly relevant to Hanwoo cattle breeding. Hence, the objective of this study was to perform GWAS to detect genomic regions and candidate genes associated with primal cut yields in Hanwoo cattle using the ssBR approach.
A total of 3,467 Hanwoo steers born between 2008 and 2017 were included in this study. All steers were slaughtered at approximately 24 months of age and were progeny of 442 sires and 3,357 dams. The pedigree data consisted of 15,117 animals after tracing the pedigree file back 10 generations and pruning with SECATEURS software (Meyer, 2003). During the pruning process, 3,692 individuals were removed from the pedigree. All phenotypic data were collected by the Hanwoo Improvement Center (HIC) of the National Agricultural Cooperative Federation, South Korea. The ten primal cut yields considered in the present study (kg, composed of both unique and composite meat cuts from the forequarters and hindquarters) included tenderloin, sirloin, striploin, chuck, brisket, top round, bottom round, shank, flank, and rib; the locations of each cut on the carcass are illustrated in Supplementary Figure S1. The descriptive statistics and heritability estimates of the primal cut traits are shown in Table 1.
TABLE 1. Descriptive statistics and heritability estimates for the primal cut traits in Hanwoo cattle.
In total, 12,764 animals were genotyped initially using three different SNP platforms, Illumina BovineSNP50K version 2 (n = 3,720), version 3 (n = 4,121), and customized Hanwoo version 1 (n = 4,923). Individuals (and SNPs) with a call rate of less than 90% and those without a valid phenotype were also excluded. The genotyped animals with Illumina BovineSNP50K version 2 were used as a reference populations to impute target animals (The genotyped animals using Illumina BovineSNP50K version 3 and customized Hanwoo version 1) using FImpute V3 software (Sargolzaei et al., 2014), and 52,791 SNPs on the 29 chromosomes were finally obtained. The analyses included genotypes for 2,957 steers with phenotypes and 788 their paternal ancestors. Quality control procedures were conducted using the BLUPF90 software (Misztal et al., 2015). SNPs with minor allele frequency less than 0.01 (8,783 SNPs), and a maximum difference between the observed and expected frequency of 0.15 as a departure of heterozygous from the Hardy-Weinberg equilibrium (21 SNPs) were discarded. After quality control, 3,745 animals with the genotypes on 43,987 SNPs were remained for subsequent analyses.
The single-step Bayesian regression (ssBR) method proposed by Fernando et al. (2014; 2016) was utilized to perform GWAS analyses, which combined all available phenotypes, genotypes, and pedigree information in a single-step. The estimation of genetic and residual variances as well as GWAS analyses were performed using univariate single-step Bayes B, with π being 0.99. The model for the single-step Bayesian GWAS (Fernando et al., 2014; Cheng et al., 2015; Fernando et al., 2016; Fernando et al., 2017) was as follows for genotyped animals:
and non-genotyped animals:
where yi is a phenotype for individual i; βj is the jth effects including slaughter date (180 levels) and slaughter age (days from birth to slaughter) was considered as covariates; Xij is the incidence covariate corresponding to the βj for individual i; Zik is the incidence covariate corresponding to the kth random animal effect for individual i; u = [u1, u2, …,
The effects of β are assigned to the flat priors. In addition, the additive genetic (σu2), residual (σe2), and marker (σα2) variances were assumed to have a scaled inverted chi-square prior with scale parameters
The analysis was performed using the JWAS Julia package for whole-genome analyses (Cheng et al., 2018) to obtain the posterior distributions of SNP effects using Markov chain Monte Carlo (MCMC). This method with 110,000 iterations was implemented to provide the posterior mean effects of the SNPs within each 1-Mb window and variance components after discarding the first 10,000 samples for burn-in and a thinning interval of 10. In total, 2,522 windows (1-Mb) across the 29 autosomes were included in the analyses. The window posterior probabilities of association (WPPA) for each window were also calculated.
The markers effect in each MCMC was estimated by using single-step Bayes B in addition to the polygenic additive genetic variance (σ2a) and residual variance (σ2e). The direct genomic value (DGV) that is attributed to markers is estimated as:
The genomic variance (σ2m) was estimated using Gibbs sampling technique described by Sorensen et al. (2001). Then total genetic variance was estimated by summation of σ2m and σ2u. In addition, the phenotypic variance was estimated by summation total genetic and environment variances. The heritability was obtained using total genetic variance divided by phenotypic variance.
The percentage of genomic variance (GV%) explained by each 1-Mb window in any particular iteration was calculated by dividing the genomic variance of the window by the genomic variance of the whole genome in the same iteration. Similarity, the proportion of additive genetic variance (AGV%) determined using each window markers were also obtained.
Genome windows with WPPA ≥0.8 (Wang et al., 2020) were considered as possible QTL regions associated with the studied traits. Candidate genes were searched for 1-Mb window using the Ensembl database and the Map Viewer tool of the bovine genome based on the starting and ending coordinates of significant windows. Further information on the function of these genes was obtained from the National Center for Biotechnology Information (NCBI) (http://www.ncbi.nlm.nih.gov/gene/), and GeneCards (www.genecards.org). A Manhattan plot was created using the ggplot2 package (Wickham, 2009) in R software. To understand and identify the biological processes and pathways, gene ontology (GO) and Kyoto Encyclopedia of Genes and Genomes (KEGG) enrichment were carried out using the Database for Annotation, Visualization, and Integrated Discovery (DAVID) and web tool g:Profiler (Raudvere et al., 2019). Only GO terms with a significant p value of 0.05 and genes involved in biological processes, molecular functions, and cellular components were highlighted.
The number of records, means, minimum, maximum, standard deviations, phenotypic coefficient of variation, and heritability estimates for the 10 primal cut traits are provided in Table 1. The mean values of these traits ranged from 6.04 to 57.55 with standard deviation between 0.76 and 7.53. The coefficients of variation ranged from 11.89 to 25.72%, indicating considerable phenotypic variation of the investigated traits in the Hanwoo cattle population. Moderate to high heritability estimates were obtained for primal cut yields, which ranged from 0.32 ± 0.03 to 0.61 ± 0.04. Estimated variance components are also summarized in Supplementary Table S1.
