- 1Department of Laboratory Medicine, Karolinska Institutet, Karolinska University, Hospital Huddinge, Stockholm, Sweden
- 2Department Clinical Translation Research, Singapore General Hospital, Singapore, Singapore
- 3RED OMNI Human Genetics, Genentech, South San Francisco, CA, United States
- 4Biomedical Research Center (CINBIO) Singular Research Center, University of Vigo, Vigo, Spain
- 5Maze Therapeutics, South San Francisco, CA, United States
- 6Department of Biosciences and Nutrition, Karolinska Institutet, Huddinge, Sweden
- 7BGI-Shenzhen, Shenzhen, China
Immunoglobulin A Deficiency (IgAD) is a polygenic primary immune deficiency, with a strong genetic association to the human leukocyte antigen (HLA) region. Previous genome-wide association studies (GWAS) have identified five non-HLA risk loci (IFIH1, PVT1, ATG13-AMBRA1, AHI1 and CLEC16A). In this study, we investigated the genetic interactions between different HLA susceptibility haplotypes and non-MHC genes in IgAD. To do this, we stratified IgAD subjects and healthy controls based on HLA haplotypes (N = 10,993), and then performed GWAS to identify novel genetic regions contributing to IgAD susceptibility. After replicating previously published HLA risk haplotypes, we compared individuals carrying at least one HLA risk allele (HLA-B*08:01-DRB1*03:01-DQB1*02:01 or HLA-DRB1*07:01-DQB1*02:02 or HLA-DRB1*01-DQB1*05:01) with individuals lacking an HLA risk allele. Subsequently, we stratified subjects based on the susceptibility alleles/haplotypes and performed gene-based association analysis using 572,856 SNPs and 24,125 genes. A significant genome-wide association in STXBP6 (rs4097492; p = 7.63 × 10−9) was observed in the cohort carrying at least one MHC risk allele. We also identified a significant gene-based association for B3GNT6 (PGene = 2.1 × 10–6) in patients not carrying known HLA susceptibility alleles. Our findings indicate that the etiology of IgAD differs depending on the genetic background of HLA susceptibility haplotypes.
Introduction
Immunoglobulin A deficiency (IgAD) is the most common primary immune deficiency (Yazdani et al., 2017), defined as serum levels of IgA ≤ 0.07 g/L in individuals >4 years of age who have normal serum levels of other immunoglobulins (Conley et al., 1999; Picard et al., 2015). The clinical presentation of IgAD is heterogeneous, ranging from asymptomatic blood donors to patients suffering from recurrent respiratory and gastrointestinal infections (Wang et al., 2011; Jorgensen et al., 2013). IgAD exhibits strong familial aggregation typical of a complex polygenic trait, and the strongest genetic associations have been reported in the human major histocompatibility complex (MHC) region (Ambrus et al., 1977; Cobain et al., 1983; Hammarström and Smith, 1983; MacHulla et al., 2000). The prevalence of autoimmunity, which are also strongly associated with the MHC, is strikingly higher in individuals with IgAD (Wang et al., 2011). Like autoimmune diseases, IgAD is most common in individuals of European ancestry. Thus far, two genome-wide association studies (GWAS) and one human leukocyte antigen (HLA) fine-mapping study of IgAD risk have interrogated the genetic causes of IgAD (Dostal et al., 2007; Ferreira et al., 2010; Ferreira et al., 2012; Bronson et al., 2016). However, the genetic etiology of IgAD still remains unclear.
The HLA-B*08-DRB1*03:01-DQB1*02 haplotype is the strongest genetic risk factor for IgAD in N. European populations (Olerup et al., 1990; Ferreira et al., 2012) (combined p = 3.37 × 10–43; OR = 3.33). There are two additional HLA haplotypes associated with IgAD risk: HLA-B*44-DRB1*07:01-DQB1*02 and HLA-B*14-DRB1*01:02-DQB1*05 (Olerup et al., 1990; Ferreira et al., 2012). In addition to strong linkage to the HLA region, IgAD is associated with variants in the IFIH1, PVT1, ATG13-AMBRA1, AHI1 and CLEC16A gene regions (Ferreira et al., 2010; Bronson et al., 2016). Multiple studies indicated a pleiotropic effect of IFIH1 in modulating autoimmunity, and the IFIH1 gene region has been implicated in susceptibility to celiac disease (CD), systemic lupus erythematosus (SLE) and type 1 diabetes (T1D) (Ferreira et al., 2010; Diogo et al., 2018). Interestingly, variants in other autoimmunity genes associated with IgAD (CLEC16A, ATG13, and AHI1) are also associated with multiple sclerosis (MS) (van Luijn et al., 2015; Graves et al., 2018), though evidence for shared causal variants between IgAD and MS in these regions has not been reported.
In this study, we investigated the genetic interactions between different HLA susceptibility haplotypes and non-MHC genes in IgAD. To do this, we stratified IgAD subjects based on HLA haplotypes, and then performed genome-wide association studies (GWAS) to identify novel genetic regions contributing to IgAD susceptibility.
Materials and Methods
Sample Collection
In total, 806 anonymized IgAD patients and 10,187 controls were genotyped (N = 10,993), including 767 Swedish IgAD cases, 485 healthy Swedish controls collected from a previous study (Ferreira et al., 2012), and 9,741 Swedish twin samples (monozygotic (MZ): 4063, one per family; dizygotic (DZ): 5678, two per family) (Frankowiack et al., 2015), 39 of whom were identified with IgAD (serum IgA< 0.07 g/L). Ethical approval was obtained from the Regional ethical review board in Stockholm.
Genotyping and Filtering
Genotyping was performed using arrays developed by Illumina, Inc. (San Diego, CA, United States). IgAD cases were genotyped on Omni1-Quad and Omni2.5 by Genentech Inc (South San Francisco, CA, United States) and the Mutation Analysis Core Facility at the Karolinska University Hospital (Stockholm, Sweden). Controls were genotyped on Omni1-Quad (Ferreira et al., 2010), except for the twin gene controls (Magnusson et al., 2013) which were genotyped on the OmniExpress. SNPs were mapped to genome build hg19 coordinates using liftOver. In addition, strand, alleles and positions were updated according to strand data mapped to hg19 (Rayner, 2011). Prior to merging datasets, we used the Genotype Harmonizer (Deelen et al., 2014) to align the format and the strands for all arrays (reference: 1000 Genomes Project Phase 3 integrated variant set). In addition, variants with evidence of deviation from Hardy-Weinberg equilibrium in the controls (p < 1 × 10−6) and a genotyping rate < 97% were removed.
Imputation and Verification of HLA Alleles
We used a haplotype graph model to impute four-digit HLA alleles (HLA*IMP:02) (Dilthey et al., 2013), using a European reference panel and absolute posterior probability (Q2) ≥ 0.7 as a cut-off for HLA-B, HLA-DRB1 and HLA-DQB1. We validated our HLA imputation by comparing it to four-digit HLA types [PCR-SSP, sequence-based typing (BST)] in 150 IgAD cases, as well as 25 healthy controls. In addition, we had two-digit HLA types for 617 cases. After verification, 636 cases and 7,798 controls with high-confidence four-digit HLA alleles were included in the analyses.
Sub-Classification of Population Cohort and Association Analysis
We initiated the analysis by comparing individuals carrying at least one HLA risk haplotype (i.e., HLA-B*08:01-DRB1*03:01-DQB1*02:01 or HLA-DRB1*07:01-DQB1*02:02 or HLA-DRB1*01-DQB1*05:01) with individuals lacking a risk haplotype. The significantly associated variants in the sample cohort were then verified using the control cohort. Only unique variants in the cases were considered as having an association with the IgAD. We next applied the same strategy to study and verify the signal by using the cohort carrying at least one HLA-B*08:01-DRB1*03:01-DQB1*02:01 risk haplotype, the most numerous cohort (54% of total IgAD individuals). The analysis was first performed by comparing all individuals without HLA-B*08:01-DRB1*03:01-DQB1*02:01, followed by a comparison with individuals lacking all risk haplotypes.
For further analysis, cohorts homozygous for HLA-B*08:01-DRB1*03:01-DQB1*02:01 (68 IgAD and 123 controls), HLA-DRB1*07:01-DQB1*02:02 (7 IgAD and 30 controls) and HLA-DRB1*01-DQB1*05:01 (34 IgAD and 68 controls) were selected. Additionally, we also investigated cohorts homozygous for single alleles, i.e. HLA-B*08:01, HLA-DRB1*03:01, HLA-DRB1*07:01 and HLA-DQB1*05:01.
