- 1Section Genomics of Neurodegenerative Diseases and Aging, Department of Clinical Genetics, Vrije Universiteit Amsterdam, Amsterdam, Netherlands
- 2Alzheimer Centre, Department of Neurology, Amsterdam Neuroscience, Vrije Universiteit Amsterdam, Amsterdam, Netherlands
- 3Delft Bioinformatics Lab, Delft University of Technology, Delft, Netherlands
- 4Department of Complex Trait Genetics, Center for Neurogenomics and Cognitive Research, VU, Amsterdam, Netherlands
- 5Department of Epidemiology and Data Sciences, Vrije Universiteit Amsterdam, Amsterdam, Netherlands
Human longevity is influenced by the genetic risk of age-related diseases. As Alzheimer’s disease (AD) represents a common condition at old age, an interplay between genetic factors affecting AD and longevity is expected. We explored this interplay by studying the prevalence of AD-associated single-nucleotide-polymorphisms (SNPs) in cognitively healthy centenarians, and replicated findings in a parental-longevity GWAS. We found that 28/38 SNPs that increased AD-risk also associated with lower odds of longevity. For each SNP, we express the imbalance between AD- and longevity-risk as an effect-size distribution. Based on these distributions, we grouped the SNPs in three groups: 17 SNPs increased AD-risk more than they decreased longevity-risk, and were enriched for β-amyloid metabolism and immune signaling; 11 variants reported a larger longevity-effect compared to their AD-effect, were enriched for endocytosis/immune-signaling, and were previously associated with other age-related diseases. Unexpectedly, 10 variants associated with an increased risk of AD and higher odds of longevity. Altogether, we show that different AD-associated SNPs have different effects on longevity, including SNPs that may confer general neuro-protective functions against AD and other age-related diseases.
Introduction
The human lifespan is determined by a beneficial combination of environmental and genetic factors (Partridge et al., 2018; Melzer et al., 2019). Long-lived individuals tend to cluster in families, suggesting that genetic factors play a considerable role (Perls et al., 2002; Caselli et al., 2006), however, the research of genetic variants that influence human lifespan has yielded contrasting results: only the association of the APOE alleles and few additional variants (in/near CDKN2B and ABO) consistently replicated across studies (Deelen et al., 2019; Timmers et al., 2019). While the replication rate in independent studies is low, a large collection of genetic variants has been associated with longevity through genome-wide association studies (GWAS) in different studies and populations (Deelen et al., 2019; Timmers et al., 2019). The majority of these variants was previously associated with other age-related conditions, including cardiovascular disease, autoimmune and neurological disorders, suggesting that the genetics underlying human longevity depends on a lower genetic risk for age-related diseases (Deelen et al., 2019; Melzer et al., 2019; Timmers et al., 2019).
Of all age-related diseases, late-onset Alzheimer’s disease (AD) is the most common type of dementia and one of the most prevalent causes of death at old age (Alzheimer’s Association, 2012). The largest risk factor for AD is aging: at 100 years of age, the disease’s incidence is about 40% per year (Corrada et al., 2010). Genetic factors play a significant role in AD as heritability was estimated to range 60–80% (Gatz et al., 2006): the strongest common genetic risk factor for AD is the APOE-ε4 allele, and large collaborative GWAS have identified 41 additional common variants associated with a slight modification of the risk of AD (Sims et al., 2017; Jansen et al., 2019; Kunkle et al., 2019; de Rojas et al., 2021). Despite high incidence rates of AD at very old ages, AD is not an inevitable consequence of aging, as demonstrated by individuals who surpass the age of 100 years with high levels of cognitive functioning (Holstege et al., 2018).
As AD-associated variants increase the risk of AD, leading to earlier death, a negative effect on longevity for these variants should be expected. However, apart from APOE alleles, genetic variants that influence the risk of AD were not found to affect the human lifespan in previous GWAS. In fact, often we assume that AD-associated variants affect AD only, but this may still not hold true. For example, at the molecular level, there may be other age-related traits that share (part of) the biological pathways underlying AD. Nevertheless, for an AD-associated variant that affects AD only, the relative effect on longevity should be proportional to the corresponding effect on AD, albeit in a different direction. This means that if a variant increases the risk of AD 2-fold, then carriers will have twice as much AD-related mortality as non-carriers, and as a consequence, they will have twice as little chance to age into a cognitively healthy centenarian. However, in case a genetic variant is protective against multiple conditions, it might be expected that the overall effect on longevity results larger than the absolute effect on AD alone.
We have previously shown that cognitively healthy centenarians are depleted with genetic variants that increased the risk of AD compared to a general population. Yet, the extent of depletion was variant specific, suggesting that a subset of AD-variants may be specifically beneficial to reach extremely old ages in good cognitive health (Tesi et al., 2019; Tesi et al., 2020). In addition, the extent to which AD-associated variants affect other age-related diseases is mostly unknown (Ponomareva et al., 2013). Using the notion of effect-size proportionality, we set out to investigate the relationship between AD- and longevity-risk for genetic variants associated with AD.
Methods
Populations and Selection of Genetic Variants
We included N = 358 centenarians from the 100-plus Study cohort, which comprises Dutch-speaking individuals aged 100 years or older who self-report to be cognitively healthy, which is confirmed by a proxy (Holstege et al., 2018). As population controls, we used population-matched, cognitively healthy individuals from five studies: 1) the Longitudinal Aging Study of Amsterdam (LASA, N = 1,779), (Huisman et al., 2011; Hoogendijk et al., 2016), 2) the memory clinic of the Alzheimer center Amsterdam and SCIENCe project (N = 1,206), (Slot et al., 2018; van der Flier and Scheltens, 2018), 3) the Netherlands Brain Bank (N = 40), (Rademaker et al., 2018), 4) the twin study of Amsterdam (N = 201) (Willemsen et al., 2010) and 5) the 100-plus Study (partners of centenarian’s children, N = 86). (Holstege et al., 2018). see 5.1 Supplementary Methods: Populations for a detailed description of these cohorts. Throughout the manuscript, we will refer to the union of the individuals from these five studies as population subjects. The Medical Ethics Committee of the Amsterdam UMC (METC) approved all studies. All participants and/or their legal representatives provided written informed consent for participation in clinical and genetic studies.
