- 1Key Laboratory of Animal Genetics, Breeding and Reproduction, MARA, National Engineering Laboratory of Animal Breeding, College of Animal Science and Technology, China Agricultural University, Beijing, China
- 2Department of Animal Sciences, Purdue University, West Lafayette, IN, United States
- 3Beijing Dairy Cattle Center, Beijing, China
- 4Beijing Sunlon Livestock Development Company Limited, Beijing, China
Fertility and reproductive performance are key drivers of dairy farm profitability. Hence, reproduction traits have been included in a large majority of worldwide dairy cattle selection indexes. The reproductive traits are lowly heritable but can be improved through direct genetic selection. However, most scientific studies and dairy cattle breeding programs have focused solely on the genetic effects of the dam (GED) on reproductive performance and, therefore, ignored the contribution of the service sire in the phenotypic outcomes. This study aimed to investigate the service sire effects on female reproductive traits in Holstein cattle from a genomic perspective. Genetic parameter estimation and genome-wide association studies (GWAS) were performed for the genetic effect of service sire (GESS) on conception rate (CR), 56-day non-return rate (NRR56), calving ease (CE), stillbirth (SB), and gestation length (GL). Our findings indicate that the additive genetic effects of both sire and dam contribute to the phenotypic variance of reproductive traits measured in females (0.0196 vs. 0.0109, 0.0237 vs. 0.0133, 0.0040 vs. 0.0289, 0.0782 vs. 0.0083, and 0.1024 vs. 0.1020 for GESS and GED heritability estimates for CR, NRR56, CE, SB, and GL, respectively), and these two genetic effects are positively correlated for SB (0.1394) and GL (0.7871). Interestingly, the breeding values for GESS on insemination success traits (CR and NRR56) are unfavorably and significantly correlated with some production, health, and type breeding values (ranging from −0.449 to 0.274), while the GESS values on calving traits (CE, SB, and GL) are usually favorably associated with those traits (ranging from −0.493 to 0.313). One hundred sixty-two significant single-nucleotide polymorphisms (SNPs) and their surrounding protein-coding genes were identified as significantly associated with GESS and GED, respectively. Six genes overlapped between GESS and GED for calving traits and 10 genes overlapped between GESS for success traits and calving traits. Our findings indicate the importance of considering the GESS when genetically evaluating the female reproductive traits in Holstein cattle.
Introduction
In recent decades, the genetic selection for functional traits, including reproductive performance, has received great emphasis in dairy cattle selection indexes, aiming to achieve more balanced and sustainable breeding goals (Egger-Danner et al., 2015). Female fertility has only been broadly included in national selection indexes of dairy cattle breeding programs over the past five decades (Cole and VanRaden, 2018). Although most dairy cattle breeding programs focus only on the genetic effects of the dam (GED) when genetically evaluating fertility and calving traits, there are evidence that the service sire may also have a genetic influence on female reproductive performance (Barton et al., 1984; Van Tassell et al., 2003; Averill et al., 2004)—for instance, the direct effects of the service sire (e.g., semen quality and viability) on female reproductive performance have been considered as indirect effects (Jansen, 1985). Jaton et al. (2017) reported that the heritability estimates of service sire on embryo quality were lower than the donor (0.02 versus 0.04) but still statistically significant. In 2008, a national evaluation model of sire conception rate (SCR) was established in the United Sates by the Animal Improvement Program Laboratory of the United States Department of Agriculture (Norman et al., 2008). SCR is measured as confirmed pregnancy ratio (in percentage) of each service sire. They also implemented a sire–maternal grandsire (S-MGS) model to estimate the genetic component of service sire in calving performance (Van Tassell et al., 2003; Jiang et al., 2018). Even though low heritability estimates have been reported for indirect indicators of male fertility (Berry et al., 2011; Tiezzi et al., 2013), it can still be improved through genomic selection (Lillehammer et al., 2011). Hence, understanding the genetic mechanisms underlying male fertility and developing accurate genomic prediction models are of great importance but still underexplored.
The determination of the genetic effect of service sire (GESS) on reproductive traits relies on genetic and genomic analyses, including the estimation of genetic parameters and genome-wide association studies (GWAS). Previous studies have identified interesting genomic regions associated with SCR, as reviewed by Taylor et al. (2018). However, there are few reports of the GESS on other reproductive traits at the genomic level (Fang et al., 2019; Jiang et al., 2018). In this context, the main objectives of this study were as follows: (Egger-Danner et al., 2015) to investigate the genetic background of service sire on female reproductive performance, including conception rate (CR), 56-day non-return rate (NRR56), calving ease (CE), stillbirth (SB), and gestation length (GL) in Holstein cattle and (Cole and VanRaden, 2018) to identify genomic regions and candidate genes associated with GESS on female reproduction performance.
Materials and Methods
Phenotypic and Genomic Data
Phenotypes
Records of birth dates, insemination events, pregnancy diagnoses, and calving information collected in 39 farms (Sunlon Livestock Development Co. Ltd., Beijing, China) from 1987 to 2020 were extracted from the AfiFarm software (AfiFarm1) and used in this study. The reproductive traits include CR (1 = pregnant, 0 = non-pregnant), NRR56 (1 = non-return, 0 = return), CE (scores from 1 to 3, in which 1 = unassisted, 2 = easy pull, 3 = hard pull and surgery needed), SB (1 = calf was alive 24 h after birth and 2 = calf was dead), and GL (in days). The last insemination before a positive pregnancy diagnosis in each parity was considered as pregnancy. Furthermore, the cows with calving records but without a positive pregnancy diagnosis were considered pregnant when last inseminated before calving. Insemination records that had the next insemination within 1–17 days or had neither insemination nor calving records after that time period were excluded from the NRR56 calculation. CE and SB were directly coded from raw data after excluding ambiguous records (3%) caused by mis-recording. GL records lower than 260 and greater than 302 days were also deleted. A descriptive summary for each trait after data editing is shown in Table 1. In total, 163,818 Holstein cows had phenotypes and were serviced by 1,952 bulls. The average (±SD) number of services per bull was 489 ± 1,947. A pedigree file spanning over 13 generations was provided by the Beijing Dairy Cattle Center (BDCC, Beijing, China) and consisted of 503,118 cows and 151,273 bulls born between 1957 and 2020. The estimated breeding values (EBVs) for six production traits (milk yield, milk protein yield, milk protein ratio, milk fat yield, milk fat ratio, and somatic cell score), two health traits (reproductive diseases and udder diseases), and five type traits (dairy character, milking system, capacity, rump, and overall conformation) traits were also provided by BDCC.
Genotypes
A total of 3,477 Holstein bulls were genotyped with the Illumina BovineSNP50 BeadChip (Illumina, Inc., San Diego, CA, United States) containing 54,609 single-nucleotide polymorphism (SNP) markers. These genotypes were imputed to the Illumina 150K Bovine Beadchip (containing 123,268 SNPs) using the BEAGLE v5.1 software (Browning et al., 2018) and a reference population consisting of 3,119 cows and 81 bulls. The SNP information was updated from an older version of the cattle reference genome (UMD 3.1) assembly to the current one (ARS-UCD 1.2) using the UCSC LiftOver tool2. Eight thousand five SNPs with missing position in the latest reference genome were removed from further analyses. The reference population was divided into a sub-reference population (2,725 individuals genotyped with the 150K SNP panel) and a sub-validation population (475 individuals genotyped with the 150K SNP panel but masked to only the 50K panel SNPs) for assessing the accuracy of genotype imputation as the concordance rate of imputed SNPs. Only SNPs with imputation accuracy greater than 90% were kept for further analyses. Furthermore, SNPs with minor allele frequency lower than 0.05, unknown chromosome or genome position, and extreme deviation from the Hardy–Weinberg equilibrium (p-value lower than 10–6) were removed. After data editing, 109,274 SNPs located in the autosomes and pseudo-autosomal regions of the X chromosome were retained in the dataset.