Chi square tests of association on genotypes for each cohort were performed independently, using only variants that overlapped between the arrays used to genotype the cohort. Variants reaching genome-wide significance (p < 5 × 10−8) were considered significantly associated with IgAD. In addition, variants with p < 2 × 10−7, and FDR ≤ 0.05 were considered to show a suggestive significant association with IgAD. In addtion, the locus zoom plots (Pruim et al., 2010) were visually inspected to confirm that the association signal is consistent with the LD pattern of the SNPs in the region. Furthermore, visualization of the linkage effect for several multi-allelic HLA types was analyzed using the Disentangler software (Kumasaka et al., 2010; Okada et al., 2011a).
Gene-Based Association Analysis in Different Subgroups
GCTA-fastBAT analysis was performed to investigate gene-based associations (Bakshi et al., 2016). The method performs a set-based association analysis for human complex traits using summary-level data from genome-wide association studies (GWAS) and linkage disequilibrium (LD) data from a reference sample with individual-level genotypes. In total, 24,125 genes (including 1,522 miRNA genes) (hg19) were included in the analysis. Genes in the MHC region (chr6:25300000–33800000) were excluded due to strong LD in the region. The gene region was defined as + 50 kb from both 3′ and 5′ UTR of the genes. The LD cut off was set at 0.9. Assuming independence of the gene-level tests, non-MHC genes that had at least five SNPs in the region and PGene < 2.10 × 10−6 were considered significant. However, this threshold is conservative since there is overlap between genes, so 2.1 × 10−6 < PGene < 2.10 × 10−4 was also reported and considered to be suggestive of association.
LD Proxy Analysis of Associated Variants
LD proxy analysis was performed using LDlink (Machiela and Chanock, 2015) (EUR; r2 > 0.9 and D' > 0.9).
Polygenic Risk Score Pathway Set Analysis
PRS pathway based analysis was performed using PRsice/PRSet (Choi and O'Reilly, 2019) to explore the differences of associated pathways in the cohort carrying at least one MHC susceptibility allele as compared to individuals lacking a risk haplotype. A total of 4,762 pathways genes sets from the Molecular Signatures Database (MSigDB) (Subramanian et al., 2005; Liberzon et al., 2015) were included in the analysis. The pathway sets that had at least 15 SNPs in a gene set and had a total p < 5 × 10−6 were considered significant. The prevalence of IgAD in Sweden (1: 600) was used to adjust the R2 (variance explained).
Results
HLA Association Analysis and Multiple Haplotype Interaction Investigation
8,434 samples passed QC and were included in the analysis (636 IgAD and 7,798 controls). In the single haplotype analysis, the HLA-B*08:01-DRB1*03:01-DQB1*02:01 haplotype showed the strongest association with IgAD (OR = 3.59, P+++ = 3.17 × 10–82), while HLA-DRB1*07:01-DRB1*02:02 (OR = 1.84, P++ = 1.19 × 10–9) and HLA-DRB1*01:01- DQB1*05:01 (OR = 1.41, P++ = 1.31 × 10–4) showed weaker associations (Table 1). The HLA-DRB1*01:02 DQB1*05:01 effect was not possible to calculate due to the low frequency in controls (F < 0.01). Hence, a combined signal of HLA-DRB1*01-DQB1*05:01 (a combination of HLA-DRB1*01:01 and HLA-DRB1*01:02) was investigated and a strong association signal was detected (OR = 1.84, P++ = 3.90 × 10–14). However, the presence of HLA-B*08:01 (OR = 1.32, P+-- = 1.17 × 10–1) or HLA-DRB1*03:01-DQB1*02:01 (OR = 1.32, p = 7.24 × 10–2) alone was not associated with IgAD susceptibility. Similarly, the presence of HLA-DRB1*07:01 (OR = 1.06, P PPA = 7.16 × 10–1) was not associated with IgAD (Table 1). HLA-DQB1*02:02 is in complete LD with HLA-DRB1*07:01 and thus, we did not identify any case that allowed us to investigate the effect of HLA-DQB1*02:02 without the presence of HLA-DRB1*07:01. Similarly, the number of cases and controls was too low to determine the effect of HLA-DRB1*01 or HLA-DQB1*05:01 alone.
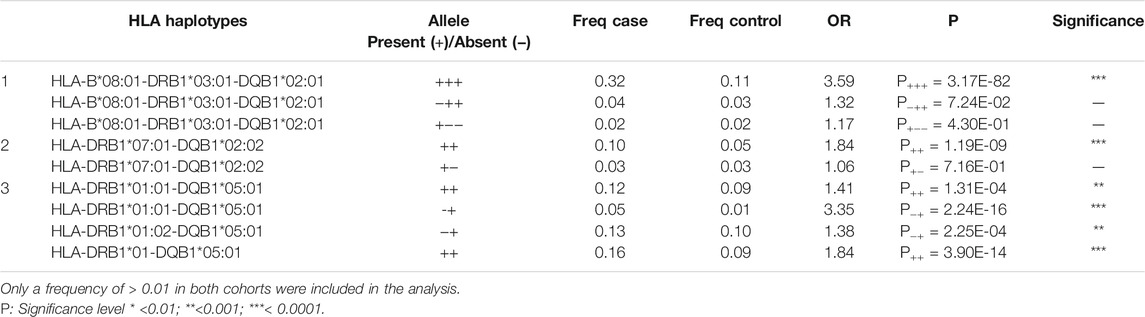
TABLE 1. HLA haplotype association analysis for high-risk susceptibility alleles in the IgAD cohort.
As shown in Figure 1A, in the IgAD patients, HLA-DQB1*02:01 and HLA-DRB1*03:01 are in perfect LD. HLA-DQB1*02:02 and HLA-DRB1*07:01 are also in perfect LD. HLA-DRB1*07:01 occurred with HLA-DQB1*02:02 in 74.7% of the analyzed individuals and with HLA-DQB1*03:03 in 24.7% of individuals (Figure 1B). 72% of the HLA-DRB1*01:01 alleles and 22.8% of the HLA-DRB1*01:02 alleles occurred with HLA-DQB1*05:01, whereas the remaining 5.2% mainly occur with HLA-DRB1*01:03 and DRB1*10:01 (Figure 1C).
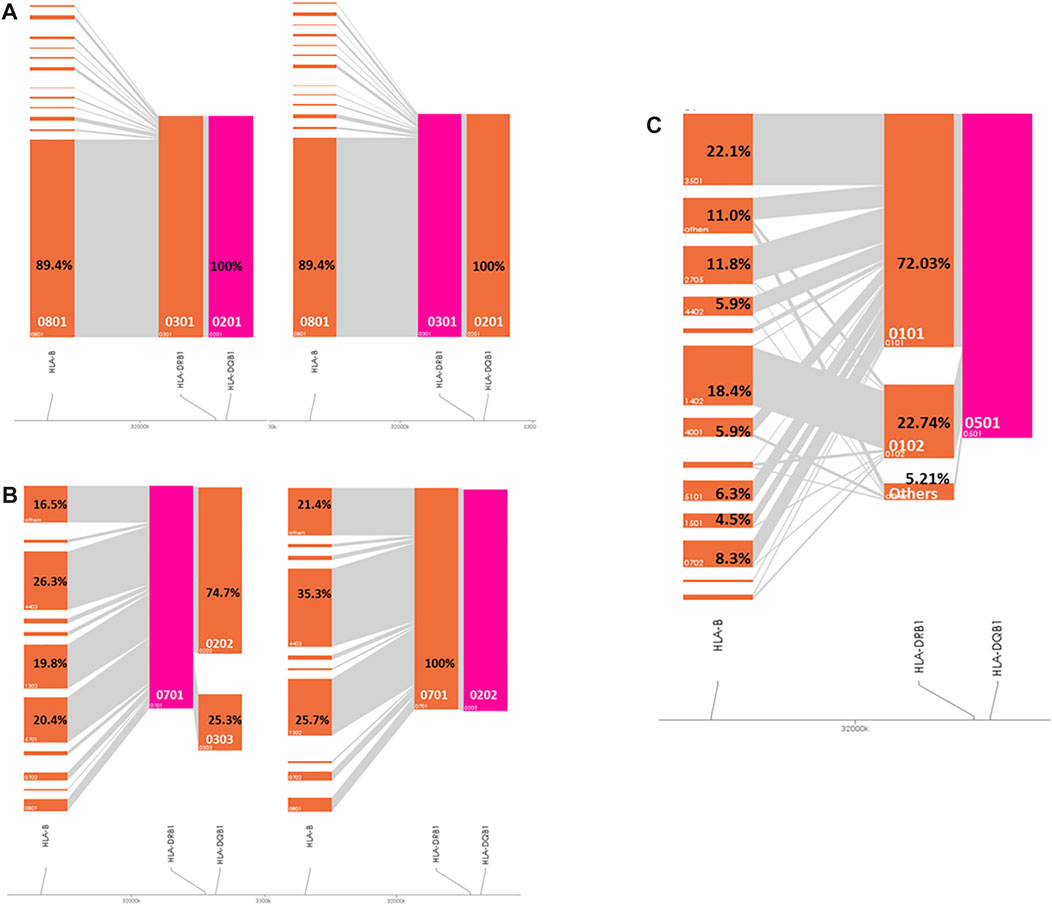
FIGURE 1. Visualization of the HLA haplotype structure in IgAD patients. Pink color marks the investigated haplotype. (A) HLA-B*08:01-DRB1*03:01-DRB1*02:01 cohort; (B) HLA-DRB1*07:01-DQB1*02:02 cohort; (C) HLA-DRB1*01-DQB1*05:01 cohort.
