- Department of Respiratory Medicine, Nanjing Chest Hospital, Affiliated Nanjing Brain Hospital, Nanjing Medical University, Nanjing, China
Background: aldolase A (ALDOA) has been reported to be involved in kinds of cancers. However, the role of ALDOA in lung adenocarcinoma has not been fully elucidated. In this study, we explored the prognostic value and correlation with immune infiltration of ALDOA in lung adenocarcinoma.
Methods: The expression of ALDOA was analyzed with the Oncomine database, the Cancer Genome Atlas (TCGA), and the Human Protein Atlas (HPA). Mann-Whitney U test was performed to examine the relationship between clinicopathological characteristics and ALDOA expression. The receiver operating characteristic (ROC) curve and Kaplan-Meier method were conducted to describe the diagnostic and prognostic importance of ALDOA. The Search Tool for the Retrieval of Interacting Genes (STRING) and Cytoscape were used to construct PPI networks and identify hub genes. Functional annotations and immune infiltration were conducted.
Results: The mRNA and protein expression of ALDOA were higher in lung adenocarcinoma than those in normal tissues. The overexpression of ALDOA was significantly correlated with the high T stage, N stage, M stage, and TNM stage. Kaplan-Meier showed that high expression of ALDOA was correlated with short overall survival (38.9 vs 72.5 months, p < 0.001). Multivariate analysis revealed that ALDOA (HR 1.435, 95%CI, 1.013–2.032, p = 0.042) was an independent poor prognostic factor for overall survival. Functional enrichment analysis showed that positively co-expressed genes of ALDOA were involved in the biological progress of mitochondrial translation, mitochondrial translational elongation, and negative regulation of cell cycle progression. KEGG pathway analysis showed enrichment function in carbon metabolism, the HIF-1 signaling pathway, and glycolysis/gluconeogenesis. The “SCNA” module analysis indicated that the copy number alterations of ALDOA were correlated with three immune cell infiltration levels, including B cells, CD8+ T cells, and CD4+ T cells. The “Gene” module analysis indicated that ALDOA gene expression was negatively correlated with infiltrating levels of B cells, CD8+ T cells, CD4+ T cells, and macrophages.
Conclusion: Our study suggested that upregulated ALDOA was significantly correlated with tumor progression, poor survival, and immune infiltrations in lung adenocarcinoma. These results suggest that ALDOA is a potential prognostic biomarker and therapeutic target in lung adenocarcinoma.
Introduction
According to the latest data from global cancer statistics, lung cancer is the most commonly diagnosed cancer and the leading cause of cancer-related death around the whole world (Bray et al., 2018). Lung adenocarcinoma is the most common pathological type and accounts for more than 40% of all lung cancers (Travis et al., 2015; Denisenko et al., 2018). Despite advances that have been made in early diagnosis and treatment for lung adenocarcinoma in the past years, including targeted therapy and immunotherapy (Zhou and Yao, 2016; Hanna et al., 2017; Xu et al., 2018), the prognosis of lung adenocarcinoma patients remains bleak (Zhang et al., 2019). Therefore, it is imperative to search for novel prognostic markers and therapeutic targets for lung adenocarcinoma.
Aldolase A (ALDOA), also called muscle-type aldolase, is mainly expressed in muscle tissues (Tochio et al., 2010). ALDOA encodes a glycolytic enzyme that catalyzes the reversible conversion of fructose-1,6-bisphosphate to glyceraldehyde 3-phosphate and dihydroxyacetone phosphate. Ectopic expression of ALDOA is important in the development of cardiac hypertrophy, heart failure, and many cardio-cerebrovascular diseases (Hu et al., 2013). Furthermore, ALDOA has been reported to be involved in gluconeogenesis and glycolysis (Zeng et al., 2016). Based on both gluconeogenesis and glycolysis can provide energy for tumor proliferation, accumulating evidence has indicated that ALDOA plays an important role in the pathological progress of several cancers. A paper from Saito et al. indicated that upregulated ALDOA in cervical adenocarcinoma can increase the metastasis and invasion of cervical adenocarcinoma cells via promoting epithelial-mesenchymal transition (EMT) (Saito et al., 2020). Concerning non-small cell lung cancer, Fu et al. reported that ALDOA can activate the EGFR/MAPK pathway to promote cyclin D1 expression, enhance proliferation and G1/G transition, and facilitate aerobic glycolysis (Fu et al., 2018). These findings indicate that ALDOA plays an important role in tumor progression.
In the present study, we conducted bioinformatics analyses on ALDOA in lung adenocarcinoma patients, including transcriptional expression and mutation analysis, survival analysis, functional enrichment analysis. We also performed co-expression analysis, constructed the predicted protein-protein interaction (PPI) networks, and identified hub genes of co-expressed genes with ALDOA. Moreover, we determined the relationship between ALDOA expression and immune cell infiltration in lung adenocarcinoma. Our results link the expression of ALDOA with a poor prognosis and provide a potential therapeutic target for lung adenocarcinoma.
Materials and Methods
Oncomine Database
Oncomine (https://www.oncomine.org/) is an online platform that provides solutions to compute gene expression signatures, clusters, and gene-set modules, automatically extracting biological insights (Rhodes et al., 2007). In this study, we conducted Oncomine to evaluate the mRNA expression of ALDOA in lung adenocarcinoma. The results drew from a series of lung adenocarcinoma studies, including Selamat lung, Landi lung, Hou lung, Okayama lung, Stearman lung, Su lung, and Garber lung (Garber et al., 2001; Stearman et al., 2005; Su et al., 2007; Landi et al., 2008; Hou et al., 2010; Okayama et al., 2012; Selamat et al., 2012).
The Cancer Genome Atlas (TCGA)
TCGA (https://portal.gdc.cancer.gov/) is a genomics data resource that characterized, and analyzed cancer samples (Tomczak et al., 2015). In this study, we analyzed the transcription level of ALDOA in multiple cancers from TCGA. The mRNA expression and associated clinical data of ALDOA in lung adenocarcinoma were also downloaded from TCGA. The mRNA data of FPKM format has been converted into TPM.
Tumor Immune Estimation Resource (TIMER)
TIMER (http://timer.cistrome.org/) is an online database and allows users to analyze the differential expression between tumor and normal tissues across all TCGA tumors, and study the correlation between gene expression and immune infiltration level (Li et al., 2020). In the present study, we conducted TIMER to determine the expression of ALDOA in diverse cancer types. Moreover, we applied TIMER to explore the correlation between ALDOA expression and the abundance of tumor-infiltrating immune cells (B cells, CD4+ T cells, CD8+ T cells, neutrophils, macrophages, and dendritic cells). In addition, TIMER was used to study the correlation between ALDOA expression and gene markers of tumor-infiltrating immune cells.
UALCAN
The UALCAN (http://ualcan.path.uab.edu/) is a comprehensive online web resource that provides easy access to analyze publicly available cancer omics data (Chandrashekar et al., 2017). In this study, we performed UALCAN to compare the mRNA and protein expression of ALDOA from TCGA and Clinical Proteomic Tumor Analysis Consortium (CPTAC, https://proteomics.cancer.gov/programs/cptac) (Edwards et al., 2015).
The Human Protein Atlas (HPA)
The HPA database (https://proteinatlas.org/) is aimed to map all the human proteins with an integration of various omics technologies (Uhlén et al., 2015; Uhlen et al., 2017). All the data of human proteins includes expression profiles in cells, tumor tissues, and normal tissues. In this study, we performed HPA to confirm the protein expression of ALDOA in lung adenocarcinoma.
Gene Expression Profiling Interactive Analysis (GEPIA2)
GEPIA 2 (http://gepia2.cancer-pku.cn/) is a web-based tool to provide interactive and customizable functions, including gene expression analysis, correlation analysis, survival analysis, similar genes detection, and dimensionality reduction analysis (Tang et al., 2019). In this study, we conducted GEPIA2 to examine the correlation between ALDOA expression and overall survival. In addition, GEPIA2 was used to assess the correlation between ALDOA expression and gene markers of tumor-infiltrating immune cells.
The Kaplan Meier Plotter
The Kaplan Meier plotter (http://www.kmplot.com/analysis/) is an online tool to assess the effect of 54k genes on survival across cancers including breast, ovarian, lung, and gastric cancer (Nagy et al., 2021). Gene expression data and information of relapse-free and overall survival are downloaded from Gene Expression Omnibus (GEO), European Genome-phenome Archive (EGA), and TCGA. In this study, we performed a Kaplan Meier plotter to validate the prognostic value of ALDOA in lung adenocarcinoma.
c-BioPortal Database
The c-Bio Cancer Genomics Portal (https://www.cbioportal.org/) is an open-access online resource for interactive exploration of many cancer genomics databases (Cerami et al., 2012). The cancer research community can utilize genomic data easily and directly with c-BioPortal. In this study, we performed c-BioPortal databases to explore mutation data of ALDOA in lung adenocarcinoma, obtain its prognostic value in altered lung adenocarcinoma patients, acquire co-expressed genes of ALDOA, and determine the correlation between ALDOA and mRNA expression of 10 hub genes.
STRING Database and Cytoscape Platform
The Search Tool for the Retrieval of Interacting Genes (STRING, http://string-db.org, Version 11.0) is an online database to analyze functional enrichment and PPI networks (Szklarczyk et al., 2019). Cytoscape (Version 3.6.1) is an open-source software platform for integrating and visualizing complex networks (Shannon et al., 2003). In this study, to construct PPI networks of co-expressed genes and identify hub genes, we imported the co-expressed genes into STRING and then explored the degree scores with cytoHubba tool kits in Cytoscape.