Genetic variants in our populations were determined by standard genotyping and imputation methods. All samples were genotyped using the same commercial kit. After establishing quality control of the genetic data (see 5.2 Supplementary Methods: Quality control), 2,905 population subjects and 343 cognitively healthy centenarians were left for the analyses (Table 1). We then selected 41 variants representing the current genetic landscape of AD (Supplementary Table S1) (de Rojas et al., 2021). We restricted our analysis to high-quality variants with a minor allele frequency >1% in our cohorts, which led to the exclusion of 3/41 variants (rare variants in the TREM2 gene rs143332484 and rs75932628 and ABI3 gene rs616338), leaving 38 variants for the analyses.
Alzheimer’s Disease and Longevity Variant Effect Sizes
We first retrieved the effect-size on AD (
Imbalance of Variant Effect Direction
We represented each variant as a data point whose coordinates are defined by the variant’s effect on AD (
Replication of Findings in Large Genome-Wide Association Studies Cohorts
To find additional evidence for our findings, we inspected the association statistics of the 38 AD-associated variants in the largest GWAS on parental longevity. (Timmers et al., 2019). Briefly, in this study offspring’s genotypes were used to model parental age at death. We did not use a case-control GWAS of longevity as the most recent included our cohort, thus the resulting associations would be biased. (Deelen et al., 2019). In this dataset, we looked at the significance of association with longevity of the 38 variants (p-values were corrected with FDR) and their direction of effect. Finally, we tested the consistency in the expected/unexpected directions between our study and the GWAS on parental longevity using binomial tests.
Linking Variants With Functional Clusters
To investigate each variant’s functional consequences, we calculated the variant-pathway mapping, which indicates the degree of involvement of each genetic variant in AD-associated pathways (Supplementary Figure S2). see 5.3 Supplementary Methods: variant-pathway mapping for a detailed explanation of our approach. Briefly, the variant-pathway mapping depends on 1) the number of genes each variant was associated with and 2) the biological pathways each gene was associated with. We calculated the variant-pathway mapping for all 38 AD-associated variants. Finally, we compared the variant-pathway mapping within each group of variants defined based on the
Cell-Type Annotation at The Level of Each Cluster
To further explore the biological basis of the different groups of variants (Longevity-, AD- and Unex-groups), we calculated the degree of enrichment of each group for specific brain cell-types (see 5.4 Supplementary Methods: cell-type annotation for a detailed description). This annotation depends on the number of genes each variant was associated with, and the expression of these genes in the different brain cell-types, i.e., astrocytes, oligodendrocytes, microglia, endothelial cells, and neurons. We finally compared the cell-specific annotations within each group of variants (Longevity-, AD- and Unex-groups) using Wilcoxon sum rank tests and correcting p-values using FDR, which indicated whether a group of variants was enriched for specific brain cell-types (Supplementary Figure S2).
Implementation
Quality control of genotype data, population stratification analysis and relatedness analysis were performed with PLINK (v2.0 and v1.9). All subsequent analyses were performed with R (v3.6.3), Bash, and Python (v3.6) scripts. All scripts are freely available at https://github.com/TesiNicco/Disentangle_AD_Age. Variant-gene annotation and gene-set enrichment analyses were performed through the web-server that is freely accessible at https://snpxplorer.net. (Tesi et al., 2021).
Results
Alzheimer’s Disease-Associated Variants Also Associate With Longevity
We explored the association with longevity of 38 genetic variants previously associated with AD from GWAS (Supplementary Table S1). We tested these variants in 343 centenarians who self-reported to be cognitively healthy (mean age at inclusion 101.4 ± 1.3, 74.7% females), as opposed to 2,905 population subjects (mean age at inclusion 68.3 ± 11.5, 50.7% females). We found a significant association with longevity for two variants after multiple testing correction (FDR<5%, variants in the APOE gene; rs429358 and rs7412, Supplementary Table S2). We compared the direction of effect on longevity with that on AD as found in literature: of the 38 variants, 28 showed an association in the expected direction, i.e., alleles that increased AD risk were associated with lower odds of longevity, and this was significantly more than expected by chance (p = 0.005 including APOE variants, p = 0.01 excluding APOE variants, see 2.2 Methods).
Distributions of the Imbalance in the Effect Direction ( )
To study the relationship between the effect on AD and longevity for all 38 AD-associated variants in more detail, we created distributions of the imbalance in the variant effect direction (
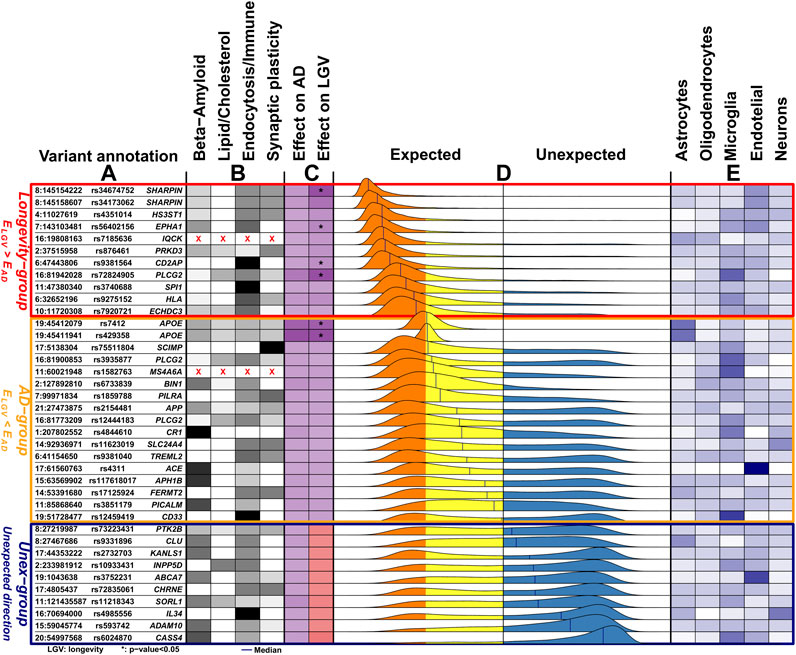
FIGURE 1. Overview of the 38 genetic variants associated with Alzheimer’s disease. (A) The genomic position of the variants (chromosome: position), variant identifier, and closest gene. Genomic positions are with respect to GRCh37 (hg19). (B) The variant-pathway mapping score of association with the four functional clusters (darker colors representing stronger associations). Variants annotated with red crosses could not be annotated to any one of the functional clusters as no biological processes are associated with the related genes. (C) The effect size on AD (from literature) and the observed effect size on longevity (LGV) for each variant (darker color indicating stronger effect). The same color indicates expected direction (i.e., increased risk of AD and decreased chance of longevity), while different colors, visible in the Unex-group of variants, indicates unexpected direction. For the longevity effects, we also annotate variants for which we observed a significant association (unadjusted p-value < 0.05). (D) The distribution of the imbalance direction of variant effect (
Grouping Variants Based on Distributions
Based on the median value of each
Alzheimer’s Disease-Associated Variants in Large Genome-Wide Association Studies of Longevity
To find additional evidence for longevity associations, we inspected the effect of AD-associated variants in the largest GWAS of parental longevity. (Timmers et al., 2019). Of the 38 AD-associated variants, association statistics were available for 34 of the variants (missing from Longevity-group: rs72824905 and rs3740688; missing from Unex-group: rs2732703 and rs10933431). Overall, 21/26 (81%) of the variants in the expected direction in our study (of which 6/9 variants in Longevity- and 15/17 variants in the AD-group), were also in the expected direction in the independent parental longevity dataset. Variants in the expected direction in the first analysis were significantly more likely to be in the expected direction in the replication analysis (p = 0.01, based on a binomial test, Figure 2). Six AD-associated variants reached significance in the parental-longevity GWAS after correcting for multiple comparisons (FDR<5%): variants in the APOE gene (rs429358 and rs7412) and those in/near PRKD3 (rs8764613), CD2AP (rs9381564), APH1B (rs117618,017) and BIN1 (rs6733839). Of these, variants in/near PRKD3 and CD2AP belonged to the Longevity-group in our analysis. Conversely, only 2/8 (25%) variants that we observed in the unexpected direction in our study were also in the unexpected direction in the parental-longevity GWAS, such that these variants were not more likely to be in the unexpected direction (p = 0.29, based on a binomial test, Figure 2).