Estimation of Genetic Parameters
The variance and covariance components for each reproductive trait were estimated using the AI-REML algorithm implemented in the DMUAI module of the DMU v6 software (Madsen et al., 2006). A previous study evaluating similar traits indicated that the heritability estimates of linear and threshold models tend to be similar (Meijering, 1985; Boichard and Manfredi, 1994). Therefore, the following linear mixed model was fitted:
where y represents the vector of phenotypic observations (i.e., CR, NRR56, CE, SB, or GL), β is the vector of fixed effects included in the model, in which different systematic effects were fitted for each trait [i.e., AI technician, parity, semen type, and number of inseminations for CR and NRR56 and calf sex, parity, and group of calf size (divided according to their birth weights: 30–40, 40–50, and 50–60 kg) for CE, SB, and GL]. All of the fixed effects significantly (P < 0.05) influenced the dependent variables (CR, NRR56, CE, SB, and SB) and were identified based on mixed model analysis using the PROC MIXED function implemented in the SAS software (version 9.1.3; SAS Institute Inc., Cary, NC, United States); um and uf are the vectors of the random animal effects accounted by GESS and GED, respectively; pem and pef are the vectors of the random permanent environmental effects of the service sire and dam, respectively; hym is the vector of the random herd–year–month effects; e is the vector of the random residual effects; and, X, Z1, W1, W2 and Z2 are the corresponding incidence matrices. We assumed that:
with:
where and are the additive genetic variances of service sire and dam, respectively; σm,f is the genetic covariance of service sire and dam; and are the permanent environmental variances of service sire and dam, respectively; is the herd–year–month variance; is the residual variance; ⊗ is the Kronecker product function; A is the additive genetic relationship matrix among the animals; and I is an identity matrix. For calving traits, the model could be considered as an improved sire–dam model that assumes that the GESS has a genetic covariance with the GED. Compared to the traditional evaluation models of calving traits that only consider the animal effect, the current model includes both service sire and dam effects in the female reproductive performance phenotypes through GESS and GED, respectively. Differently from direct and maternal (paternal) effects usually assumed to be correlated, this was not the case for the genetic components of service sire and dam in the current study. Therefore, the GESS heritability estimates were calculated as follows:
and the repeatability estimates were calculated as follows:
The GED repeatability was estimated in the same way but replacing in the numerator by .
The standard error of the heritability and repeatability estimates, respectively, were calculated using the Delta method (Su et al., 2007). A Wald test was carried out to determine the statistical difference between the genetic parameter estimates and zero. In addition, correlations between the genomic breeding values for GESS of the reproduction traits, as well as production, health, and type traits, were estimated using the method proposed by Calo et al. (1973) and bull EBVs (filtered based on EBV reliability, as described below). Standard errors (SE) of the approximate genetic correlations were calculated based on the formula proposed by Sokal and Rohlf (1981). The predicted transmitting ability (PTA) of six production traits (milk yield, milk protein yield, milk protein ratio, milk fat yield, milk fat ratio, and somatic cell score), two health traits (reproductive disease and udder disease), and five type traits (dairy character, milking system, capacity, rump, and overall conformation) with reliabilities higher than 20, 20, and 30%, respectively, were provided by BDCC. The statistical models used for the genetic evaluation of these traits are described in Miglior et al. (2009) and Wu et al. (2013). Individuals with PTA reliabilities for GESS on reproductive traits above 40% were used for the calculation of correlation of breeding values.
Genome-Wide Association Study and Functional Enrichment Analyses
The “Fixed and random model Circulating Probability Unification” (FarmCPU) R package (Liu X. et al., 2016) was used to perform single-SNP regression analyses. FarmCPU is a multi-locus model that incorporates multiple markers simultaneously as covariates to partially remove the confounding effect between testing markers and kinship (Liu X. et al., 2016). De-regressed proofs (DRP) of the GESS and GED for female reproductive traits were derived following the procedures suggested by Wiggans et al. (2011). Individuals with accuracies greater than 10% were used as dependent variables in the GWAS model. The obtained p-values were corrected for multiple testing by calculating the false discovery rate (FDR) (Benjamini et al., 2001) at the 5% genome-wise level. Quantile–quantile (Q–Q) plots and the inflation factor λ (Devlin and Roeder, 1999) were used to investigate population stratification by comparing the observed and expected distributions of –log(p-value).
Positional genes located at up to 200 kb upstream and downstream of the significant SNPs were identified using the BiomaRt package (Durinck et al., 2005). This 200-kb window was defined based on the linkage disequilibrium level of the studied population (Supplementary Figure 1). The ClueGO module in Cytoscape (Bindea et al., 2009) was used to identify candidate genes, Gene Ontology (GO), and Kyoto Encyclopedia of Genes and Genomes (KEGG) terms. Furthermore, the Cattle Quantitative Trait Locus (QTL) database (Cattle QTLdb Release 423) was used to identify important trait–QTL associations previously reported in the literature.
Results and Discussion
Descriptive Statistics of Phenotype
The descriptive statistics of the five reproductive traits evaluated are shown in Table 1. For the insemination success traits (CR and NRR56), the mean CR was 43 ± 49% and the mean NRR56 was 50 ± 50% in Chinese Holstein cattle. These estimates are lower than those reported by Guo et al. (2014), which were inferred from the number of services recorded from 2001 to 2010. The average CE, SB, and GL were 1.06 ± 0.27, 1.07 ± 0.25, and 278.36 ± 6.18 days, respectively. The proportion of CE scores higher than 1 was 5.21%, and the SB rate was 6.67%. Vieira-Neto et al. (2017) reported a mean GL of 276 ± 6 days in Holstein cows, which is slightly lower than that of the current study. GL is affected by various environmental effects, such as temperature (McClintock et al., 2003) and cow parity number (King et al., 1985), which may have led to the discrepancy of GL between different populations.
Genetic Parameters for Female Reproductive Traits Considering GESS
Heritability and Repeatability Estimates
The (co)variance components, heritability, and genetic correlations for GESS and GED for all five reproduction traits are shown in Table 2. Overall, the heritability estimates for the success traits were low, but the heritability of GESS were significantly higher than those of GED (0.020 ± 0.004 vs. 0.011 ± 0.000 for CR and 0.024 ± 0.005 vs. 0.013 ± 0.001 for NRR56), indicating that service sires actually have greater genetic impact on insemination success than the mating cows. Fertility traits are known to have low heritability (VanRaden et al., 2004), which makes it more difficult to obtain faster genetic progress compared to more heritable traits. Therefore, larger datasets and novel phenotypes (Fleming et al., 2019) should be generated for increasing genetic progress for fertility performance. The small, but significant, genetic contribution of service sires on success traits indicates the possibility of genetically improving the GESS. The repeatability estimates of these reproduction traits were almost equal to their heritabilities, except for the GED on NRR56 (0.031 ± 0.001 vs. 0.013 ± 0.001), indicating the inconsistency among these records. Hence, more repeated records are needed for greater EBV accuracies. Another reason for the low repeatability might be the ignorance of non-additive genetic effects, which could lead to the underestimation of genetic parameters. For most traits, the genetic variance of the GESS was comparable to that of the GED, demonstrating that service sires have considerable genetic contribution to female reproductive outcomes. The heritability estimates for female CR in other studies range from 0.01 to 0.03 (Azzam et al., 1988; Bagnato and Oltenacu, 1993; Muuttoranta et al., 2019). These low estimates support the estimates of GED on CR in this study. The heritability of GED on NRR56 was consistent with the value (0.01) reported by Sun and Su (2010) but different from that of Hoekstra et al. (1994) (0.04) and Eghbalsaied (2011) (0.002–0.003). These discrepancies may be attributed to the differences in the populations evaluated and the statistical models used since GESS was only included in the current study. Some studies have considered the GESS as a non-genetic random effect apart from the GED, and the heritabilities obtained from such models were shown to be lower than 0.02 for CR and NRR56 (Weigel and Rekaya, 2000; Kuhn and Hutchison, 2008). However, Tiezzi et al. (2011) suggested that the heritabilities generated using the approach mentioned above are lower than those fitting GESS. Furthermore, Tiezzi et al. (2013) reported that the heritability and repeatability of the GESS on NRR56 were both 0.01 for Italian Brown Swiss cattle. The characteristics of the two populations evaluated might have caused the discrepancy in the estimates observed. In the study of Tiezzi et al. (2013), a smaller and older population than that in the current study was used, and the cattle in their population performed better in NRR56 (0.70 for average), indicating a better management and genetic level of their herds.