The identification of genomic regions related to the traits of interest was performed using a ssBR approach. Only windows with WPPA ≥0.8, were considered significant. Manhattan plots displayed the proportion of genomic variance (Figure 1), additive genetic variance (Supplementary Figure S2) explained and the posterior probability of association by each 1-Mb window for the studied traits (Figure 2). Positional candidate genes for primal cut traits were also detected within the significant windows. A summary of significant windows associated with the traits under study, such as the number of SNPs in each window, explained GV% or AGV%, and WPPA, as well as candidate genes, is shown in Table 2. A total of 16 relevant genomic regions (1-Mb window) were found to be associated with the 10 traits recorded in this study. These regions were distributed over nine different chromosomes: 3, 4, 6, 10, 11, 14, 16, 19, and 25. Of these significant windows, five genomic windows were pleiotropic QTL, meaning that the QTL had an effect on multiple traits, which were located on chromosomes 6 at 38–39, 11 at 21–22, 14 at 6–7 Mb and 26–27, and 19 at 26–27 Mb. The genomic window located on chromosome 14 at 26–27 Mb explained a large proportion of the genomic variance across the nine analyzed traits, including tenderloin, sirloin, striploin, chuck, brisket, top round, bottom round, shank, and flank. The largest QTL window was observed for shank, which explained 20.51% of the genomic variance, was located in the region of 26–27 Mb on chromosome 14. The QTL window with the smallest proportion of genomic variance (0.87%) was identified for bottom round, located on chromosome 14 at 6–7 Mb. Candidate genes responsible for the genomic variance explained by the 1-Mb window were identified using the Bos taurus genome map. A total of 154 genes were identified within the significant regions to be associated with the traits of interest. Of these, 92 genes were codified proteins, 3 were miRNA (microRNA), 6 were tRNA (RNA transporter), and 53 were pseudogenes (Table 2). Functional enrichment analysis revealed 18 biological processes, six molecular functions, seven cellular components, and seven KEGG pathways. The following biological process terms were highlighted: growth (GO:0040007), positive regulation of growth (GO:0045927), tissue development (GO:0009888), muscle structure development (GO:0061061), skeletal system development (GO:0001501), animal organ development (GO:0048513), cellular response to chemical stimulus (GO:0070887), lipid metabolic process (GO:0006629), response to lipid (GO:0033993), glycolipid biosynthetic process (GO:0009247), and cell cycle (GO:0007049) being the most significant for the traits under study. In the case of the molecular function, calcium channel activity (GO:0005262), protein binding (GO:0005515), ligase activity (GO:0016874), lipid binding (GO:0008289), catalytic activity (GO:0003824), and nucleotide binding (GO:0000166) were significant terms. The significant enrichments for cellular component, including endoplasmic reticulum membrane (GO:0005789), intracellular (GO:0005622), endoplasmic reticulum (GO:0005783), actin cytoskeleton (GO:0015629), extracellular region (GO:0005576), organelle (GO:0043226), and cytoplasmic region (GO:0099568) were obtained. Moreover, KEGG pathway analysis revealed that the identified candidate genes involved in primal cut yields were enriched in metabolic pathways (bta01100), focal adhesion (bta04510), ECM-receptor interaction (bta04512), glycolysis/gluconeogenesis (bta00010), fat digestion and absorption (bta04975), Rap1 signaling pathway (bta04015), and calcium signaling pathway (bta04020). Functional gene set annotation and enrichment pathways are presented in Table 3.
FIGURE 1. Manhattan plots of the percentage of genomic variance explained by 1-Mb windows for primal cut traits in Hanwoo cattle.
FIGURE 2. Plots of window posterior probabilities of association (WPPA) obtained by the single-step Bayesian regression method for primal cut traits in Hanwoo cattle. The dash line is threshold 0.8 for significantly of windows.
TABLE 2. Gene identification and proportion of variance explained by 1-Mb windows associated with the primal cut traits in Hanwoo cattle.
TABLE 3. Gene Ontology (GO) terms and KEGG pathways significantly enriched using candidate genes associated with the primal cut traits.
The aim of this study was to identify genomic regions associated with primal cut yields using a ssBR approach in Hanwoo cattle. The marker effect model in a Bayesian framework would seem to be useful for GWAS because they account for uncertainty in parameters required to construct posterior distributions for QTL inference, thereby improving accuracy of genomic predictions and the power of QTL detection (Fernando and Garrick, 2013; Mehrban et al., 2017). In recent years, interest in exploring genomic regions that control economically important traits in beef cattle has increased due to advances in high-throughput genotyping techniques and the constant availability of molecular data, statistical methods, and ease of application of GWAS. Primal cut traits have recently been proposed as potential indicator of carcass weight and overall carcass merit (Berry et al., 2019) given that the genetic correlations between these traits and carcass weight are generally moderate to strong (Choi et al., 2015; Judge et al., 2019). Nevertheless, selection for the weight of primal cuts requires knowledge of the genetic basis for these traits, which may be useful in future genomic evaluations targeting the improvement of weight in the more valuable primal cuts, and consequently increasing the profitability of the meat production system. Our results showed that primal cut yields were moderate to highly heritable, being in accordance with those reported in Hanwoo cattle (Choi et al., 2015), Chianina cattle (Sarti et al., 2013), Simmental cattle (Zhu et al., 2019), and Irish cattle (Berry et al., 2019; Judge et al., 2019). Among all identified window regions, the QTL on chromosome 14 at position 26–27 Mb had a larger impact than any of the other QTLs and was associated with a greater number of traits. This region, which is related to tenderloin, sirloin, striploin, chuck, brisket, top round, bottom round, shank, and flank, explained between 4.26 and 20.51% of the genomic variance across all these traits. A total of 11 genes were detected on chromosome 14 at 26–27 Mb regions. Among these, FAM110B, UBXN2B, NSMAF, TOX, SDCBP, and CYP7A1 were notable. This region also seems to be most significantly associated with carcass and growth traits in beef cattle (Magalhaes et al., 2016; Roberts, 2018; Zhang et al., 2019; Grigoletto et al., 2020). Positional candidate genes of FAM110B, UBXN2B, NSMAF, CYP7A1, SDCBP, and TOX have been previously reported to be associated with carcass weight in Hanwoo cattle (Lee et al., 2013; Bhuiyan et al., 2018; Naserkheil et al., 2020). For instance, Lee et al. (2013) identified the six most significant SNPs associated with carcass weight in Hanwoo that were located in or nearby TOX, FAM110B, and SDCBP. Similarly, Srikanth et al. (2020) reported that the most significant SNPs on chromosome 14 were located in UBXN2B, CYP7A1, SDCBP, and TOX, which have been regarded as positional candidate genes for carcass weight in Hanwoo cattle. It was also reported that CYP7A1 and SDCBP are positional candidate genes for carcass weight and eye muscle area in Hanwoo cattle (Bhuiyan et al., 2018; Srivastava et al., 2020), weaning weight in Brangus cattle (Weng et al., 2016), and feed efficiency traits in Nellore cattle (Brunes et al., 2021). The TOX gene located within this region is associated with reproductive traits in Nellore (de Camargo et al., 2015), residual