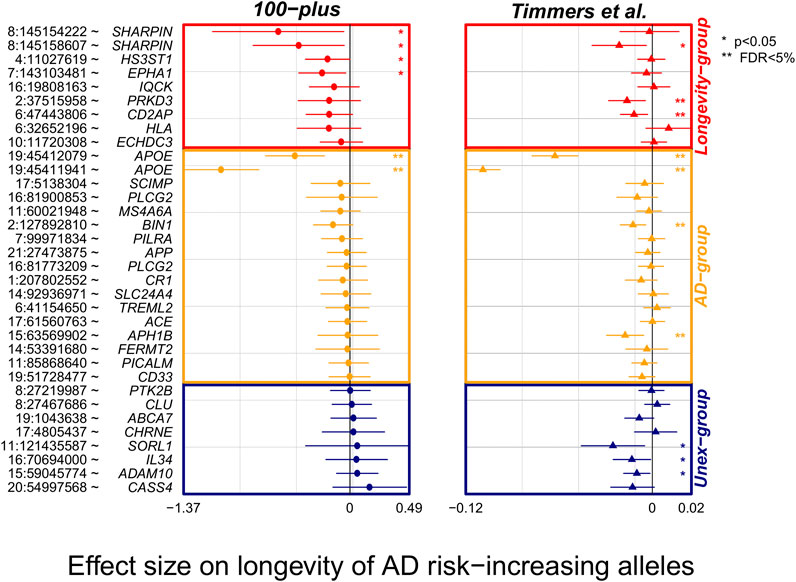
FIGURE 2. Forest plot of association statistics of AD-variants in our study and the largest GWAS of parental longevity. The plot shows the association of AD-variants in our study and the largest by-proxy GWAS on parental longevity (Timmers et al., 2019). The association statistics of 34/38 variants were available from publicly available summary statistics of Timmers et al. study. Plotted effect-sizes are with respect to the AD-risk increasing allele. Thus, an expected direction of effect is shown for variants with a negative estimate. Nominally significant associations with AD (p < 0.05) are annotated with an asterisk (*), and significant associations after FDR correction are annotated with two asterisks (**).
Functional Characterization of Variants
The 38 AD-associated variants included coding variants (N = 10), intronic variants (N = 20), and intergenic variants (N = 8) (Supplementary Table S3). 12/28 of the intronic/intergenic variants had eQTL associations. In total, the 38 variants mapped to 68 unique genes, with most variants mapping to one gene (N = 21) and fewer mapping to 2 genes (N = 10), 3 genes (N = 2), 4 genes (N = 1), 5 genes (N = 2), 6 and 7 genes (N = 1, respectively) (Supplementary Figure S3 and Supplementary Table S3).
We performed gene-set enrichment analysis using a sampling-based approach to explore the biological processes enriched in the 68 genes associated with AD-variants (see 2.4 Methods and Supplementary Figure S2). We found 115 significantly enriched biological processes after correction for multiple tests (FDR<5%, Supplementary Table S4). After clustering these terms based on their semantic similarity, we found four main clusters of biological processes: 1)
• Next, we calculated the variant-pathway mapping score (see 2.4 Methods and Supplementary Figure S2), which indicates how well a variant is associated with each of the 4 functional clusters. In total, we calculated the variant-pathway mapping for 30 variants and we imputed the annotation of 6 variants (Supplementary Table S5), while 2 variants could not be annotated (variants rs7185636 and rs1582763 in/near IQCK and MS4A6A genes), because the associated genes were not annotated with any biological process function (Supplementary Table S5). Finally, we tested whether the Longevity-, AD- and Unex-groups were enriched at the functional level by comparing the distribution of variant-pathway mapping within each group (see 2.4 Methods, Figure 3, and Supplementary Figure S2). The Longevity-group was significantly enriched for the endocytosis/immune signaling functional cluster; the AD-group for the endocytosis/immune signaling,
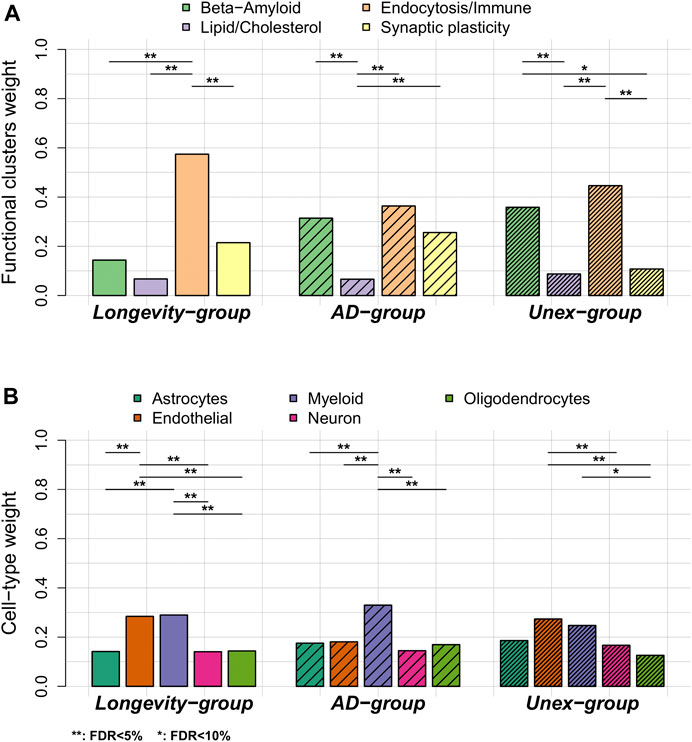
FIGURE 3. Comparison of functional annotation and cell-type annotation within the Longevity-, AD- and Unex-groups. (A) The weights of the 4 functional clusters within the Longevity-, AD- and Unex-groups. (B) The weights of the different cell-types in the brain, per group. Differences in functional weights and cell-type weights within each group were calculated using Wilcoxon sum rank tests. The resulting p-values were FDR-corrected.
