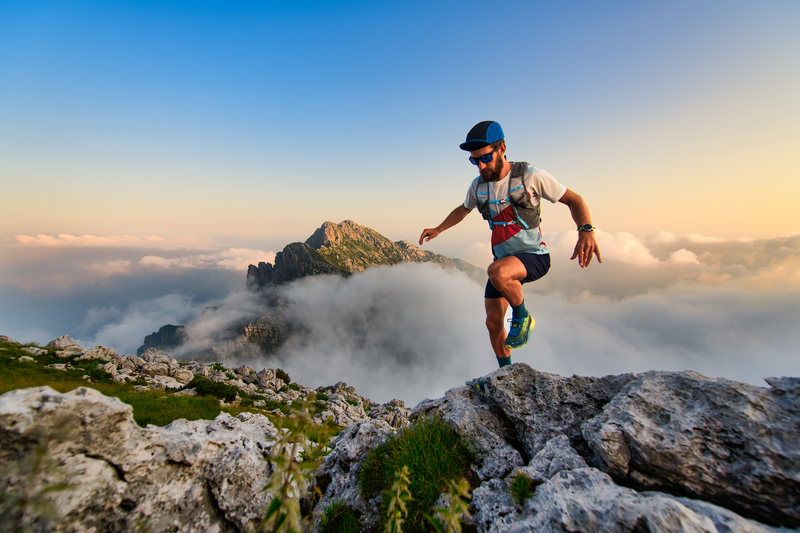
95% of researchers rate our articles as excellent or good
Learn more about the work of our research integrity team to safeguard the quality of each article we publish.
Find out more
ORIGINAL RESEARCH article
Front. Genet. , 21 October 2021
Sec. Statistical Genetics and Methodology
Volume 12 - 2021 | https://doi.org/10.3389/fgene.2021.745224
This article is part of the Research Topic Systems Genetics of Human Complex Diseases, Volume II View all 24 articles
Significant genetic association exists between rheumatoid arthritis (RA) and cardiovascular disease. The associated mechanisms include common inflammatory mediators, changes in lipoprotein composition and function, immune responses, etc. However, the causality of RA and vascular/heart problems remains unknown. Herein, we performed Mendelian randomization (MR) analysis using a large-scale RA genome-wide association study (GWAS) dataset (462,933 cases and 457,732 controls) and six cardio-cerebrovascular disease GWAS datasets, including age angina (461,880 cases and 447,052 controls), hypertension (461,880 cases and 337,653 controls), age heart attack (10,693 cases and 451,187 controls), abnormalities of heartbeat (461,880 cases and 361,194 controls), stroke (7,055 cases and 454,825 controls), and coronary heart disease (361,194 cases and 351,037 controls) from United Kingdom biobank. We further carried out heterogeneity and sensitivity analyses. We confirmed the causality of RA with age angina (OR = 1.17, 95% CI: 1.04–1.33, p = 1.07E−02), hypertension (OR = 1.45, 95% CI: 1.20–1.75, p = 9.64E−05), age heart attack (OR = 1.15, 95% CI: 1.05–1.26, p = 3.56E−03), abnormalities of heartbeat (OR = 1.07, 95% CI: 1.01–1.12, p = 1.49E−02), stroke (OR = 1.06, 95% CI: 1.01–1.12, p = 2.79E−02), and coronary heart disease (OR = 1.19, 95% CI: 1.01–1.39, p = 3.33E−02), contributing to the understanding of the overlapping genetic mechanisms and therapeutic approaches between RA and cardiovascular disease.
Cardiovascular disease remains the leading cause of human death, with an estimated 17.3 million people worldwide dying of cardiovascular disease each year, which is expected to increase to 23.6 million by 2030 (Laslett et al., 2012; Smith et al., 2012; Leong et al., 2017). Epidemiological studies have shown that the occurrence of cardiovascular disease is caused by various factors, with obesity, diabetes, smoking, hyperlipidemia, atherosclerosis, hypertension, and blood viscosity being its potential risk factor (Leong et al., 2017; Xu et al., 2019). Importantly, traditional cardiovascular disease risk factors account for a large proportion of rheumatoid arthritis (RA) (An et al., 2016). RA patients were 48% more likely to have cardiovascular disease than normal people and a 50% higher incidence of cardiovascular disease-related mortality (Avina-Zubieta et al., 2008, 2012; Sokka et al., 2008; England et al., 2018). However, most of the previous studies have examined the association between RA and atherosclerosis and congestive heart failure, ignoring other phenotypes of heart disease and vascular problems (England et al., 2018). Moreover, the exact causality is still unknown.
