- 1Department of Respiratory and Critical Care Medicine, Affiliated Central Hospital, Shenyang Medical College, Shenyang, China
- 2Department of Pathogen Biology, Shenyang Medical College, Shenyang, China
Asthma is a common chronic respiratory disease. In the past 10 years, genome-wide association study (GWAS) has been widely used to identify the common asthma genetic variants. Importantly, these publicly available asthma GWAS datasets provide important data support to investigate the causal association of kinds of risk factors with asthma by a Mendelian randomization (MR) design. It is known that socioeconomic status is associated with asthma. However, it remains unclear about the causal association between socioeconomic status and asthma. Here, we selected 162 independent educational attainment genetic variants as the potential instruments to evaluate the causal association between educational attainment and asthma using large-scale GWAS datasets of educational attainment (n = 405,072) and asthma (n = 30,810). We conducted a pleiotropy analysis using the MR-Egger intercept test and the MR pleiotropy residual sum and outlier (MR-PRESSO) test. We performed an MR analysis using inverse-variance weighted, weighted median, MR-Egger, and MR-PRESSO. The main analysis method inverse-variance weighted indicated that each 1 standard deviation increase in educational attainment (3.6 years) could reduce 35% asthma risk [odds ratio (OR) = 0.65, 95% confidence interval (CI) 0.51–0.85, P = 0.001]. Importantly, evidence from other MR methods further supported this finding, including weighted median (OR = 0.55, 95% CI 0.38–0.80, P = 0.001), MR-Egger (OR = 0.48, 95% CI 0.16–1.46, P = 0.198), and MR-PRESSO (OR = 0.65, 95% CI 0.51–0.85, P = 0.0015). Meanwhile, we provide evidence to support that educational attainment protects against asthma risk dependently on cognitive performance using multivariable MR analysis. In summary, we highlight the protective role of educational attainment against asthma. Our findings may have public health applications and deserve further investigation.
Introduction
Asthma is a common chronic respiratory disease (Beasley et al., 2015; Han et al., 2020; von Mutius and Smits, 2020). It is estimated that asthma could affect over 300 million people in the world and result in a substantial burden (Beasley et al., 2015; Han et al., 2020; von Mutius and Smits, 2020). During the past 30 years, asthma death rates have decreased greatly (Beasley et al., 2015; von Mutius and Smits, 2020). However, there are still no effective therapeutic regimens (Beasley et al., 2015; von Mutius and Smits, 2020). Hence, it is important to identify the risk factors for asthma, especially those with the causation association for asthma (Beasley et al., 2015; von Mutius and Smits, 2020).
In the past 10 years, genome-wide association study (GWAS) has been widely used to identify the common asthma genetic variants (Demenais et al., 2018; Zhu et al., 2018; Shrine et al., 2019; Han et al., 2020). In 2018, the Trans-National Asthma Genetic Consortium (TAGC) conducted a GWAS analysis of asthma using 23,948 cases and 118,538 controls from kinds of populations, including European, African, Japanese, and Latino ancestries (von Mutius and Smits, 2020). They successfully found five new asthma loci (Demenais et al., 2018). Zhu et al. (2018) conducted a genome-wide cross-trait analysis of asthma and allergic diseases using large-scale GWAS datasets from the UK Biobank, including 33,593 cases and 76,768 controls of European ancestry. They found a significant genetic correlation between asthma and allergic diseases and highlighted 38 shared loci (Zhu et al., 2018). Shrine et al. (2019) carried out a GWAS analysis to identify common genetic variants associated with moderate-to-severe asthma by a two-stage design, including 5,135 asthma cases and 25,675 controls in stage 1 and 5,414 asthma cases and 21,471 controls in stage 2. Importantly, all these selected individuals are of European ancestry (Shrine et al., 2019). Interestingly, they reported 24 novel genetic variants to be significantly associated with moderate-to-severe asthma (Shrine et al., 2019). Han et al. (2020) conducted a GWAS analysis of asthma using 64,538 asthma cases and 329,321 controls from the UK Biobank. They further performed an asthma GWAS meta-analysis of the UK Biobank and the TAGC (Demenais et al., 2018; Han et al., 2020). Finally, Han et al. identified 66 novel asthma loci (Demenais et al., 2018; von Mutius and Smits, 2020).
Importantly, these publicly available asthma GWAS datasets provide important data support to investigate the causal association of kinds of risk factors with asthma by Mendelian randomization (MR) design or polygenic score (Granell et al., 2014; Minelli et al., 2018; Skaaby et al., 2018; Rosa et al., 2019; Xu et al., 2019; Zhao and Schooling, 2019; Chen et al., 2020; Mulugeta et al., 2020; Shen et al., 2020; Sun et al., 2020; Au Yeung et al., 2021a; Park et al., 2021; Raita et al., 2021). Some risk factors have been reported to increase the risk of asthma, including soluble interleukin-6 receptor level (Rosa et al., 2019; Raita et al., 2021), childhood body mass index (BMI) (Au Yeung et al., 2021a), adult BMI (Granell et al., 2014; Skaaby et al., 2018; Xu et al., 2019; Sun et al., 2020; Au Yeung et al., 2021a), major depressive disorder (Mulugeta et al., 2020), early pubertal maturation (Chen et al., 2020), and age at puberty (Minelli et al., 2018). Meanwhile, other risk factors are associated with reduced risk of asthma, including estimated glomerular filtration rate (Park et al., 2021), lifetime smoking (Shen et al., 2020), and linoleic acid (Zhao and Schooling, 2019).
In addition to these risk factors discussed earlier, socioeconomic status is also associated with asthma (Eagan et al., 2004; Hancox et al., 2004; Kozyrskyj et al., 2010; Brite et al., 2020). However, it remains unclear about the causal association between socioeconomic status and asthma (Eagan et al., 2004; Hancox et al., 2004; Kozyrskyj et al., 2010; Brite et al., 2020). Here, we selected 162 independent educational attainment genetic variants as the potential instruments to evaluate the causal association between educational attainment and asthma.
Materials and Methods
Educational Attainment Genome-Wide Association Study Dataset
We selected 162 independent genetic variants that influence educational attainment to be the potential instrumental variables (Okbay et al., 2016). In brief, these genetic variants are identified by a recent large-scale GWAS dataset of educational attainment in individuals of European descent (n = 405,072) (Okbay et al., 2016). The educational attainment was a continuous variable measuring by the number of years of schooling completed (EduYears) and was assessed at age or older than 30 years (Okbay et al., 2016). This large-scale GWAS dataset is based on the meta-analysis of GWAS results from the discovery stage (Social Science Genetic Association Consortium, including 293,723 individuals) and replication stage (UK Biobank, including 111,349 individuals) (Okbay et al., 2016). The participating cohorts in the discovery stage are provided in Table 1. Finally, this meta-analysis identified 162 independent genetic variants with the genome-wide significance (P < 5.00E-08), as provided in Supplementary Table 1 (Okbay et al., 2016).