Expression of Alzheimer’s Disease-Associated Genes in Brain Cell-types
We explored whether specific brain cell types, i.e., astrocytes, oligodendrocytes, microglia, endothelial cells and neurons, were enriched within each group of variants (see 2.5 Methods, Supplementary Tables S5, S6). Figure 1 shows the collapsed cell-type expression for all 38 AD-associated variants. We then tested the enrichment for cell-type expression within the Longevity-, AD- and Unex-groups. The Longevity-group was significantly enriched for myeloid and endothelial cells, the AD-group for myeloid cells, while the Unex-group was significantly enriched for endothelial cells (Figure 3).
Discussion
Summary of the Findings
We studied the effect on longevity of 38 genetic variants previously associated with AD through GWAS (de Rojas et al., 2021). We found that a majority of 74% of the alleles that increase the risk of AD is associated with lower odds of becoming a centenarian (expected direction). Overall, most variants (N = 17) had a larger effect on AD than on longevity: these variants were associated with
Alzheimer’s Disease-Associated Variants and Their Effect on Healthy Aging
A single study previously explored the extent to which 10 AD-associated variants affect longevity: apart from APOE locus, none of the other 10 tested AD-associated variants significantly associated with longevity (Shi et al., 2012). In addition to APOE, four variants showed a negative effect on longevity while increasing AD-risk (in/near ABCA7, EPHA1, CD2AP, and CLU). In agreement with these findings, we also found that only the APOE variants significantly associated with longevity, and variants in/near EPHA1 and CD2AP belong to the Longevity-group. However, in our study, we found that most alleles associated with an increased risk of AD associated with a decreased chance of longevity. The inability to observe such an inverse relationship between variant effects on AD and longevity in the previous study may be explained by the relatively small sample sizes, combined with a low number of (well-established) AD variants analyzed (N = 10). In our study, groups sizes were also relatively small, but the centenarians had a relatively high level of cognitive health, which might have contributed to an increased effect size of AD-associated genetic variants in our comparison (Tesi et al., 2019; Tesi et al., 2020).
Different Trajectories of Effect of Alzheimer’s Disease-Associated Variants on Longevity
Variants With a Larger Effect on Alzheimer’s Disease Than Longevity
For most variants with effects in the expected direction, the risk-increasing effect on AD was more extensive than the negative effect on survival/longevity. These variants, which include both APOE alleles, might negatively affect lifespan because carriers are removed from the population with increasing age due to AD-associated mortality. For the APOE variants specifically, the distribution of the imbalance in the effect directions (
Variants With a Larger Effect on Longevity Than Alzheimer’s Disease
The second-largest group of variants constituted a subset of 11 variants with a larger effect on longevity than the effect on AD, which suggests that these variants may be involved in other age-related diseases or general age-related processes. The AD-association of most of these variants is relatively recent, likely due to small effect sizes (ORs) or variants rareness; both features necessitate a very large number of samples to identify these variants as significantly associating with the disease. The variants within this group were specifically enriched for immune response and endocytosis, which are known hallmarks of longevity (Solé-Domènech et al., 2016; Partridge et al., 2018; Sadighi Akha, 2018). In addition to the low frequency, non-synonymous variant in the PLCG2 gene (rs72824905, MAF: 0.6%), which was recently observed to be protective against AD, frontotemporal dementia (FTD) and dementia with Lewy bodies, other variants within this group were previously linked with disease risk factors. One of the two non-synonymous variants in the SHARPIN gene, variant rs34173062 (MAF: 5.7%), has been associated with respiratory system diseases in GWAS (Astle et al., 2016; Kichaev et al., 2019; Olafsdottir et al., 2020). Variant rs7185636 (MAF: 17.1%), intronic of the IQCK gene, is in complete linkage with a variant (rs7191155, R2 = 0.95), which was previously associated with body-mass index (BMI) (Hoffmann et al., 2018). The variant rs876461 (MAF: 13.0%) near the PRKD3 gene is in linkage with variant rs13420463 (R2 = 0.42), which has been associated with systolic blood pressure (Warren et al., 2017). Further, the variant near CD2AP gene associates with the development and maintenance of the blood-brain barrier, a specialized vascular structure of the central nervous system which, when disrupted, has been linked with epilepsy, stroke and AD (Cochran et al., 2015). Variant rs9275152 (MAF: 10.4%) maps to the complex Human-Leukocyte-Antigen (HLA) region, which codes for cell-surface proteins responsible for the regulation of the adaptive immune system. In numerous GWAS, variants in the HLA region were associated with autoimmune diseases, cancer, and longevity (Bodis et al., 2018; Timmers et al., 2019). The AD-associated variant in this region (rs9275152) is also a risk variant for Parkinson’s disease (Bandres-Ciga et al., 2019). Finally, the genomic region surrounding the SPI1 gene (in which variant rs3740688 maps) has been previously associated with cognitive traits (intelligence, depression) (Davies et al., 2018) and, with lower evidence, with kidney disease and cancer (Pattaro et al., 2016; Michailidou et al., 2017). The remaining variants rs56402156, rs7920721, and rs4351014 (in/near EPHA1, ECHDC3, and HS3ST1) have not been directly associated with other traits, although their associated genes were implicated in systemic lupus erythematosus (HS3ST1) and cancer (EPHA1, ECHDC3) (Herath et al., 2009; Rafiq et al., 2014; Langefeld et al., 2017). Together, these findings suggest that the counterpart of each risk-increasing allele, the AD-protective alleles, might give a survival advantage that is not only specific to AD. Their functional and cell-type annotations suggest that they contribute to the maintenance of regulatory stimuli in the immune and endosomal systems, which may be essential to maintain brain and overall physical health, necessary to reach extremely old ages in good cognitive health (Tesi et al., 2020).