Analysis of the Influence of Non-MHC Variants in IgAD Patients Homozygous for High-Risk HLA Allele
Based on the above haplotype and linkage analyses, we subdivided the patients into HLA susceptibility groups to investigate non-MHC gene interactions with the known susceptibility haplotypes.
We first analyzed the difference between individuals carrying at least one HLA risk allele with individuals lacking any risk allele. Based on the cross-comparison strategy (Supplementary Figure S1), we identified one significantly associated non-MHC variant, rs4097492 (OR = 0.23, p = 7.63 × 10–9), an intronic variant of the STXBP6 gene on chromosome 14 (Figure 2A). Next, we compared individuals carrying at least one HLA-B*08:01-DRB1*03:01-DQB1*02:01 haplotype to individuals who lack the HLA-B*08:01-DRB1*03:01-DQB1*02:01 haplotype (but including individuals carrying another risk haplotypes). There was no significant association.
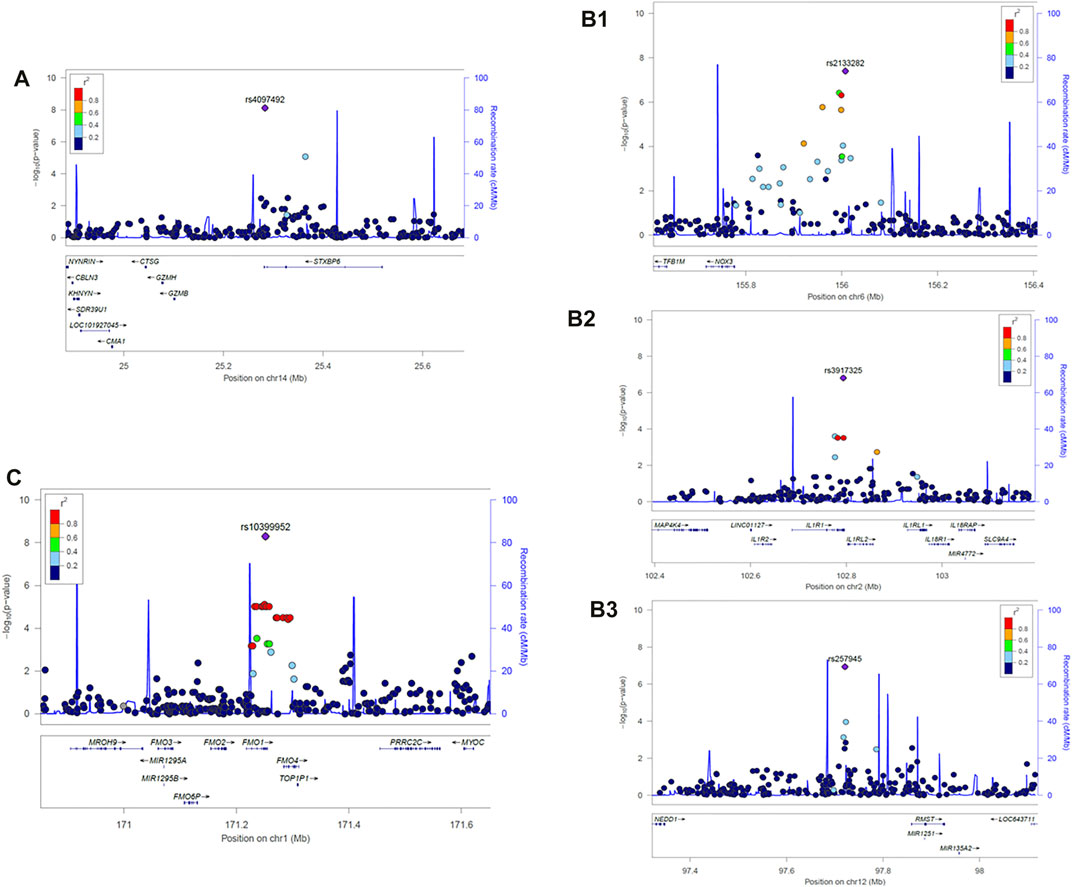
FIGURE 2. LocusZoom plot of the associated loci in IgAD patients carrying respective MHC susceptibility haplotypes. (A) Carrying at least one MHC risk haplotype (HLA-B*08:01-DRB1*03:01-DQB1*02:01 or HLA-DRB1*07:01-DQB1*02:02 or HLA-DRB1*01-DQB1*05:01), rs4097492, Chr14:25283341 (B) HLA-DRB1*07:01-DQB1*02:02 homozygous cohort, (B1): rs2133282, Chr6:156007516, (B2): rs3917325, Chr2: 102793907, (B3): rs257945, Chr12:97720902; (C) HLA-DRB1*01-DQB1*05:01 homozygous cohort, rs10399952, Chr1:171251663. The associated locus (top SNP) is represented by purple circle in each LocusZoom plot. All the other SNPs are colored based on their correlation (r2) with the labeled top SNP. The recombination rates estimated from 1,000 Genomes (EUR) data are shown in solid blue line. Genes are marked below by horizontal blue lines and the arrows on the horizontal blue lines show the direction of transcription. Gene designations and physical positions and are based on the Genome Reference Consortium Human Build 37, GRCh37.
In the HLA-DRB1*07:01-DQB1*02:02 homozygous cohorts, one significant (<5 × 10–8) and two strongly suggestive variants (<2 × 10–7; FDR ≤ 0.05) were identified (Figure 2B1). The peak variant was rs2133282 (OR = 33, p = 3.97 × 10–8; FDR = 0.02), an intergenic variant located between the NADPH oxidase NOX3 and the tumor suppressor ARID1B on chromosome 6. The two novel strong suggestive variants were rs3917325 (OR = 59, p = 1.57 × 10–7; FDR< 0.05), an UTR3 variant of IL1R1 on chromosome 2 and rs257945 (OR = 38.67, p = 1.14 × 10–7; FDR = 0.05), an intergenic variant located between NEDD1 and RMST on chromosome 12. For the HLA-DRB1*07:01 single allele homozygous cohort, a UTR3 variant of the Interleukin Receptor 1 (IL1R1) on chromosome 2 (rs3917325) was significantly associated (OR = 21.83, p = 3.55 × 10–8; FDR = 0.01) (Figure 2B2).
For the HLA-DRB1*01-DQB1*05:01 homozygous individuals, one significant marker, rs10399952 (OR = 15.4, p = 5.05 × 10−9), a variant of microsomal flavin-containing monooxygenase 1(FMO1) on chromosome 1, was detected (Figure 2C). We did not detect any strong signal (<5 × 10–8 or < 2 × 10–7) in the HLA-B*08:01-DRB1*03:01-DQB1*02:01 homozygous cohort based on this analysis method (Supplementary Figure S2D), nor in the single allele homozygous cohorts carrying HLA-B*08:01, DRB1*03:01 or DQB1*05:01 (Supplementary Figure S2E–G).
Analysis of Influence Genes Using Gene-Based Analysis in Different Subgroups
The effect sizes of individual genetic variants are usually small because of the polygenic nature of human complex traits and diseases which limits the statistical power to detect them. Emerging evidence has suggested that diseases or traits associated variants identified in genome-wide association studies (GWAS) tend to be located in gene-rich regions (Yang et al., 2011; Schork et al., 2013). SNPs in and around genes have been shown to explain more phenotypic variation (Yang et al., 2011) and tend to have enriched replicable associations at higher rates (Smith et al., 2011) than intergenic SNPs. In addition, multiple associated variants at a single locus are generally observed (Wood et al., 2014). Therefore, for the investigation of complex trait genes, it is more powerful to test the aggregated effect of a set of SNPs within a gene region.
To enhance our detection power, we analyzed the data using a gene-based association analysis. As HLA is co-dominantly expressed, only patients homozygous for the susceptibility HLA haplotypes were included in the analysis. The HLA-DRB1*07:01 HLA-DQB1*02:02 cohort was excluded due to the small number of cases (<20). In addition, we also tested patient cohorts carrying no HLA susceptibility alleles.