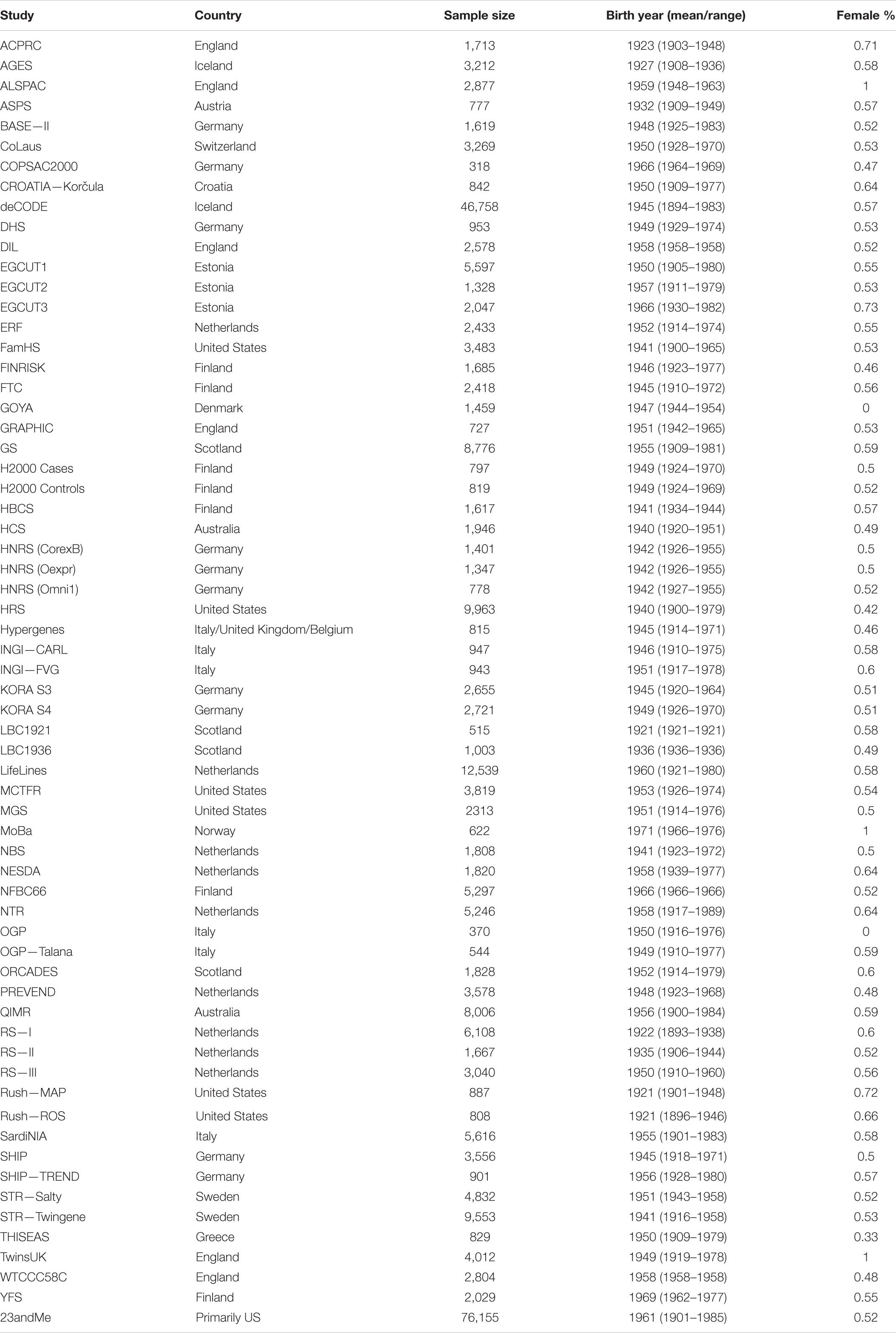
Table 1. Participating cohorts in Educational attainment GWAS discovery stage (Okbay et al., 2016).
Cognitive Performance Genome-Wide Association Study Dataset
We selected a large-scale GWAS dataset of cognitive performance in 257,841 individuals of European descent (Okbay et al., 2016). It is based on the sample-size-weighted meta-analysis of two large-scale GWAS datasets from the COGENT consortium (n = 35,298) and UK Biobank (n = 222,543) (Okbay et al., 2016). In COGENT, the phenotype measure was the first unrotated principal component of performance on at least three neuropsychological tests (or at least two IQ-test subscales) (Okbay et al., 2016). In the UK Biobank, the phenotype measure was a standardized score on a test of verbal–numerical reasoning (Okbay et al., 2016). More detailed information is provided in the original study (Okbay et al., 2016).
Asthma Genome-Wide Association Study Dataset
We selected the large-scale asthma GWAS dataset in 30,810 individuals of European ancestry, including 5,135 moderate–severe asthma cases and 25,675 controls, as described in the original study (Shrine et al., 2019). These selected moderate–severe asthma cases are from the Genetics of Asthma Severity and Phenotypes study (GASP, n = 1,858), the Unbiased Biomarkers in Prediction of respiratory disease outcomes project (U-BIOPRED, n = 281), and the UK Biobank (n = 2,996) (Shrine et al., 2019). The selected controls are from the U-BIOPRED (n = 75) and the UK Biobank (n = 25,600) (Shrine et al., 2019). In GASP and U-BIOPRED, moderate-to-severe asthma patients were evaluated using clinical records based on the British Thoracic Society 2014 guidelines (Shrine et al., 2019). In the UK Biobank, moderate-to-severe asthma cases were diagnosed by a doctor (Shrine et al., 2019). The key demographic characteristics, including age and sex, are provided in Table 2 or the original study (Shrine et al., 2019).
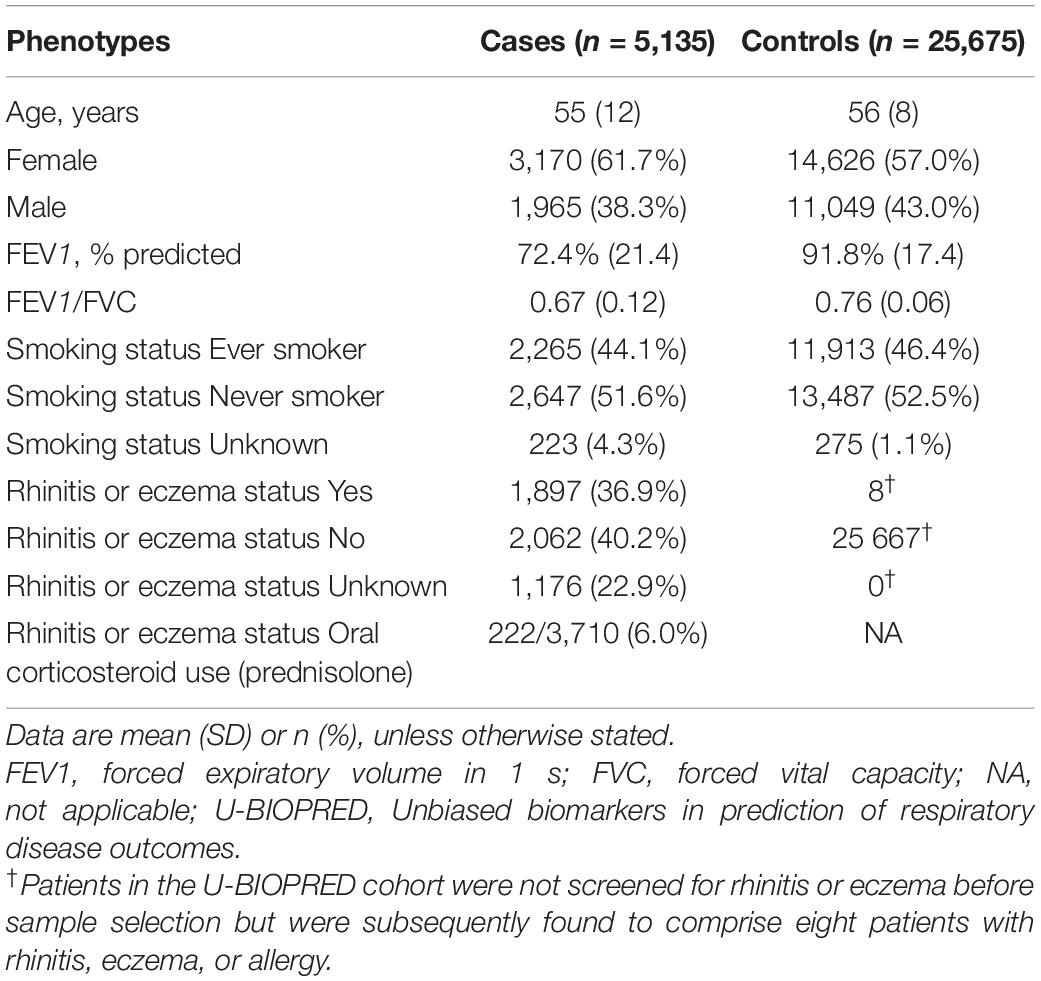
Table 2. Baseline characteristics of asthma cases and controls (Shrine et al., 2019).