For calving traits, the heritability estimates of GESS are lower than the GED for CE (0.004 ± 0.001 vs. 0.029 ± 0.002) and similar for GL (0.102 ± 0.001 vs. 0.102 ± 0.000) but higher for SB (0.078 ± 0.010 vs. 0.008 ± 0.001). Both GESS and GED show low heritability for SB and CE, though a moderate estimation was observed for GL. Except for the GED for SB (0.021 ± 0.002) and GL (0.116 ± 0.004), the repeatabilities were almost equal to the corresponding heritabilities. Each cow has only one calving record in each parity, which results in poor consistency among repeated data. The low heritability estimates of SB and CE indicate that these traits might benefit more from genomic information. A S-MGS model was used to evaluate calving traits in previous studies, which resulted in low heritability for CE and SB (0.07–0.13) (Van Tassell et al., 2003; Heringstad et al., 2007). The S-MGS model converted the direct and maternal variances to sire and maternal grandsire variances, which was, to some extent, different from those of the present study. Due to the differences in the model used in the current study, here we simply compare our findings with the results of the S-MGS model from previous studies. The maternal heritabilities estimated by other models ranged from 0.01 to 0.13 for GL (Jamrozik et al., 2005; Crews, 2006; Mujibi and Crews, 2009) and 0.02–0.08 for CE and SB (Luo et al., 2002; Jamrozik et al., 2005; Eaglen et al., 2013). The GED variance obtained in the current study for SB was 0.0002, which is much lower than the estimates from a previous study with Norwegian Red cows (Heringstad et al., 2007). The heritability of the GED was higher than that of the GESS for CE, which may have been caused by a larger maternal effect (Jamrozik et al., 2005)—for instance, cow body conformation is genetically associated with calving difficulty (Dadati et al., 1985; Ga et al., 2021), indicating the crucial role of GED on CE.
Genetic Correlations
Former genomic analyses reported that some genes (e.g., SPP1) regulate both tissue and embryonic growth (Weintraub et al., 2004; Rangaswami et al., 2006), which is important for both the male and female aspects of calving traits. Furthermore, some genes related to spermatogenesis have been shown to affect cow reproduction performance (Peddinti et al., 2010; Li et al., 2012b; Buzanskas et al., 2017). Thus, the genetic covariances of male and female were considered. The genetic correlations between the GESS and GED were significantly different from zero for SB and GL (P < 0.05; based on a t-test). The correlation coefficient was considerably high in GL, whereas that in CE was low and non-significant (P > 0.05; CE: 0.099 ± 0.081; SB: 0.139 ± 0.071; GL: 0.787 ± 0.040). The results indicate the homogeneity between the GESS and the GED for SB and GL. The genetic covariances of the insemination traits between the effects of service bull and dams were usually ignored in previous studies (Berry et al., 2011). We evaluated these covariances because there are evidence of low but statistically significant correlations between GESS and GED [e.g., NRR56: 0.010 ± 0.002; (Tiezzi et al., 2013)]. However, there was no significant correlation between the two terms in the current study (CR: 0.044 ± 0.066 and NRR56: −0.094 ± 0.063). The genetic correlations between the direct and maternal effects for SB and CE can be quite variable, ranging from −0.24 to 0.12 (Luo et al., 1999; Steinbock et al., 2003; Wiggans et al., 2003; Cole et al., 2007; Heringstad et al., 2007; Vanderick et al., 2014). For GL, the correlations are usually negative and stronger (−0.13 ∼ −0.85) (Cubas et al., 1991; Bennett and Gregory, 2001; Hansen et al., 2004; Crews, 2006; Cervantes et al., 2010). These discrepancies are reasonable, because we considered both the direct effect of dam and maternal effect as dam effect.
The correlations of breeding values of the GESS of reproductive traits as well as production, health, and type traits are shown in Table 3. For all the traits, the genetic information of approximately 400–500 individuals with reliability greater than 40% for reproductive traits and 20% for the other traits was used for the calculation of breeding value correlations. Interestingly, the GESS was negatively correlated with most production and type traits (e.g., milk yield and overall conformation), while positive correlations were observed between GESS and health traits such as reproductive disorders. We found that the GESS on CR was unfavorably and significantly related to milk yield (−0.218 ± 0.039), indicating that selection exclusively on milk production might indirectly result in a decline of insemination performance of the service sire. Murray et al. (1977) reported a negative correlation between male fertility and milk yield (−0.26), which is in contrast to the positive correlation (0.13–0.29) reported by Raheja et al. (1989). Further verification about the biological correlation between male fertility and milk production is needed due to the inconsistent relationships observed. The genetic correlations between GESS on success traits and reproductive disease were positive (0.174 ± 0.043 for CR and 0.203 ± 0.042 for NRR56). Progesterone is regarded as a responsible factor for cattle ovarian follicular cysts (Silvia et al., 2002). Ramal-Sanchez et al. (2020) also suggested that progesterone is related to sperm release, which affects the fertilization ability of spermatozoa. Therefore, reproduction-related hormones might account for the potential biological relation between GESS on success traits and reproductive disease. Cows with a higher incidence of ovarian cysts tend to have lower fertility. The genetic correlation between GESS on GL and overall conformation was negative, indicating that undesirable body conformation might lead to longer GL, with worse development after birth (Bourdon and Brinks, 1982). These correlations indicate that direct selection for production, health, and type traits may have a favorable effect on service sire calving performance but may lead to an unfavorable decline in service sire mating performance.