With the enhanced method, CD40 (p = 6.9 × 10–5), with a total of 29 SNPs in the analyzed region, was found to be suggestively associated with IgAD patients homozygous for the HLA-B*08:01-DRB1*03:01-DQB1*02:01 haplotype. DHX38 (p = 8.6 × 10–5) and 14 SNPs in a region containing a novel inhibitor of protein phosphatase 4 (Han et al., 2015), showed suggestive evidence for association in patients homozygous for HLA-DRB1*01-DQB1*05:01.
In patients who do not carry any of the major HLA susceptibility haplotypes, we identified a significant association with B3GNT6 (p = 2.1 × 10–6), which encodes an important precursor in the biosynthesis of mucin-type glycoproteins (Supplementary Table S1). In addition, another region containing six genes showed suggestive evidence for association with IgAD. Many of the genes in this region have been associated with autoimmune diseases or DNA repair (Supplementary Table S1). The locations of all the identified genes from two different methods are shown in Figure 3 according to the HLA susceptibility groups.
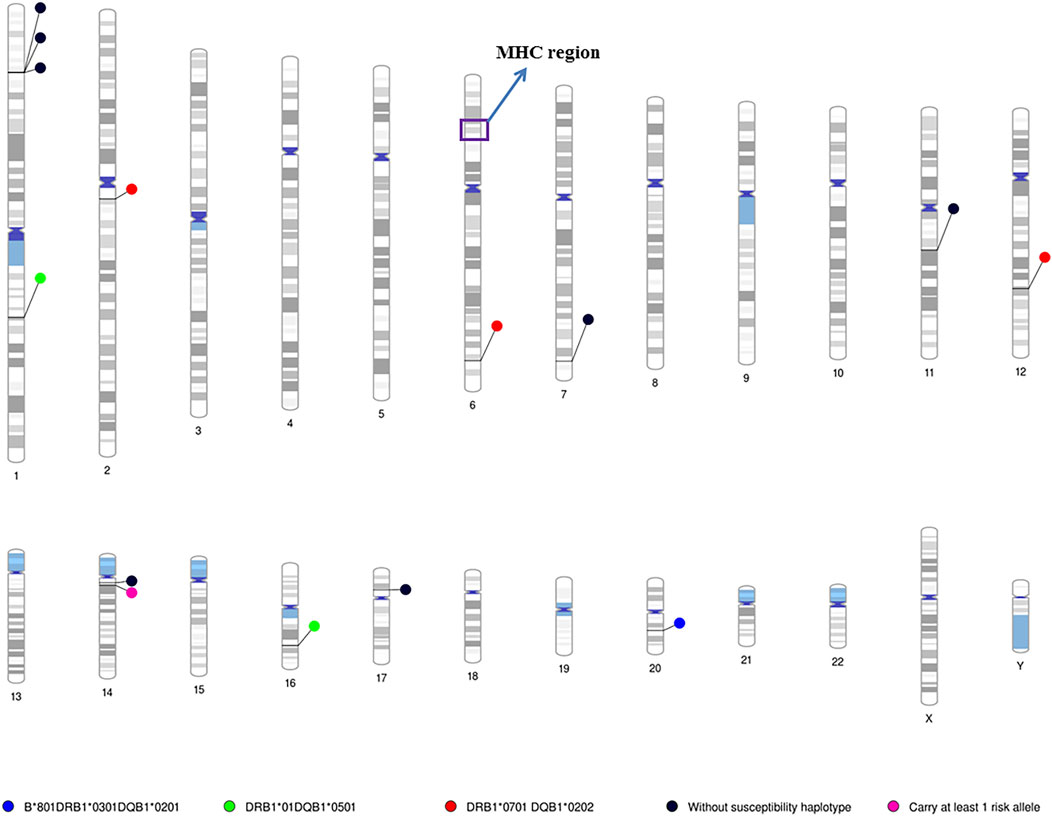
FIGURE 3. Chromosome ideograms for all identified susceptibility genes/loci in the patients with different HLA risk alleles. Chromosome ideograms were generated using the Phenogram software (Wolfe et al., 2013). Blue circle: location of genes/locus associated with the HLA-B*08:01-DRB1*03:01-DQB1*02:01 homozygous cohort; Green circle: location of genes/locus associated with the HLA-DRB1*01-DQB1*05:01 homozygous cohorts; Red circle: location of genes/locus associated with the HLA-DRB1*07:01-DQB1*02:02 homozygous cohorts; Black circle: location of genes/locus associated with patients do not carry any MHC susceptibility haplotye. Pink circle: location of genes/locus associated with patients carrying at least one MHC susceptibility genes. The MHC region is highlighted in purple box. Coloured regions indicated the cytogenetic band on each chromosome according to the predefined setting of the Phenogram, which is based on ideogram documented in the UCSC database (Furey and Haussler, 2003).
Linkage Disequilibrium Proxy Analysis
Ldlink analysis was performed to explore proxy and putatively functional variants within the nearby genetic region that correlated with the associated variants. Rs4097492 proxies are clustered close to the STXBP6 genes (Supplementary Figure S4A). Supplementary Figure S4B shows that rs213382 proxies are clustering near the NOX3 gene. Based on the results, rs213382 is not linked with the nearby mir1202 gene. On the other hand, rs3917325 proxies cluster around the IL1R1 and IL1RL2 gene regions (Supplementary Figure S4C). Similarly, rs10399952 proxies cluster close to the FMO1 and FMO4 genes (Supplementary Figure S4E); additionally, rs10399952 is an eQTL of FMO4 (Ex Portal (2021).Ex, 2021). On the other hand, rs257945 proxies are close to the RMST gene but are not in strong LD with the nearby mir1251 and mir135A2 microRNAs (Supplementary Figure S4D).
Polygenic Risk Score Pathway Set Analysis
PRSet pathway/gene set based analysis was performed to explore the pathway association in each cohort carrying MHC risk allele using the individuals lacking all risk haplotypes as a reference. The analysis results suggested that individuals carrying HLA-B*08:01-DRB1*03:01-DQB1*02:01 risk haplotypes were enriched for association with various immune conditions e.g., allergy, asthma, autoimmunity (e.g., SLE, T1D) (Figure 4A). Two significant pathway sets, i.e. asthma and intestinal immune network for IgA production, were detected in the cohorts carrying the HLA-DRB1*01-DQB1*05:01 risk haplotypes (Figure 4B). However, no significant pathway sets (p < 2 × 10−6) were identified in the cohort carrying HLA-DRB1*07:01-DQB1*02:02.
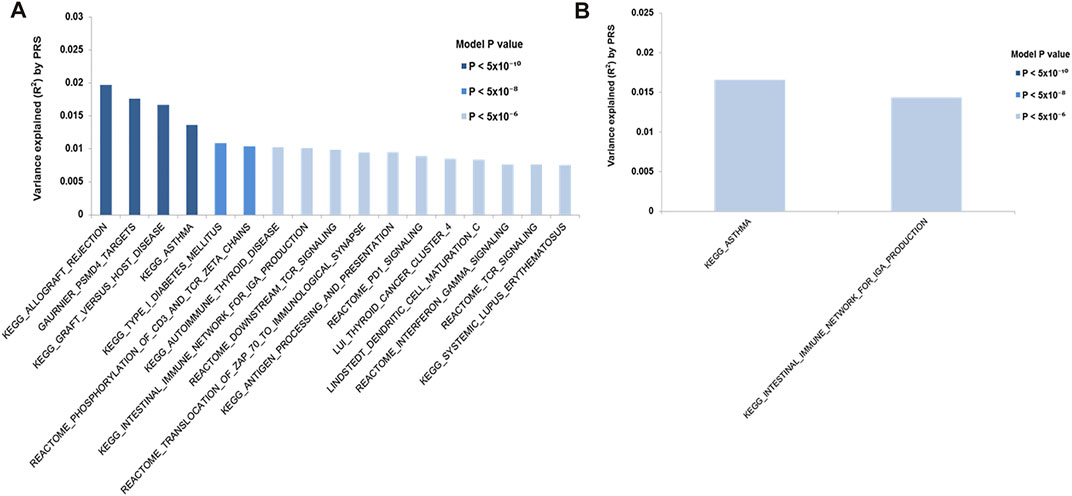
FIGURE 4. PRS (Polygenic risk score) pathways/gene sets analysis. PRS pathway/gene set analysis for IgAD cohort carrying different MHC risk haplotypes. Only significant pathway/genes set (p < 2 × 10−6) are shown. The y-axis indicates R (Conley et al., 1999), a measure of the variance explained. On the x-axis showing the significant pathways/gene sets. The color of the bar indicate the p value threshold. (A) HLA-B*08:01-DRB1*03:01-DRB1*02:01 cohort; (B) HLA-DRB1*01-DQB1*05:01
Discussion
MHC risk haplotypes for IgAD are neither fully penetrant nor required for disease, however, it is not yet known whether biological interaction between an MHC susceptibility allele and a non-MHC susceptibility allele contributes to disease onset.