Variants Associated With Increased Risk of Alzheimer’s Disease and Increased Longevity Risk
Unexpectedly, ten variants increased the risk of AD while at the same time increasing the chance to reach ages over 100 in good cognitive health, which is an unexpected balance. We note that the
Strengths and Weaknesses
We acknowledge that our findings are based on relatively small sample sizes, especially for the cognitively healthy centenarian group. This phenotype is rare, and individuals need to be individually approached for study inclusion (Holstege et al., 2018), which is prohibitive for large sample collection. For this reason, we did not perform extensive gender-specific analyses and thus we cannot rule out possible interaction effects in males and/or females. However, we note that the frequency of AD-associated SNPs was not different between male centenarians and female centenarians. As population subjects in our comparison, we used individuals from five different cohorts: all individuals were from the same (Dutch) population, all tested cognitively intact, and did not develop dementia at the time of analyses. Although using a genetically homogeneous population represents a strength of this study, observed effects may differ in different populations and ethnicities, especially regarding the effects on longevity. This will require further study. It is known that the analysis of variants with small effect-sizes in relatively small sample sizes leads to large confidence intervals: we took this uncertainty into account by bootstrapping effect-sizes, causing the,
Conclusion
Most AD-associated variants that increase the risk of the disease are associated with lower odds of longevity. We identified a subset of variants with a larger effect on longevity than on AD, that were previously associated as risk-factors for other age-related diseases, and that are selectively enriched for endocytosis and immune signaling functions.
Data Availability Statement
The datasets presented in this study can be found in online repositories. The names of the repository/repositories and accession number(s) can be found in the article/Supplementary Material.
Ethics Statement
The studies involving human participants were reviewed and approved by Medical Ethics Committee of the Amsterdam UMC (METC) approved all studies. The patients/participants provided their written informed consent to participate in this study.
Author Contributions
Conceptualization, NT, MHul, SL, MR and HH; Data curation, SL, MHul, IJ, NS, NSc, PS, WF, MHui and HH; Formal analysis, NT; Funding acquisition, PS, WF, MHui, MR and HH; Methodology, NT, MHul, SL, MR and HH; Project administration, PS, WF, MHui, MR and HH; Software, NT, SL, MHul, MR; Supervision, NT, SL, MHul, MR and HH; Visualization, NT; Writing – original draft, NT, SL, MHul, MR and HH; Writing – review & editing, NT, SL, MHul, IJ, NS, NSc, PS, WF, MHui, MR and HH.
Funding
The Alzheimer center Amsterdam is supported by Stichting Alzheimer Nederland and Stichting VUmc fonds. The clinical database structure was developed with funding from Stichting Dioraphte. The SCIENCe project is supported by a research grant from Gieskes-Strijbis fonds and stichting Dioraphte. Genotyping of the Dutch case-control samples was performed in the context of EADB (European Alzheimer DNA biobank), funded by the JPco-fuND FP-829–029 (ZonMW projectnumber 733051061). The 100-plus Study was supported by Stichting Alzheimer Nederland (WE09.2014–03), Stichting Diorapthe, horstingstuit foundation, Memorabel (ZonMW projectnumber 733050814), and Stichting VUmc fonds. Genotyping of the 100-plus study was performed in the context of EADB (European Alzheimer DNA biobank) funded by the JPco-fuND FP-829–029 (ZonMW projectnumber 733051061). Longitudinal Aging Study Amsterdam (LASA) is largely supported by a grant from the Netherlands Ministry of Health, Welfare and Sports, Directorate of Long-Term Care.
Conflict of Interest
The authors declare that the research was conducted in the absence of any commercial or financial relationships that could be construed as a potential conflict of interest.
Publisher’s Note
All claims expressed in this article are solely those of the authors and do not necessarily represent those of their affiliated organizations, or those of the publisher, the editors and the reviewers. Any product that may be evaluated in this article, or claim that may be made by its manufacturer, is not guaranteed or endorsed by the publisher.
Acknowledgments
The following studies and consortia have contributed to this manuscript. Amsterdam Dementia Cohort (ADC): Research at the Alzheimer center Amsterdam is part of the neurodegeneration research program of Amsterdam Neuroscience. 100-plus Study: we are grateful for the collaborative efforts of all participating centenarians and their family members and/or relatives. Wiesje van der Flier holds the Pasman chair. Longitudinal Aging Study of Amsterdam (LASA): the authors are grateful to all LASA participants, the fieldwork team and all researchers for their ongoing commitment to the study.