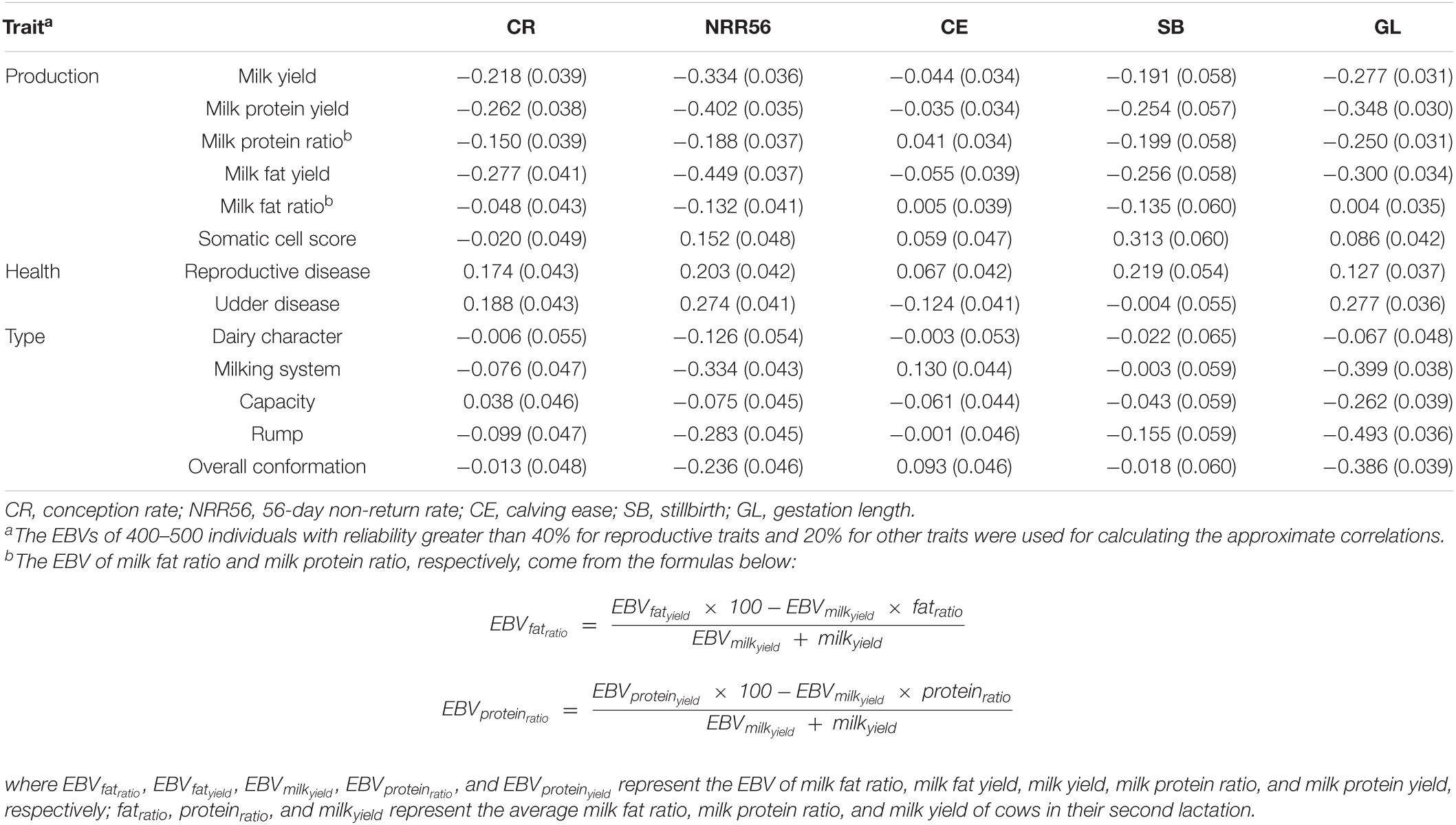
Table 3. The correlations of breeding values for the genetic effects of service sire of reproductive traits and production, health, and type traits in Holstein cattle.
Genome-Wide Association Studies
The estimated breeding values obtained from former genetic estimation with accuracy above 10% were used for DRP calculation. Hence, the EBV of 2,996 and 1,147 individuals were used in GWAS for GESS and GED, respectively. The detailed information of the genomic regions and candidate genes for GESS and GED for the five reproductive traits are summarized in Tables 4, 5. In addition, the Q–Q plots and Manhattan plots are provided in Figures 1–4. The Q–Q plots and λ of GESS on 56-day non-return rate indicated a slight inflation of the results. A total of 162 significant SNPs were detected, including two significant SNPs located in the X chromosome (pseudo-autosomal region) (Johnson et al., 2019). The P-values ranged from 1.34 × 10–10 to 1.05 × 10–5, and FDR ranged from <0.001 to 0.050. Furthermore, we mapped the significant SNPs to the Cattle QTL database (see Text Footnote 3), and the overlapped QTL regions are listed in Tables 6, 7.
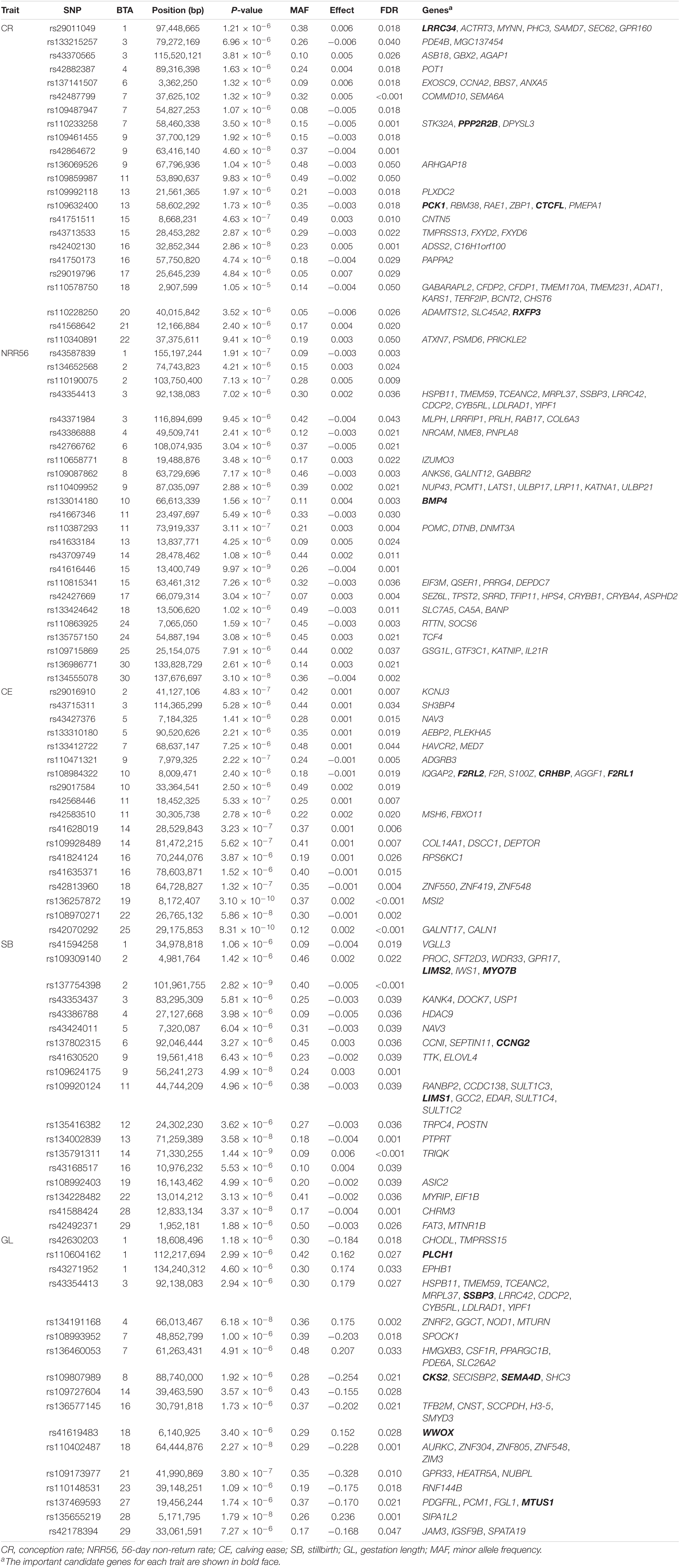
Table 4. Significant single-nucleotide polymorphism (SNP) and nearby genes of the genetic effects of service sire on five reproductive traits carried out by genome-wide association studies in Holstein cattle.