HLA was first described as a risk locus for IgAD through the association with classical HLA class I and class II alleles (Ambrus et al., 1977; Cobain et al., 1983; Hammarström and Smith, 1983), and the extended HLA-A*01-B*08-DRB1*03-DQB1*02 haplotype has been identified as the strongest genetic risk factor for IgAD in N. European populations (Olerup et al., 1990). In addition, significant associations with two other haplotypes, DRB1*01:02-DQB1*05:01 and DRB1*07:01-DQB1*02, have been reported (Olerup et al., 1990; Ferreira et al., 2012). Nonetheless, the causal HLA risk allele(s) have not yet been identified.
Interaction between an HLA allele and a non-MHC genetic variant has been suggested in several autoimmune diseases, including T1D (Bjørnvold et al., 2006; Steck et al., 2006; Smyth et al., 2008), SLE (Bjørnvold et al., 2006), Graves Disease (GD) (Hodge et al., 2006; Kula et al., 2006; Jacobson et al., 2008; Takahashi and Kimura, 2010) and Myasthenia gravis (MG) (Varade et al., 2017). However, to date, it has not been described in IgAD. In this study, potential HLA/non-MHC interactions were investigated. In addition, we also assessed the previously identified association signal in autoimmune diseases and IgAD according to HLA risk alleles (Supplementary Table S1). In total, 14 novel genes/loci (4 significant and 10 suggestive) were identified in patients carrying different HLA susceptibility haplotypes (Figure 3). The majority have been implicated in immune function and autoimmune diseases. However, none of them have been reported to modify risk of HLA alleles in autoimmune diseases.
Our results show that in patients who carry at least one HLA risk haplotype, a common genetic variation in an intronic region of Syntaxin Binding Protein 6 (STXBP6) was significantly associated with protection against IgAD. This finding suggests that individuals who carry at least one risk haplotype and who do not carry the protective STXBP6 allele have a higher risk of developing disease. The signal remained significant when we compared individuals carrying at least one HLA-B*08:01-DRB1*03:01-DQB1*02:01 haplotype to individuals lacking a risk allele. However, when we performed a comparison of individuals carrying at least one HLA-B*08:01-DRB1*03:01-DQB1*02:01 allele with individuals lacking HLA-B*08:01-DRB1*03:01-DQB1*02:01 only (individuals carrying another risk haplotype were included), the signal was weaker (p = 7.00 × 10−4). The observation may be due to the lack of an added effect from the other two risk haplotypes. When we compared individual carrying at least one HLA-DRB1*07:01-DQB1*02:02 or HLA-DRB1*01-DQB1*05:01 haplotype with individuals lacking a risk allele, there was no genome-wide signal (p < 5.00 × 10–8) and the signal at rs4097492 was weaker (OR = 0.31, p = 5.13 × 10–5) due to the small cohorts of the other two risk haplotypes. This observation suggests that it is a modest association signal for individual carrying HLA-DRB1*07:01-DQB1*02:02 or HLA-DRB1*01-DQB1*05:01 risk haplotype.
STXBP6, a gene associated with white blood cell counts (Kichaev et al., 2019; Okada et al., 2011b), contains a phosphatidylinositol 4,5-bisphosphate (PIP2) binding domain (http://www.ebi.ac.uk/interpro/entry/ InterPro/ IPR028258/) and has been reported to play a role in regulating soluble N-ethylmaleimide-sensitive factor attachment protein receptor (SNARE) complex formation (Scales et al., 2002). PIP2 is in the PI3K signaling pathway, and rare mutations in PIK3R1 (involved in the phosphorylation of PIP2 to PIP3) are associated with an immune deficiency that includes lack of IgA production. (Walsh and Fruman, 2014). In the STRING database (Jensen et al., 2009), STXBP6 is associated with SNARE proteins, including STX4, SNAP25, STXBP5 (Supplementary Figure S3). One of these SNARE proteins, STX4, appears to play an essential role in the secretion of antibodies by human plasma cells (Gómez-Jaramillo et al., 2014). In addition, the expression of STXBP6 (http://www.proteinatlas.org) (Uhlén et al., 2015) is enriched in gamma delta (γδ) T cells (Blood Atlas. Blood Atlas, 2020). γδ T cells express a unique T-cell receptor (TCR) composed of one γ-chain as well as one δ-chain. γδ T cells are involved in the initiation of immune responses. Generally found in low frequency in the body, γδ T cells are most abundant at mucosal surfaces such as the gut, skin, and lungs. It is thus possible STXBP6 may be indirectly implicated in IgA secretion.
For the patients homozygous for HLA-B*08:01-DRB1*03:01-DQB1*02:01, CD40 showed suggestive evidence of association with IgAD. CD40 is a transmembrane receptor which belongs to the TNF receptor superfamily and is expressed on B cells, monocytes and dendritic cells (Paulie et al., 1985). It is a crucial player in both innate and adaptive immune responses and involved in the regulation of humoral immunity and cytokine production. Decreased expression of CD40 on monocytes of children with IgAD has previously been observed (Kowalczyk et al., 2006) and CD40 has also been implicated in the etiology of a variety of immune diseases such as RA, asthma, T1D and MS (Park et al., 2007; Australia and New Zealand, 2009; van der Linden et al., 2009).
The DEAH-box helicase 38 (DHX38) gene and rs10399952, a variant in FMO1gene was associated with the development of IgAD in patients homozygous for HLA-DRB1*01-DQB1*05:01. rs10399952 is an eQTL of FMO4 (Ex Portal (2021).Ex, 2021), FMO4 is one of the significantly differentially expressed genes identified in the galactose-deficient IgA inducing mesangial cells (Liu et al., 2017). DHX38 is an RNA helicase, involved in the alteration of RNA secondary structure such as translation initiation as well as ribosome and spliceosome assembly (Schwer and Guthrie, 1991; Wang and Guthrie, 1998; Hegele et al., 2012). Recent studies have suggested that it is a novel inhibitor of protein phosphatase 4 (PP4) (Han et al., 2015). As PP4 is essential for the germinal center formation and class switch recombination in mice (Chen et al., 2014), suggesting that DHX38 may be involved in the development of IgAD in the HLA-DRB1*01-DQB1*05:01 subgroup.
We identified a UTR variant, rs3917325, (MAF: 0.038) in IL1R1 associated with IgAD in the HLA-DRB1*07:01-DQB1*02:02 cohort. Interestingly, LD pair testing, using the EUR population in the 1000G genome cohort, shows that the variant is in linkage with rs10490571 (D’:0.95, R2:0.03), a locus reported as being associated with Immunoglobulin A nephropathy (IgAN) (Xie et al., 2017), a disease which is linked to overproduction of IgA. This observation suggests that IL1R1 may potentially play a role in IgA production.
The cohorts lacking any HLA susceptibility alleles comprise 18.6% (118 out of 636) of the total number of patients. From gene-based association analysis in IgAD patients who do not carry the HLA risk haplotypes, we identified a significant association with B3GNT6, a precursor in the biosynthesis of mucin-type glycoproteins. B3GNT6 has previously been reported to be associated with inflammatory colitis (An et al., 2007).
The clinical presentation of IgAD varies, ranging from asymptomatic “healthy” blood donors to symptomatic patients, supporting our observation of heterogeneity in the non-MHC association in individuals with IgAD depending on the HLA risk haplotypes. Further research is warranted to replicate our results, which may open up interesting perspectives for future research.
We further tested the differences between the stratified cohort using the PRS pathway/gene set analysis. The result show that, as compared to the cohorts lacking any susceptibility alleles, cohorts carrying the HLA-B*08:01-DRB1*03:01-DQB1*02:01 risk haplotype had a strong association with immune pathways including interferon-gamma signalling, TCR signalling, PD1 signaling, antigen processing and presentation as well as various immune diseases such as asthma, SLE and T1D. As IgAD has been suggested to be associated with risk for these autoimmune disorders (Wang et al., 2011) and asthma (Urm et al., 2013), our observation suggest a potentially shared common genetic regulatory pathway in the cohorts carrying the HLA-B*08:01-DRB1*03:01-DQB1*02:01 risk haplotype. Similarly, cohorts carrying the HLA-DRB1*01-DQB1*05:01 risk haplotype may potentially have a shared genetic/pathway with asthma and intestinal immune diseases.