Mendelian randomization (MR) could estimate causality without bias, which has been used in previous studies to explore the association between phenotypes (Jansen et al., 2014; Smith et al., 2017; Hemani et al., 2018; Cheng et al., 2019b, 2021; Zhuang et al., 2019; Qiu et al., 2021). Causality between multiple metabolic characteristics, nutrient elements, and common diseases with cardiovascular disease have been demonstrated (Ference et al., 2017; Larsson et al., 2017, 2020; Yeung et al., 2018; Rosoff et al., 2020; Arvanitis et al., 2021). However, strong evidence linking RA to cardiovascular disease is still lacking. Herein, we mainly selected inverse-variance weighted (IVW), weighted median, and MR-Egger methods for MR analysis. We provided strong evidence that RA contributed to six vascular-/heart problem-related phenotypes, which could be of great significance for clinical disease prevention and treatment.
We obtained large-scale genome-wide association study (GWAS) summary datasets from the United Kingdom Biobank on RA and six cardiovascular disease phenotypes, and all of the participants were of European ancestry. From 2006 to 2010, the United Kingdom Biobank Assessment Center recruited 386,005 participants from the United Kingdom to participate in self-reporting of non-cancer illness (Li et al., 2015; Sun et al., 2019). RA GWAS (462,933 cases and 457,732 controls) was derived from the non-cancer illness study. At the same time, the United Kingdom Biobank Assessment Center carried out the study of vascular/heart problems diagnosed by doctors, covering 501,555 participants. These heart or vascular problems included age angina (461,880 cases and 447,052 controls), age high blood pressure (461,880 cases and 337,653 controls), age stroke (7,055 cases and 454,825 controls), and age heart attack (10,693 cases and 451,187 controls). In addition, we supplemented two other United Kingdom Biobank studies on coronary heart disease (CHD) (361,194 cases and 351,037 controls) and abnormalities of heartbeat (461,880 cases and 361,194 controls).
In order to enhance the statistical power of genetic variants, we deleted single-nucleotide polymorphisms (SNPs) with a minor allele frequency (MAF) < 1%. Moreover, we removed variants with physical distance less than 10,000 kb and R2 < 0.001 to avoid linkage disequilibrium (LD). For preprocessed exposure (RA) data, we selected genetic variants that passed genome-wide association threshold (p < 5E−08) as instrumental variables (IVs) to satisfy IV assumption 1 of MR analysis: variants should be strongly associated with exposure (RA) (Hemani et al., 2018).
In the absence of individual-level data, we used MR, a powerful statistical method, to infer the causality between two phenotypes. MR analysis eliminates the need to consider confounders and reverse causality. Two-sample MR requires that the samples of exposure and outcome be independent, which greatly expands the application range of MR (Hemani et al., 2018). Details of MR analysis have been described in previous reports (Davey Smith and Hemani, 2014; Bowden et al., 2015, 2016; Yavorska and Burgess, 2017; Cheng et al., 2018, 2019b; Hemani et al., 2018; Hu et al., 2020, 2021; Qiu et al., 2021). Herein, we first aligned alleles on the forward strand and harmonized SNP effects of exposure and outcome (Ong et al., 2021). If the variant in IVs was lacking in outcome, we allowed the proxy SNP with a strong LD to replace it (Hemani et al., 2018). Subsequently, we performed the inverse-variance weighted (IVW) estimator to estimate the association between RA and vascular/heart disease (Burgess and Thompson, 2017). In the case of certain invalid instruments or directional pleiotropy bias, the weighted median and MR-Egger estimators could help to make further judgment (Bowden et al., 2017; Burgess and Thompson, 2017; Hartwig et al., 2017; Cheng, 2019; Cheng et al., 2019a). Finally, we carried out reverse MR analysis to evaluate the evidence for reverse causal association.