Supplementary Material
The Supplementary Material for this article can be found online at: https://www.frontiersin.org/articles/10.3389/fgene.2021.748781/full#supplementary-material
References
Alzheimer's Association (2012). 2012 Alzheimer’s Disease Facts and Figures. Alzheimer’s Demen. 8, 131–168. doi:10.1016/j.jalz.2012.02.001
Astle, W. J., Elding, H., Jiang, T., Allen, D., Ruklisa, D., Mann, A. L., et al. (2016). The Allelic Landscape of Human Blood Cell Trait Variation and Links to Common Complex Disease. Cell 167, 1415–1429.e19. doi:10.1016/j.cell.2016.10.042
Bandres-Ciga, S., Ahmed, S., Sabir, M. S., Blauwendraat, C., Adarmes-Gómez, A. D., Bernal-Bernal, I., et al. (2019). The Genetic Architecture of Parkinson Disease in Spain: Characterizing Population-specific Risk, Differential Haplotype Structures, and Providing Etiologic Insight. Mov Disord. 34, 1851–1863. doi:10.1002/mds.27864
Bodis, G., Toth, V., and Schwarting, A. (2018). Role of Human Leukocyte Antigens (HLA) in Autoimmune Diseases. Rheumatol. Ther. 5, 5–20. doi:10.1007/s40744-018-0100-z
Bone, W. P., Siewert, K. M., Jha, A., Klarin, D., Damrauer, S. M., VA, Million, Veteran Program Chang, K. M., et al. (2021). Multi-trait Association Studies Discover Pleiotropic Loci between Alzheimer’s Disease and Cardiometabolic Traits. Alzheimers Res. Ther. 13, 34. doi:10.1186/s13195-021-00773-z
Caselli, G., Pozzi, L., Vaupel, J. W., Deiana, L., Pes, G., Carru, C., et al. (2006). Family Clustering in Sardinian Longevity: a Genealogical Approach. Exp. Gerontol. 41, 727–736. doi:10.1016/j.exger.2006.05.009
Christakoudi, S., Evangelou, E., Riboli, E., and Tsilidis, K. K. (2021). GWAS of Allometric Body-Shape Indices in UK Biobank Identifies Loci Suggesting Associations with Morphogenesis, Organogenesis, Adrenal Cell Renewal and Cancer. Sci. Rep. 11, 10688. doi:10.1038/s41598-021-89176-6
Cochran, J. N., Rush, T., Buckingham, S. C., and Roberson, E. D. (2015). The Alzheimer’s Disease Risk Factor CD2AP Maintains Blood–Brain Barrier Integrity. Hum. Mol. Genet. 24, 6667–6674. doi:10.1093/hmg/ddv371
Cole, B. S., Hall, M. A., Urbanowicz, R. J., Gilbert‐Diamond, D., and Moore, J. H. (2017). Analysis of Gene‐Gene Interactions. Curr. Protoc. Hum. Genet. 95, 1.14.1–1.14.10. doi:10.1002/cphg.45
Conti, D. V., Darst, B. F., Moss, L. C., Saunders, E. J., Sheng, X., Chou, A., et al. (2021). Trans-ancestry Genome-wide Association Meta-Analysis of Prostate Cancer Identifies New Susceptibility Loci and Informs Genetic Risk Prediction. Nat. Genet. 53, 65–75. doi:10.1038/s41588-020-00748-0
Corrada, M. M., Brookmeyer, R., Paganini-Hill, A., Berlau, D., and Kawas, C. H. (2010). Dementia Incidence Continues to Increase with Age in the Oldest Old: The 90+ Study. Ann. Neurol. 67, 114–121. doi:10.1002/ana.21915
Dato, S., Crocco, P., De Rango, F., Iannone, F., Maletta, R., Bruni, A. C., et al. (2021). IP6K3 and IPMK Variations in LOAD and Longevity: Evidence for a Multifaceted Signaling Network at the Crossroad between Neurodegeneration and Survival. Mech. Ageing Dev. 195, 111439. doi:10.1016/j.mad.2021.111439
Davies, G., Lam, M., Harris, S. E., Trampush, J. W., Luciano, M., Hill, W. D., et al. (2018). Study of 300,486 Individuals Identifies 148 Independent Genetic Loci Influencing General Cognitive Function. Nat. Commun. 9, 2098. doi:10.1038/s41467-018-04362-x
de Rojas, I., Moreno-Grau, S., Tesi, N., Grenier-Boley, B., Andrade, V., Jansen, I. E., et al. (2021). Common Variants in Alzheimer’s Disease and Risk Stratification by Polygenic Risk Scores. Nat. Commun. 12, 3417. doi:10.1038/s41467-021-22491-8
Deelen, J., Evans, D. S., Arking, D. E., Tesi, N., Nygaard, M., Liu, X., et al. (2019). A Meta-Analysis of Genome-wide Association Studies Identifies Multiple Longevity Genes. Nat. Commun. 10, 3669. doi:10.1038/s41467-019-11558-2
Efthymiou, A. G., and Goate, A. M. (2017). Late Onset Alzheimer’s Disease Genetics Implicates Microglial Pathways in Disease Risk. Mol. Neurodegener. 12, 43. doi:10.1186/s13024-017-0184-x
Gatz, M., Reynolds, C. A., Fratiglioni, L., Johansson, B., Mortimer, J. A., Berg, S., et al. (2006). Role of Genes and Environments for Explaining Alzheimer Disease. Arch. Gen. Psychiatry 63, 168–174. doi:10.1001/archpsyc.63.2.168
Hansen, D. V., Hanson, J. E., and Sheng, M. (2018). Microglia in Alzheimer’s Disease. J. Cel Biol. 217, 459–472. doi:10.1083/jcb.201709069
Harold, D., Abraham, R., Hollingworth, P., Sims, R., Gerrish, A., Hamshere, M. L., et al. (2009). Genome-wide Association Study Identifies Variants at CLU and PICALM Associated with Alzheimer’s Disease. Nat. Genet. 41, 1088–1093. doi:10.1038/ng.440
Herath, N. I., Doecke, J., Spanevello, M. D., Leggett, B. A., and Boyd, A. W. (2009). Epigenetic Silencing of EphA1 Expression in Colorectal Cancer Is Correlated with Poor Survival. Br. J. Cancer 100, 1095–1102. doi:10.1038/sj.bjc.6604970
Hoffmann, T. J., Ehret, G. B., Nandakumar, P., Ranatunga, D., Schaefer, C., Kwok, P-Y., et al. (2017). Genome-wide Association Analyses Using Electronic Health Records Identify New Loci Influencing Blood Pressure Variation. Nat. Genet. 49, 54–64. doi:10.1038/ng.3715
Hoffmann, T. J., Choquet, H., Yin, J., Banda, Y., Kvale, M. N., Glymour, M., et al. (2018). A Large Multiethnic Genome-wide Association Study of Adult Body Mass Index Identifies Novel Loci. Genetics 210, 499–515. doi:10.1534/genetics.118.301479