IFIH1 has been found to be associated with the development of IgAD in previous studies (Bronson et al., 2016; Ferreira et al., 2010). However, we did not detect an association signal in either the cohorts carrying homozygous MHC risk haplotypes, nor in those who do not carry any MHC risk haplotype. Since a compound heterozygous MHC risk haplotype effect has been described in SLE and RA (Shimane et al., 2013; Graham et al., 2007; Agrawal et al., 1995), further investigation in larger patient groups, including cohorts carrying compound heterozygous MHC risk alleles together with all homozygous cohorts was performed. A modest association signal in the IFIH1 gene (PGene = 2.15 × 10–4) (Supplementary Table S2) was observed using this strategy, suggesting that the IFIH1 association is relatively minor and potentially associated with IgAD patients carrying two MHC risk haplotypes. We also investigated the genes previously implicated in the susceptibility to IgAD, including AHI1, ATG13-AMBRA1, CLEC16A, mir-6891 and PVT1 (Bronson et al., 2016; Chitnis et al., 2017). However, they did not pass the suggested significance threshold (p < 2.1 × 10−4).
The limitations of our study include a modest sample size for the disease cohort. The restriction has prevented us to split the data into discovery set and replication set. Hence, an independent replication cohort with similar power cannot be performed using the current collected samples. However, a random disease sub-cohort test has shown that the identified peak SNP, rs4097492 is the only point that has at least a suggestive significant p-value in all ten random samples tests (p = 1.72 × 10−7 - p = 9.60 × 10−10 for 400 random IgAD samples and p = 1.05 × 10−7 - p = 1.22 × 10−10 for 500 random IgAD samples). Additionally, a 108 permutations test show that the peak SNP, rs 4097492 has an empirical p-value of 8 × 10−8 (Supplementary Table S3). Furthermore, functional network analysis suggests that the STXBP6 may be indirectly implicated in IgA secretion. All the approaches have reassured us of the robustness of our findings. Nonetheless, further research with well-powered independent cohort is warranted to replicate our results. In addition, further increase in the sample size may help unravel more modest association signals.
In summary, we have identified multiple new susceptibility genes/variants for IgAD and shown that the pathogenesis of IgAD may differ depending on the presence of selected HLA susceptibility haplotypes. This may be potentially due to the interaction of non-MHC genes with the selected HLA susceptibility haplotypes. Further work is required to validate the novel associations and investigation of the regulatory role of associated variants through functional studies, including studies on the protein-protein interaction of HLA and non-MHC genes. Understanding the interaction/epistatic interaction between HLA and non-MHC genes may ultimately help us better understand the etiology of IgAD.
Data Availability Statement
The data is deposited in the European Variation Archive (EVA), the project accession number is PRJEB49292.
Ethics Statement
The studies involving human participants were reviewed and approved by the Ethics committee of the Karolinska Institutet. Written informed consent was obtained from the participants in accordance with the principles of the ethics committee of the Karolinska Institutet.
Author Contributions
CL interpreted the data, analyzed the results, drafted and edited the manuscript. PB provided critical feedback, aided in interpreting the results and edited the manuscript. JV analyzed the HLA results and edited the manuscript. TB provided critical feedback and key elements in data analysis, reviewed and edited the manuscript. LH provided guidance, supervised the findings of this work and edited the manuscript. All authors read and approved the final manuscript.
Funding
This work was supported by the Swedish Research Council and funds from the Karolinska Institutet.
Conflict of Interest
PB was employed by RED OMNI Human Genetics, Genentech; TB was employed by Maze Therapeutics. The remaining authors declare that the research was conducted in the absence of any commercial or financial relationships that could be construed as a potential conflict of interest.
The remaining authors declare that the research was conducted in the absence of any commercial or financial relationships that could be construed as a potential conflict of interest.
The handling editor declared a past co-authorship with one of the authors (LH).
Acknowledgments
We are sincerely grateful to all IgAD patients and controls who participated in this study. This study was supported by the Swedish Research Council, the Swedish Heart-Lung Foundation and the Talent Development Fund, Singhealth Foundation (CL).
Supplementary Material
The Supplementary Material for this article can be found online at: https://www.frontiersin.org/articles/10.3389/fgene.2021.736235/full#supplementary-material
References
Agrawal, S., Aggarwal, A., Dabadghao, S., Nak, S., and Misra, R. (1995). Compound Heterozygosity of HLA-DR4 and DR1 Antigens in Asian Indians Increases the Risk of Extra-articular Features in Rheumatoid Arthritis. Rheumatology 34, 41–44. doi:10.1093/rheumatology/34.1.41
Ambrus, M., Hernádi, E., and Bajtai, G. (1977). Prevalence of HLA-A1 and HLA-B8 Antigens in Selective IgA Deficiency. Clinical Immunol. Immunopathology 7, 311–314. doi:10.1016/0090-1229(77)90062-9
An, G., Wei, B., Xia, B., McDaniel, J. M., Ju, T., Cummings, R. D., et al. (2007). Increased Susceptibility to Colitis and Colorectal Tumors in Mice Lacking Core 3-derived O-Glycans. J. Exp. Med. 204, 1417–1429. doi:10.1084/jem.20061929
Australia and New Zealand Multiple Sclerosis Genetics Consortium (ANZgene) (2009). Genome-wide Association Study Identifies New Multiple Sclerosis Susceptibility Loci on Chromosomes 12 and 20. Nat. Genet. 41, 824–828. doi:10.1038/ng.396
Bakshi, A., Zhu, Z., Vinkhuyzen, A. A. E., Hill, W. D., McRae, A. F., Visscher, P. M., et al. (2016). Fast Set-Based Association Analysis Using Summary Data from GWAS Identifies Novel Gene Loci for Human Complex Traits. Sci. Rep. 6, 32894. doi:10.1038/srep32894
Bjørnvold, M., Amundsen, S. S., Stene, L. C., Joner, G., Dahl-Jørgensen, K., Njølstad, P. R., et al. (2006). FOXP3 Polymorphisms in Type 1 Diabetes and Coeliac Disease. J. Autoimmun. 27, 140–144.
Blood Atlas. Blood Atlas - STXBP6 - the Human Protein Atlas, Human Protein Atlas, www.proteinatlas.org/ENSG00000168952-STXBP6/blood/t-cells#hpa_gdt-cell. 2020.
Bronson, P. G., Chang, D., Bhangale, T., Seldin, M. F., Ortmann, W., Ferreira, R. C., et al. (2016). Common Variants at PVT1, ATG13-AMBRA1, AHI1 and CLEC16A Are Associated with Selective IgA Deficiency. Nat. Genet. 48, 1425–1429. doi:10.1038/ng.3675
Chen, M. Y., Chen, Y. P., Wu, M. S., Yu, G. Y., Lin, W. J., Tan, T. H., et al. (2014). PP4 Is Essential for Germinal Center Formation and Class Switch Recombination in Mice. Plos One 9. doi:10.1371/journal.pone.0107505
Chitnis, N., Clark, P. M., Kamoun, M., Stolle, C., Brad Johnson, F., and Monos, D. S. (2017). An Expanded Role for HLA Genes: HLA-B Encodes a microRNA that Regulates IgA and Other Immune Response Transcripts. Front. Immunol. 8, 583. doi:10.3389/fimmu.2017.00583
Choi, S. W., and O'Reilly, P. F. (2019). PRSice-2: Polygenic Risk Score Software for Biobank-Scale Data. Gigascience 8, giz082. doi:10.1093/gigascience/giz082
Cobain, T. J., French, M. A., Christiansen, F. T., and Dawkins, R. L. (1983). Association of IgA Deficiency with HLA A28 and B14. Tissue Antigens 22, 151–154. doi:10.1111/j.1399-0039.1983.tb01181.x
Conley, M. E., Notarangelo, L. D., and Etzioni, A. (1999). Diagnostic Criteria for Primary Immunodeficiencies. Clinical Immunol. 93, 190–197. doi:10.1006/clim.1999.4799
Deelen, P., Bonder, M., van der Velde, K., Westra, H.-J., Winder, E., Hendriksen, D., et al. (2014). Genotype Harmonizer: Automatic Strand Alignment and Format Conversion for Genotype Data Integration. BMC Res. Notes 7, 901. doi:10.1186/1756-0500-7-901
Dilthey, A., Leslie, S., Moutsianas, L., Shen, J., Cox, C., Nelson, M. R., et al. (2013). Multi-population Classical HLA Type Imputation. Plos Comput. Biol. 9, e1002877. doi:10.1371/journal.pcbi.1002877
Diogo, D., Tian, C., Franklin, C. S., Alanne-Kinnunen, M., March, M., Spencer, C. C. A., et al. (2018). Phenome-wide Association Studies across Large Population Cohorts Support Drug Target Validation. Nat. Commun. 9, 4285. doi:10.1038/s41467-018-06540-3
Dostal, A., Linnankivi, T., Somer, M., Kähkönen, M., Litzman, J., and Tienari, P. (2007). Mapping susceptibility gene locus for IgA deficiency at del(18)(q22.3?q23); report of familial cryptic chromosome t(18q; 10p) translocations. Int. J. Immunogenet. 34, 143–147. doi:10.1111/j.1744-313x.2007.00652.x
Ferreira, R. C., Pan-Hammarström, Q., Graham, R. R., Fontán, G., Lee, A. T., Ortmann, W., et al. (2012). High-density SNP Mapping of the HLA Region Identifies Multiple Independent Susceptibility Loci Associated with Selective IgA Deficiency. Plos Genet. 8, e1002476. doi:10.1371/journal.pgen.1002476
Ferreira, R. C., Pan-Hammarström, Q., Graham, R. R., Gateva, V., Fontán, G., Lee, A. T., et al. (2010). Association of IFIH1 and Other Autoimmunity Risk Alleles with Selective IgA Deficiency. Nat. Genet. 42, 777–780. doi:10.1038/ng.644
Frankowiack, M., Kovanen, R.-M., Repasky, G. A., Lim, C. K., Song, C., Pedersen, N. L., et al. (2015). The Higher Frequency of IgA Deficiency Among Swedish Twins Is Not Explained by HLA Haplotypes. Genes Immun. 16, 199–205. doi:10.1038/gene.2014.78
Furey, T. S., and Haussler, D. (2003). Integration of the Cytogenetic Map with the Draft Human Genome Sequence. Hum. Mol. Genet. 12, 1037–1044. doi:10.1093/hmg/ddg113
Gómez-Jaramillo, L., Delgado-Pérez, L., Reales, E., Mora-López, F., Mateos, R. M., García-Poley, A., et al. (2014). Syntaxin-4 Is Implicated in the Secretion of Antibodies by Human Plasma Cells. J. Leukoc. Biol. 95, 305–312. doi:10.1189/jlb.0113031
Graham, R. R., Ortmann, W., Rodine, P., Espe, K., Langefeld, C., Lange, E., et al. (2007). Specific Combinations of HLA-DR2 and DR3 Class II Haplotypes Contribute Graded Risk for Disease Susceptibility and Autoantibodies in Human SLE. Eur. J. Hum. Genet. 15, 823–830. doi:10.1038/sj.ejhg.5201827
Graves, J. S., Barcellos, L. F., Simpson, S., Belman, A., Lin, R., Taylor, B. V., et al. (2018). The Multiple Sclerosis Risk Allele within the AHI1 Gene Is Associated with Relapses in Children and Adults. Mult. Scler. Relat. Disord. 19, 161–165. doi:10.1016/j.msard.2017.10.008
GTEx Portal (2021). GTEx Portal. . https://gtexportal.org/home/snp/rs10399952 (Accessed November 1, 2021).