We performed a series of sensitivity tests to ensure that our results were robust, including heterogeneity tests to assess heterogeneity between IVs, leave-one-out analysis (Wei et al., 2019, 2021; Govindaraj et al., 2020; Hasan et al., 2021) to assess whether a single SNP over-drove outcome, and funnel plots and MR-Egger to assess potential horizontal pleiotropy (Bowden et al., 2017; Hartwig et al., 2017; Rosoff et al., 2020). The statistical tests for MR analysis were undertaken using the R package of meta and TwoSampleMR (Hemani et al., 2018). The statistically significant association is defined as p < 0.05.
Eight genetic variants were used as IVs to evaluate the association between RA and cardiovascular disease (Table 1). Two SNPs needed to be proxied, but we lacked evidence for the presence of wrong effect alleles, strand issues, palindromic SNPs, and incompatible alleles. Due to the heterogeneity in some studies, we preferred to use the random-effects model (Table 2). By performing IVW analysis, we confirmed the causality of RA with age angina (OR = 1.17, 95% CI: 1.04–1.33, p = 1.07E−02), hypertension (OR = 1.45, 95% CI: 1.20–1.75, p = 9.64E–05), age heart attack (OR = 1.15, 95% CI: 1.05–1.26, p = 3.56E–03), abnormalities of heartbeat (OR = 1.07, 95% CI: 1.01–1.12, p = 1.49E−02), stroke (OR = 1.06, 95% CI: 1.01–1.12, p = 2.79E−02), and CHD (OR = 1.19, 95% CI: 1.01–1.39, p = 3.33E−02) (Figure 1). Detailed MR results are shown in the Supplementary Material. No evidence of reverse causality existed in any of the studies. Thus, RA made a significant contribution to common phenotypes associated with vascular or cardiac problems.
Figure 1. Mendelian randomization (MR) analysis between rheumatoid arthritis (RA) and six cardiovascular diseases. TE, treatment effects (β); se TE, standard error of treatment effect (se).
Unlike IVW, MR-Egger allows horizontal pleiotropy between IVs and exposure and outcome, and the weighted median allows a more powerful variant to have a greater impact on the overall result (Bowden et al., 2017; Hartwig et al., 2017; Qiu et al., 2021). Other MR calculation methods are a powerful supplement to IVW, especially when the IV assumptions of MR framework is not satisfied perfectly. In all methods, our results were robust, with small intercepts and high p-values in MR-Egger, which meant that horizontal pleiotropy made almost no effect on the results (Figure 2 and Table 2). According to funnel plots, rs7731626 and rs460568 in angina, and rs6679677 and rs9265076 in CHD, there existed a certain horizontal pleiotropy; however, little influence affected the overall results (Figure 3). Moreover, no single SNP over-drove the overall results.
Figure 2. MR tests of RA with angina, hypertension, heart attack, abnormalities of heartbeat, stroke, and coronary heart disease. The estimate of intercept can be interpreted as an estimate of the average pleiotropy of all single-nucleotide polymorphisms (SNPs), and the slope coefficient provides an estimate of the bias of the causal effect. (A) Angina. (B) Hypertension. (C) Heart attack. (D) Abnormalities of heartbeat. (E) Stroke. (F) Coronary heart disease.
Figure 3. Funnel plots of RA with angina, hypertension, heart attack, abnormalities of heartbeat, stroke, and coronary heart disease. The x-axis represents odds ratio (OR), and the y-axis represents standard error (se). (A) Angina. (B) Hypertension. (C) Heart attack. (D) Abnormalities of heartbeat. (E) Stroke. (F) Coronary heart disease.
In this study, we carried out MR analysis to demonstrate that RA was positively associated with six heart and vascular diseases. Observational studies evaluated that the patients with RA had a significantly increased risk of cardiovascular disease (An et al., 2016; Crowson et al., 2018). Crowson et al. (2018) followed up 5,638 RA patients for 5.8 years and found that about 30% of them eventually developed cardiovascular disease. RA might increase the risk of the six heart and vascular diseases we mentioned at the same time (Kitas and Gabriel, 2011; Dougados et al., 2014; Hadwen et al., 2021). Thus, we subdivided the phenotypes of cardiac and vascular diseases to provide different risk values, which might be a significant help for the clinical cotreatment of RA and cardiovascular disease.