feed intake and mid-test metabolic weight in SimAngus (Seabury et al., 2017), and development of puberty in Brahman cattle (Fortes et al., 2012). In addition, the UBXN2B gene was found to be associated with mid-test metabolic weight in SimAngus (Seabury et al., 2017) and carcass weight, carcass fat, and carcass conformation in Simmental cattle (Purfield et al., 2019), which is known as a protein-coding gene involved in endoplasmic reticulum biogenesis. FAM110B has been reported to be associated with fat thickness in composite beef cattle (Roberts, 2018). This gene functions in the cell cycle and cell growth and might play an important role in increasing carcass weight in beef cattle by increasing cell number and size. A pleiotropic QTL on chromosome 6 at 38–39 Mb was associated with tenderloin, striploin, brisket, top round, bottom round, and shank, which explained the largest (6.46%) and smallest (2.7%) proportion of genomic variance for bottom round and tenderloin, respectively. This region harbors relevant candidate genes, including PKD2, SPP1, MEPE, IBSP, LAP3, NCAPG, and LCORL. Most of the positional genes detected on chromosome 6 have previously been associated with many economically important traits in beef and dairy cattle. In a study on Brangus beef cattle, Weng et al. (2016) reported that most positional genes associated with direct birth weight, weaning weight, and yearling weight are located on chromosome 6. Similarly, Saatchi et al. (2014) identified a large-effect pleiotropic QTL located on chromosome 6 at 37–42 Mb was associated with direct birth weight, calving ease, carcass weight, rib eye muscle area, and weaning weight across several cattle breeds. In addition, it has previously been reported that a large number of significant SNPs associated with skeleton trait in Simmental cattle that were harbored by LAP3, FAM184B, LCORL, and NCAPG genes (Xia et al., 2017). A major QTL on chromosome 6, extending from 36 to 39 Mb related to carcass weight, was also identified in Japanese Black cattle (Nishimura et al., 2012). Interestingly, the NCAPG and LCORL genes, while being associated with the skeletal type traits (Hoshiba et al., 2013; Doyle et al., 2020) have also been regarded as positional candidate genes for direct calving ease, feed intake, gain, meat, and carcass traits (Lindholm-Perry et al., 2011; Bongiorni et al., 2012; Purfield et al., 2019), as well as growth and lipid deposition (Snelling et al., 2010; Weikard et al., 2010) across several breeds. Moreover, Liu et al. (2015) identified LCORL as a positional gene related to weight and carcass composition traits in chickens based on GWAS and differentially expressed gene studies. Other notable positional candidate genes in this region including PKD2, LAP3, SPP1, MEPE, IBSP and MED28, have been associated with carcass weight and growth traits (Lindholm-Perry et al., 2011; Weng et al., 2016; Roberts, 2018; Naserkheil et al., 2020), milk production (Olsen et al., 2005), and reproductive traits and puberty (Daetwyler et al., 2008; Cánovas et al., 2014) in beef and dairy cattle.
The other large-effect pleiotropic QTL identified in multiple traits (tenderloin, sirloin, brisket, top round, bottom round, shank, and flank) was located on chromosome 19 at position 26–27 Mb. This region explained between 1 and 2.07% of the genomic variance across the traits of interest, and harbors candidate genes, including PITPNM3, WSCD1, MIS12, and RABEP1. A recent study on linear type traits conducted by Doyle et al. (2020b) identified PITPNM3 as a potential gene for chest depth in Angus cattle. The WSCD1 gene encodes a protein with sulfotransferase activity involved in glucose metabolism, and is associated with udder depth in dairy cattle (Li et al., 2021), feed efficiency and feeding behaviors in pigs (Guo et al., 2015), and body size in beef cattle (An et al., 2020). The MIS12 and RABEP1 genes have also been recently linked to milk production traits in dairy cattle (Cai et al., 2020; Li et al., 2021). Two other pleiotropic genomic windows were located on chromosome 11 at 21–22 Mb, and on chromosome 14 at position 6–7 Mb, which the proportion of genomic variance explained by these windows ranged from 1.13 to 1.69 and from 0.87 to 1.9, respectively. The region on chromosome 14 that was found to be associated with sirloin, brisket, bottom round, and shank had no positional candidate genes in cattle, whereas its orthologous region on human chromosome 8 containing KHDRBS3 gene that may play a role as a negative regulator of cell growth and inhibition of cell proliferation (Ma et al., 2017). For QTL region on chromosome 11, a total of 15 genes annotated were found to be related to brisket, top round, bottom round, and shank. Overall, 11 chromosomal regions identified in this study were trait-specific QTLs for six traits. Three trait-specific QTLs were identified for tenderloin, which are distributed on chromosomes 4 at 77–78 Mb, 6 at 37–38 Mb, and 10 at 32–33 Mb. The trait-specific QTL on chromosome 6 was responsible for 2.74% of the genomic variance in tenderloin and harbors the positional candidate genes PPM1K, ABCG2, and PIGY. The PPM1K gene is involved in cellular survival, phosphorus metabolic process, amino acid dephosphorylation, and development by regulating mitochondrial permeability transition pore function, which have been shown to be associated with increased carcass weight, mid-point metabolic weight, reduction of residual feed intake, feed efficiency conversion ratio, and marbling score in crossbred beef cattle (McClure et al., 2010). The ABCG2 gene is related to body weight (Weng et al., 2016) and milk yield and composition traits (Cohen-Zinder et al., 2005), which is involved in iron transport and metabolism. PIGY is a member of the PIG gene family, which encodes the glycosylphosphatidylinositol-N-acetylglucosaminyltransferase (GPI-GnT) complex and plays an important role in cell–cell interactions. A previous study reported that there were significant effects of copy number variation of the PIGY gene on growth traits in three Chinese sheep breeds (Feng et al., 2020). Brisket also had three trait-specific QTLs located on chromosomes 3 at 98–99, 10 at 49–50, and 25 at 32–33 Mb and explained 1.27, 0.92, and 0.92% of the genomic variance for this trait, respectively. The top round had two trait-specific QTLs. One was located on chromosome 4 at 6–7 Mb (GV% = 1.46), and the other on chromosome 25 at 4–5 Mb (GV% = 0.93). A QTL for the bottom round was identified on chromosome 16 at 25–26 Mb, which accounted for 1.13% of the genomic variance and harbors the positional candidate gene DUSP10. Previous studies have shown that the DUSP10 gene is associated with growth traits (Ribeiro et al., 2021) and carcass weight (Chang et al., 2018) in beef cattle. The QTL located on chromosome 6 at position 39–40 Mb was associated with shank and had a greater proportion of genomic variance (4.89%) than other trait-specific QTLs. Only a QTL on chromosome 4 at 8–9 Mb, which was responsible for 3.23% of the genomic variance for the rib, was identified. The CDK14 gene is located in this region and is associated with fatty acid profile (C18:1 trans-9) in the intramuscular fat of Longissimus thoracis muscle of Nellore cattle (Lemos et al., 2016). According to these results, most primal cut traits are probably controlled by several QTLs with large effects. Among these, genomic regions located on chromosomes 6 and 14 were considered as hot spots for several causal variants related to many economically important traits in beef cattle. A similar conclusion was given by Mehrban et al. (2021), who identified major QTLs on chromosomes 6 and 14 for EMA, yearling weight, and particularly for CW using the weighted single-step GWAS in Hanwoo cattle.