Hammarström, L., and Smith, C. I. (1983). HLA-A, B, C and DR Antigens in Immunoglobulin A Deficiency. Tissue Antigens 21, 75–79. doi:10.1111/j.1399-0039.1983.tb00375.x
Han, S., Park, J., and Lee, D.-H. (2015). Protein DHX38 Is a Novel Inhibitor of Protein Phosphatase 4. Anim. Cell Syst. 19, 236–244. doi:10.1080/19768354.2015.1074106
Hegele, A., Kamburov, A., Grossmann, A., Sourlis, C., Wowro, S., Weimann, M., et al. (2012). Dynamic Protein-Protein Interaction Wiring of the Human Spliceosome. Mol. Cell 45, 567–580. doi:10.1016/j.molcel.2011.12.034
Hodge, S. E., Ban, Y., Strug, L. J., Greenberg, D. A., Davies, T. F., Concepcion, E. S., et al. (2006). Possible Interaction between HLA-Drβ1 and Thyroglobulin Variants in Graves' Disease. Thyroid 16, 351–355. doi:10.1089/thy.2006.16.351
Jacobson, E. M., Huber, A., and Tomer, Y. (2008). The HLA Gene Complex in Thyroid Autoimmunity: from Epidemiology to Etiology. J. Autoimmun. 30, 58–62. doi:10.1016/j.jaut.2007.11.010
Jensen, L. J., Kuhn, M., Stark, M., Chaffron, S., Creevey, C., Muller, J., et al. (2009). STRING 8--a Global View on Proteins and Their Functional Interactions in 630 Organisms. Nucleic Acids Res. 37, D412–D416. doi:10.1093/nar/gkn760
Jorgensen, G. H., Gardulf, A., Sigurdsson, M. I., Sigurdardottir, S. T., Thorsteinsdottir, I., Gudmundsson, S., et al. (2013). Clinical Symptoms in Adults with Selective IgA Deficiency: a Case-Control Study. J. Clin. Immunol. 33, 742–747. doi:10.1007/s10875-012-9858-x
Kichaev, G., Bhatia, G., Loh, P.-R., Gazal, S., Burch, K., Freund, M. K., et al. (2019). Leveraging Polygenic Functional Enrichment to Improve GWAS Power. Am. J. Hum. Genet. 104, 65–75. doi:10.1016/j.ajhg.2018.11.008
Kowalczyk, D., Macura-Biegun, A., and Zembala, M. (2006). The Expression of CD40 on Monocytes of Children with Primary Humoral Immunodeficiencies. Pediatr. Res. 59, 816–819. doi:10.1203/01.pdr.0000219298.96471.18
Kula, D., Bednarczuk, T., Jurecka-Lubieniecka, B., Polanska, J., Hasse-Lazar, K., Jarzab, M., et al. (2006). Interaction of HLA-DRB1 Alleles with CTLA-4 in the Predisposition to Graves' Disease: the Impact of DRB1*07. Thyroid 16, 447–453. doi:10.1089/thy.2006.16.447
Kumasaka, N., Nakamura, Y., and Kamatani, N. (2010). The Textile Plot: a New Linkage Disequilibrium Display of Multiple-Single Nucleotide Polymorphism Genotype Data. PLoS One 5, e10207. doi:10.1371/journal.pone.0010207
Liberzon, A., Birger, C., Thorvaldsdóttir, H., Ghandi, M., Mesirov, J. P., and Tamayo, P. (2015). The Molecular Signatures Database Hallmark Gene Set Collection. Cell Syst. 1, 417–425. doi:10.1016/j.cels.2015.12.004
Liu, P., Lassén, E., Nair, V., Berthier, C. C., Suguro, M., Sihlbom, C., et al. (2017). Transcriptomic and Proteomic Profiling Provides Insight into Mesangial Cell Function in IgA Nephropathy. Jasn 28, 2961–2972. doi:10.1681/asn.2016101103
Machiela, M. J., and Chanock, S. J. (2015). LDlink: a Web-Based Application for Exploring Population-specific Haplotype Structure and Linking Correlated Alleles of Possible Functional Variants: Fig. 1. Bioinformatics 31, 3555–3557. doi:10.1093/bioinformatics/btv402
MacHulla, H. K., Schonermarck, U., Schaaf, A., Muller, L. P., Kloss, C., Kruger, J., et al. (2000). HLA-A, B, Cw and DRB1, DRB3/4/5, DQB1, DPB1 Frequencies in German Immunoglobulin A-Deficient Individuals. Scand. J. Immunol. 52, 207–211. doi:10.1046/j.1365-3083.2000.00765.x
Magnusson, P. K. E., Almqvist, C., Rahman, I., Ganna, A., Viktorin, A., Walum, H., et al. (2013). The Swedish Twin Registry: Establishment of a Biobank and Other Recent Developments. Twin Res. Hum. Genet. 16, 317–329. doi:10.1017/thg.2012.104
Okada, Y., Hirota, T., Kamatani, Y., Takahashi, A., Ohmiya, H., Kumasaka, N., et al. (2011). Identification of Nine Novel Loci Associated with white Blood Cell Subtypes in a Japanese Population. Plos Genet. 7, e1002067. doi:10.1371/journal.pgen.1002067
Okada, Y., Yamazaki, K., Umeno, J., Takahashi, A., Kumasaka, N., Ashikawa, K., et al. (2011). HLA-Cw*1202-B*5201-DRB1*1502 Haplotype Increases Risk for Ulcerative Colitis but Reduces Risk for Crohn's Disease. Gastroenterology 141, 864–8715. doi:10.1053/j.gastro.2011.05.048
Olerup, O., Edvard Smith, C. I., and Hammarström, L. (1990). Different Amino Acids at Position 57 of the HLA-Dqβ Chain Associated with Susceptibility and Resistance to IgA Deficiency. Nature 347, 289–290. doi:10.1038/347289a0
Park, J. H., Chang, H. S., Park, C.-S., Jang, A.-S., Park, B. L., Rhim, T. Y., et al. (2007). Association Analysis of CD40 Polymorphisms with Asthma and the Level of Serum Total IgE. Am. J. Respir. Crit. Care Med. 175, 775–782. doi:10.1164/rccm.200609-1286oc
Paulie, S., Ehlin-Henriksson, B., Mellstedt, H., Koho, H., Ben-Aissa, H., and Perlmann, P. (1985). A P50 Surface Antigen Restricted to Human Urinary Bladder Carcinomas and B Lymphocytes. Cancer Immunol. Immunother. 20, 23–28. doi:10.1007/BF00199769
Picard, C., Al-Herz, W., Bousfiha, A., Casanova, J.-L., Chatila, T., Conley, M. E., et al. (2015). Primary Immunodeficiency Diseases: an Update on the Classification from the International Union of Immunological Societies Expert Committee for Primary Immunodeficiency 2015. J. Clin. Immunol. 35, 696–726. doi:10.1007/s10875-015-0201-1
Pruim, R. J., Welch, R. P., Sanna, S., Teslovich, T. M., Chines, P. S., Gliedt, T. P., et al. (2010). LocusZoom: Regional Visualization of Genome-wide Association Scan Results. Bioinformatics 26, 2336–2337. doi:10.1093/bioinformatics/btq419
Rayner, N. W. (2011). Development and Use of a Pipeline to Generate Strand and Position Information for Common Genotyping Chips.