Holstege, H., Beker, N., Dijkstra, T., Pieterse, K., Wemmenhove, E., Schouten, K., et al. (2018). The 100-plus Study of Cognitively Healthy Centenarians: Rationale, Design and Cohort Description. Eur. J. Epidemiol. 33, 1229–1249. doi:10.1007/s10654-018-0451-3
Hoogendijk, E. O., Deeg, D. J. H., Poppelaars, J., van der Horst, M., Broese van Groenou, M. I., Comijs, H. C., et al. (2016). The Longitudinal Aging Study Amsterdam: Cohort Update 2016 and Major Findings. Eur. J. Epidemiol. 31, 927–945. doi:10.1007/s10654-016-0192-0
Huisman, M., Poppelaars, J., van der Horst, M., Beekman, A. T., Brug, J., van Tilburg, T. G., et al. (2011). Cohort Profile: The Longitudinal Aging Study Amsterdam. Int. J. Epidemiol. 40, 868–876. doi:10.1093/ije/dyq219
Jansen, I. E., Savage, J. E., Watanabe, K., Bryois, J., Williams, D. M., Steinberg, S., et al. (2019). Genome-wide Meta-Analysis Identifies New Loci and Functional Pathways Influencing Alzheimer’s Disease Risk. Nat. Genet. 51, 404–413. doi:10.1038/s41588-018-0311-9
Jinam, T. A. (2017). “Human Leukocyte Antigen (HLA) Region in Human Population Studies,” in Evolution of the Human Genome I. Editor N Saitou (Tokyo: Springer Japan), 173–179. doi:10.1007/978-4-431-56603-8_9
Jun, G., Ibrahim-Verbaas, C. A., Vronskaya, M., Lambert, J-C., Chung, J., Naj, A. C., et al. (2016). A Novel Alzheimer Disease Locus Located Near the Gene Encoding Tau Protein. Mol. Psychiatry 21, 108–117. doi:10.1038/mp.2015.23
Kichaev, G., Bhatia, G., Loh, P-R., Gazal, S., Burch, K., Freund, M. K., et al. (2019). Leveraging Polygenic Functional Enrichment to Improve GWAS Power. Am. J. Hum. Genet. 104, 65–75. doi:10.1016/j.ajhg.2018.11.008
Koyama, S., Ito, K., Terao, C., Akiyama, M., Horikoshi, M., Momozawa, Y., et al. (2020). Population-specific and Trans-ancestry Genome-wide Analyses Identify Distinct and Shared Genetic Risk Loci for Coronary Artery Disease. Nat. Genet. 52, 1169–1177. doi:10.1038/s41588-020-0705-3
Kunkle, B. W., Grenier-Boley, B., Sims, R., Bis, J. C., Damotte, V., Naj, A. C., et al. (2019). Genetic Meta-Analysis of Diagnosed Alzheimer’s Disease Identifies New Risk Loci and Implicates Aβ, Tau, Immunity and Lipid Processing. Nat. Genet. 51, 414–430. doi:10.1038/s41588-019-0358-2
Lambert, J-C., Heath, S., Even, G., Campion, D., Sleegers, K., Hiltunen, M., et al. (2009). Genome-wide Association Study Identifies Variants at CLU and CR1 Associated with Alzheimer’s Disease. Nat. Genet. 41, 1094–1099. doi:10.1038/ng.439
Langefeld, C. D., Ainsworth, H. C., Cunninghame Graham, D. S., Kelly, J. A., Comeau, M. E., Marion, M. C., et al. (2017). Transancestral Mapping and Genetic Load in Systemic Lupus Erythematosus. Nat. Commun. 8, 16021. doi:10.1038/ncomms16021
Liu, S., Liu, J., Weng, R., Gu, X., and Zhong, Z. (2019). Apolipoprotein E Gene Polymorphism and the Risk of Cardiovascular Disease and Type 2 Diabetes. BMC Cardiovasc. Disord. 19, 213. doi:10.1186/s12872-019-1194-0
Mackay, T. F., and Moore, J. H. (2014). Why Epistasis Is Important for Tackling Complex Human Disease Genetics. Genome Med. 6, 125. doi:10.1186/gm561
Mackay, T. F. C. (2014). Epistasis and Quantitative Traits: Using Model Organisms to Study Gene–Gene Interactions. Nat. Rev. Genet. 15, 22–33. doi:10.1038/nrg3627
McGrath, E. R., Beiser, A. S., DeCarli, C., Plourde, K. L., Vasan, R. S., Greenberg, S. M., et al. (2017). Blood Pressure from Mid- to Late Life and Risk of Incident Dementia. Neurology 89, 2447–2454. doi:10.1212/wnl.0000000000004741
Melzer, D., Pilling, L. C., and Ferrucci, L. (2019). The Genetics of Human Ageing. Nat. Rev. Genet. 21, 88–101. doi:10.1038/s41576-019-0183-6
Michailidou, K., Lindström, S., Dennis, J., Beesley, J., Hui, S., Kar, S., et al. (2017). Association Analysis Identifies 65 New Breast Cancer Risk Loci. Nature 551, 92–94. doi:10.1038/nature24284
Olafsdottir, T. A., Theodors, F., Bjarnadottir, K., Bjornsdottir, U. S., Agustsdottir, A. B., Stefansson, O. A., et al. (2020). Eighty-eight Variants Highlight the Role of T Cell Regulation and Airway Remodeling in Asthma Pathogenesis. Nat. Commun. 11, 393. doi:10.1038/s41467-019-14144-8
Partridge, L., Deelen, J., and Slagboom, P. E. (2018). Facing up to the Global Challenges of Ageing. Nature 561, 45–56. doi:10.1038/s41586-018-0457-8
Pattaro, C., Teumer, A., Gorski, M., Chu, A. Y., Li, M., Mijatovic, V., et al. (2016). Genetic Associations at 53 Loci Highlight Cell Types and Biological Pathways Relevant for Kidney Function. Nat. Commun. 7, 10023. doi:10.1038/ncomms10023
Pauls, S. D., and Marshall, A. J. (2017). Regulation of Immune Cell Signaling by SHIP1: A Phosphatase, Scaffold Protein, and Potential Therapeutic Target. Eur. J. Immunol. 47, 932–945. doi:10.1002/eji.201646795
Perls, T. T., Wilmoth, J., Levenson, R., Drinkwater, M., Cohen, M., Bogan, H., et al. (2002). Life-long Sustained Mortality Advantage of Siblings of Centenarians. Proc. Natl. Acad. Sci. USA 99, 8442–8447. doi:10.1073/pnas.122587599
Ponomareva, N., Andreeva, T., Protasova, M., Shagam, L., Malina, D., Goltsov, A., et al. (2013). Age-dependent Effect of Alzheimer’s Risk Variant of CLU on EEG Alpha Rhythm in Non-demented Adults. Front. Aging Neurosci. 5, 86. doi:10.3389/fnagi.2013.00086
Rademaker, M. C., de Lange, G. M., and Palmen, S. J. M. C. (2018). “The Netherlands Brain Bank for Psychiatry,” in Handbook of Clinical Neurology (Elsevier), 150, 3–16. doi:10.1016/b978-0-444-63639-3.00001-3
Rafiq, S., Khan, S., Tapper, W., Collins, A., Upstill-Goddard, R., Gerty, S., et al. (2014). A Genome Wide Meta-Analysis Study for Identification of Common Variation Associated with Breast Cancer Prognosis. PLoS ONE 9, e101488. doi:10.1371/journal.pone.0101488