Gene ontology and pathway enrichment analyses were carried out to gain insight into the genes identified within QTL windows using g:Profiler and DAVID functional classification clustering tools (Table 3). Our analyses revealed the significant GO terms classified into the biological processes, cellular components, molecular functions, and seven KEGG pathways were enriched for the studied traits. It is interesting to note that the majority of the common genes identified for the primal cut traits are involved in growth-related processes: growth, positive regulation of growth, muscle structure development, regulation of cell development, muscle organ development, tissue development, skeletal system development, biomineral tissue development, and animal organ development. Among them, six genes, namely HLX, SOS1, SDCBP, ANXA2, FZD1, and MEPE, were highlighted as the main candidates for the traits under study in at least three biological pathways. The HLX gene, located on chromosome 16 at approximately 25–26 Mb, is a protein-coding gene that is involved in embryogenesis and hematopoiesis. It has also been shown to be associated with intramuscular fat in composite beef cattle (Roberts, 2018). The SDCBP gene is located on a conserved region on chromosome 14. It encodes a protein that binds to a variety of transmembrane proteins and plays a crucial role in carcass and meat quality traits. ANXA2 gene is known to encode a member of a widely distributed, phospholipid-binding, calcium-regulated, peripheral membrane protein family known as annexins. This gene is involved in molecular functions related to calcium channel activity and lipid binding. It is not surprising then, that the role of calcium in meat tenderness and muscle contraction, and is a key regulator of muscle growth in beef cattle (Sadkowski et al., 2009). ANXA2 knockout affects white adipose tissue hypotrophy due to reduced fatty acid uptake in mice (Salameh et al., 2016). Furthermore, this gene is associated with feed conversion efficiency in beef cattle (Al-Husseini et al., 2014). The GO terms related to the lipid metabolic process, cellular responses to the chemical stimulus, cell cycle, localization, and regulation of fat cell differentiation were also significantly represented in the pleiotropic QTL windows (Table 3). Pathway enrichment revealed that eight genes from six window regions (located on chromosomes 4, 6, 11, 14, and 25) were significantly associated with the metabolic pathway (bta01100). Among the genes harbored in this pathway, CYP7A1 is involved in the transport, synthesis, and secretion of cholesterol, steroids, and other lipids (Zhao et al., 2013), which play a crucial role in digestion and absorption of dietary fat and contribute in maintaining the balance of cholesterol and lipid metabolism within the body (Monte et al., 2009). Interestingly, the extracellular matrix (ECM)-receptor interaction (bta04512) and focal adhesion (bta04510) pathways were enriched in the genes SPP1 and IBSP from a pleiotropic QTL located on chromosome 6 at 38–39 Mb, which functions as a positive regulator in skeletal muscle cells and is involved in bone mineralization processes. The ECM-receptor interaction pathway plays a key role in tissue, organ morphogenesis, cell maintenance, and tissue structure and function (Mariman and Wang, 2010). It was previously reported that the ECM-receptor is upregulated in subcutaneous fat and intramuscular fat and appears to be involved in adipogenesis and meat tenderness (Taye et al., 2018). Focal adhesion also participates in important biological processes and serves as a mechanical link to ECM receptors and other molecules. These results will improve our understanding of enriched molecular processes, pathways, and genes associated with the primal cut traits and shed some light on how different pathways control these traits.
In the current study, 16 genomic regions (SNP windows) were found to be associated with 10 primal cut traits in Hanwoo cattle using a single-step Bayesian regression GWAS. Within these windows, five QTLs had pleiotropic effects, with the most significant region located on chromosome 14 at position 26–27 Mb. Several candidate genes with potential functions in tissue development, regulation of growth, and lipid metabolism for the related traits were highlighted, among which SPP1, IBSP, PKD2, SDCBP, PIGY, CYP7A1, and MEPE were well-known. Moreover, our findings can contribute to a better understanding of the genetic basis and biological processes underlying the traits of interest; consequently, information on QTL regions can be used to search for causal mutations and marker-assisted or genomic selection in Hanwoo breeding schemes.
The datasets presented in this study can be found in online repositories. The names of the repository/repositories and accession number(s) can be found below: [(https://www.hknu.ac.kr/dhlee/2797/subview), (https://www.aiak.or.kr) and (https://www.mtrace.go.kr)].
The animal study was reviewed and approved by National Institute of Animal Science (NIAS), Rural Development Administration of South Korea.
HM and DL conceived and designed the study and contributed to the discussion of the results. MP provided genotype and phenotypic data for analysis and interpreted the results. MN and HM conceived the study and analyzed the data. MN drafted the manuscript. All authors have read and approved the final manuscript.
This study was supported by the Cooperative Research Program for Agriculture Science and Technology Development (Project No. PJ015987) from the Rural Development Administration (RDA), Republic of Korea. This study was supported by the 2021 RDA Research Associate Fellowship Program of the National Institute of Animal Science, Rural Development Administration, Republic of Korea.
The authors declare that the research was conducted in the absence of any commercial or financial relationships that could be construed as a potential conflict of interest.
All claims expressed in this article are solely those of the authors and do not necessarily represent those of their affiliated organizations, or those of the publisher, the editors and the reviewers. Any product that may be evaluated in this article, or claim that may be made by its manufacturer, is not guaranteed or endorsed by the publisher.
We are grateful to the staff of the Korean Hanwoo Improvement Center of the National Agricultural Cooperative Federation for supplying data.
The Supplementary Material for this article can be found online at: https://www.frontiersin.org/articles/10.3389/fgene.2021.752424/full#supplementary-material
Supplementary Figure S1 | Location of 10 carcass primal cut yields in Hanwoo cattle.
Supplementary Figure S2 | Manhattan plots of the percentage of additive genetic variance explained by 1-Mb windows for primal cut traits in Hanwoo cattle.
HIC, hanwoo improvement center; GWAS, genome-wide association study; ssGBLUP, single-step genomic best linear unbiased prediction; ssBR, single-step Bayesian regression; QTL, quantitative trait loci; SNP, single nucleotide polymorphism; BFT, backfat thickness; CW, carcass weight; EMA, eye muscle area; MS, marbling score; GO, gene ontology; KEGG, kyoto encyclopedia of genes and genomes; MCMC, markov chain monte carlo; GV, genomic variance; AGV, additive genetic variance; WPPA, window posterior probability of association.