Our study may have many advantages over previous observational studies. First, we used seven large-scale GWAS datasets. RA GWAS alone involved more than 900,000 participants. The seven studies were all from European descent, avoiding potential population stratification. Second, MR greatly avoids the influence of confounding factors and reverse causality because the alleles of the SNP site are randomly assigned much earlier than the occurrence of any potential confounding factors. Third, we applied a variety of MR methods to jointly verify the robustness of the results. When some instruments were unavailable or horizontal pleiotropy, an unbiased causal estimate could still be given.
However, certain limitations existed in our study. First, there may be overlap of some samples in RA GWAS and cardiovascular disease GWAS, which leads to weak instruments bias (Pierce and Burgess, 2013). In addition, some of the genetic variants have a certain degree of heterogeneity or horizontal pleiotropy, such as rs7731626 and rs9265076, in the analysis of RA and angina. For population stratification from gender, age, and ancestry, inappropriate proxy SNP may be the potential reasons for them.
In conclusion, we explored the causality between RA and six cardio-cerebrovascular diseases for the first time, and all the results showed the risk effect of RA. Eight variants as IVs may be the link between RA and cardiovascular disease. We expect to find out the genetic association between chronic diseases more deeply in the future.
The original contributions presented in the study are included in the article/Supplementary Material, further inquiries can be directed to the corresponding author/s.
SQ and LH designed the study, analyzed the data, and wrote the manuscript. ML and SJ revised the manuscript. YH supervised the research and revised the manuscript. All authors contributed to the article and approved the submitted version.
This work was supported by the National Key R&D Program of China (2017YFC1201201, 2018YFC0910504, and 2017YFC0907503), the Natural Science Foundation of China (61801147 and 82003553), and Heilongjiang Postdoctoral Science Foundation (LBH-Z6064), all to YH.
The authors declare that the research was conducted in the absence of any commercial or financial relationships that could be construed as a potential conflict of interest.
All claims expressed in this article are solely those of the authors and do not necessarily represent those of their affiliated organizations, or those of the publisher, the editors and the reviewers. Any product that may be evaluated in this article, or claim that may be made by its manufacturer, is not guaranteed or endorsed by the publisher.
We would like to thank the United Kingdom Biobank for the GWAS summary statistics (http://www.nealelab.is/uk-biobank/).
The Supplementary Material for this article can be found online at: https://www.frontiersin.org/articles/10.3389/fgene.2021.745224/full#supplementary-material
An, J., Alemao, E., Reynolds, K., Kawabata, H., Solomon, D. H., Liao, K. P., et al. (2016). Cardiovascular outcomes associated with lowering low-density lipoprotein cholesterol in rheumatoid arthritis and matched nonrheumatoid arthritis. J. Rheumatol. 43, 1989–1996. doi: 10.3899/jrheum.160110
Arvanitis, M., Qi, G. H., Bhatt, D. L., Post, W. S., Chatterjee, N., Battle, A., et al. (2021). Linear and nonlinear mendelian randomization analyses of the association between diastolic blood pressure and cardiovascular events the J-curve revisited. Circulation 143, 895–906. doi: 10.1161/circulationaha.120.049819
Avina-Zubieta, J. A., Choi, H. K., Sadatsafavi, M., Etminan, M., Esdaile, J. M., and Lacaille, D. (2008). Risk of cardiovascular mortality in patients with rheumatoid arthritis: a meta-analysis of observational studies. Arthritis Rheum. 59, 1690–1697.