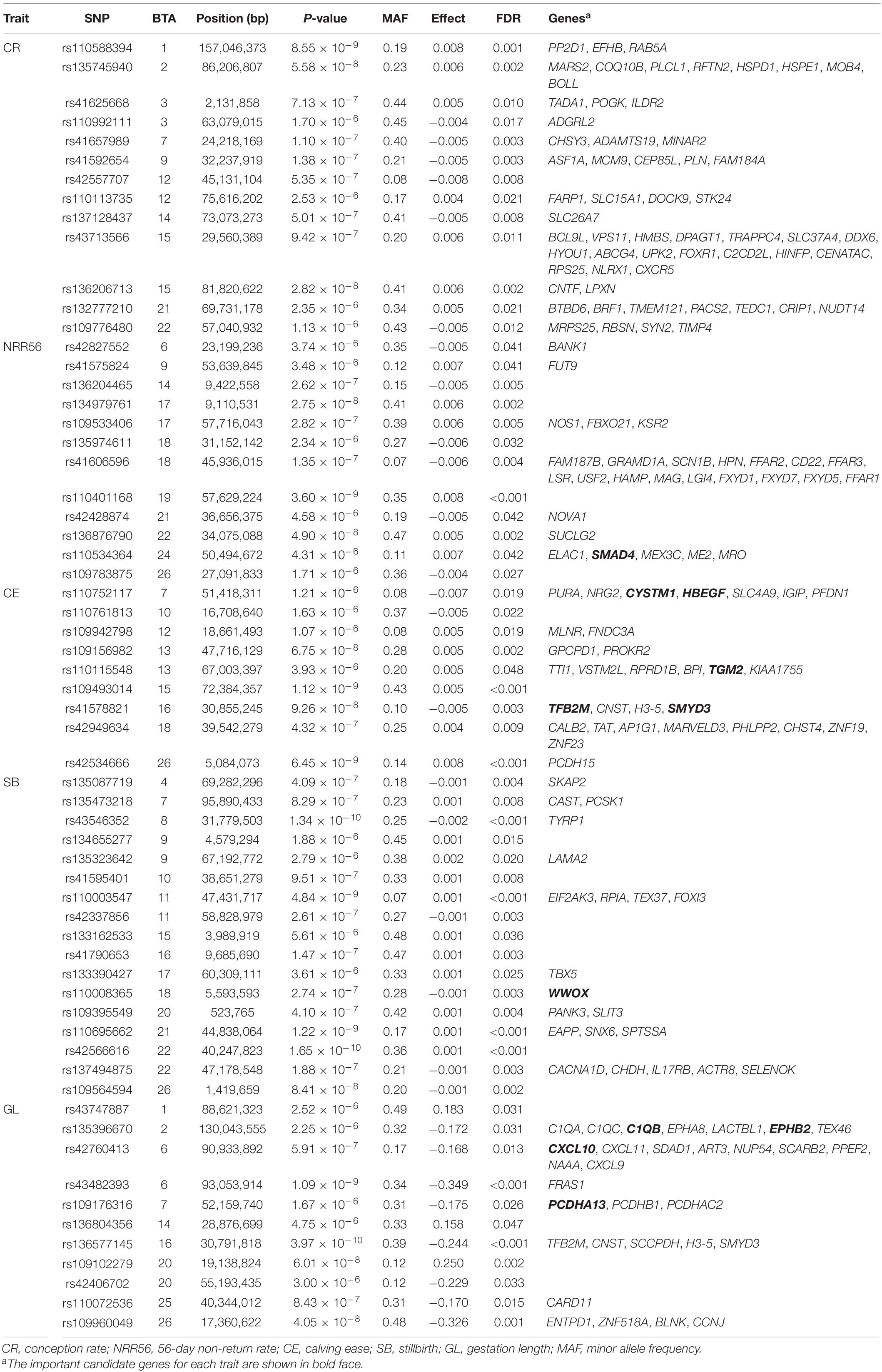
Table 5. Significant single-nucleotide polymorphism (SNP) and near-by genes of the genetic effects of dam on five reproductive traits carried out by genome-wide association studies in Holstein cattle.
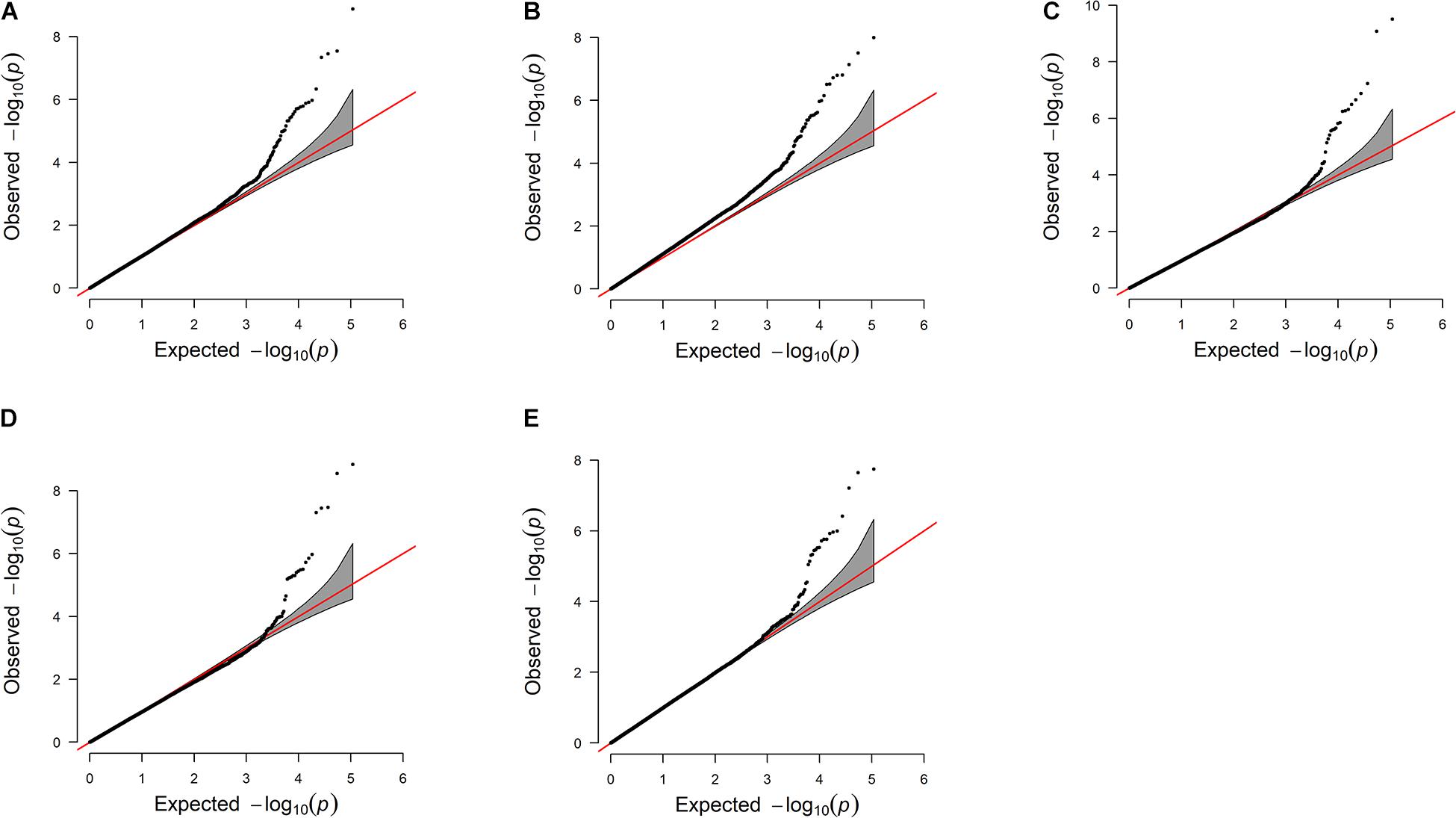
Figure 1. Quantile–quantile plots of the genome-wide association studies for genetic effect of service sire on reproductive traits (A–E). The x-axis and the y-axis represent the expected and observed −log10(P−value), respectively. (A) Conception rate—the λ value is 1.06. (B) 56-day non-return rate—the λ value is 1.15. (C) Calving ease—the λ value is 0.96. (D) Stillbirth—the λ value is 0.95. (E) Gestation length—the λ value is 0.99.