Al-Husseini, W., Gondro, C., Quinn, K., Herd, R. M., Gibson, J. P., and Chen, Y. (2014). Expression of Candidate Genes for Residual Feed Intake in Angus Cattle. Anim. Genet. 45 (1), 12–19. doi:10.1111/age.12092
An, B., Xu, L., Xia, J., Wang, X., Miao, J., Chang, T., et al. (2020). Multiple Association Analysis of Loci and Candidate Genes that Regulate Body Size at Three Growth Stages in Simmental Beef Cattle. BMC Genet. 21 (1), 32–11. doi:10.1186/s12863-020-0837-6
Bedhane, M., van der Werf, J., Gondro, C., Duijvesteijn, N., Lim, D., Park, B., et al. (2019). Genome-wide Association Study of Meat Quality Traits in Hanwoo Beef Cattle Using Imputed Whole-Genome Sequence Data. Front. Genet. 10, 1235. doi:10.3389/fgene.2019.01235
Berry, D. P., Pabiou, T., Fanning, R., Evans, R. D., and Judge, M. M. (2019). Linear Classification Scores in Beef Cattle as Predictors of Genetic merit for Individual Carcass Primal Cut Yields1. J. Anim. Sci. 97 (6), 2329–2341. doi:10.1093/jas/skz138
Bhuiyan, M. S. A., Lim, D., Park, M., Lee, S., Kim, Y., Gondro, C., et al. (2018). Functional Partitioning of Genomic Variance and Genome-wide Association Study for Carcass Traits in Korean Hanwoo Cattle Using Imputed Sequence Level SNP Data. Front. Genet. 9, 217. doi:10.3389/fgene.2018.00217
Bongiorni, S., Mancini, G., Chillemi, G., Pariset, L., and Valentini, A. (2012). Identification of a Short Region on Chromosome 6 Affecting Direct Calving Ease in Piedmontese Cattle Breed. PLoS One 7 (12), e50137. doi:10.1371/journal.pone.0050137
Brunes, L. C., Baldi, F., Lopes, F. B., Lôbo, R. B., Espigolan, R., Costa, M. F. O., et al. (2021). Weighted Single‐step Genome‐wide Association Study and Pathway Analyses for Feed Efficiency Traits in Nellore Cattle. J. Anim. Breed. Genet. 138 (1), 23–44. doi:10.1111/jbg.12496
Cai, Z., Dusza, M., Guldbrandtsen, B., Lund, M. S., and Sahana, G. (2020). Distinguishing Pleiotropy from Linked QTL between Milk Production Traits and Mastitis Resistance in Nordic Holstein Cattle. Genet. Sel Evol. 52 (1), 19–15. doi:10.1186/s12711-020-00538-6
Cánovas, A., Reverter, A., DeAtley, K. L., Ashley, R. L., Colgrave, M. L., Fortes, M. R. S., et al. (2014). Multi-tissue Omics Analyses Reveal Molecular Regulatory Networks for Puberty in Composite Beef Cattle. PloS one 9 (7), e102551. doi:10.1371/journal.pone.0102551
Chang, T., Xia, J., Xu, L., Wang, X., Zhu, B., Zhang, L., et al. (2018). A Genome-wide Association Study Suggests Several Novel Candidate Genes for Carcass Traits in Chinese Simmental Beef Cattle. Anim. Genet. 49 (4), 312–316. doi:10.1111/age.12667
Cheng, H., Qu, L., Garrick, D. J., and Fernando, R. L. (2015). A Fast and Efficient Gibbs Sampler for BayesB in Whole-Genome Analyses. Genet. Sel Evol. 47 (1), 80–87. doi:10.1186/s12711-015-0157-x
Cheng, H., Fernando, R., and Garrick, D. (2018). “JWAS: Julia Implementation of Whole-Genome Analysis Software,” in Proceedings Of the World congress on Genetics Applied to Livestock Production), 859.
Choi, T. J., Alam, M., Cho, C. I., Lee, J. G., Park, B., Kim, S., et al. (2015). Genetic Parameters for Yearling Weight, Carcass Traits, and Primal-Cut Yields of Hanwoo Cattle1. J. Anim. Sci. 93 (4), 1511–1521. doi:10.2527/jas.2014-7953
Cohen-Zinder, M., Seroussi, E., Larkin, D. M., Loor, J. J., Everts-Van Der Wind, A., Lee, J.-H., et al. (2005). Identification of a Missense Mutation in the Bovine ABCG2 Gene with a Major Effect on the QTL on Chromosome 6 Affecting Milk Yield and Composition in Holstein Cattle. Genome Res. 15 (7), 936–944. doi:10.1101/gr.3806705
Daetwyler, H. D., Schenkel, F. S., Sargolzaei, M., and Robinson, J. A. B. (2008). A Genome Scan to Detect Quantitative Trait Loci for Economically Important Traits in Holstein Cattle Using Two Methods and a Dense Single Nucleotide Polymorphism Map. J. Dairy Sci. 91 (8), 3225–3236. doi:10.3168/jds.2007-0333
de Camargo, G. M. F., Costa, R. B., de Albuquerque, L. G., Regitano, L. C. A., Baldi, F., and Tonhati, H. (2015). Polymorphisms in TOX and NCOA2 Genes and Their Associations with Reproductive Traits in Cattle. Reprod. Fertil. Dev. 27 (3), 523–528. doi:10.1071/rd13360
Doyle, J. L., Berry, D. P., Veerkamp, R. F., Carthy, T. R., Evans, R. D., Walsh, S. W., et al. (2020a). Genomic Regions Associated with Muscularity in Beef Cattle Differ in Five Contrasting Cattle Breeds. Genet. Sel Evol. 52 (1), 2–18. doi:10.1186/s12711-020-0523-1
Doyle, J. L., Berry, D. P., Veerkamp, R. F., Carthy, T. R., Walsh, S. W., Evans, R. D., et al. (2020b). Genomic Regions Associated with Skeletal Type Traits in Beef and Dairy Cattle Are Common to Regions Associated with Carcass Traits, Feed Intake and Calving Difficulty. Front. Genet. 11, 20. doi:10.3389/fgene.2020.00020