Ryu, S. J., Cho, K. A., Oh, Y. S., and Park, S. C. (2006). Role of Src-specific Phosphorylation Site on Focal Adhesion Kinase for Senescence-Associated Apoptosis Resistance. Apoptosis 11, 303–313. doi:10.1007/s10495-006-3978-9
Sadighi Akha, A. A. (2018). Aging and the Immune System: An Overview. J. Immunol. Methods 463, 21–26. doi:10.1016/j.jim.2018.08.005
Shi, H., Belbin, O., Medway, C., Brown, K., Kalsheker, N., Carrasquillo, M., et al. (2012). Genetic Variants Influencing Human Aging from Late-Onset Alzheimer’s Disease (LOAD) Genome-wide Association Studies (GWAS). Neurobiol. Aging 33, 1849.e5-e18. doi:10.1016/j.neurobiolaging.2012.02.014
Sims, R., van der Lee, S. J., Naj, A. C., Bellenguez, C., Badarinarayan, N., Jakobsdottir, J., et al. (2017). Rare Coding Variants in PLCG2, ABI3, and TREM2 Implicate Microglial-Mediated Innate Immunity in Alzheimer’s Disease. Nat. Genet. 49, 1373–1384. doi:10.1038/ng.3916
Slot, R. E. R., Verfaillie, S. C. J., Overbeek, J. M., Timmers, T., Wesselman, L. M. P., Teunissen, C. E., et al. (2018). Subjective Cognitive Impairment Cohort (SCIENCe): Study Design and First Results. Alzheimer’s Res. Ther. 10, 76. doi:10.1186/s13195-018-0390-y
Solé-Domènech, S., Cruz, D. L., Capetillo-Zarate, E., and Maxfield, F. R. (2016). The Endocytic Pathway in Microglia during Health, Aging and Alzheimer’s Disease. Ageing Res. Rev. 32, 89–103. doi:10.1016/j.arr.2016.07.002
Surendran, P., Feofanova, E. V., Lahrouchi, N., Ntalla, I., Karthikeyan, S., Cook, J., et al. (2020). Discovery of Rare Variants Associated with Blood Pressure Regulation through Meta-Analysis of 1.3 Million Individuals. Nat. Genet. 52, 1314–1332. doi:10.1038/s41588-020-00713-x
Tesi, N., van der Lee, S. J., Hulsman, M., Jansen, I. E., Stringa, N., van Schoor, N., et al. (2019). Centenarian Controls Increase Variant Effect Sizes by an Average Twofold in an Extreme Case–Extreme Control Analysis of Alzheimer’s Disease. Eur. J. Hum. Genet. 27, 244–253. doi:10.1038/s41431-018-0273-5
Tesi, N., van der Lee, S. J., Hulsman, M., Jansen, I. E., Stringa, N., van Schoor, N. M., et al. (2020). Immune Response and Endocytosis Pathways Are Associated with the Resilience against Alzheimer’s Disease. Transl. Psychiatry 10, 332. doi:10.1038/s41398-020-01018-7
Tesi, N., van der Lee, S., Hulsman, M., Holstege, H., and Reinders, M. J. T. (2021). snpXplorer: a Web Application to Explore Human SNP-Associations and Annotate SNP-Sets. Nucleic Acids Res. 49, W603–W612. doi:10.1093/nar/gkab410
Timmers, P. R., Mounier, N., Lall, K., Fischer, K., Ning, Z., Feng, X., et al. (2019). Genomics of 1 Million Parent Lifespans Implicates Novel Pathways and Common Diseases and Distinguishes Survival Chances. ELife 8, e39856. doi:10.7554/eLife.39856
van der Flier, W. M., and Scheltens, P. (2018). Amsterdam Dementia Cohort: Performing Research to Optimize Care. J. Alzheimer’s Dis. 62, 1091–1111. doi:10.3233/jad-170850
Vujkovic, M., Keaton, J. M., Lynch, J. A., Miller, D. R., Zhou, J., Tcheandjieu, C., et al. (2020). Discovery of 318 New Risk Loci for Type 2 Diabetes and Related Vascular Outcomes Among 1.4 Million Participants in a Multi-Ancestry Meta-Analysis. Nat. Genet. 52, 680–691. doi:10.1038/s41588-020-0637-y
Wang, Y., and Colonna, M. (2014). Interkeukin-34, a Cytokine Crucial for the Differentiation and Maintenance of Tissue Resident Macrophages and Langerhans Cells: Highlights. Eur. J. Immunol. 44, 1575–1581. doi:10.1002/eji.201344365
Warren, H. R., Evangelou, E., Cabrera, C. P., Gao, H., Ren, M., Mifsud, B., et al. (2017). Genome-wide Association Analysis Identifies Novel Blood Pressure Loci and Offers Biological Insights into Cardiovascular Risk. Nat. Genet. 49, 403–415. doi:10.1038/ng.3768
Willemsen, G., de Geus, E. J. C., Bartels, M., van Beijsterveldt, C. E. M. T., Brooks, A. I., Estourgie-van Burk, G. F., et al. (2010). The Netherlands Twin Register Biobank: A Resource for Genetic Epidemiological Studies. Twin Res. Hum. Genet. 13, 231–245. doi:10.1375/twin.13.3.231
Keywords: cognitively healthy, centenarians, aging, alzheimer’s disease, effect on aging, protective variants
Citation: Tesi N, Hulsman M, van der Lee SJ, Jansen IE, Stringa N, van Schoor NM, Scheltens P, van der Flier WM, Huisman M, Reinders MJT and Holstege H (2021) The Effect of Alzheimer’s Disease-Associated Genetic Variants on Longevity. Front. Genet. 12:748781. doi: 10.3389/fgene.2021.748781
Received: 28 July 2021; Accepted: 24 November 2021;
Published: 21 December 2021.
Edited by:
Blanka Rogina, University of Connecticut Health Center, United StatesReviewed by:
Giuseppe Passarino, University of Calabria, ItalySrinivas Ayyadevara, Central Arkansas Veterans Healthcare System Eugene J. Towbin Healthcare Center, United States
Copyright © 2021 Tesi, Hulsman, van der Lee, Jansen, Stringa, van Schoor, Scheltens, van der Flier, Huisman, Reinders and Holstege. This is an open-access article distributed under the terms of the Creative Commons Attribution License (CC BY). The use, distribution or reproduction in other forums is permitted, provided the original author(s) and the copyright owner(s) are credited and that the original publication in this journal is cited, in accordance with accepted academic practice. No use, distribution or reproduction is permitted which does not comply with these terms.
*Correspondence: Henne Holstege, aC5ob2xzdGVnZUBhbXN0ZXJkYW11bWMubmw=