Pleiotropy Analysis
MR is established based on three key assumptions. Assumption 1: genetic variants (instrumental variables) should be significantly associated with the exposure (educational attainment). Hence, we selected 162 independent genetic variants associated with educational attainment with the genome-wide significance (P < 5.00E-08), as described earlier. Both assumption 2 and assumption 3 are known as no pleiotropy, as described in recent MR studies (Larsson et al., 2020; Au Yeung et al., 2021b; Sun et al., 2021; Yuan et al., 2021; Zhao and Schooling, 2021; Zhuang et al., 2021b). Hence, we conducted a pleiotropy analysis using the MR-Egger intercept test (Bowden et al., 2015; Burgess and Thompson, 2017) and the MR pleiotropy residual sum and outlier (MR-PRESSO) test (Verbanck et al., 2018); both have widely used in recent MR studies (Larsson et al., 2020; Au Yeung et al., 2021b; Sun et al., 2021; Yuan et al., 2021; Zhao and Schooling, 2021; Zhuang et al., 2021b). The significance threshold P < 0.05 indicated evidence of pleiotropy.
Mendelian Randomization Analysis
For univariable MR analysis, we selected the inverse-variance weighted (IVW) as the main MR analysis method. Meanwhile, we also selected other additional MR analysis methods, including weighted median, MR-Egger method, and MR-PRESSO, as used in recent MR studies (Bowden et al., 2015; Burgess and Thompson, 2017; Liu et al., 2018; Larsson et al., 2020; Au Yeung et al., 2021b; Sun et al., 2021; Yuan et al., 2021; Zhao and Schooling, 2021; Zhuang et al., 2021b). For multivariable MR analysis, we selected the multivariable IVW method, multivariable median-based method, and multivariable MR-Egger method. The odds ratio (OR) and 95% confidence interval (CI) of asthma correspond to approximately per 3.6 years increase [approximately 1 standard deviation (SD)] in EduYears. R (version x64 4.0.3), R package “MendelianRandomization,” and MR-PRESSO were used to perform the MR analysis. The significance threshold P < 0.05 indicated evidence of causal association. To test the influence of a single genetic variant, we also conducted a sensitivity analysis using leave-one-out permutation (Liu et al., 2018).
Power Analysis
The variance of educational attainment (R2) explained by the selected genetic variants was calculated using the effect allele frequency, the effect size beta (β), and the number of the selected genetic variants (k), as described in a previous study (Locke et al., 2015).
Based on the R2 and other necessary information, including sample size, type-I error rate, proportion of cases in the study, and true OR of the outcome variable per SD of the exposure variable, the statistical power was calculated using mRnd (Power calculations for MR) (Brion et al., 2013).
Results
Educational Attainment Genetic Variants and Asthma
We selected 162 independent genetic variants influencing educational attainment and extracted their corresponding summary statistics in the asthma GWAS dataset. The results showed that 141 unique genetic variants were available in the asthma GWAS dataset. Only five genetic variants are associated with asthma risk with P < 0.05, including rs1378214 (P = 0.000531), rs76878669 (P = 0.00607), rs7772172 (P = 0.00666), rs113520408 (P = 0.0183), and rs9556958 (P = 0.0388). These findings indicated that all these selected genetic variants showed a more significant trend associated with educational attainment. Table 3 provides the more detailed results about these 141 genetic variants.
Pleiotropy Analysis
Evidence from the MR-Egger intercept test supported that these 141 genetic variants showed no significant pleiotropy with intercept = 0.005, P = 0.581. Importantly, evidence from the MR-PRESSO global test further highlighted no significant horizontal pleiotropy P = 0.375. Hence, these 141 genetic variants could be selected as the effective instrumental variables.
Univariable Mendelian Randomization Analysis
The main analysis method IVW indicated that each 1 SD increase in educational attainment (3.6 years) could reduce 35% asthma risk (OR = 0.65, 95% CI 0.51–0.85, P = 0.001). Importantly, evidence from other MR methods further supported this finding, including weighted median (OR = 0.55, 95% CI 0.38–0.80, P = 0.001), MR-Egger (OR = 0.48, 95% CI 0.16–1.46, P = 0.198), and MR-PRESSO (OR = 0.65, 95% CI 0.51–0.85, P = 0.0015). Figures 1–3 show the individual causal estimates using the IVW method, weighted median, and MR-Egger, respectively. We further conduct a sensitivity analysis using the leave-one-out permutation. The results suggested no single genetic variant to significantly affect the estimates between educational attainment and the risk of asthma.