Feng, Z., Li, X., Cheng, J., Jiang, R., Huang, R., Wang, D., et al. (2020). Copy Number Variation of the PIGY Gene in Sheep and its Association Analysis with Growth Traits. Animals 10 (4), 688. doi:10.3390/ani10040688
Fernando, R. L., Cheng, H., Golden, B. L., and Garrick, D. J. (2016). Computational Strategies for Alternative Single-step Bayesian Regression Models with Large Numbers of Genotyped and Non-genotyped Animals. Genet. Sel Evol. 48 (1), 96–98. doi:10.1186/s12711-016-0273-2
Fernando, R. L., Dekkers, J. C., and Garrick, D. J. (2014). A Class of Bayesian Methods to Combine Large Numbers of Genotyped and Non-genotyped Animals for Whole-Genome Analyses. Genet. Sel Evol. 46 (1), 50–13. doi:10.1186/1297-9686-46-50
Fernando, R. L., and Garrick, D. (2013). “Bayesian Methods Applied to GWAS,” in Genome-wide Association Studies and Genomic Prediction (Springer), 237–274. doi:10.1007/978-1-62703-447-0_10
Fernando, R., Toosi, A., Wolc, A., Garrick, D., and Dekkers, J. (2017). Application of Whole-Genome Prediction Methods for Genome-wide Association Studies: a Bayesian Approach. Jabes 22 (2), 172–193. doi:10.1007/s13253-017-0277-6
Fortes, M. R. S., Lehnert, S. A., Bolormaa, S., Reich, C., Fordyce, G., Corbet, N. J., et al. (2012). Finding Genes for Economically Important Traits: Brahman Cattle Puberty. Anim. Prod. Sci. 52 (3), 143–150. doi:10.1071/an11165
Grigoletto, L., Ferraz, J. B. S., Oliveira, H. R., Eler, J. P., Bussiman, F. O., Abreu Silva, B. C., et al. (2020). Genetic Architecture of Carcass and Meat Quality Traits in Montana Tropical Composite Beef Cattle. Front. Genet. 11, 123. doi:10.3389/fgene.2020.00123
Guo, Y. M., Zhang, Z. Y., Ma, J. W., Ai, H. S., Ren, J., and Huang, L. S. (2015). A Genomewide Association Study of Feed Efficiency and Feeding Behaviors at Two Fattening Stages in a White Duroc × Erhualian F2 Population1. J. Anim. Sci. 93 (4), 1481–1489. doi:10.2527/jas.2014-8655
Habier, D., Fernando, R. L., Kizilkaya, K., and Garrick, D. J. (2011). Extension of the Bayesian Alphabet for Genomic Selection. BMC bioinformatics 12 (1), 186–212. doi:10.1186/1471-2105-12-186
Hoshiba, H., Setoguchi, K., Watanabe, T., Kinoshita, A., Mizoshita, K., Sugimoto, Y., et al. (2013). Comparison of the Effects Explained by Variations in the bovinePLAG1andNCAPGgenes on Daily Body Weight Gain, Linear Skeletal Measurements and Carcass Traits in Japanese Black Steers from a Progeny Testing Program. Anim. Sci. J. 84 (7), 529–534. doi:10.1111/asj.12033
Jo, C., Cho, S. H., Chang, J., and Nam, K. C. (2012). Keys to Production and Processing of Hanwoo Beef: A Perspective of Tradition and Science. Anim. Front. 2 (4), 32–38. doi:10.2527/af.2012-0060
Judge, M. M., Pabiou, T., Murphy, J., Conroy, S. B., Hegarty, P. J., and Berry, D. P. (2019). Potential Exists to Change, through Breeding, the Yield of Individual Primal Carcass Cuts in Cattle without Increasing Overall Carcass Weight1. J. Anim. Sci. 97 (7), 2769–2779. doi:10.1093/jas/skz152
Kim, S., Alam, M., and Park, M. N. (2017). Breeding Initiatives for Hanwoo Cattle to Thrive as a Beef Industry–A Review Study. J. Anim. Breed. Genomics 1 (2), 103.
Lee, S. H., Choi, B. H., Lim, D., Gondro, C., Cho, Y. M., Dang, C. G., et al. (2013). Genome-wide Association Study Identifies Major Loci for Carcass Weight on BTA14 in Hanwoo (Korean Cattle). PloS one 8 (10), e74677. doi:10.1371/journal.pone.0074677
Lemos, M. V., Chiaia, H. L., Berton, M. P., Feitosa, F. L., Aboujaoud, C., Camargo, G. M., et al. (2016). Genome-wide Association between Single Nucleotide Polymorphisms with Beef Fatty Acid Profile in Nellore Cattle Using the Single Step Procedure. BMC genomics 17 (1), 213–216. doi:10.1186/s12864-016-2511-y
Li, B., VanRaden, P. M., Null, D. J., O'Connell, J. R., and Cole, J. B. (2021). Major Quantitative Trait Loci Influencing Milk Production and Conformation Traits in Guernsey Dairy Cattle Detected on Bos taurus Autosome 19. J. Dairy Sci. 104 (1), 550–560. doi:10.3168/jds.2020-18766
Lindholm-Perry, A. K., Sexten, A. K., Kuehn, L. A., Smith, T. P., King, D. A., Shackelford, S. D., et al. (2011). Association, Effects and Validation of Polymorphisms within the NCAPG - LCORL Locus Located on BTA6 with Feed Intake, Gain, Meat and Carcass Traits in Beef Cattle. BMC Genet. 12 (1), 103–113. doi:10.1186/1471-2156-12-103
Liu, R., Sun, Y., Zhao, G., Wang, H., Zheng, M., Li, P., et al. (2015). Identification of Loci and Genes for Growth Related Traits from a Genome-wide Association Study in a Slow- × Fast-Growing Broiler Chicken Cross. Genes Genom 37 (10), 829–836. doi:10.1007/s13258-015-0314-1
Ma, X., Ren, L., Zhang, N., Liu, C., Zhu, Y., and Xiao, J. (2017). Knock-down of KHDRBS3 Gene Inhibits Proliferation of Human Ovarian Cancer CAOV-3 Cells. Xi Bao Yu Fen Zi Mian Yi Xue Za Zhi 33 (8), 1062–1066.