Avina-Zubieta, J. A., Thomas, J., Sadatsafavi, M., Lehman, A. J., and Lacaille, D. (2012). Risk of incident cardiovascular events in patients with rheumatoid arthritis: a meta-analysis of observational studies. Ann. Rheum. Dis. 71, 1524–1529. doi: 10.1136/annrheumdis-2011-200726
Bowden, J., Del Greco, M. F., Minelli, C., Davey Smith, G., Sheehan, N., and Thompson, J. (2017). A framework for the investigation of pleiotropy in two-sample summary data mendelian randomization. Stat. Med. 36, 1783–1802. doi: 10.1002/sim.7221
Bowden, J., Smith, G. D., and Burgess, S. (2015). Mendelian randomization with invalid instruments: effect estimation and bias detection through Egger regression. Int. J. Epidemiol. 44, 512–525. doi: 10.1093/ije/dyv080
Bowden, J., Smith, G. D., Haycock, P. C., and Burgess, S. (2016). Consistent estimation in mendelian randomization with some invalid instruments using a weighted median estimator. Genet. Epidemiol. 40, 304–314. doi: 10.1002/gepi.21965
Burgess, S., and Thompson, S. G. (2017). Interpreting findings from mendelian randomization using the MR-Egger method. Eur. J. Epidemiol. 32, 377–389. doi: 10.1007/s10654-017-0255-x
Cheng, L. (2019). Computational and biological methods for gene therapy. Curr. Gene Ther. 19, 210–210. doi: 10.2174/156652321904191022113307
Cheng, L., Zhao, H., Wang, P., Zhou, W., Luo, M., Li, T., et al. (2019a). Computational methods for identifying similar diseases. Mol. Ther. Nucleic Acids 18, 590–604. doi: 10.1016/j.omtn.2019.09.019
Cheng, L., Zhu, Z., Wang, C., Wang, P., He, Y. O., and Zhang, X. (2021). COVID-19 induces lower levels of IL-8, IL-10, and MCP-1 than other acute CRS-inducing diseases. Proc. Natl. Acad. Sci. U. S. A. 118:e2102960118. doi: 10.1073/pnas.2102960118
Cheng, L., Zhuang, H., Ju, H., Yang, S., Han, J., Tan, R., et al. (2019b). Exposing the causal effect of body mass index on the risk of type 2 diabetes mellitus: a mendelian randomization study. Front. Genet. 10:94. doi: 10.3389/fgene.2019.00094
Cheng, L., Zhuang, H., Yang, S., Jiang, H., Wang, S., and Zhang, J. (2018). Exposing the causal effect of C-reactive protein on the risk of type 2 Diabetes mellitus: a mendelian randomization study. Front. Genet. 9:657. doi: 10.3389/fgene.2018.00657
Crowson, C. S., Rollefstad, S., Ikdahl, E., Kitas, G. D., Van Riel, P., Gabriel, S. E., et al. (2018). Impact of risk factors associated with cardiovascular outcomes in patients with rheumatoid arthritis. Ann. Rheum. Dis. 77, 48–54.
Davey Smith, G., and Hemani, G. (2014). Mendelian randomization: genetic anchors for causal inference in epidemiological studies. Hum. Mol. Genet. 23, R89–R98.
Dougados, M., Soubrier, M., Antunez, A., Balint, P., Balsa, A., Buch, M. H., et al. (2014). Prevalence of comorbidities in rheumatoid arthritis and evaluation of their monitoring: results of an international, cross-sectional study (COMORA). Ann. Rheum. Dis. 73, 62–68.
England, B. R., Thiele, G. M., Anderson, D. R., and Mikuls, T. R. (2018). Increased cardiovascular risk in rheumatoid arthritis: mechanisms and implications. BMJ 361, k1036. doi: 10.1136/bmj.k1036
Ference, B. A., Ginsberg, H. N., Graham, I., Ray, K. K., Packard, C. J., Bruckert, E., et al. (2017). Low-density lipoproteins cause atherosclerotic cardiovascular disease. 1. Evidence from genetic, epidemiologic, and clinical studies. A consensus statement from the European Atherosclerosis Society Consensus Panel. Eur. Heart J. 38, 2459–2472. doi: 10.1093/eurheartj/ehx144
Govindaraj, R. G., Subramaniyam, S., and Manavalan, B. (2020). Extremely-randomized-tree-based Prediction of N(6)-methyladenosine Sites in Saccharomyces cerevisiae. Curr. Genomics 21, 26–33. doi: 10.2174/1389202921666200219125625
Hadwen, B., Stranges, S., and Barra, L. (2021). Risk factors for hypertension in rheumatoid arthritis patients-a systematic review. Autoimmun. Rev. 20:102786. doi: 10.1016/j.autrev.2021.102786
Hartwig, F. P., Davey Smith, G., and Bowden, J. (2017). Robust inference in summary data mendelian randomization via the zero modal pleiotropy assumption. Int. J. Epidemiol. 46, 1985–1998. doi: 10.1093/ije/dyx102
Hasan, M. M., Shoombuatong, W., Kurata, H., and Manavalan, B. (2021). Critical evaluation of web-based DNA N6-methyladenine site prediction tools. Brief. Funct. Genomics 20, 258–272. doi: 10.1093/bfgp/elaa028
Hemani, G., Zheng, J., Elsworth, B., Wade, K. H., Haberland, V., Baird, D., et al. (2018). The MR-Base platform supports systematic causal inference across the human phenome. Elife 7:e34408.