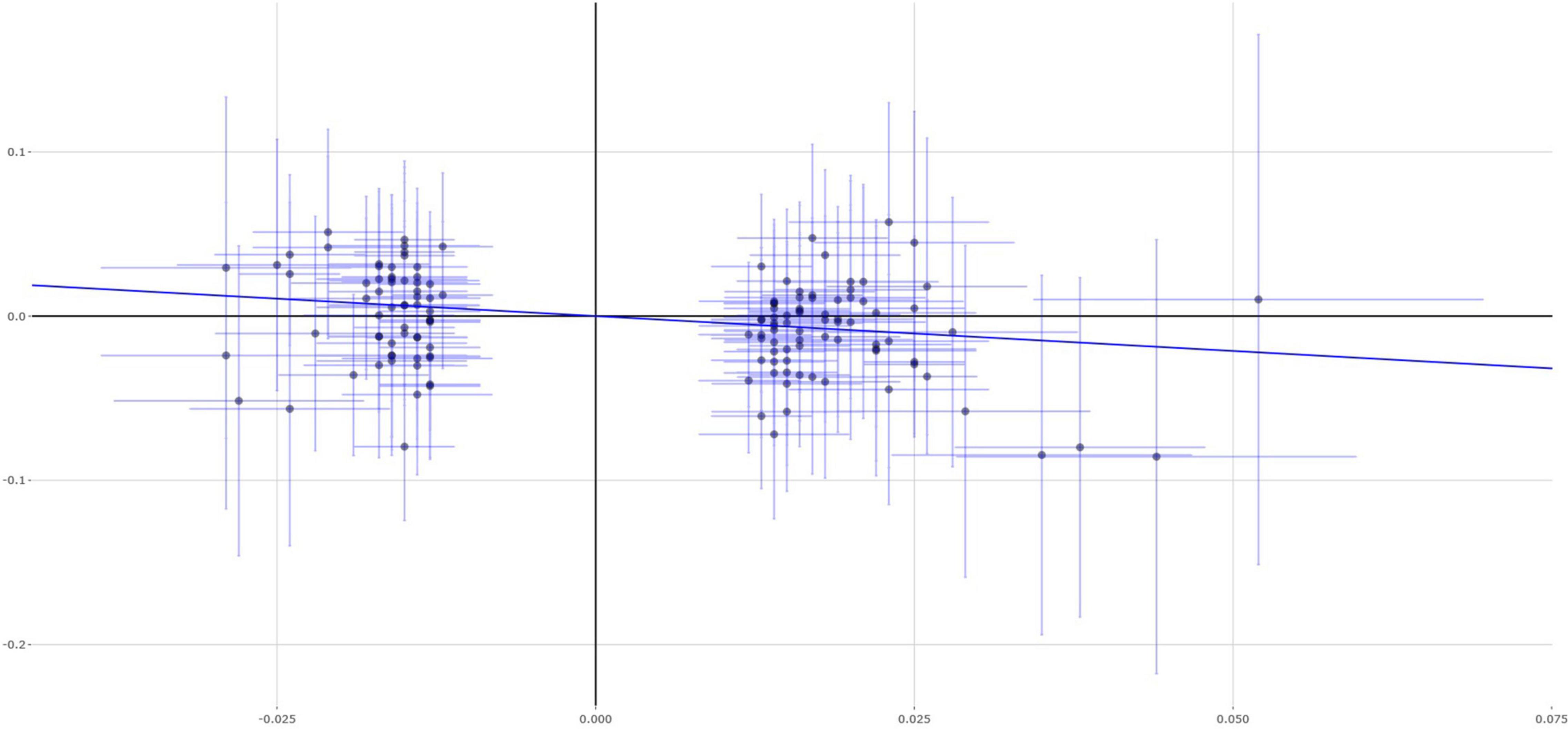
Figure 1. Single estimates about causal association between educational attainment and asthma from MR analysis using IVW method. This scatter plots represent 141 genetic variants associated with educational attainment on x-axis and risk of asthma on y-axis. Continuous line represents causal effect of educational attainment on risk of asthma. IVW, inverse variance weighted.
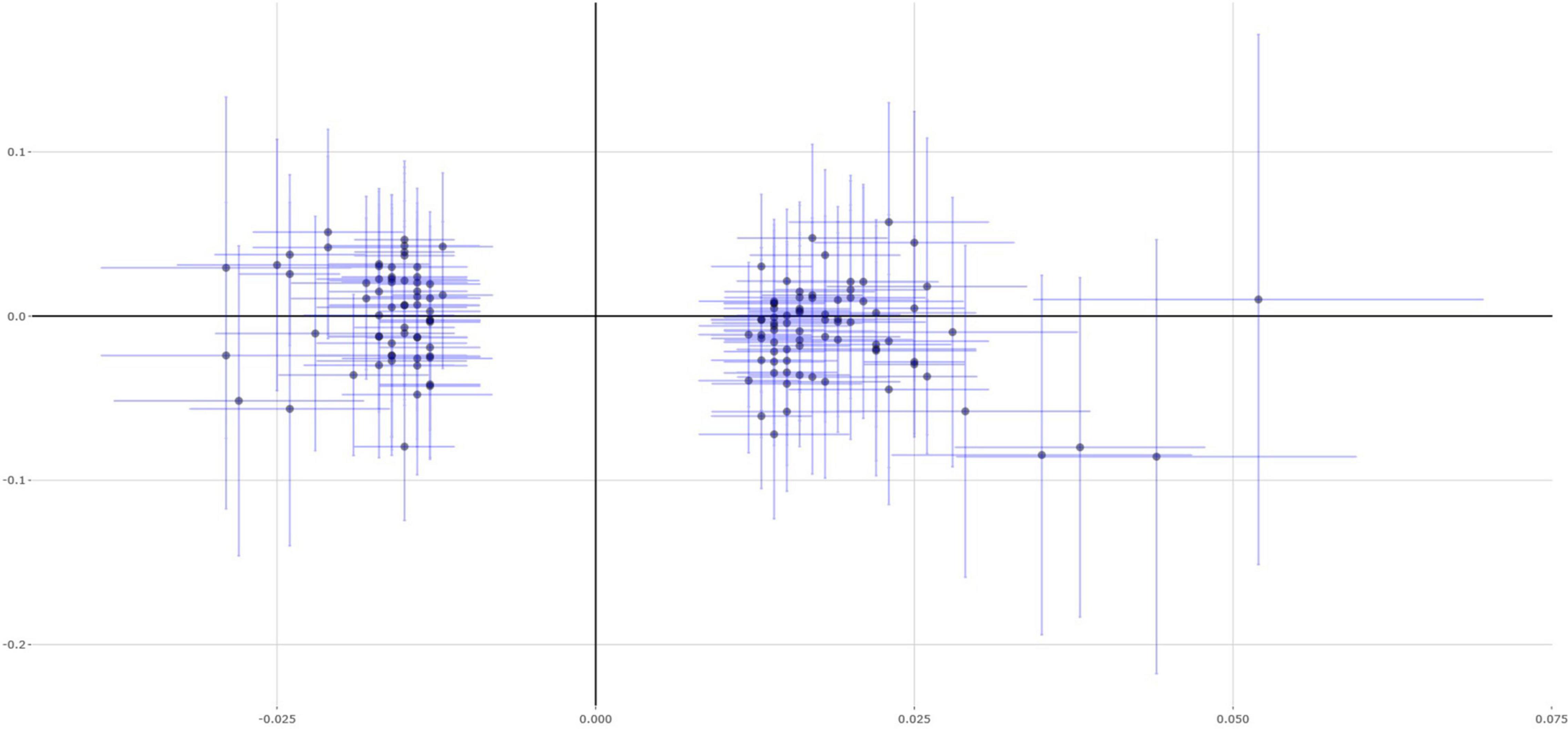
Figure 2. Single estimates about causal association between educational attainment and asthma from MR analysis using weighted median method. This scatter plots represent 141 genetic variants associated with educational attainment on x-axis and risk of asthma on y-axis. Continuous line represents causal effect of educational attainment on risk of asthma.