Magalhães, A. F. B., de Camargo, G. M. F., Fernandes, G. A., Gordo, D. G. M., Tonussi, R. L., Costa, R. B., et al. (2016). Genome-wide Association Study of Meat Quality Traits in Nellore Cattle. PLoS One 11 (6), e0157845. doi:10.1371/journal.pone.0157845
Mariman, E. C. M., and Wang, P. (2010). Adipocyte Extracellular Matrix Composition, Dynamics and Role in Obesity. Cell. Mol. Life Sci. 67 (8), 1277–1292. doi:10.1007/s00018-010-0263-4
Matukumalli, L. K., Lawley, C. T., Schnabel, R. D., Taylor, J. F., Allan, M. F., Heaton, M. P., et al. (2009). Development and Characterization of a High Density SNP Genotyping Assay for Cattle. PloS one 4 (4), e5350. doi:10.1371/journal.pone.0005350
McClure, M. C., Morsci, N. S., Schnabel, R. D., Kim, J. W., Yao, P., Rolf, M. M., et al. (2010). A Genome Scan for Quantitative Trait Loci Influencing Carcass, post-natal Growth and Reproductive Traits in Commercial Angus Cattle. Anim. Genet. 41 (6), 597–607. doi:10.1111/j.1365-2052.2010.02063.x
Mehrban, H., Lee, D. H., Moradi, M. H., IlCho, C., Naserkheil, M., and Ibáñez-Escriche, N. (2017). Predictive Performance of Genomic Selection Methods for Carcass Traits in Hanwoo Beef Cattle: Impacts of the Genetic Architecture. Genet. Sel Evol. 49 (1), 1–13. doi:10.1186/s12711-016-0283-0
Mehrban, H., Naserkheil, M., Lee, D. H., Cho, C., Choi, T., Park, M., et al. (2021). Genomic Prediction Using Alternative Strategies of Weighted Single-step Genomic BLUP for Yearling Weight and Carcass Traits in Hanwoo Beef Cattle. Genes 12 (2), 266. doi:10.3390/genes12020266
Meyer, K. (2003). Secateurs software.[WWW Document]. Available at: http://agbu.une.edu.au/∼kmeyer/PRUNE/secateurs.pdf.
Meyer, K., and Tier, B. (2012). "SNP Snappy": A Strategy for Fast Genome-wide Association Studies Fitting a Full Mixed Model. Genetics 190 (1), 275–277. doi:10.1534/genetics.111.134841
Misztal, I., Legarra, A., and Aguilar, I. (2009). Computing Procedures for Genetic Evaluation Including Phenotypic, Full Pedigree, and Genomic Information. J. Dairy Sci. 92 (9), 4648–4655. doi:10.3168/jds.2009-2064
Misztal, I., Tsuruta, S., Lourenco, D., Aguilar, I., Legarra, A., and Vitezica, Z. (2015). Manual for BLUPF90 Family of Programs. Athens, Greece: University of Georgia, 199.
Monte, M. J., Marin, J. J., Antelo, A., and Vazquez-Tato, J. (2009). Bile Acids: Chemistry, Physiology, and Pathophysiology. Wjg 15 (7), 804. doi:10.3748/wjg.15.804
Moser, G., Lee, S. H., Hayes, B. J., Goddard, M. E., Wray, N. R., and Visscher, P. M. (2015). Simultaneous Discovery, Estimation and Prediction Analysis of Complex Traits Using a Bayesian Mixture Model. PLoS Genet. 11 (4), e1004969. doi:10.1371/journal.pgen.1004969
Naserkheil, M., Bahrami, A., Lee, D., and Mehrban, H. (2020). Integrating Single-step GWAS and Bipartite Networks Reconstruction Provides Novel Insights into Yearling Weight and Carcass Traits in Hanwoo Beef Cattle. Animals 10 (10), 1836. doi:10.3390/ani10101836
Nishimura, S., Watanabe, T., Mizoshita, K., Tatsuda, K., Fujita, T., Watanabe, N., et al. (2012). Genome-wide Association Study Identified Three Major QTL for Carcass Weight Including the PLAG1-CHCHD7 QTN for Stature in Japanese Black Cattle. BMC Genet. 13 (1), 40–11. doi:10.1186/1471-2156-13-40
Olsen, H. G., Lien, S., Gautier, M., Nilsen, H., Roseth, A., Berg, P. R., et al. (2005). Mapping of a Milk Production Quantitative Trait Locus to a 420-kb Region on Bovine Chromosome 6. Genetics 169 (1), 275–283. doi:10.1534/genetics.104.031559
Peters, S. O., Kizilkaya, K., Garrick, D. J., Fernando, R. L., Reecy, J. M., Weaber, R. L., et al. (2012). Bayesian Genome-wide Association Analysis of Growth and Yearling Ultrasound Measures of Carcass Traits in Brangus Heifers1. J. Anim. Sci. 90 (10), 3398–3409. doi:10.2527/jas.2011-4507
Purfield, D. C., Evans, R. D., and Berry, D. P. (2019). Reaffirmation of Known Major Genes and the Identification of Novel Candidate Genes Associated with Carcass-Related Metrics Based on Whole Genome Sequence within a Large Multi-Breed Cattle Population. BMC genomics 20 (1), 720–817. doi:10.1186/s12864-019-6071-9
Raudvere, U., Kolberg, L., Kuzmin, I., Arak, T., Adler, P., Peterson, H., et al. (2019). g:Profiler: a Web Server for Functional Enrichment Analysis and Conversions of Gene Lists (2019 Update). Nucleic Acids Res. 47 (W1), W191–W198. doi:10.1093/nar/gkz369
Ribeiro, V. M. P., Gouveia, G. C., Moraes, M. M. d., Araújo, A. E. M. d., Raidan, F. S. S., Fonseca, P. A. d. S., et al. (2021). Genes Underlying Genetic Correlation between Growth, Reproductive and Parasite burden Traits in Beef Cattle. Livestock Sci. 244, 104332. doi:10.1016/j.livsci.2020.104332
Roberts, A. (2018). Genome-wide Association Study for Carcass Traits in a Composite Beef Cattle Breed. Livestock Sci. 213, 35–43.