Scales, S. J., Hesser, B. A., Masuda, E. S., and Scheller, R. H. (2002). Amisyn, a Novel Syntaxin-Binding Protein that May Regulate SNARE Complex Assembly. J. Biol. Chem. 277, 28271–28279. doi:10.1074/jbc.m204929200
Schork, A. J., Thompson, W. K., Pham, P., Torkamani, A., Roddey, J. C., Sullivan, P. F., et al. (2013). All SNPs Are Not Created Equal: Genome-wide Association Studies Reveal a Consistent Pattern of Enrichment Among Functionally Annotated SNPs. Plos Genet. 9, e1003449. doi:10.1371/journal.pgen.1003449
Schwer, B., and Guthrie, C. (1991). PRP16 Is an RNA-dependent ATPase that Interacts Transiently with the Spliceosome. Nature 349, 494–499. doi:10.1038/349494a0
Shimane, K., Kochi, Y., Suzuki, A., Okada, Y., Ishii, T., Horita, T., et al. (2013). An Association Analysis of HLA-DRB1 with Systemic Lupus Erythematosus and Rheumatoid Arthritis in a Japanese Population: Effects of *09:01 Allele on Disease Phenotypes. Rheumatology 52, 1172–1182. doi:10.1093/rheumatology/kes427
Smith, E. N., Koller, D. L., Panganiban, C., Szelinger, S., Zhang, P., Badner, J. A., et al. (2011). Genome-wide Association of Bipolar Disorder Suggests an Enrichment of Replicable Associations in Regions Near Genes. Plos Genet. 7, e1002134. doi:10.1371/journal.pgen.1002134
Smyth, D. J., Cooper, J. D., Howson, J. M. M., Walker, N. M., Plagnol, V., Stevens, H., et al. (2008). PTPN22 Trp620 Explains the Association of Chromosome 1p13 with Type 1 Diabetes and Shows a Statistical Interaction with HLA Class II Genotypes. Diabetes 57, 1730–1737. doi:10.2337/db07-1131
Steck, A. K., Liu, S.-Y., McFann, K., Barriga, K. J., Babu, S. R., Eisenbarth, G. S., et al. (2006). Association of the PTPN22/LYP Gene with Type 1 Diabetes. Pediatr. Diabetes 7, 274–278. doi:10.1111/j.1399-5448.2006.00202.x
Subramanian, A., Tamayo, P., Mootha, V. K., Mukherjee, S., Ebert, B. L., Gillette, M. A., et al. (2005). Gene Set Enrichment Analysis: a Knowledge-Based Approach for Interpreting Genome-wide Expression Profiles. Proc. Natl. Acad. Sci. 102, 15545–15550. doi:10.1073/pnas.0506580102
Takahashi, M., and Kimura, A. (2010). HLA and CTLA4 Polymorphisms May Confer a Synergistic Risk in the Susceptibility to Graves' Disease. J. Hum. Genet. 55, 323–326. doi:10.1038/jhg.2010.20
Uhlén, M., Fagerberg, L., Hallström, B. M., Lindskog, C., Oksvold, P., Mardinoglu, A., et al. (2015). Proteomics. Tissue-Based Map of the Human Proteome. Science 347, 1260419. doi:10.1126/science.1260419
Urm, S.-H., Yun, H. D., Fenta, Y. A., Yoo, K. H., Abraham, R. S., Hagan, J., et al. (2013). Asthma and Risk of Selective IgA Deficiency or Common Variable Immunodeficiency: a Population-Based Case-Control Study. Mayo Clinic Proc. 88, 813–821. doi:10.1016/j.mayocp.2013.05.021
van der Linden, M. P. M., Feitsma, A. L., le Cessie, S., Kern, M., Olsson, L. M., Raychaudhuri, S., et al. (2009). Association of a Single-Nucleotide Polymorphism inCD40with the Rate of Joint Destruction in Rheumatoid Arthritis. Arthritis Rheum. 60, 2242–2247. doi:10.1002/art.24721
van Luijn, M. M., Kreft, K. L., Jongsma, M. L., Mes, S. W., Wierenga-Wolf, A. F., van Meurs, M., et al. (2015). Multiple Sclerosis-Associated CLEC16A Controls HLA Class II Expression via Late Endosome Biogenesis. Brain 138, 1531–1547. doi:10.1093/brain/awv080
Varade, J., Wang, N., Lim, C. K., Zhang, T., Zhang, Y., Liu, X., et al. (2017). Novel Genetic Loci Associated HLA-B*08:01 Positive Myasthenia Gravis. J. Autoimmun. 88, 43–49. doi:10.1016/j.jaut.2017.10.002
Walsh, C. M., and Fruman, D. A. (2014). Too Much of a Good Thing: Immunodeficiency Due to Hyperactive PI3K Signaling. J. Clin. Invest. 124, 3688–3690. doi:10.1172/jci77198
Wang, N., Shen, N., Vyse, T. J., Anand, V., Gunnarson, I., Sturfelt, G., et al. (2011). Selective IgA Deficiency in Autoimmune Diseases. Mol. Med. 17, 1383–1396. doi:10.2119/molmed.2011.00195
Wang, Y., and Guthrie, C. (1998). PRP16, a DEAH-Box RNA Helicase, Is Recruited to the Spliceosome Primarily via its Nonconserved N-Terminal Domain. RNA 4, 1216–1229. doi:10.1017/s1355838298980992
Wolfe, D., Dudek, S., Ritchie, M. D., and Pendergrass, S. A. (2013). Visualizing Genomic Information across Chromosomes with PhenoGram. BioData Mining 6, 18. doi:10.1186/1756-0381-6-18
Wood, A. R., Esko, T., Yang, J., Vedantam, S., Pers, T. H., Gustafsson, S., et al. (2014). Defining the Role of Common Variation in the Genomic and Biological Architecture of Adult Human Height. Nat. Genet. 46, 1173–1186. doi:10.1038/ng.3097
Xie, M., Zhang, D., Zhang, Y., Yang, X., Su, Y., Wang, Y., et al. (2017). Association of Genetic Polymorphisms in IL-1R1 and IL-1R2 Genes with IgA Nephropathy in the Han Chinese Population. Oncotarget 8, 50673–50679. doi:10.18632/oncotarget.16929
Yang, J., Manolio, T. A., Pasquale, L. R., Boerwinkle, E., Caporaso, N., Cunningham, J. M., et al. (2011). Genome Partitioning of Genetic Variation for Complex Traits Using Common SNPs. Nat. Genet. 43, 519–525. doi:10.1038/ng.823
Keywords: immunoglobulin a deficiency, major histocompatibility complex (MHC), non-MHC genes, HLA risk allele, Stratification
Citation: Lim CK, Bronson PG, Varade J, Behrens TW and Hammarström L (2021) STXBP6 and B3GNT6 Genes are Associated With Selective IgA Deficiency. Front. Genet. 12:736235. doi: 10.3389/fgene.2021.736235
Received: 04 July 2021; Accepted: 11 November 2021;
Published: 17 December 2021.
Edited by:
Mikko Risto Juhana Seppänen, Helsinki University Central Hospital, FinlandReviewed by:
Javier Martin, Instituto de Parasitología y Biomedicina López-Neyra (IPBLN), SpainXiao Chang, Children’s Hospital of Philadelphia, United States
Copyright © 2021 Lim, Bronson, Varade, Behrens and Hammarström. This is an open-access article distributed under the terms of the Creative Commons Attribution License (CC BY). The use, distribution or reproduction in other forums is permitted, provided the original author(s) and the copyright owner(s) are credited and that the original publication in this journal is cited, in accordance with accepted academic practice. No use, distribution or reproduction is permitted which does not comply with these terms.
*Correspondence: Lennart Hammarström, TGVubmFydC5IYW1tYXJzdHJvbUBraS5zZQ==