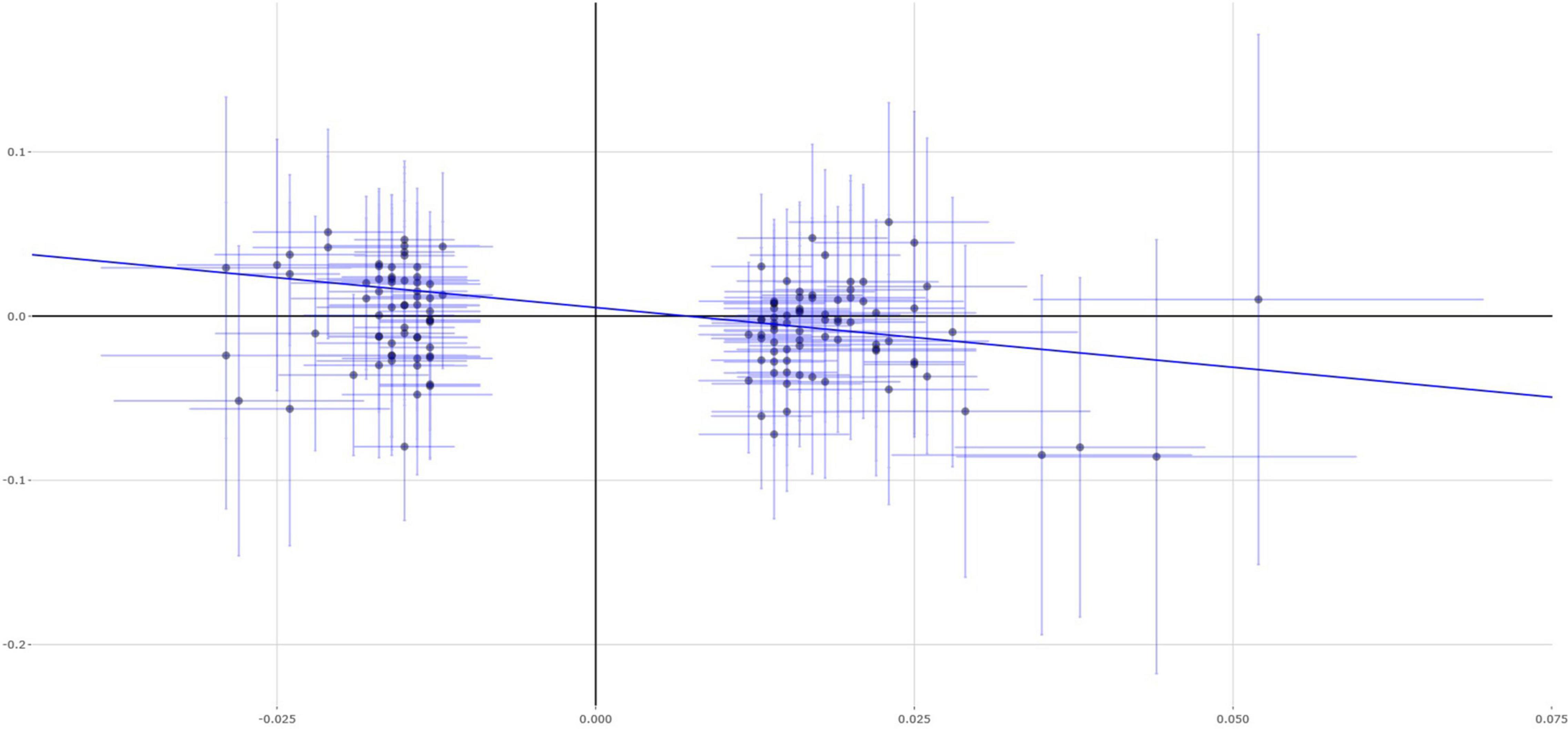
Figure 3. Single estimates about causal association between educational attainment and asthma from MR analysis using MR-Egger method. This scatter plots represent 141 genetic variants associated with educational attainment on x-axis and risk of asthma on y-axis. Continuous line represents causal effect of educational attainment on risk of asthma.
Multivariable Mendelian Randomization Analysis
In multivariable MR analysis, we evaluated the effect of cognitive performance on the causal association between educational attainment and the risk of asthma. However, all three multivariable MR analysis methods indicated no significant causal association between educational attainment and the risk of asthma, including multivariable IVW method (OR = 0.64, 95% CI 0.35–1.17, P = 0.144), multivariable median-based method (OR = 0.63, 95% CI 0.28–1.42, P = 0.265), and multivariable MR-Egger method (OR = 0.32, 95% CI 0.08–1.22, P = 0.094). Hence, these findings provide evidence to support that educational attainment protects against asthma risk dependently on cognitive performance.
Power Analysis
One hundred forty-one educational attainment genetic variants finally selected in our MR analysis explain a total of 3.87% of educational attainment variance. Power analysis using mRnd showed that our MR analysis had 80% power to detect OR of 0.79 or lower per SD increase in educational attainment for the risk of asthma. Meanwhile, our MR analysis has 100% power to detect the OR of 0.65 using IVW, the OR of 0.55 using weighted median, the OR of 0.48 using MR-Egger, and the OR of 0.65 using MR-PRESSO.
Discussion
Until recently, multiple large-scale GWAS analyses have been conducted to report novel asthma genetic variants (Demenais et al., 2018; Zhu et al., 2018; Shrine et al., 2019; Han et al., 2020). Importantly, these GWAS datasets are publicly available and promote additional analyses, such as MR analysis, to evaluate the causal association between common risk factors and asthma. These risk factors include soluble interleukin-6 receptor level (Rosa et al., 2019; Raita et al., 2021), childhood BMI (Au Yeung et al., 2021a), adult BMI (Granell et al., 2014; Skaaby et al., 2018; Xu et al., 2019; Sun et al., 2020; Au Yeung et al., 2021a), major depressive disorder (Mulugeta et al., 2020), early pubertal maturation (Chen et al., 2020), age at puberty (Minelli et al., 2018), estimated glomerular filtration rate (Park et al., 2021), lifetime smoking (Shen et al., 2020), and linoleic acid (Zhao and Schooling, 2019).
It is reported that socioeconomic status is also a risk factor for asthma (Eagan et al., 2004; Hancox et al., 2004; Kozyrskyj et al., 2010; Brite et al., 2020). In the World Trade Center Health Registry study, Brite et al. (2020) analyzed the data from 30,452 individuals and found that individuals with lower socioeconomic status had worse asthma outcomes. In the Western Australian Pregnancy Cohort (Raine) Study, Kozyrskyj et al. (2010) analyzed the data from 2,868 children and found that children with lower socioeconomic status tended to develop persistent asthma. However, Hancox et al. (2004) reported inconsistent findings in a prospective cohort study including approximately 1,000 individuals in New Zealand. They found no significant between socioeconomic status during childhood and the prevalence of asthma (Hancox et al., 2004). Hence, the causal association between socioeconomic status and asthma remains unclear, which further promotes us to perform an MR analysis using the large-scale GWAS datasets.
Using 162 independent educational attainment genetic variants, we successfully extracted the summary association results of 141 unique genetic variants from the asthma GWAS dataset. The pleiotropy analysis indicated these genetic variants to be effective instruments. MR analysis showed each 1 SD increase in educational attainment (4.2 years) reduced 35% asthma risk (OR = 0.65, 95% CI 0.51–0.85, P = 0.001) using IVW. Importantly, other additional analysis methods and sensitivity methods supported this finding. However, multivariable MR analysis showed that educational attainment protected against asthma risk dependently on cognitive performance.