Statistical Analyses
Statistical analyses and visualization of expression differences were performed with R (V 3.6.3, https://www.r-project.org/) and R package ggplot2. Mann-Whitney U test was conducted to observe the differences between lung adenocarcinoma tissues and adjacent normal tissues. R package pROC (Robin et al., 2011) and clusterProfiler (Yu et al., 2012) were conducted to explore the diagnostic importance and functional enrichment analysis of co-expressed genes in lung adenocarcinoma.
Results
Expression of ALDOA in Lung Adenocarcinoma From Oncomine
To evaluate the transcription level of ALDOA in multiple lung adenocarcinoma studies, we performed an analysis on Oncomine. As shown in Figures 1A–H, the transcription level of ALDOA was upregulated in lung adenocarcinoma tissues than in normal tissues. The fold change of ALDOA differed from 1.697 to 2.154, and mRNA expression was up to the top 14%. These data indicated transcription level of ALDOA is increased in lung adenocarcinoma tissues.
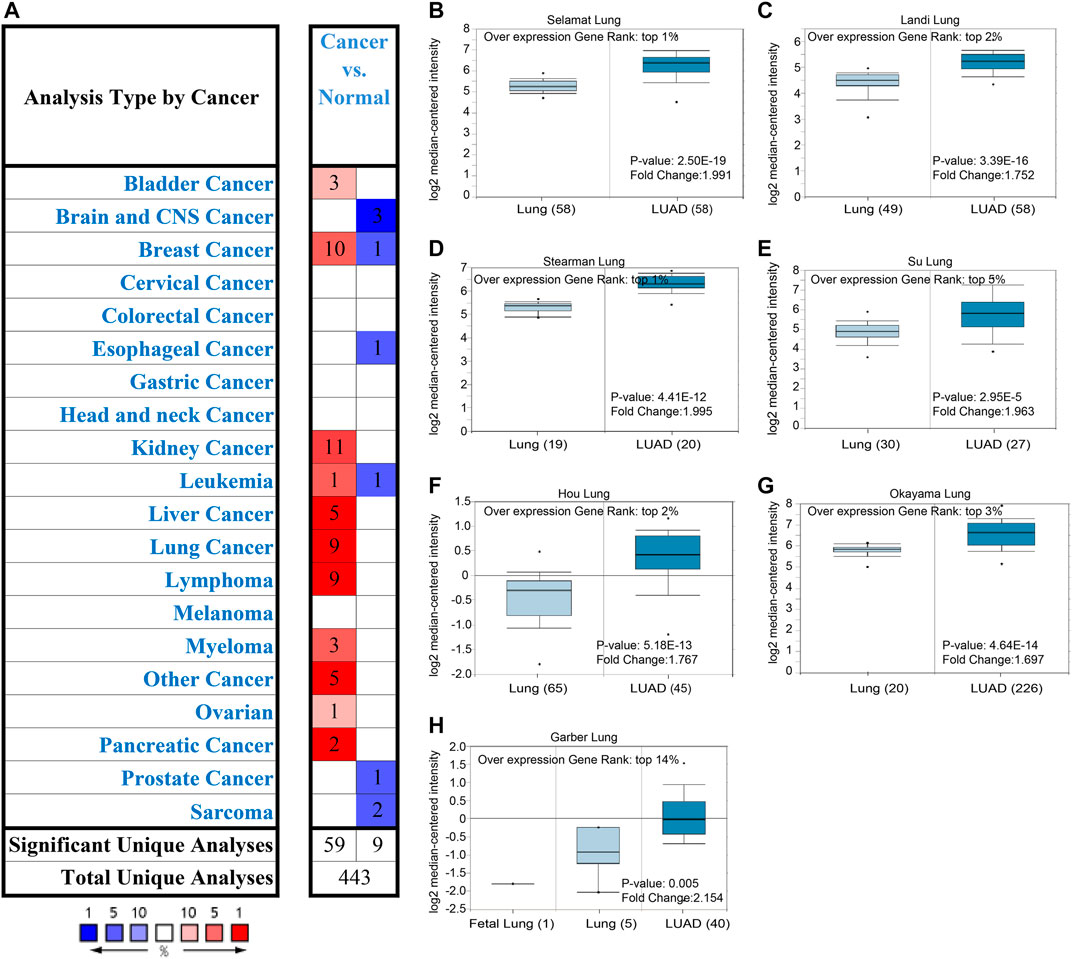
FIGURE 1. Expression of ALDOA from Oncomine (A) ALDOA expression in different types of cancers. Red means up-regulated and blue means down-regulated (B-H) BOX plot showing the mRNA expression levels of ALDOA in Selamat lung, Landi lung, Hou lung, Okayama lung, Stearman lung, Su lung, and Garber lung datasets, respectively.
Expression of ALDOA in Pan-Cancer Perspective and Lung Adenocarcinoma From TCGA, UALCAN, and HPA
To further evaluate the expression of ALDOA in multiple cancers, we performed an analysis on TCGA with TIMER. As shown in Figure 2A, the mRNA expression of ALDOA was upregulated in 14 cancer types, including lung adenocarcinoma and lung squamous cell carcinoma. ALDOA was downregulated in two cancer types, including GBM and PRAD. The result from UALCAN indicated that both mRNA and protein expression of ALDOA in lung adenocarcinoma tissues were significantly higher than that in normal tissues (p = 1.62E-12, p = 7.75E-31, respectively) (Figures 2B,C). Consistent with the results of UALCAN, HPA showed protein expression of ALDOA in lung adenocarcinoma tissue was higher than that in normal lung tissue (Figures 2D,E). All these results indicate that ALDOA is upregulated in lung adenocarcinoma tissues.
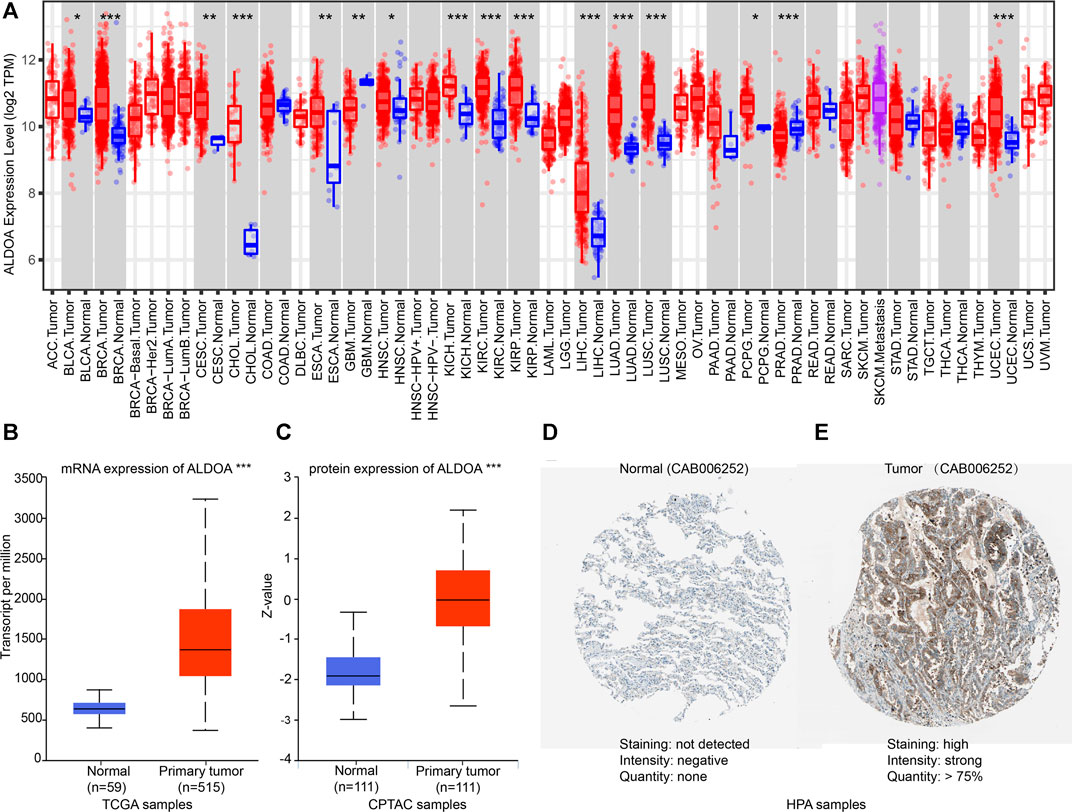
FIGURE 2. Expression of ALDOA from TCGA, CPTAC, and HPA (A) ALDOA was upregulated in 14 cancer types, including BLCA, BRCA, CESC, CHOL, ESCA, HNSC, KICH, KIRC, KIRP, LIHC, LUAD, LUSC, PCPG, and UCEC. ALDOA was downregulated in GBM and PRAD (B) The mRNA expression of ALDOA in 59 normal tissues and 515 lung adenocarcinoma tissues (C) The protein expression of ALDOA in 111 lung normal tissues and 111 lung adenocarcinoma tissues (D) The protein expression of ALDOA in normal tissue. Staining was not detected, intensity was negative, and quantity was none (E) The protein expression of ALDOA in lung adenocarcinoma tissue. Staining was high, intensity was strong, and quantity >75%. HPA normal: https://www.proteinatlas.org/ENSG00000149925-ALDOA/tissue/lung#img,HPA tumor: https://www.proteinatlas.org/ENSG00000149925-ALDOA/pathology/lung+cancer#img (*, p < 0.05; **, p < 0.01, ***, p < 0.001).