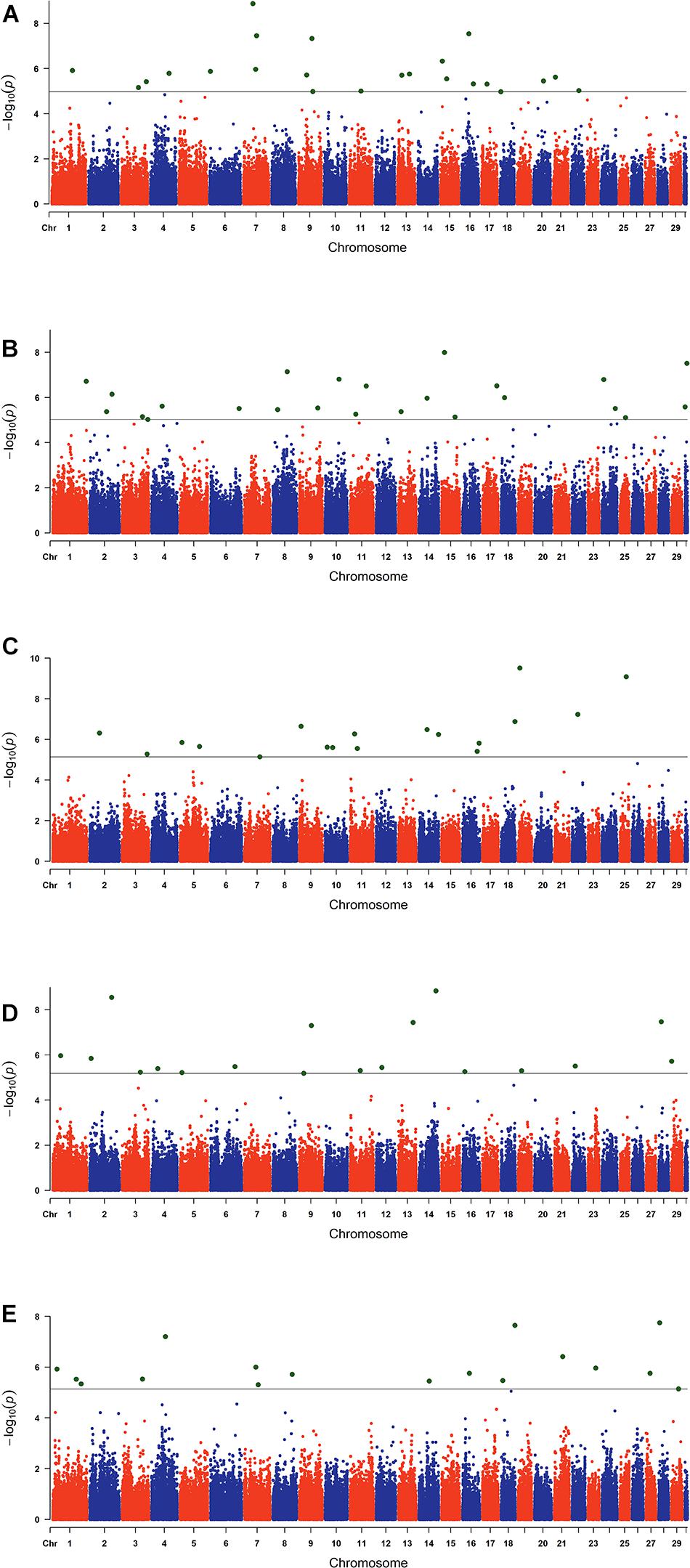
Figure 2. Manhattan plots of the genome-wide association studies for genetic effect of service sire on reproductive traits. The x-axis and the y-axis represent the chromosome number and the observed −log10(P−value), respectively. The single-nucleotide polymorphisms were plotted against their genomic positions. The lines in the plots indicate the thresholds of false discovery rate (0.05) in the corresponding traits: (A) conception rate, (B) 56-day non-return rate, (C) calving ease, (D) stillbirth, and (E) gestation length.
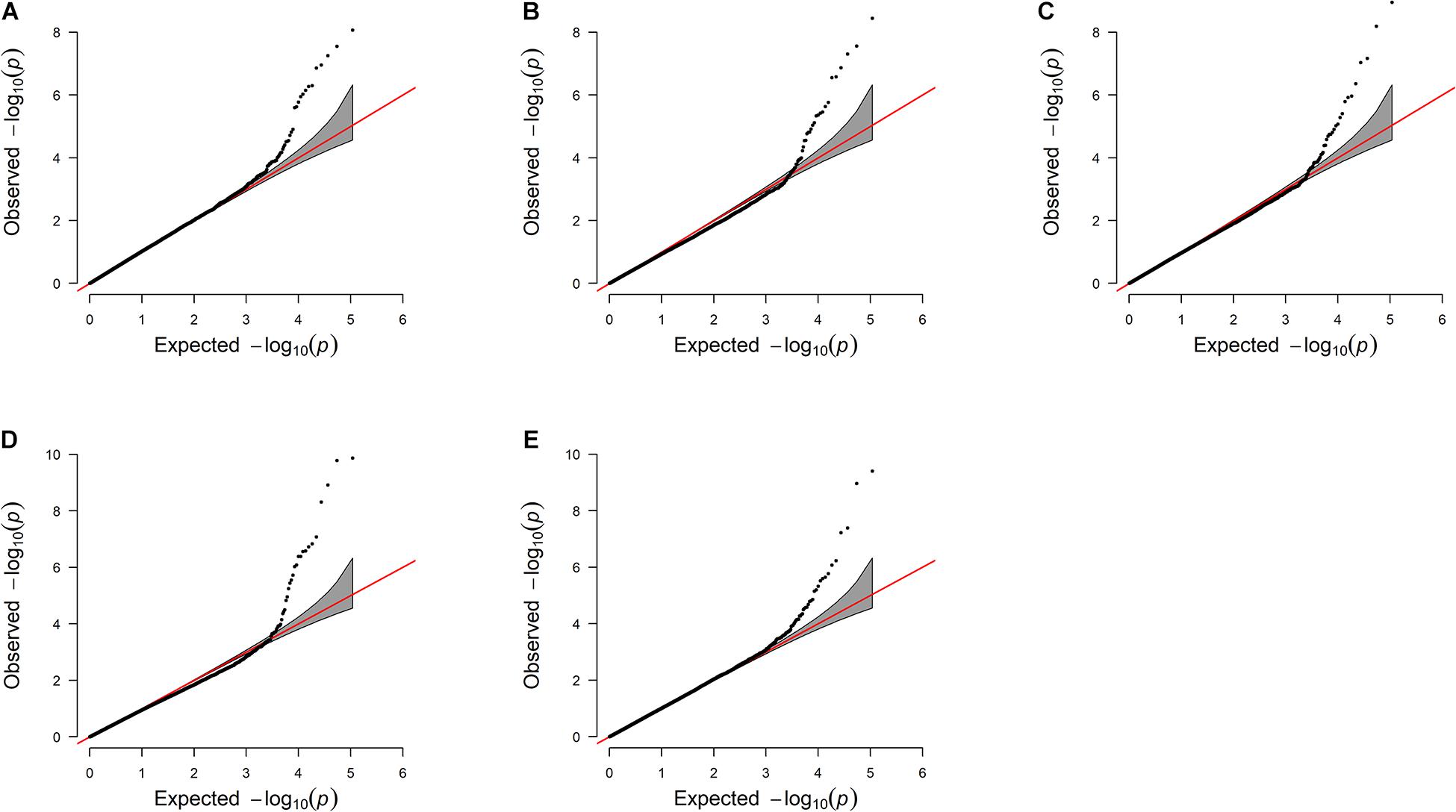
Figure 3. Quantile–quantile plots of genome-wide association studies for genetic effects of the dam on reproductive traits (A–E). The x-axis and the y-axis represent the expected and observed −log10(P−value). (A) Conception rate—the λ value is 1.03. (B) 56-day non-return rate—the λ value is 0.92. (C) Calving ease—the λ value is 0.96. (D) Stillbirth—the λ value is 0.94. (E) Gestation length—the λ value 1.00.