Until now, univariable and multivariable MR studies have evaluated the association of educational attainment and/or cognitive performance on other human complex diseases or phenotypes. Wang et al. (2021) conducted a two-sample univariable and multivariable MR to evaluate the causal effects of educational attainment and cognition on the risk of epilepsy. Using univariable MR analysis, they found that both educational attainment and cognitive performance could reduce the risk of epilepsy (Wang et al., 2021). Using multivariable MR analysis, they found that only educational attainment protected against epilepsy independent of cognitive performance (Wang et al., 2021).
Gill et al. (2019) conducted a two-sample univariable MR to evaluate the effect of education and cognitive performance, respectively, on the risk of coronary heart disease and ischemic stroke. Meanwhile, they performed a multivariable MR to adjust for the effects of cognitive performance and education, respectively (Gill et al., 2019). Using univariable MR analysis, they found a causal association between high education and reduced risk of coronary heart disease and stroke (Gill et al., 2019). Meanwhile, they also found that high cognitive performance could also reduce the risk of coronary heart disease but not stroke (Gill et al., 2019). Using multivariable MR analysis, they found that education could protect against coronary heart disease and stroke independent of cognitive function (Gill et al., 2019). However, the cognitive performance had no causal association with coronary heart disease or stroke by adjusting for education (Gill et al., 2019). Carter et al. (2019) found that BMI, systolic blood pressure, and smoking behavior could mediate the protective role of education on the risk of cardiovascular outcomes, including coronary heart disease, stroke, myocardial infarction, and cardiovascular disease (all subtypes; all measured in OR).
Liang et al. (2021) identified that educational attainment protected against type 2 diabetes independently of cognitive performance. Rosoff et al. (2020) found that educational attainment could reduce the risk of suicide attempts in individuals with and without psychiatric disorders independent of cognition. Zhang et al. (2020) found that high educational attainment, but not cognitive performance, was causally associated with a reduced risk of amyotrophic lateral sclerosis. Meanwhile, MR studies have found that increased education could reduce the risk of ischemic stroke (Gill et al., 2019; Xiuyun et al., 2020; Harshfield et al., 2021) and Alzheimer’s disease (Larsson et al., 2017; Anderson et al., 2020; Andrews et al., 2021; Zhuang et al., 2021a). Anderson et al. (2020) recently examined whether educational attainment and cognitive performance had causal effects on the risk of Alzheimer’s disease, independently of each other. They found that educational attainment affected the risk of Alzheimer’s disease dependently of cognitive performance (Anderson et al., 2020). However, cognitive performance affected the risk of Alzheimer’s disease independently of educational attainment (Anderson et al., 2020).
Hence, all these findings discussed earlier indicated that educational attainment had causal effects on the risk of epilepsy (Wang et al., 2021), coronary heart disease (Gill et al., 2019), stroke (Gill et al., 2019), type 2 diabetes (Liang et al., 2021), and suicide attempt (Rosoff et al., 2020), independently of cognitive performance. However, the causal effect of educational attainment on the risk of Alzheimer’s disease may be mediated by cognitive performance (Anderson et al., 2020). Our findings are consistent with recent MR findings in other human complex diseases or phenotypes.
Since 2018, multiple large-scale asthma GWAS datasets have been reported, as described in the Introduction. Here, we only selected the large-scale asthma GWAS dataset in 30,810 individuals of European ancestry from Shrine et al. (2019). In brief, these GWAS samples are from GASP, U-BIOPRED, and the UK Biobank (Shrine et al., 2019). In 2018, TAGC examined the common asthma variants by a meta-analysis of worldwide asthma GWAS datasets, including 23,948 asthma cases and 118,538 controls (Demenais et al., 2018). However, all these individuals are from ethnically diverse populations, including European ancestry, African ancestry, Japanese ancestry, and Latino ancestry (Demenais et al., 2018). It is known that all these selected educational attainment genetic variants are from the large-scale GWAS dataset in individuals of European descent (n = 405,072) (Okbay et al., 2016). Hence, we did not select the asthma GWAS dataset from TAGC in our MR analysis (Demenais et al., 2018). Zhu et al. (2018) conducted a genome-wide cross-trait analysis to investigate the shared genetic etiology in asthma and allergic diseases by analyzing large-scale GWAS datasets from the UK Biobank, including 25,685 allergic diseases subjects, 14,085 asthma subjects, and 76,768 controls. Hence, both Shrine et al. (2019) and Zhu et al. (2018) have used the UK Biobank samples. Hence, we did not select the asthma GWAS dataset from Zhu et al. (2018) in our MR analysis. Han et al. (2020) conducted a GWAS using 64,538 asthma cases and 329,321 controls from UK Biobank and then performed a meta-analysis using the UK Biobank and the TAGC datasets. However, they did provide the effect size and the corresponding standard error for each variant in the GWAS summary dataset (Han et al., 2020). Importantly, there is a sample overlap in both studies from Shrine et al. (2019) and Han et al. (2020), as both shared the UK Biobank samples. Hence, we did not select the GWAS dataset from the UK Biobank or the GWAS dataset from the meta-analysis of the UK Biobank and TAGC in our MR analysis (Han et al., 2020).
Meanwhile, our MR analysis still has some limitations. First, our findings are based on the educational attainment GWAS dataset and asthma GWAS dataset in individuals of European ancestry (Okbay et al., 2016; Shrine et al., 2019). It remains unclear about the causal association between educational attainment and asthma in other ancestries. Hence, replication MR studies are required to investigate our findings in the future. Second, both the educational attainment GWAS dataset and asthma GWAS dataset include the samples from the UK Biobank (Okbay et al., 2016; Shrine et al., 2019). In brief, the replication stage in the educational attainment GWAS dataset included 111,349 individuals from the UK Biobank (Okbay et al., 2016). The asthma GWAS dataset included 2,996 asthma cases from the UK Biobank and 25,600 controls from the UK Biobank (Shrine et al., 2019). Hence, the educational attainment GWAS dataset and the asthma GWAS dataset may not be independent. Hence, independent GWAS datasets are also required to evaluate our findings further.
In summary, we highlight the protective role of educational attainment against asthma with 100% statistical power using univariable MR analysis. Meanwhile, we provide evidence to support that educational attainment protects against asthma risk dependently on cognitive performance using multivariable MR analysis. Our findings may have public health applications and deserve further investigation.
Data Availability Statement
The original contributions presented in the study are included in the article/Supplementary Material, further inquiries can be directed to the corresponding author/s.
Author Contributions
YL and BY designed the project and analyzed the data. All authors wrote the first draft of the manuscript, revised, and approved the final manuscript.
Conflict of Interest
The authors declare that the research was conducted in the absence of any commercial or financial relationships that could be construed as a potential conflict of interest.
Publisher’s Note
All claims expressed in this article are solely those of the authors and do not necessarily represent those of their affiliated organizations, or those of the publisher, the editors and the reviewers. Any product that may be evaluated in this article, or claim that may be made by its manufacturer, is not guaranteed or endorsed by the publisher.
Acknowledgments
We thank the Social Science Genetic Association Consortium and the UK Biobank for providing the educational attainment GWAS datasets. We also thank GASP, U-BIOPRED, and the UK Biobank for the asthma GWAS datasets.