Hu, Y., Sun, J. Y., Zhang, Y., Zhang, H., Gao, S., Wang, T., et al. (2021). rs1990622 variant associates with Alzheimer’s disease and regulates TMEM106B expression in human brain tissues. BMC Med. 19:11. doi: 10.1186/s12916-020-01883-5
Hu, Y., Zhang, H., Liu, B., Gao, S., Wang, T., Han, Z., et al. (2020). rs34331204 regulates TSPAN13 expression and contributes to Alzheimer’s disease with sex differences. Brain 143:e95. doi: 10.1093/brain/awaa302
Jansen, H., Samani, N. J., and Schunkert, H. (2014). Mendelian randomization studies in coronary artery disease. Eur. Heart J. 35, 1917–1924. doi: 10.1093/eurheartj/ehu208
Kitas, G. D., and Gabriel, S. E. (2011). Cardiovascular disease in rheumatoid arthritis: state of the art and future perspectives. Ann. Rheum. Dis. 70, 8–14. doi: 10.1136/ard.2010.142133
Larsson, S. C., Back, M., Rees, J. M. B., Mason, A. M., and Burgess, S. (2020). Body mass index and body composition in relation to 14 cardiovascular conditions in UK Biobank: a mendelian randomization study. Eur. Heart J. 41, 221–226. doi: 10.1093/eurheartj/ehz388
Larsson, S. C., Burgess, S., and Michaelsson, K. (2017). Association of genetic variants related to serum calcium levels with coronary artery disease and myocardial infarction. JAMA 318, 371–380. doi: 10.1001/jama.2017.8981
Laslett, L. J., Alagona, P., Clark, B. A., Drozda, J. P., Saldivar, F., Wilson, S. R., et al. (2012). The worldwide environment of cardiovascular disease: prevalence, diagnosis, therapy, and policy issues a report from the American College of Cardiology. J. Am. Coll. Cardiol. 60, S1–S49.
Leong, D. P., Joseph, P. G., Mckee, M., Anand, S. S., Teo, K. K., Schwalm, J. D., et al. (2017). Reducing the global burden of cardiovascular disease, part 2 Prevention and treatment of cardiovascular disease. Circ. Res. 121, 695–710. doi: 10.1161/circresaha.117.311849
Li, P., Guo, M., Wang, C., Liu, X., and Zou, Q. (2015). An overview of SNP interactions in genome-wide association studies. Brief. Funct. Genomics 14, 143–155. doi: 10.1093/bfgp/elu036
Ong, J. S., Dixon-Suen, S. C., Han, X., An, J. Esophageal Cancer Consortium, 23 and Me Research Team, et al. (2021). A comprehensive re-assessment of the association between vitamin D and cancer susceptibility using mendelian randomization. Nat. Commun. 12:246.