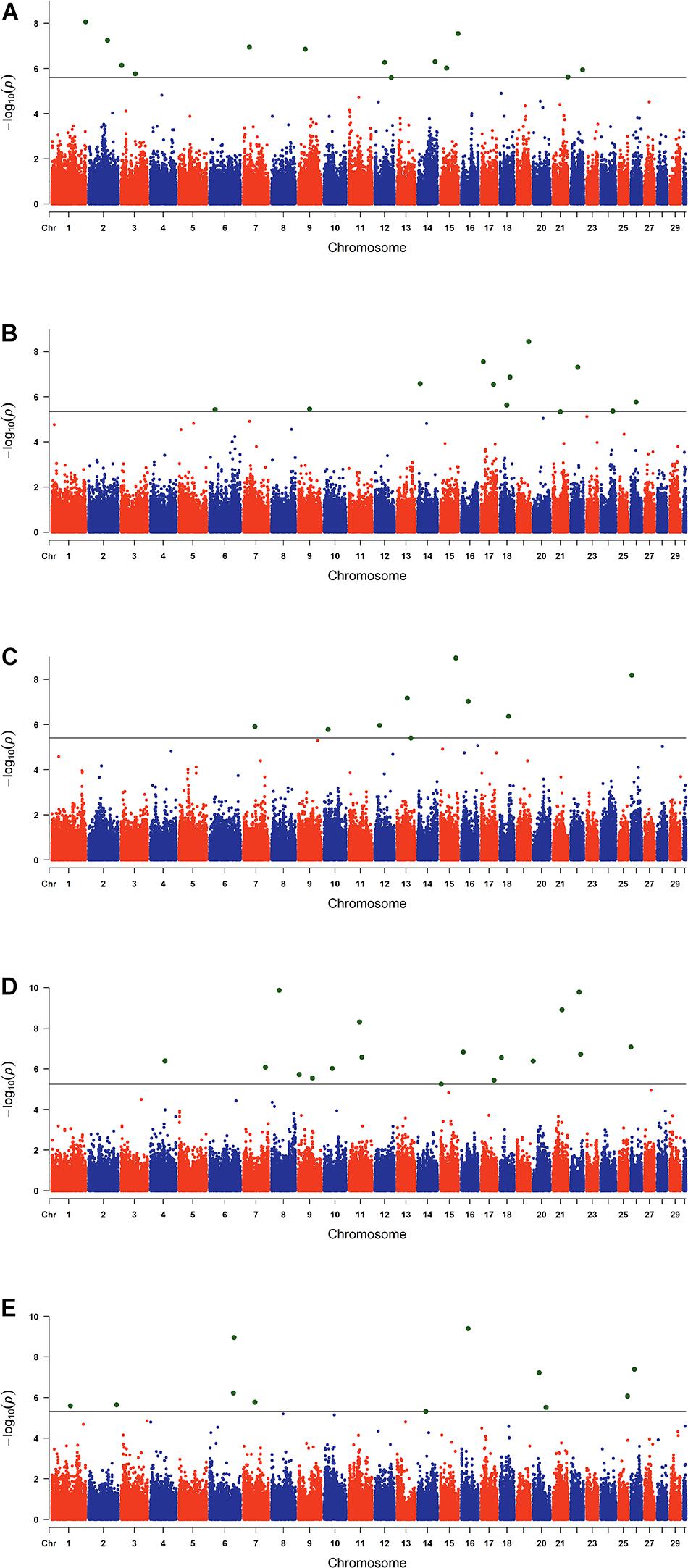
Figure 4. Manhattan plots of the genome-wide association studies for the genetic effects of the dam on reproductive traits. The x-axis and the y-axis represent the chromosome number and the observed −log10(P−value), respectively. The single-nucleotide polymorphisms were plotted against their genomic positions. The lines in the plots indicate the thresholds of false discovery rate (0.05) in the corresponding traits: (A) conception rate, (B) 56-day non-return rate, (C) calving ease, (D) stillbirth, and (E) gestation length.
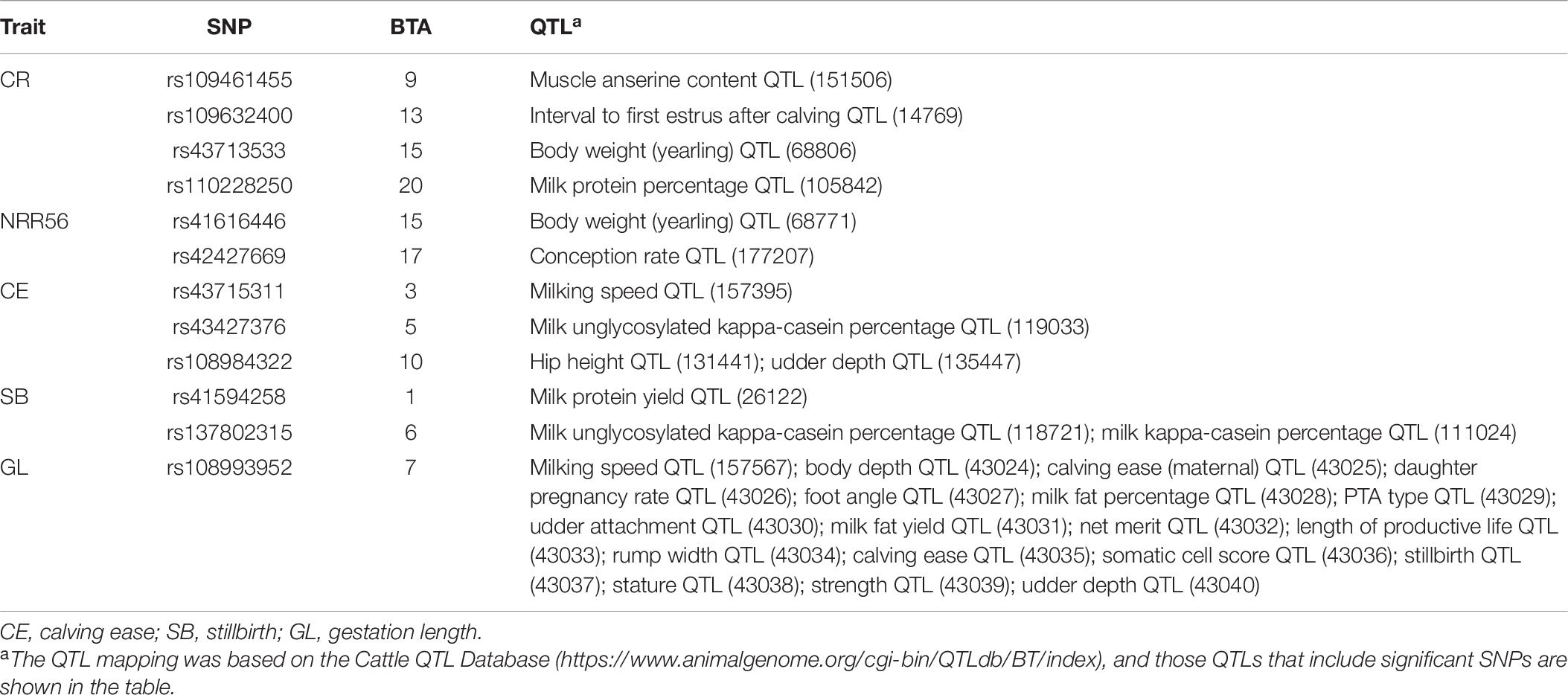
Table 6. Significant single-nucleotide polymorphisms (SNPs) of the genetic effects of service sire and overlapping quantitative trait loci (QTLs).