Supplementary Material
The Supplementary Material for this article can be found online at: https://www.frontiersin.org/articles/10.3389/fgene.2021.716364/full#supplementary-material
References
Anderson, E. L., Howe, L. D., Wade, K. H., Ben-Shlomo, Y., Hill, W. D., Deary, I. J., et al. (2020). Education, intelligence and Alzheimer’s disease: evidence from a multivariable two-sample Mendelian randomization study. Int. J. Epidemiol. 49, 1163–1172. doi: 10.1093/ije/dyz280
Andrews, S. J., Fulton-Howard, B., O’reilly, P., Marcora, E., and Goate, A. M. (2021). Causal associations between modifiable risk factors and the Alzheimer’s phenome. Ann. Neurol. 89, 54–65. doi: 10.1002/ana.25918
Au Yeung, S. L., Li, A. M., and Schooling, C. M. (2021a). A life course approach to elucidate the role of adiposity in asthma risk: evidence from a Mendelian randomisation study. J. Epidemiol. Commun. Health 75, 277–281.
Au Yeung, S. L., Zhao, J. V., and Schooling, C. M. (2021b). Evaluation of glycemic traits in susceptibility to COVID-19 risk: a Mendelian randomization study. BMC Med. 19:72. doi: 10.1186/s12916-021-01944-3
Beasley, R., Semprini, A., and Mitchell, E. A. (2015). Risk factors for asthma: is prevention possible? Lancet 386, 1075–1085. doi: 10.1016/S0140-6736(15)00156-7
Bowden, J., Davey Smith, G., and Burgess, S. (2015). Mendelian randomization with invalid instruments: effect estimation and bias detection through Egger regression. Int. J. Epidemiol. 44, 512–525. doi: 10.1093/ije/dyv080
Brion, M. J., Shakhbazov, K., and Visscher, P. M. (2013). Calculating statistical power in Mendelian randomization studies. Int. J. Epidemiol. 42, 1497–1501. doi: 10.1093/ije/dyt179
Brite, J., Alper, H. E., Friedman, S., Takemoto, E., and Cone, J. (2020). Association between socioeconomic status and asthma-related emergency department visits among world trade center rescue and recovery workers and survivors. JAMA Netw. Open 3:e201600. doi: 10.1001/jamanetworkopen.2020.1600
Burgess, S., and Thompson, S. G. (2017). Interpreting findings from Mendelian randomization using the MR-Egger method. Eur. J. Epidemiol. 32, 377–389. doi: 10.1007/s10654-017-0255-x
Carter, A. R., Gill, D., Davies, N. M., Taylor, A. E., Tillmann, T., Vaucher, J., et al. (2019). Understanding the consequences of education inequality on cardiovascular disease: Mendelian randomisation study. BMJ 365:l1855. doi: 10.1136/bmj.l1855
Chen, Y. C., Fan, H. Y., Yang, C., and Lee, Y. L. (2020). Early pubertal maturation and risk of childhood asthma: a Mendelian randomization and longitudinal study. Allergy 75, 892–900. doi: 10.1111/all.14009
Demenais, F., Margaritte-Jeannin, P., Barnes, K. C., Cookson, W. O. C., Altmuller, J., Ang, W., et al. (2018). Multiancestry association study identifies new asthma risk loci that colocalize with immune-cell enhancer marks. Nat. Genet. 50, 42–53. doi: 10.1038/s41588-017-0014-7
Eagan, T. M., Gulsvik, A., Eide, G. E., and Bakke, P. S. (2004). The effect of educational level on the incidence of asthma and respiratory symptoms. Respir. Med. 98, 730–736. doi: 10.1016/j.rmed.2004.02.008
Gill, D., Efstathiadou, A., Cawood, K., Tzoulaki, I., and Dehghan, A. (2019). Education protects against coronary heart disease and stroke independently of cognitive function: evidence from Mendelian randomization. Int. J. Epidemiol. 48, 1468–1477. doi: 10.1093/ije/dyz200
Granell, R., Henderson, A. J., Evans, D. M., Smith, G. D., Ness, A. R., Lewis, S., et al. (2014). Effects of BMI, fat mass, and lean mass on asthma in childhood: a Mendelian randomization study. PLoS Med. 11:e1001669. doi: 10.1371/journal.pmed.1001669
Han, Y., Jia, Q., Jahani, P. S., Hurrell, B. P., Pan, C., Huang, P., et al. (2020). Genome-wide analysis highlights contribution of immune system pathways to the genetic architecture of asthma. Nat. Commun. 11:1776. doi: 10.1038/s41467-020-15649-3
Hancox, R. J., Milne, B. J., Taylor, D. R., Greene, J. M., Cowan, J. O., Flannery, E. M., et al. (2004). Relationship between socioeconomic status and asthma: a longitudinal cohort study. Thorax 59, 376–380. doi: 10.1136/thx.2003.010363
Harshfield, E. L., Georgakis, M. K., Malik, R., Dichgans, M., and Markus, H. S. (2021). Modifiable lifestyle factors and risk of stroke: a Mendelian randomization analysis. Stroke 52, 931–936. doi: 10.1161/STROKEAHA.120.031710
Kozyrskyj, A. L., Kendall, G. E., Jacoby, P., Sly, P. D., and Zubrick, S. R. (2010). Association between socioeconomic status and the development of asthma: analyses of income trajectories. Am. J. Public Health 100, 540–546. doi: 10.2105/AJPH.2008.150771
Larsson, S. C., Mason, A. M., Kar, S., Vithayathil, M., Carter, P., Baron, J. A., et al. (2020). Genetically proxied milk consumption and risk of colorectal, bladder, breast, and prostate cancer: a two-sample Mendelian randomization study. BMC Med. 18:370. doi: 10.1186/s12916-020-01839-9
Larsson, S. C., Traylor, M., Malik, R., Dichgans, M., Burgess, S., and Markus, H. S. (2017). Modifiable pathways in Alzheimer’s disease: Mendelian randomisation analysis. BMJ 359:j5375. doi: 10.1136/bmj.j5375
Liang, J., Cai, H., Liang, G., Liu, Z., Fang, L., Zhu, B., et al. (2021). Educational attainment protects against type 2 diabetes independently of cognitive performance: a Mendelian randomization study. Acta Diabetol. 58, 567–574. doi: 10.1007/s00592-020-01647-w
Liu, G., Zhao, Y., Jin, S., Hu, Y., Wang, T., Tian, R., et al. (2018). Circulating vitamin E levels and Alzheimer’s disease: a Mendelian randomization study. Neurobiol. Aging 72, 181.e1–189.e9. doi: 10.1016/j.neurobiolaging.2018.08.008
Locke, A. E., Kahali, B., Berndt, S. I., Justice, A. E., Pers, T. H., Day, F. R., et al. (2015). Genetic studies of body mass index yield new insights for obesity biology. Nature 518, 197–206. doi: 10.1038/nature14177
Minelli, C., Van Der Plaat, D. A., Leynaert, B., Granell, R., Amaral, A. F. S., Pereira, M., et al. (2018). Age at puberty and risk of asthma: a Mendelian randomisation study. PLoS Med. 15:e1002634. doi: 10.1371/journal.pmed.1002634
Mulugeta, A., Zhou, A., King, C., and Hypponen, E. (2020). Association between major depressive disorder and multiple disease outcomes: a phenome-wide Mendelian randomisation study in the UK Biobank. Mol. Psychiatry 25, 1469–1476. doi: 10.1038/s41380-019-0486-1
Okbay, A., Beauchamp, J. P., Fontana, M. A., Lee, J. J., Pers, T. H., Rietveld, C. A., et al. (2016). Genome-wide association study identifies 74 loci associated with educational attainment. Nature 533, 539–542. doi: 10.1038/nature17671
Park, S., Lee, S., Kim, Y., Cho, S., Kim, K., Kim, Y. C., et al. (2021). Kidney function and obstructive lung disease: a bidirectional Mendelian randomisation study. Eur. Respir. J. doi: 10.1183/13993003.00848-2021 [Epub ahead of print].