The Relationship Between Clinicopathological Characteristics and ALDOA Expression in Lung Adenocarcinoma Patients From TCGA
We downloaded the mRNA expression and associated clinical data of ALDOA in lung adenocarcinoma from TCGA. The clinicopathological characteristics of lung adenocarcinoma patients were shown in Table 1. To examine the relationship between clinicopathological characteristics and ALDOA expression in TCGA cohorts, we conducted the Mann-Whitney U test. As shown in Figure 3, ALDOA mRNA expression in lung adenocarcinoma patients was significantly increased with high T stage (p = 0.025), N stage (p = 0.013), M stage (p = 0.002), and TNM stage (p < 0.001). However, no significantly differences were found between ALDOA mRNA expression and other characteristics, such as gender (p = 0.329), age (p = 0.594), smoke condition (p = 0.754), and anatomic subdivision (right vs left, p = 0.456; central vs peripheral, p = 0.682). To sum up, these data suggest that ALDOA might play an important role in tumorigenesis and metastasis of lung adenocarcinoma.
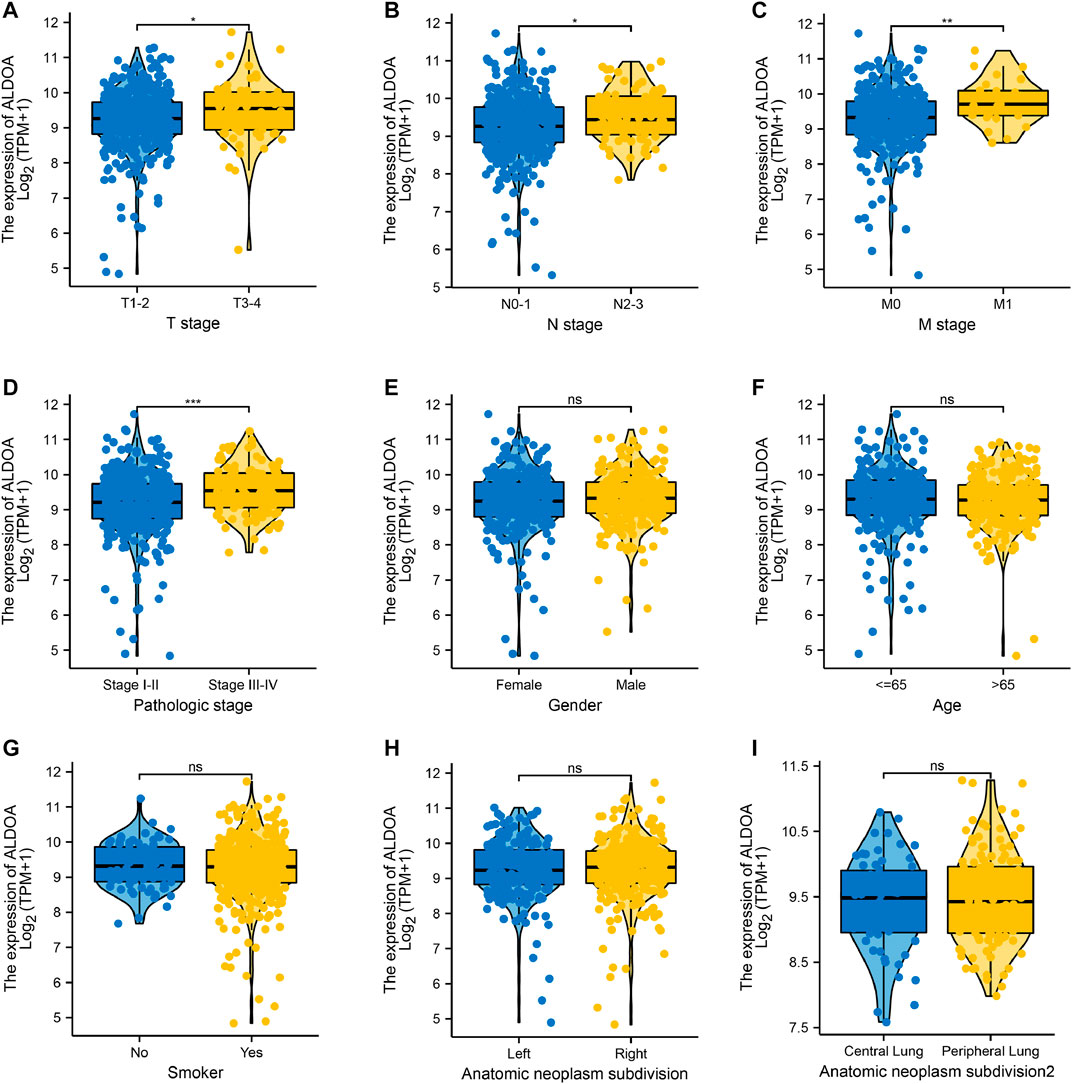
FIGURE 3. The relationship between clinicopathological characteristics and ALDOA expression in lung adenocarcinoma patients from TCGA (A-D) ALDOA mRNA expression was significantly increased with high T stage, N stage, M stage, and TNM stage (E-I) No significant differences were found in gender, age, smoke condition, and anatomic subdivision (right vs left, central vs peripheral) (ns, no significant; *, p < 0.05; **, p < 0.01, ***, p < 0.001).
Diagnostic Value of ALDOA for Distinguishing Lung Adenocarcinoma Tissues From Normal Tissues
To study the diagnostic value of ALDOA for distinguishing adenocarcinoma tissues from normal tissues, we performed ROC curve analysis with R package pROC. As shown in Figure 4. ALDOA had an AUC value of 0.909 (95% CI: 0.883–0.935). With a cutoff of 8.598, ALDOA had a sensitivity, specificity, and accuracy of 84.7, 93.2, and 85.5%, respectively. This result indicates that ALDOA might be used as a diagnostic biomarker for distinguishing lung adenocarcinoma tissues from normal tissues.
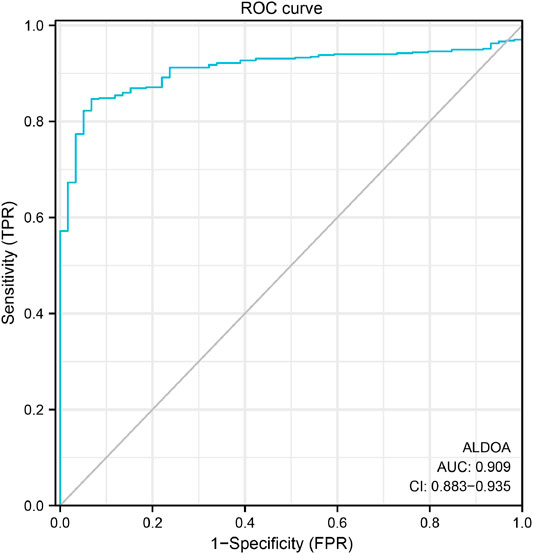
FIGURE 4. ROC curve for distinguishing lung adenocarcinoma tissues from normal tissues. With a cutoff of 8.598, ALDOA had a sensitivity, specificity, and accuracy of 84.7, 93.2, and 85.5%, respectively.
Correlation Between ALDOA Expression and Overall Survival
To explore the correlation between ALDOA expression and overall survival and disease-free survival in lung adenocarcinoma patients, the GEPIA2 server and Kaplan Meier plotter were performed. As shown in Figures 5A,B, the GEPIA2 server indicated that the overall survival rate of lung adenocarcinoma patients with high ALDOA expression was significantly lower than that of patients with low ALDOA expression (p = 0.00021). However, the correlation of ALDOA expression with disease-free survival rate was not statistically significant (Figure 5B). Consistently, the Kaplan Meier plotter showed that lung adenocarcinoma patients with high ALDOA were correlated with short overall survival (41.0 vs 55.1 months, p = 0.0022) compared to low ALDOA mRNA expression (Figure 5C). There was no statistically significant between high/low expression of ALDOA for recurrence-free survival (68.2 vs 101.5 months, p = 0.38) (Figure 5D). These data indicated that high mRNA expression of ALDOA is correlated with short overall survival in lung adenocarcinoma.