Pierce, B. L., and Burgess, S. (2013). Efficient design for mendelian randomization studies: subsample and 2-sample instrumental variable estimators. Am. J. Epidemiol. 178, 1177–1184. doi: 10.1093/aje/kwt084
Qiu, S., Cao, P., Guo, Y., Lu, H., and Hu, Y. (2021). Exploring the causality between hypothyroidism and non-alcoholic fatty liver: a mendelian randomization study. Front. Cell Dev. Biol. 9:643582. doi: 10.3389/fcell.2021.643582
Rosoff, D. B., Smith, G. D., Mehta, N., Clarke, T. K., and Lohoff, F. W. (2020). Evaluating the relationship between alcohol consumption, tobacco use, and cardiovascular disease: a multivariable mendelian randomization study. PLoS Med. 17:e1003410. doi: 10.1371/journal.pmed.1003410
Smith, G. D., Paternoster, L., and Relton, C. (2017). When will mendelian randomization become relevant for clinical practice and public health? JAMA 317, 589–591. doi: 10.1001/jama.2016.21189
Smith, S. C., Collins, A., Ferrari, R., Holmes, D. R., Logstrup, S., Mcghie, D. V., et al. (2012). Our time: a call to save preventable death from cardiovascular disease (heart disease and stroke). Circulation 126, 2769–2775. doi: 10.1161/cir.0b013e318267e99f
Sokka, T., Abelson, B., and Pincus, T. (2008). Mortality in rheumatoid arthritis: 2008 update. Clin. Exp. Rheumatol. 26, S35–S61.
Sun, L., Liu, G., Su, L., and Wang, R. (2019). HS-MMGKG: a fast multi-objective harmony search algorithm for two-locus model detection in GWAS. Curr. Bioinform. 14, 749–761. doi: 10.2174/1574893614666190409110843
Wei, L., He, W., Malik, A., Su, R., Cui, L., and Manavalan, B. (2021). Computational prediction and interpretation of cell-specific replication origin sites from multiple eukaryotes by exploiting stacking framework. Brief. Bioinform. 22:bbaa275.
Wei, L., Su, R., Luan, S., Liao, Z., Manavalan, B., Zou, Q., et al. (2019). Iterative feature representations improve N4-methylcytosine site prediction. Bioinformatics 35, 4930–4937. doi: 10.1093/bioinformatics/btz408
Xu, L., Huang, J., Zhang, Z., Qiu, J., Guo, Y., Zhao, H., et al. (2019). Bioinformatics study on serum triglyceride levels for analysis of a potential risk factor affecting blood pressure variability. Curr. Bioinform. 14, 376–385. doi: 10.2174/1574893614666190109152809
Yavorska, O. O., and Burgess, S. (2017). MendelianRandomization: an R package for performing mendelian randomization analyses using summarized data. Int. J. Epidemiol. 46, 1734–1739. doi: 10.1093/ije/dyx034
Yeung, S. L. A., Luo, S., and Schooling, C. M. (2018). The impact of glycated hemoglobin (HbA(1c)) on Cardiovascular disease risk: a mendelian randomization study using UK Biobank. Diabetes Care 41, 1991–1997. doi: 10.2337/dc18-0289
Keywords: Mendelian randomization, genome-wide association studies, rheumatoid arthritis, cardiovascular disease, inverse-variance weighted
Citation: Qiu S, Li M, Jin S, Lu H and Hu Y (2021) Rheumatoid Arthritis and Cardio-Cerebrovascular Disease: A Mendelian Randomization Study. Front. Genet. 12:745224. doi: 10.3389/fgene.2021.745224
Received: 21 July 2021; Accepted: 20 August 2021;
Published: 21 October 2021.
Edited by:
Liangcai Zhang, Janssen Research and Development, United StatesReviewed by:
Quan Zou, University of Electronic Science and Technology of China, ChinaCopyright © 2021 Qiu, Li, Jin, Lu and Hu. This is an open-access article distributed under the terms of the Creative Commons Attribution License (CC BY). The use, distribution or reproduction in other forums is permitted, provided the original author(s) and the copyright owner(s) are credited and that the original publication in this journal is cited, in accordance with accepted academic practice. No use, distribution or reproduction is permitted which does not comply with these terms.
*Correspondence: Yang Hu, aHV5YW5nQGhpdC5lZHUuY24=
†These authors share first authorship
Disclaimer: All claims expressed in this article are solely those of the authors and do not necessarily represent those of their affiliated organizations, or those of the publisher, the editors and the reviewers. Any product that may be evaluated in this article or claim that may be made by its manufacturer is not guaranteed or endorsed by the publisher.
Research integrity at Frontiers
Learn more about the work of our research integrity team to safeguard the quality of each article we publish.