Raita, Y., Zhu, Z., Camargo, C. A. Jr., Freishtat, R. J., Ngo, D., Liang, L., et al. (2021). Relationship of soluble interleukin-6 receptors with asthma: a Mendelian randomization study. Front. Med. 8:665057. doi: 10.3389/fmed.2021.665057
Rosa, M., Chignon, A., Li, Z., Boulanger, M. C., Arsenault, B. J., Bosse, Y., et al. (2019). A Mendelian randomization study of IL6 signaling in cardiovascular diseases, immune-related disorders and longevity. NPJ Genom. Med. 4:23. doi: 10.1038/s41525-019-0097-4
Rosoff, D. B., Kaminsky, Z. A., Mcintosh, A. M., Davey Smith, G., and Lohoff, F. W. (2020). Educational attainment reduces the risk of suicide attempt among individuals with and without psychiatric disorders independent of cognition: a bidirectional and multivariable Mendelian randomization study with more than 815,000 participants. Transl. Psychiatry 10:388. doi: 10.1038/s41398-020-01047-2
Shen, M., Liu, X., Li, G., Li, Z., and Zhou, H. (2020). Lifetime smoking and asthma: a Mendelian randomization study. Front. Genet. 11:769. doi: 10.3389/fgene.2020.00769
Shrine, N., Portelli, M. A., John, C., Soler Artigas, M., Bennett, N., Hall, R., et al. (2019). Moderate-to-severe asthma in individuals of European ancestry: a genome-wide association study. Lancet Respir. Med. 7, 20–34. doi: 10.1016/S2213-2600(18)30389-8
Skaaby, T., Taylor, A. E., Thuesen, B. H., Jacobsen, R. K., Friedrich, N., Mollehave, L. T., et al. (2018). Estimating the causal effect of body mass index on hay fever, asthma and lung function using Mendelian randomization. Allergy 73, 153–164. doi: 10.1111/all.13242
Sun, J. Y., Zhang, H., Zhang, Y., Wang, L., Sun, B. L., Gao, F., et al. (2021). Impact of serum calcium levels on total body bone mineral density: a mendelian randomization study in five age strata. Clin. Nutr. 40, 2726–2733. doi: 10.1016/j.clnu.2021.03.012
Sun, Y. Q., Brumpton, B. M., Langhammer, A., Chen, Y., Kvaloy, K., and Mai, X. M. (2020). Adiposity and asthma in adults: a bidirectional Mendelian randomisation analysis of the HUNT study. Thorax 75, 202–208. doi: 10.1136/thoraxjnl-2019-213678
Verbanck, M., Chen, C. Y., Neale, B., and Do, R. (2018). Detection of widespread horizontal pleiotropy in causal relationships inferred from Mendelian randomization between complex traits and diseases. Nat. Genet. 50, 693–698. doi: 10.1038/s41588-018-0099-7
von Mutius, E., and Smits, H. H. (2020). Primary prevention of asthma: from risk and protective factors to targeted strategies for prevention. Lancet 396, 854–866. doi: 10.1016/S0140-6736(20)31861-4
Wang, M., Zhang, Z., Liu, D., Xie, W., Ma, Y., Yao, J., et al. (2021). Educational attainment protects against epilepsy independent of cognitive function: a Mendelian randomization study. Epilepsia 62, 1362–1368. doi: 10.1111/epi.16894
Xiuyun, W., Qian, W., Minjun, X., Weidong, L., and Lizhen, L. (2020). Education and stroke: evidence from epidemiology and Mendelian randomization study. Sci. Rep. 10:21208. doi: 10.1038/s41598-020-78248-8
Xu, S., Gilliland, F. D., and Conti, D. V. (2019). Elucidation of causal direction between asthma and obesity: a bi-directional Mendelian randomization study. Int. J. Epidemiol. 48, 899–907.
Yuan, S., Mason, A. M., Carter, P., Burgess, S., and Larsson, S. C. (2021). Homocysteine, B vitamins, and cardiovascular disease: a Mendelian randomization study. BMC Med. 19:97. doi: 10.1186/s12916-021-01977-8
Zhang, L., Tang, L., Xia, K., Huang, T., and Fan, D. (2020). Education, intelligence, and amyotrophic lateral sclerosis: a Mendelian randomization study. Ann. Clin. Transl. Neurol. 7, 1642–1647. doi: 10.1002/acn3.51156
Zhao, J. V., and Schooling, C. M. (2019). The role of linoleic acid in asthma and inflammatory markers: a Mendelian randomization study. Am. J. Clin. Nutr. 110, 685–690. doi: 10.1093/ajcn/nqz130
Zhao, J. V., and Schooling, C. M. (2021). Using Mendelian randomization study to assess the renal effects of antihypertensive drugs. BMC Med. 19:79. doi: 10.1186/s12916-021-01951-4
Zhu, Z., Lee, P. H., Chaffin, M. D., Chung, W., Loh, P. R., Lu, Q., et al. (2018). A genome-wide cross-trait analysis from UK Biobank highlights the shared genetic architecture of asthma and allergic diseases. Nat. Genet. 50, 857–864. doi: 10.1038/s41588-018-0121-0
Zhuang, Z., Gao, M., Yang, R., Liu, Z., Cao, W., and Huang, T. (2021a). Causal relationships between gut metabolites and Alzheimer’s disease: a bidirectional Mendelian randomization study. Neurobiol. Aging 100, 119.e15–119.e18. doi: 10.1016/j.neurobiolaging.2020.10.022
Keywords: asthma, educational attainment, genome-wide association study, Mendelian randomization, inverse-variance weighted
Citation: Li Y, Chen W, Tian S, Xia S and Yang B (2021) Evaluating the Causal Association Between Educational Attainment and Asthma Using a Mendelian Randomization Design. Front. Genet. 12:716364. doi: 10.3389/fgene.2021.716364
Received: 28 May 2021; Accepted: 12 July 2021;
Published: 09 August 2021.
Edited by:
Guiyou Liu, Tianjin Institute of Industrial Biotechnology, Chinese Academy of Sciences, ChinaReviewed by:
Yang Hu, Harbin Institute of Technology, ChinaKeshen Li, The First Affiliated Hospital of Jinan University, China
Copyright © 2021 Li, Chen, Tian, Xia and Yang. This is an open-access article distributed under the terms of the Creative Commons Attribution License (CC BY). The use, distribution or reproduction in other forums is permitted, provided the original author(s) and the copyright owner(s) are credited and that the original publication in this journal is cited, in accordance with accepted academic practice. No use, distribution or reproduction is permitted which does not comply with these terms.
*Correspondence: Biao Yang, eWFvYmlhb3liQDEyNi5jb20=
†These authors have contributed equally to this work