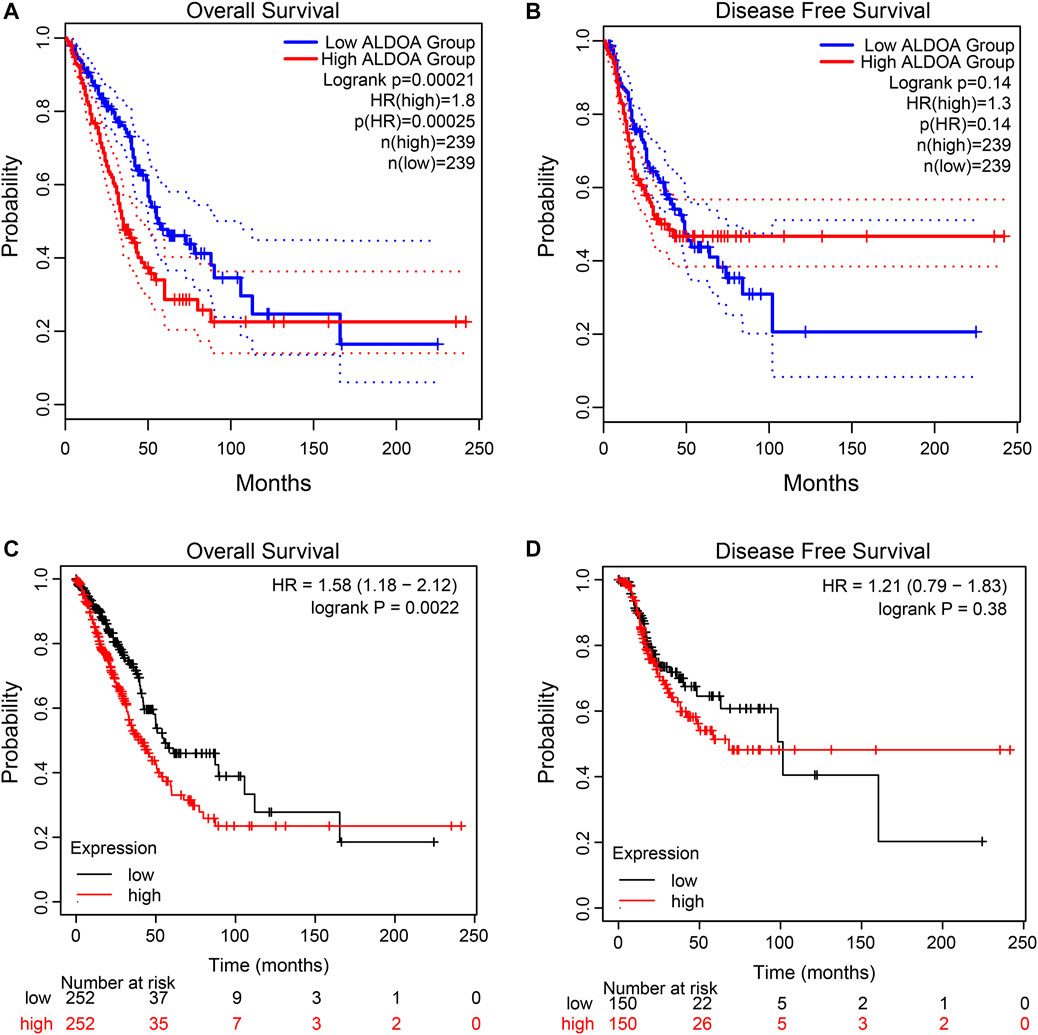
FIGURE 5. Kaplan-Meier curves for ALDOA in lung adenocarcinoma (A) Higher ALDOA led to shorter overall survival from GEPIA2 (B) ALDOA had no correlation with disease-free survival from GEPIA2 (C) High ALDOA was correlated with short overall survival from the Kaplan Meier plotter (D) ALDOA had no correlated with recurrence-free survival from the Kaplan Meier plotter.
Prognostic Importance of ALDOA mRNA in Lung Adenocarcinoma Patients
To further determine the prognostic importance of ALDOA mRNA, we conducted univariate and multivariate analyses with R package survival. Univariate analysis in Table 2 showed that the overall survival of lung adenocarcinoma patients was correlated with T stage, N stage, M stage, TNM stage, as well as the mRNA expression of ALDOA ((HR 1.799, 95%CI, 1.342–2.413, p = 0.011). Furthermore, we performed a multivariate analysis of five prognostic factors with the Cox proportional hazards model. As shown in Table 2, multivariate analysis revealed that T stage (HR 1.652, 95%CI, 1.020–2.673, p = 0.041), and mRNA expression of ALDOA (HR 1.435, 95%CI, 1.013–2.032, p = 0.042) were independent poor prognostic factors for overall survival. Moreover, a nomogram was constructed to predict the 1-, 3-, and 5-years survival probability of lung adenocarcinoma patients by combining the mRNA expression of ALDOA and clinical characteristics (Figure 6). Our data reveal that ALDOA is an independent poor prognostic factor for lung adenocarcinoma.
Genetic Mutation of ALDOA and Its Correlation With Poor Survival
To determine the mutation characteristics of ALDOA and its correlation with survival in lung adenocarcinoma, we performed an analysis on c-BioPortal databases. As shown in Figure 7A, ALDOA had a high mutation frequency of 10% in lung adenocarcinoma (TCGA, PanCancer Atlas). The main genetic mutations of ALDOA were DNA copy number amplifications and mRNA upregulation (Figure 7B). Furthermore, compared with the unaltered group (n = 449), survival analysis revealed that the altered group (n = 56) was associated with poor overall survival (Figure 7C).
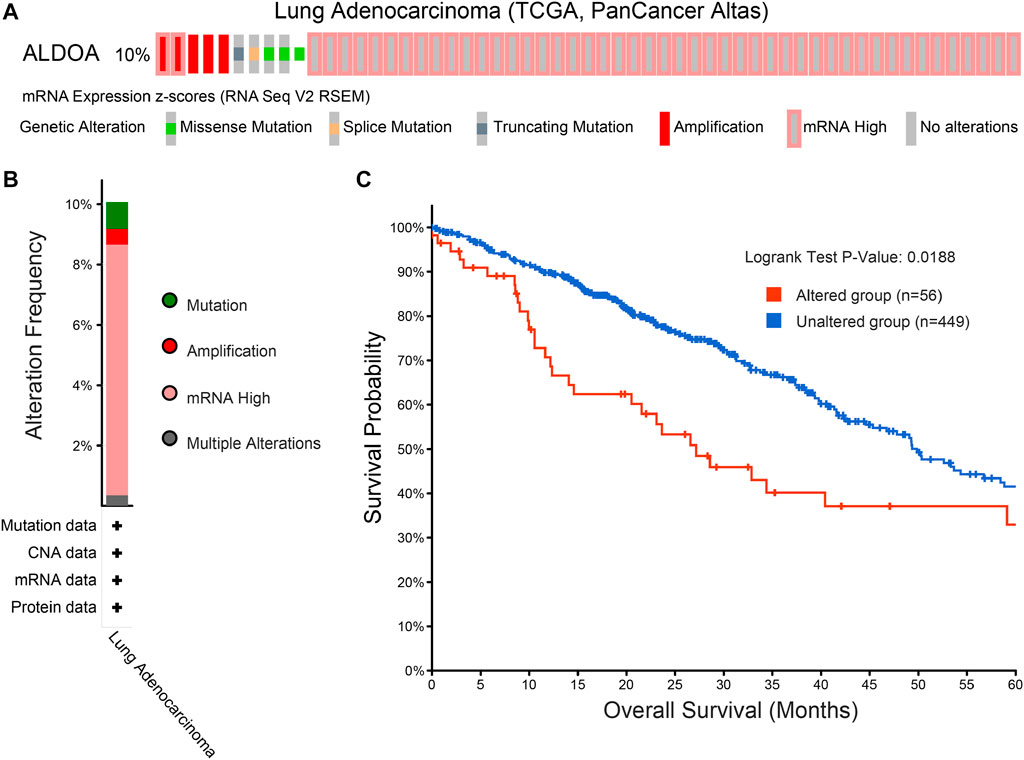
FIGURE 7. Genomic mutation of ALDOA in lung adenocarcinoma (A) OncoPrint of c-BioPortal showed the different mutation types and proportions of ALDOA (B) Cancer types summary showed the genomic alteration types in lung adenocarcinoma (C) Genomic mutation of ALDOA was correlated with poor overall survival in lung adenocarcinoma.
Functional Enrichment Analysis of Positively Co-expressed Genes of ALDOA
The top 300 co-expressed genes of ALDOA were downloaded from the c-BioPortal. As shown in Supplementary Table S1, ALDOA had 165 positively co-expressed genes. To further demonstrate the enrichment function of these positively co-expressed genes, we conducted the analyses of Gene Ontology (GO) and Kyoto Encyclopedia of Genes and Genomes (KEGG) pathway with R package clusterProfiler. GO analysis showed that positively co-expressed genes of ALDOA were involved in the biological progress of mitochondrial translation, mitochondrial translational elongation, and negative regulation of cell cycle progress (Figure 8A). They acted as structural constituents in the mitochondrial inner membrane, mitochondrial matrix, and protein complex (Figure 8B), and played an important part in the structural constituent of ribosome, isomerase activity, and monosaccharide binding (Figure 8C). KEGG pathway analysis in Figure 8D showed enrichment function in carbon metabolism, HIF-1 signaling pathway, and glycolysis/gluconeogenesis.