Saatchi, M., Schnabel, R. D., Taylor, J. F., and Garrick, D. J. (2014). Large-effect Pleiotropic or Closely Linked QTL Segregate within and across Ten US Cattle Breeds. BMC genomics 15 (1), 442–517. doi:10.1186/1471-2164-15-442
Sadkowski, T., Jank, M., Zwierzchowski, L., Oprządek, J., and Motyl, T. (2009). Comparison of Skeletal Muscle Transcriptional Profiles in Dairy and Beef Breeds Bulls. J. Appl. Genet. 50 (2), 109–123. doi:10.1007/bf03195662
Salameh, A., Daquinag, A. C., Staquicini, D. I., An, Z., Hajjar, K. A., Pasqualini, R., et al. (2016). Prohibitin/annexin 2 Interaction Regulates Fatty Acid Transport in Adipose Tissue. JCI insight 1 (10). doi:10.1172/jci.insight.86351
Sargolzaei, M., Chesnais, J. P., and Schenkel, F. S. (2014). A New Approach for Efficient Genotype Imputation Using Information from Relatives. BMC genomics 15 (1), 478–512. doi:10.1186/1471-2164-15-478
Sarti, F. M., Pieramati, C., Lubricchio, E., Giontella, A., Lasagna, E., and Panella, F. (2013). Genetic Parameters for the Weights and Yields of Carcass Cuts in Chianina Cattle1. J. Anim. Sci. 91 (9), 4099–4103. doi:10.2527/jas.2012-6105
Seabury, C. M., Oldeschulte, D. L., Saatchi, M., Beever, J. E., Decker, J. E., Halley, Y. A., et al. (2017). Genome-wide Association Study for Feed Efficiency and Growth Traits in U.S. Beef Cattle. BMC genomics 18 (1), 386–425. doi:10.1186/s12864-017-3754-y
Snelling, W. M., Allan, M. F., Keele, J. W., Kuehn, L. A., McDaneld, T., Smith, T. P. L., et al. (2010). Genome-wide Association Study of Growth in Crossbred Beef Cattle12. J. Anim. Sci. 88 (3), 837–848. doi:10.2527/jas.2009-2257
Sorensen, D., Fernando, R., and Gianola, D. (2001). Inferring the Trajectory of Genetic Variance in the Course of Artificial Selection. Genet. Res. 77 (1), 83–94. doi:10.1017/s0016672300004845
Srikanth, K., Lee, S.-H., Chung, K.-Y., Park, J.-E., Jang, G.-W., Park, M.-R., et al. (2020). A Gene-Set Enrichment and Protein-Protein Interaction Network-Based GWAS with Regulatory SNPs Identifies Candidate Genes and Pathways Associated with Carcass Traits in Hanwoo Cattle. Genes 11 (3), 316. doi:10.3390/genes11030316
Srivastava, S., Srikanth, K., Won, S., Son, J.-H., Park, J.-E., Park, W., et al. (2020). Haplotype-Based Genome-wide Association Study and Identification of Candidate Genes Associated with Carcass Traits in Hanwoo Cattle. Genes 11 (5), 551. doi:10.3390/genes11050551
Strömberg, U. (2009). Empirical Bayes and Semi-bayes Adjustments for a Vast Number of Estimations. Eur. J. Epidemiol. 24 (12), 737–741. doi:10.1007/s10654-009-9393-0
Taye, M., Yoon, J., Dessie, T., Cho, S., Oh, S. J., Lee, H.-K., et al. (2018). Deciphering Signature of Selection Affecting Beef Quality Traits in Angus Cattle. Genes Genom 40 (1), 63–75. doi:10.1007/s13258-017-0610-z
Wang, H., Misztal, I., Aguilar, I., Legarra, A., and Muir, W. M. (2012). Genome-wide Association Mapping Including Phenotypes from Relatives without Genotypes. Genet. Res. 94 (2), 73–83. doi:10.1017/s0016672312000274
Wang, T., Chen, Y. P., Bowman, P. J., Goddard, M. E., and Hayes, B. J. (2016). A Hybrid Expectation Maximisation and MCMC Sampling Algorithm to Implement Bayesian Mixture Model Based Genomic Prediction and QTL Mapping. BMC genomics 17 (1), 744–821. doi:10.1186/s12864-016-3082-7
Wang, Z., Chapman, D., Morota, G., and Cheng, H. (2020). A Multiple-Trait Bayesian Variable Selection Regression Method for Integrating Phenotypic Causal Networks in Genome-wide Association Studies. G3: Genes, Genomes, Genet. 10 (12), 4439–4448. doi:10.1534/g3.120.401618
Weikard, R., Altmaier, E., Suhre, K., Weinberger, K. M., Hammon, H. M., Albrecht, E., et al. (2010). Metabolomic Profiles Indicate Distinct Physiological Pathways Affected by Two Loci with Major Divergent Effect on Bos taurus Growth and Lipid Deposition. Physiol. genomics 42A (2), 79–88. doi:10.1152/physiolgenomics.00120.2010
Weng, Z., Su, H., Saatchi, M., Lee, J., Thomas, M. G., Dunkelberger, J. R., et al. (2016). Genome-wide Association Study of Growth and Body Composition Traits in Brangus Beef Cattle. Livestock Sci. 183, 4–11. doi:10.1016/j.livsci.2015.11.011
Xia, J., Fan, H., Chang, T., Xu, L., Zhang, W., Song, Y., et al. (2017). Searching for New Loci and Candidate Genes for Economically Important Traits through Gene-Based Association Analysis of Simmental Cattle. Sci. Rep. 7 (1), 42048–42049. doi:10.1038/srep42048
Yi, N., and Shriner, D. (2008). Advances in Bayesian Multiple Quantitative Trait Loci Mapping in Experimental Crosses. Heredity 100 (3), 240–252. doi:10.1038/sj.hdy.6801074
Zhang, R., Miao, J., Song, Y., Zhang, W., Xu, L., Chen, Y., et al. (2019). Genome-wide Association Study Identifies the PLAG1-OXR1 Region on BTA14 for Carcass Meat Yield in Cattle. Physiol. genomics 51 (5), 137–144. doi:10.1152/physiolgenomics.00112.2018
Zhao, G.-X., Liu, Y., Li, Z.-X., Lv, C.-Z., Traboulsee, A., Sadovnick, A. D., et al. (2013). Variants in the Promoter Region of CYP7A1 Are Associated with Neuromyelitis Optica but Not with Multiple Sclerosis in the Han Chinese Population. Neurosci. Bull. 29 (5), 525–530. doi:10.1007/s12264-013-1347-6
Keywords: candidate genes, QTL, carcass primal cut yield, single-step GWAS, hanwoo
Citation: Naserkheil M, Mehrban H, Lee D and Park MN (2021) Genome-wide Association Study for Carcass Primal Cut Yields Using Single-step Bayesian Approach in Hanwoo Cattle. Front. Genet. 12:752424. doi: 10.3389/fgene.2021.752424
Received: 03 August 2021; Accepted: 02 November 2021;
Published: 26 November 2021.
Edited by:
Hao Cheng, University of California, Davis, United StatesReviewed by:
Gota Morota, Virginia Tech, United StatesCopyright © 2021 Naserkheil, Mehrban, Lee and Park. This is an open-access article distributed under the terms of the Creative Commons Attribution License (CC BY). The use, distribution or reproduction in other forums is permitted, provided the original author(s) and the copyright owner(s) are credited and that the original publication in this journal is cited, in accordance with accepted academic practice. No use, distribution or reproduction is permitted which does not comply with these terms.
*Correspondence: Deukmin Lee, ZGhsZWVAaGtudS5hYy5rcg==; Mi Na Park, bWluYTA0MTJAa29yZWEua3I=
Disclaimer: All claims expressed in this article are solely those of the authors and do not necessarily represent those of their affiliated organizations, or those of the publisher, the editors and the reviewers. Any product that may be evaluated in this article or claim that may be made by its manufacturer is not guaranteed or endorsed by the publisher.
Research integrity at Frontiers
Learn more about the work of our research integrity team to safeguard the quality of each article we publish.