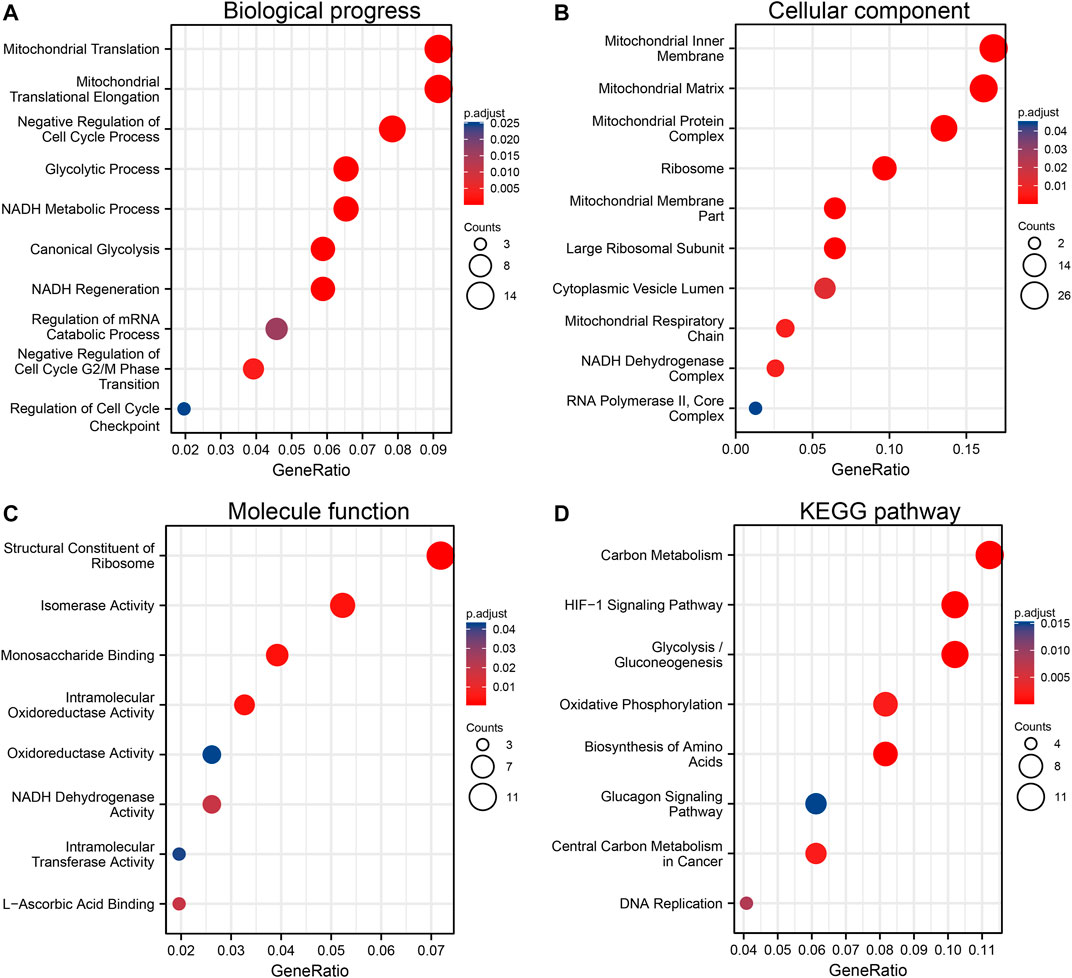
FIGURE 8. Functional enrichment analysis of positively co-expressed genes of ALDOA. (A) Biological progress of mitochondrial translation, mitochondrial translational elongation, and negative regulation of cell cycle progress (B) Cellular component of the mitochondrial inner membrane, mitochondrial matrix, and protein complex (C) Molecule function of the structural constituent of ribosome, isomerase activity, and monosaccharide binding (D) KEGG pathway analysis showed enrichment function in carbon metabolism, HIF-1 signaling pathway, and glycolysis/gluconeogenesis.
Construction of PPI Networks, Identification, and Enrichment Function of Hub Genes Among Co-expressed Genes of ALDOA
To construct PPI networks and identify hub genes of ALDOA, we imported the 165 positively co-expressed genes into STRING and then explored the degree scores with cytoHubba tool kits in Cytoscape. The PPI networks of co-expressed genes were shown in Figure 9A. As shown in Figure 9B, GADD45GIP1, MRPL22, MRPL28, MRPL21, MRPL12, MRPS12, MRPL52, MRPL17, TUFM, and MRPL53 were the top 10 hub genes of ALDOA. To further evaluate their prognostic values and correlation with ALDOA in lung adenocarcinoma, we performed analyses on GEPIA and c-BioPortal. As shown in Figure 9C, upregulation of MRPL22 (HR = 1.5, p = 0.0048), MRPL28 (HR = 1.5, p = 0.0098), MRPL21 (HR = 1.7, p = 0.001), MRPL12 (HR = 1.7, p = 0.00059), MRPS12 (HR = 1.6, p = 0.002), and MRPL17 (HR = 1.5, p = 0.0051) were correlated with poor overall survival in lung adenocarcinoma. Based on the R-value of Spearman correlation were all more than 0.4, the genes were considered as the most potential hub genes of ALDOA (Figure 9C). We also conducted enrichment function of these top 10 hub genes with R package clusterProfiler. As shown in Figure 10, GO analysis showed they were involved in the biological progress of mitochondrial translational elongation, translational elongation, and mitochondrial translation. They may be associated with a molecular function of the structural constituent of the ribosome and acted as structural constituents in the organellar ribosome, mitochondrial ribosome, and mitochondrial matrix. KEGG pathway analysis showed enrichment function in the ribosome. Taken together, all these data suggest that these hub genes may play an important role in lung adenocarcinoma by cooperating with ALDOA.
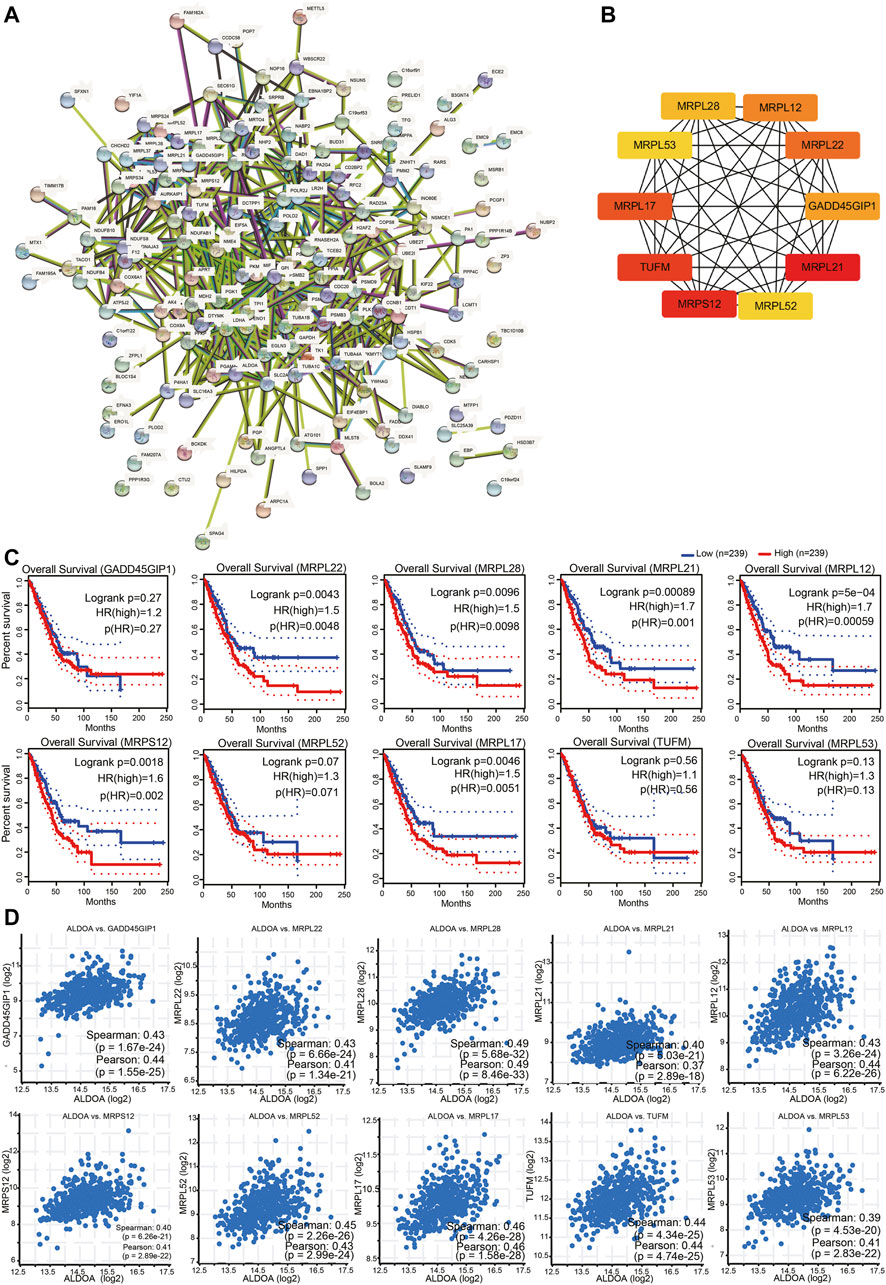
FIGURE 9. Construction of PPI networks and identification of hub genes among co-expressed genes of ALDOA (A) Construction of PPI networks by STRING with 165 positively co-expressed genes of ALDOA (B) Identification of ten hub genes of ALDOA with cytoHubba tool in Cytoscape (C) Prognostic importance analyses of 10 hub genes with GEPIA (D) Correlation between ALDOA and mRNA expression of 10 hub genes determined with c-BioPortal.
Relationship Between ALDOA Expression and Immune Infiltration in Lung Adenocarcinoma
To determine the potential relationship between ALDOA expression and immune infiltration levels in lung adenocarcinoma, we conducted a series of analyses by using TIMER. First, as shown in Figure 11A, the “SCNA” module analysis indicated that the copy number alterations of ALDOA were correlated with three immune cell infiltration levels, including B cells, CD8+ T cells, and CD4+ T cells in lung adenocarcinoma. Second, as shown in Figure 11B, the “Gene” module analysis indicated that there was no correlation between ALDOA expression and tumor purity. However, ALDOA expression was negatively correlated with infiltrating levels of B cells, CD8+ T cells, CD4+ T cells, and macrophages in lung adenocarcinoma. Third, to evaluate the impact of immune infiltration and ALDOA on the survival differences of lung adenocarcinoma patients, we used TIMER to draw Kaplan-Meier plots for immune infiltration and ALDOA. The results in Figure 11C showed that low levels of B cells, CD4+ T cells, macrophages, neutrophils, and dendritic cells were associated with poor prognosis of lung adenocarcinoma patients. On the contrary, high levels of ALDOA were correlated with the poor prognosis of lung adenocarcinoma patients. Taken together, these results suggest that ALDOA may regulate the expression level of tumor-infiltrating immune cells to affect lung adenocarcinoma and clinical prognosis.
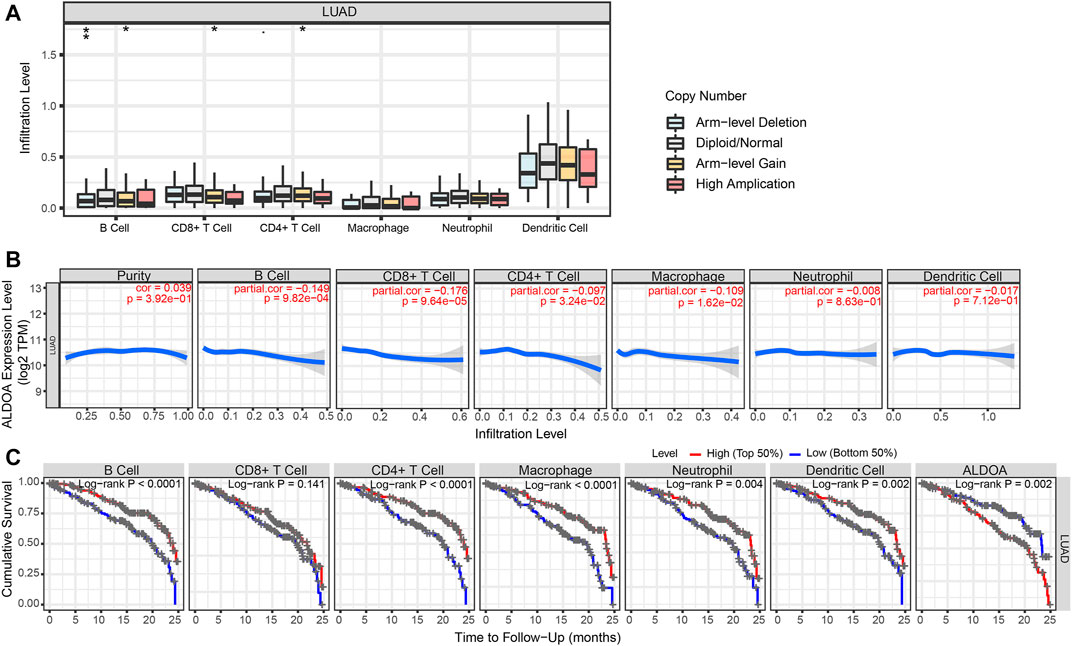
FIGURE 11. Immune cell infiltration of ALDOA in lung adenocarcinoma (A) The “SCNA” module analysis indicated that the copy number alterations of ALDOA were correlated with three immune cell infiltration levels (B) The “Gene” module analysis indicated that there was no correlation between ALDOA expression and tumor purity. However, ALDOA expression was negatively correlated with infiltrating levels of B cells, CD8+ T cells, CD4+ T cells, and macrophages (C) Low levels of B cells, CD4+ T cells, macrophages, neutrophils, and dendritic cells were associated with poor prognosis of lung adenocarcinoma patients.
Correlation Between ALDOA Expression and Gene Markers of Tumor-Infiltrating Immune Cells
To further evaluate the relationship between ALDOA and tumor-infiltrating immune cells, we next explored the correlation between ALDOA expression and immunological markers in lung adenocarcinoma using the TIMER database. We determined ALDOA expression and immunological markers of various immune cells, including B cell, CD8+ T cell, T cell (general), M1 and M2 macrophage, neutrophils, and dendritic cell. After adjusting the correlation by tumor purity, these results revealed that there was a correlation between ALDOA expression and most immune marker sets (Table 3). In particular, ALDOA was significantly correlated with T cell markers (CD3E, CD2), neutrophils markers (CD66b, CCR7), and dendritic cell markers (HLA-DPB1, BDCA-1). We also assessed the correlation between ALDOA and these markers in lung adenocarcinoma using the GEPIA2 database, and the results were similar to those in TIMER (Supplementary Table S1).
Discussion
Many studies about the dysregulation of the ALDOA gene have emerged in recent years, including colorectal cancer (Dai et al., 2018), gastric cancer (Jiang et al., 2018), and renal cell carcinoma (Huang et al., 2018). Previous bioinformatics results also indicated that ALDOA expression was correlated with prognosis in bladder cancer (Li et al., 2019), hepatocellular cancer (Tang et al., 2021). In lung cancer, overexpression of ALDOA is reported to promote lung cancer cell proliferation and metastasis (Chang et al., 2019). Moreover, Zhang et al. reported that upregulated transcriptional levels of ALDOA were correlated with cell cycle-related genes and could regulate progress in non-small cell lung cancer (Zhang et al., 2017). However, the relationship between the expression level of ALDOA and prognostic value and immune infiltration of lung adenocarcinoma has not been studied. To the best of our knowledge, for the first time, our study explored the prognostic value and correlation with immune infiltration of ALDOA in lung adenocarcinoma.
In this study, based on the data from Oncomine, TCGA, UALCAN, and HPA, we revealed that the mRNA and protein expression of ALDOA is upregulated in lung adenocarcinoma tissues. Given that there are significant differences between lung adenocarcinoma and normal tissues grouped by T stage, N stage, M stage, and TNM stage, we conclude that ALDOA might promote tumorigenesis and metastasis in lung adenocarcinoma. A paper from Marcišauskas et al. reported that ALDOA in cyst fluids and serum can be used as a diagnostic biomarker to separate stage I type 1 and type 2 ovarian cancers from benign serous adenoma (Marcišauskas et al., 2019). ROC curve can be used to examine the diagnostic value of biomarkers (Do and Le, 2021; Le et al., 2021). In the current study, ROC curve analysis suggested that ALDOA can act as a prospective non-invasive diagnostic biomarker to differentiate lung adenocarcinoma tissues from adjacent normal tissues. Previous studies reported that upregulation of ALDOA is correlated with poor prognosis in colorectal cancer (Dai et al., 2018), gastric cancer (Jiang et al., 2018), and hepatocellular carcinoma (Tang et al., 2021). Our data on survival analysis with GEPIA2 and the Kaplan Meier plotter indicated that lung adenocarcinoma patients with high ALDOA expression or genetic alteration have a poor overall survival prognosis. Univariate and multivariate analysis revealed that ALDOA is an independent poor prognostic factor for overall survival in lung adenocarcinoma. The major strength of this study is our findings raise the possibility that the upregulation of ALDOA could be a potential prognostic marker in lung adenocarcinoma.
Functional enrichment analysis was carried out to further explore the role of these positively co-expressed genes with ALDOA in lung adenocarcinoma. GO enrichment analysis showed that these positively co-expressed genes of ALDOA were involved in the biological progress of mitochondrial translation and negative regulation of cell cycle progression. KEGG pathway enrichment analysis showed enrichment function in carbon metabolism, HIF-1 signaling pathway, and glycolysis/gluconeogenesis. It is well known that the HIF-1 signaling pathway and glycolysis/gluconeogenesis play an important role in tumor invasion and metastasis (Chen et al., 2011; Rankin and Giaccia, 2016; Peng et al., 2020). Based on our results, we speculate that ALDOA may be involved in the progress of invasion and metastasis in lung adenocarcinoma. However, this should be tested in other experiments. Moreover, we also imported the positively co-expressed genes of ALDOA into the STRING database and Cytoscape to obtain the PPI network and identify hub genes. In light of STRING database analysis, we conducted a PPI network and interactions among these positively co-expressed genes. The Cytoscape with cytoHubba tool kits, GEPIA, and c-BioPortal analysis suggested that upregulated hub genes of MRPL22, MRPL28, MRPL21, MRPL12, MRPS12, and MRPL17 are correlated with poor overall survival and may play a key role by cooperating with ALDOA in lung adenocarcinoma.
Many studies about the possible role of immune infiltration have emerged in recent years. It is reported that immune infiltration is correlated with prognosis in human tumors (Pagès et al., 2010; Lei et al., 2020). However, the relationship between ALDOA expression and immune infiltration has not been investigated. In the present study, we reported that ALDOA copy number alterations were correlated with immune infiltration levels of B cells, CD8+ T cells, and CD4+ T cells in lung adenocarcinoma by TIMER. We also confirmed that ALDOA gene expression was inversely correlated with infiltrating levels of B cells, CD8+ T cells, CD4+ T cells, and macrophages in lung adenocarcinoma. Moreover, previous studies showed that there was a correlation between tumor-infiltrating immune cell expression and the prognosis of lung cancer patients (Liu et al., 2017; Wang et al., 2019; Pan et al., 2020). In this study, our results showed low levels of B cells, CD4+ T cells, macrophages, neutrophils, and dendritic cells were associated with poor prognosis of lung adenocarcinoma patients, while high ALDOA expression was correlated with poor prognosis in lung adenocarcinoma patients. Based on our data, we conclude for the first time that ALDOA is correlated with immune infiltration in lung adenocarcinoma. We further speculate that ALDOA can regulate the expression level of tumor-infiltrating immune cells to affect the clinical prognosis of lung adenocarcinoma patients.
In conclusion, our research suggests that the upregulation of ALDOA is correlated with tumorigenesis and metastasis in lung adenocarcinoma. Our results show high expression of ALDOA predicts poor prognosis and ALDOA is an independent poor prognostic factor for overall survival. ALDOA may regulate tumor-infiltrating immune cells to affect the clinical prognosis of lung adenocarcinoma patients. Our data provide a potential prognostic biomarker and therapeutic target for lung adenocarcinoma.
Data Availability Statement
The original contributions presented in the study are included in the article/Supplementary Material, further inquiries can be directed to the corresponding author.
Author Contributions
YZ designed the study. GL contributed analysis tools and wrote the manuscript. WS performed data analysis. All authors reviewed the manuscript and declared that they have no conflict of interest.
Funding
This study was funded by Wu JiePing Medical Foundation (320.6750.19059), “The 13 TH Five-Year Plan” the Major Program of Nanjing Medical Science and Technique Development Foundation (ZDX16012).
Conflict of Interest
The authors declare that the research was conducted in the absence of any commercial or financial relationships that could be construed as a potential conflict of interest.
Publisher’s Note
All claims expressed in this article are solely those of the authors and do not necessarily represent those of their affiliated organizations, or those of the publisher, the editors and the reviewers. Any product that may be evaluated in this article, or claim that may be made by its manufacturer, is not guaranteed or endorsed by the publisher.
Supplementary Material
The Supplementary Material for this article can be found online at: https://www.frontiersin.org/articles/10.3389/fgene.2021.721021/full#supplementary-material
References
Bray, F., Ferlay, J., Soerjomataram, I., Siegel, R. L., Torre, L. A., and Jemal, A. (2018). Global Cancer Statistics 2018: GLOBOCAN Estimates of Incidence and Mortality Worldwide for 36 Cancers in 185 Countries. CA: A Cancer J. Clinicians 68 (6), 394–424. doi:10.3322/caac.21492
Cerami, E., Gao, J., Dogrusoz, U., Gross, B. E., Sumer, S. O., Aksoy, B. A., et al. (2012). The cBio Cancer Genomics Portal: An Open Platform for Exploring Multidimensional Cancer Genomics Data: Figure 1. Cancer Discov. 2 (5), 401–404. doi:10.1158/2159-8290.cd-12-0095
Chandrashekar, D. S., Bashel, B., Balasubramanya, S. A. H., Creighton, C. J., Ponce-Rodriguez, I., Chakravarthi, B. V. S. K., et al. (2017). UALCAN: A Portal for Facilitating Tumor Subgroup Gene Expression and Survival Analyses. Neoplasia 19 (8), 649–658. doi:10.1016/j.neo.2017.05.002
Chang, Y. C., Chiou, J., Yang, Y. F., Su, C. Y., Lin, Y. F., Yang, C. N., et al. (2019). Therapeutic Targeting of Aldolase A Interactions Inhibits Lung Cancer Metastasis and Prolongs Survival. Cancer Res. 79 (18), 4754–4766. doi:10.1158/0008-5472.CAN-18-4080
Chen, C., Fu, X., Zhang, D., Li, Y., Xie, Y., Li, Y., et al. (2011). Varied Pathways of Stage IA Lung Adenocarcinomas Discovered by Integrated Gene Expression Analysis. Int. J. Biol. Sci. 7 (5), 551–566. doi:10.7150/ijbs.7.551
Dai, L., Pan, G., Liu, X., Huang, J., Jiang, Z., Zhu, X., et al. (2018). High Expression of ALDOA and DDX5 Are Associated with Poor Prognosis in Human Colorectal Cancer. Cmar Vol. 10, 1799–1806. doi:10.2147/cmar.s157925
Denisenko, T. V., Budkevich, I. N., and Zhivotovsky, B. (2018). Cell Death-Based Treatment of Lung Adenocarcinoma. Cell Death Dis 9 (2), 117. doi:10.1038/s41419-017-0063-y
Do, D. T., and Le, N. Q. K. (2021). Using Extreme Gradient Boosting to Identify Origin of Replication in Saccharomyces cerevisiae via Hybrid Features. Genomics. 112, 2445–2451. doi:10.1016/j.ygeno.2020.01.017
Edwards, N. J., Oberti, M., Thangudu, R. R., Cai, S., McGarvey, P. B., Jacob, S., et al. (2015). The CPTAC Data Portal: A Resource for Cancer Proteomics Research. J. Proteome Res. 14 (6), 2707–2713. doi:10.1021/pr501254j
Fu, H., Gao, H., Qi, X., Zhao, L., Wu, D., Bai, Y., et al. (2018). Aldolase A Promotes Proliferation and G1/S Transition via the EGFR/MAPK Pathway in Non-small Cell Lung Cancer. Cancer Commun. 38 (1), 18. doi:10.1186/s40880-018-0290-3
Garber, M. E., Troyanskaya, O. G., Schluens, K., Petersen, S., Thaesler, Z., Pacyna-Gengelbach, M., et al. (2001). Diversity of Gene Expression in Adenocarcinoma of the Lung. Proc. Natl. Acad. Sci. 98 (24), 13784–13789. doi:10.1073/pnas.241500798
Hanna, N., Johnson, D., Temin, S., Baker, S., Brahmer, J., Ellis, P. M., et al. (2017). Systemic Therapy for Stage IV Non-small-cell Lung Cancer: American Society of Clinical Oncology Clinical Practice Guideline Update. Jco 35 (30), 3484–3515. doi:10.1200/jco.2017.74.6065
Hou, J., Aerts, J., den Hamer, B., van IJcken, W., den Bakker, M., Riegman, P., et al. (2010). Gene Expression-Based Classification of Non-small Cell Lung Carcinomas and Survival Prediction. PLoS One 5 (4), e10312. doi:10.1371/journal.pone.0010312
Hu, L.-j., Chen, Y.-q., Deng, S.-b., Du, J.-l., and She, Q. (2013). Additional Use of an Aldosterone Antagonist in Patients with Mild to Moderate Chronic Heart Failure: a Systematic Review and Meta-Analysis. Br. J. Clin. Pharmacol. 75 (5), 1202–1212. doi:10.1111/bcp.12012
Huang, Z., Hua, Y., Tian, Y., Qin, C., Qian, J., Bao, M., et al. (2018). High Expression of Fructose-Bisphosphate Aldolase A Induces Progression of Renal Cell Carcinoma. Oncol. Rep. 39 (6), 2996–3006. doi:10.3892/or.2018.6378
Jiang, Z., Wang, X., Li, J., Yang, H., and Lin, X. (2018). Aldolase A as a Prognostic Factor and Mediator of Progression via Inducing Epithelial-Mesenchymal Transition in Gastric Cancer. J. Cel. Mol. Med. 22 (9), 4377–4386. doi:10.1111/jcmm.13732
Landi, M. T., Dracheva, T., Rotunno, M., Figueroa, J. D., Liu, H., Dasgupta, A., et al. (2008). Gene Expression Signature of Cigarette Smoking and its Role in Lung Adenocarcinoma Development and Survival. PLoS One 3 (2), e1651. doi:10.1371/journal.pone.0001651
Le, N. Q. K., Hung, T. N. K., Do, D. T., Lam, L. H. T., Dang, L. H., and Huynh, T.-T. (2021). Radiomics-based Machine Learning Model for Efficiently Classifying Transcriptome Subtypes in Glioblastoma Patients from MRI. Comput. Biol. Med. 32, 104320, 1879-0534 (Electronic). doi:10.1016/j.compbiomed.2021.104320
Lei, X., Lei, Y., Li, J.-K., Du, W.-X., Li, R.-G., Yang, J., et al. (2020). Immune Cells within the Tumor Microenvironment: Biological Functions and Roles in Cancer Immunotherapy. Cancer Lett. 470, 126–133. doi:10.1016/j.canlet.2019.11.009
Li, J., Wang, F., Gao, H., Huang, S., Cai, F., and Sun, J. (2019). ALDOLASE A Regulates Invasion of Bladder Cancer Cells via E‐cadherin‐EGFR Signaling. J. Cel Biochem 120 (8), 13694–13705. doi:10.1002/jcb.28642
Li, T., Fu, J., Zeng, Z., Cohen, D., Li, J., Chen, Q., et al. (2020). TIMER2.0 for Analysis of Tumor-Infiltrating Immune Cells. Nucleic Acids Res. 48 (W1), W509–w514. doi:10.1093/nar/gkaa407
Liu, X., Wu, S., Yang, Y., Zhao, M., Zhu, G., and Hou, Z. (2017). The Prognostic Landscape of Tumor-Infiltrating Immune Cell and Immunomodulators in Lung Cancer. Biomed. Pharmacother. 95, 55–61. doi:10.1016/j.biopha.2017.08.003
Marcišauskas, S., Ulfenborg, B., Kristjansdottir, B., Waldemarson, S., and Sundfeldt, K. (2019). Univariate and Classification Analysis Reveals Potential Diagnostic Biomarkers for Early Stage Ovarian Cancer Type 1 and Type 2. J. Proteomics 196, 57–68. doi:10.1016/j.jprot.2019.01.017
Nagy, Á., Munkácsy, G., and Győrffy, B. (2021). Pancancer Survival Analysis of Cancer Hallmark Genes. Sci. Rep. 11 (1), 6047. doi:10.1038/s41598-021-84787-5
Okayama, H., Kohno, T., Ishii, Y., Shimada, Y., Shiraishi, K., Iwakawa, R., et al. (2012). Identification of Genes Upregulated in ALK-Positive and EGFR/KRAS/ALK-negative Lung Adenocarcinomas. Cancer Res. 72 (1), 100–111. doi:10.1158/0008-5472.can-11-1403
Pagès, F., Galon, J., Dieu-Nosjean, M.-C., Tartour, E., Sautès-Fridman, C., and Fridman, W.-H. (2010). Immune Infiltration in Human Tumors: a Prognostic Factor that Should Not Be Ignored. Oncogene 29 (8), 1093–1102. doi:10.1038/onc.2009.416
Pan, Y., Sha, Y., Wang, H., Zhuang, H., Ren, X., Zhu, X., et al. (2020). Comprehensive Analysis of the Association between Tumor-Infiltrating Immune Cells and the Prognosis of Lung Adenocarcinoma. J. Cancer Res. Ther. 16 (2), 320–326. doi:10.4103/jcrt.JCRT_954_19
Peng, K., Zhuo, M., Li, M., Chen, Q., Mo, P., and Yu, C. (2020). Histone Demethylase JMJD2D Activates HIF1 Signaling Pathway via Multiple Mechanisms to Promote Colorectal Cancer Glycolysis and Progression. Oncogene 39 (47), 7076–7091. doi:10.1038/s41388-020-01483-w
Rankin, E. B., and Giaccia, A. J. (2016). Hypoxic Control of Metastasis. Science 352 (6282), 175–180. doi:10.1126/science.aaf4405
Rhodes, D. R., Kalyana-Sundaram, S., Mahavisno, V., Varambally, R., Yu, J., Briggs, B. B., et al. (2007). Oncomine 3.0: Genes, Pathways, and Networks in a Collection of 18,000 Cancer Gene Expression Profiles. Neoplasia 9 (2), 166–180. doi:10.1593/neo.07112
Robin, X., Turck, N., Hainard, A., Tiberti, N., Lisacek, F., Sanchez, J.-C., et al. (2011). pROC: an Open-Source Package for R and S+ to Analyze and Compare ROC Curves. BMC Bioinformatics 12, 77. doi:10.1186/1471-2105-12-77
Saito, Y., Takasawa, A., Takasawa, K., Aoyama, T., Akimoto, T., Ota, M., et al. (2020). Aldolase A Promotes Epithelial‐mesenchymal Transition to Increase Malignant Potentials of Cervical Adenocarcinoma. Cancer Sci. 111 (8), 3071–3081. doi:10.1111/cas.14524
Selamat, S. A., Chung, B. S., Girard, L., Zhang, W., Zhang, Y., Campan, M., et al. (2012). Genome-scale Analysis of DNA Methylation in Lung Adenocarcinoma and Integration with mRNA Expression. Genome Res. 22 (7), 1197–1211. doi:10.1101/gr.132662.111
Shannon, P., Markiel, A., Ozier, O., Baliga, N. S., Wang, J. T., Ramage, D., et al. (2003). Cytoscape: a Software Environment for Integrated Models of Biomolecular Interaction Networks. Genome Res. 13 (11), 2498–2504. doi:10.1101/gr.1239303
Stearman, R. S., Dwyer-Nield, L., Zerbe, L., Blaine, S. A., Chan, Z., Bunn, P. A., et al. (2005). Analysis of Orthologous Gene Expression between Human Pulmonary Adenocarcinoma and a Carcinogen-Induced Murine Model. Am. J. Pathol. 167 (6), 1763–1775. doi:10.1016/s0002-9440(10)61257-6
Su, L.-J., Chang, C.-W., Wu, Y.-C., Chen, K.-C., Lin, C.-J., Liang, S.-C., et al. (2007). Selection of DDX5 as a Novel Internal Control for Q-RT-PCR from Microarray Data Using a Block Bootstrap Re-sampling Scheme. BMC Genomics 8, 140. doi:10.1186/1471-2164-8-140
Szklarczyk, D., Gable, A. L., Lyon, D., Junge, A., Wyder, S., Huerta-Cepas, J., et al. (2019). STRING V11: Protein-Protein Association Networks with Increased Coverage, Supporting Functional Discovery in Genome-wide Experimental Datasets. Nucleic Acids Res. 47 (D1), D607–d613. doi:10.1093/nar/gky1131
Tang, Y., Yang, X., Feng, K., Hu, C., and Li, S. (2021). High Expression of Aldolase A Is Associated with Tumor Progression and Poor Prognosis in Hepatocellular Carcinoma. J. Gastrointest. Oncol. 12 (1), 174–183. doi:10.21037/jgo-20-534
Tang, Z., Kang, B., Li, C., Chen, T., and Zhang, Z. (2019). GEPIA2: an Enhanced Web Server for Large-Scale Expression Profiling and Interactive Analysis. Nucleic Acids Res. 47 (W1), W556–W560. doi:10.1093/nar/gkz430
Tochio, T., Tanaka, H., Nakata, S., and Hosoya, H. (2010). Fructose-1,6-bisphosphate Aldolase A Is Involved in HaCaT Cell Migration by Inducing Lamellipodia Formation. J. Dermatol. Sci. 58 (2), 123–129. doi:10.1016/j.jdermsci.2010.02.012
Tomczak, K., Czerwińska, P., and Wiznerowicz, M. (2015). The Cancer Genome Atlas (TCGA): an Immeasurable Source of Knowledge. Contemp. Oncol. (Pozn) 19 (1a), A68–A77. doi:10.5114/wo.2014.47136
Travis, W. D., Brambilla, E., Nicholson, A. G., Yatabe, Y., Austin, J. H. M., Beasley, M. B., et al. (2015). The 2015 World Health Organization Classification of Lung Tumors. J. Thorac. Oncol. 10 (9), 1243–1260. doi:10.1097/jto.0000000000000630
Uhlén, M., Fagerberg, L., Hallström, B. M., Lindskog, C., Oksvold, P., Mardinoglu, A., et al. (2015). Proteomics. Tissue-Based Map of the Human Proteome. Science 347 (6220), 1260419. doi:10.1126/science.1260419
Uhlen, M., Zhang, C., Lee, S., Sjöstedt, E., Fagerberg, L., Bidkhori, G., et al. (2017). A Pathology Atlas of the Human Cancer Transcriptome. Science 357 (6352). doi:10.1126/science.aan2507
Wang, S.-s., Liu, W., Ly, D., Xu, H., Qu, L., and Zhang, L. (2019). Tumor-infiltrating B Cells: Their Role and Application in Anti-tumor Immunity in Lung Cancer. Cell Mol Immunol 16 (1), 6–18. doi:10.1038/s41423-018-0027-x
Xu, X., Huang, Z., Zheng, L., and Fan, Y. (2018). The Efficacy and Safety of Anti-PD-1/pd-L1 Antibodies Combined with Chemotherapy or CTLA4 Antibody as a First-Line Treatment for Advanced Lung Cancer. Int. J. Cancer 142 (11), 2344–2354. doi:10.1002/ijc.31252
Yu, G., Wang, L.-G., Han, Y., and He, Q.-Y. (2012). clusterProfiler: an R Package for Comparing Biological Themes Among Gene Clusters. OMICS: A J. Integr. Biol. 16 (5), 284–287. doi:10.1089/omi.2011.0118
Zeng, Y., Lv, Y., Tao, L., Ma, J., Zhang, H., Xu, H., et al. (2016). G6PC3, ALDOA and CS Induction Accompanies Mir-122 Down-Regulation in the Mechanical Asphyxia and Can Serve as Hypoxia Biomarkers. Oncotarget 7 (46), 74526–74536. doi:10.18632/oncotarget.12931
Zhang, F., Lin, J.-D., Zuo, X.-Y., Zhuang, Y.-X., Hong, C.-Q., Zhang, G.-J., et al. (2017). Elevated Transcriptional Levels of Aldolase A (ALDOA) Associates with Cell Cycle-Related Genes in Patients with NSCLC and Several Solid Tumors. Bio Data Mining 10. doi:10.1186/s13040-016-0122-4
Zhang, L., Zhang, Z., and Yu, Z. (2019). Identification of a Novel Glycolysis-Related Gene Signature for Predicting Metastasis and Survival in Patients with Lung Adenocarcinoma. J. Transl Med. 17 (1), 423. doi:10.1186/s12967-019-02173-2
Keywords: lung adenocarcinoma, AldoA, biomarker, prognosis, immune infiltration
Citation: Lu G, Shi W and Zhang Y (2021) Prognostic Implications and Immune Infiltration Analysis of ALDOA in Lung Adenocarcinoma. Front. Genet. 12:721021. doi: 10.3389/fgene.2021.721021
Received: 05 June 2021; Accepted: 28 October 2021;
Published: 03 December 2021.
Edited by:
Rosalba Giugno, University of Verona, ItalyReviewed by:
Cheng Liang, Shandong Normal University, ChinaKhanh N. Q. Le, Taipei Medical University, Taiwan
Copyright © 2021 Lu, Shi and Zhang. This is an open-access article distributed under the terms of the Creative Commons Attribution License (CC BY). The use, distribution or reproduction in other forums is permitted, provided the original author(s) and the copyright owner(s) are credited and that the original publication in this journal is cited, in accordance with accepted academic practice. No use, distribution or reproduction is permitted which does not comply with these terms.
*Correspondence: Yu Zhang, emhhbmd5dTIxMTNfbmpAMTYzLmNvbQ==