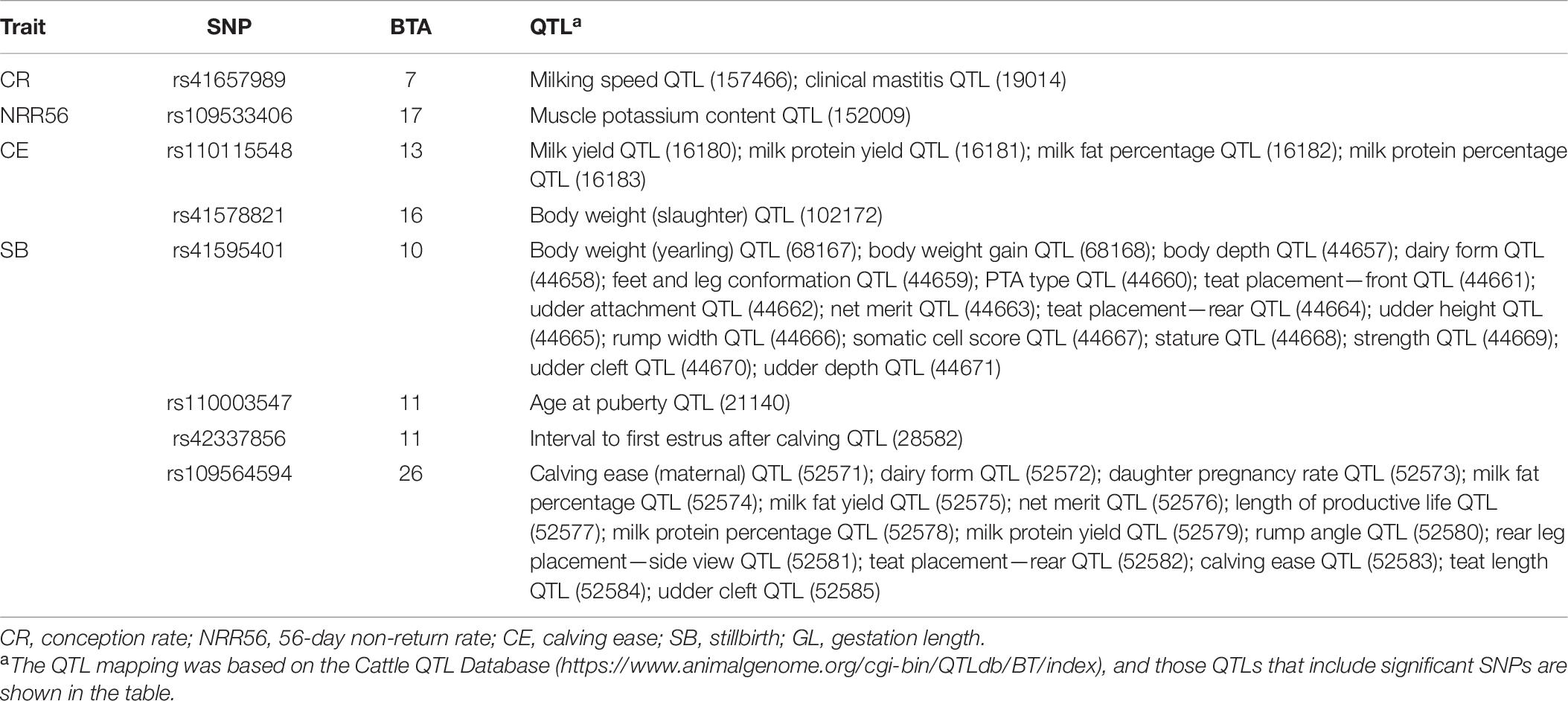
Table 7. Significant single-nucleotide polymorphisms (SNPs) of the genetic effects of dam and overlapping quantitative trait loci (QTLs).
For GESS, 100 SNPs were found to be significant, with 23, 24, 18, 18, and 17 associated with CR, NRR56, CE, SB, and GL, respectively (Table 4). For the success traits, 53 nearby annotated protein-coding genes located 200 kb upstream and downstream of the significant SNPs were mapped, including genes related to sperm development (e.g., BMP4) and early embryogenesis (e.g., LRRC34). Han and Peñagaricano (2016) also identified a genomic region (1.5 Mb) located on BTA13 which explained more than 0.50% of the total additive genetic variance for SCR (male fertility). This region contains the CTCFL gene detected in the present study. Although previous studies have attempted to identify candidate genes related to GESS on success traits (Taylor et al., 2018), novel candidate genes were identified in this study—for instance, the gene BMP4 (bone morphogenetic protein 4) was previously indicated as a candidate gene for spermatogenesis (Hu et al., 2004; Li et al., 2014) and follicle development (Nilsson and Skinner, 2003; Shimizu et al., 2004; Fatehi et al., 2005; Li and Ge, 2011). These associations indicate the paternal and maternal effects on the pre-implantation stage of embryo development, respectively (Koide et al., 2009; Li et al., 2012a). In this context, the present study also revealed the GESS on female conception. Ou et al. (2020) carried out a transcriptome analysis on spermatogonial stem cells (SSCs) and reported that LRRC34 was highly expressed in mouse SSCs and was essential for in vitro SSC proliferation. RXFP3 was identified to be differentially expressed in human sperm and was likely diminished in spermiogenesis (Heidari et al., 2018). Furthermore, other studies suggested PPP2R2B and PCK1 as candidate genes affecting the semen quality traits in livestock (Huang et al., 2016; Gao et al., 2019).
One hundred fourteen nearby proteinase genes located 200 kb upstream and downstream of the significant SNPs related to the GESS of calving traits were mapped. The fetal growth and metabolism show a specific pattern during pregnancy (Bell et al., 1993) and supposedly reflect on calving traits. Some potential genes related to calving traits in our study were previously associated with carcass and meat quality traits, including MTUS1 (Albrecht et al., 2016), PLCH1 (Lemos et al., 2016), F2RL1 (Srikanth et al., 2020; Zhang et al., 2017), MYO7B (Doyle et al., 2020; Jia et al., 2020), WWOX (Grigoletto et al., 2020), TFB2M (Jiang et al., 2006; Song et al., 2019), and SMYD3 (De Vos, 2018). Furthermore, F2RL1 was reported to affect the body size in Chinese Holstein cattle (Zhang et al., 2017). Some other genes were identified as essential genes for embryogenesis [SEMA4D (Masuda et al., 2004), and CCNG2 (Ma et al., 2015) and CKS2 (Martinsson-Ahlzén et al., 2008), which contribute to subsequent fetal development]. Perkins et al. (1995) collected human fetal plasma samples both at mid-gestation and parturient to explore the trend of corticotrophin-releasing hormone-binding protein (CRHBP) during the different gestation stages and reported CRHBP as being functional in both maternal and fetal circulation. F2RL2, LIMS2, and LIMS1 were indicated by Forde et al. (2012) as pregnancy-associated genes that are differently expressed in the endometrium of cattle during early pregnancy, indicating the potential role of these genes on successful pregnancy establishment. The SNP rs43354413 located in BTA 3 was identified as significant for GESS on NRR56 and GL, with 10 protein-coding genes in close proximity—for instance, the SSBP3 gene (located approximately 131 kb upstream of this marker) was reported to regulate mouse embryonic stem cell differentiation to trophoblast-like cells (Liu J. et al., 2016). These findings suggest the possible function of SSPB3 of GESS on reproduction performance. We used Chinese Holstein population and re-defined the genetic components of calving traits in current study, but some SNPs (rs42813960 and rs110402487) in our study are consistent with previous genomic studies of calving traits focused on BTA18 (Müller et al., 2017), indicating the potential importance of this chromosome in calving performance. Fang et al. (2019) proposed ZNF613 as a candidate gene for paternal contributions to GL, and in our population, we detected one GL-related marker located downstream of ZNF613 (rs110402487). Furthermore, we also mapped these SNPs to the Animal QTL Database (see Text Footnote 3) and subsequently found that some of them were located in QTLs associated with related traits (Table 6). Particularly, rs108993952 was related to GESS on GL and meanwhile located in the QTL related to some maternal calving traits, which is a promising candidate marker for calving performance.
Sixty-two SNPs were found to be significant for GED, with 13, 12, 9, 17, and 11 being associated with CR, NRR56, CE, SB, and GL, respectively (Table 5). One hundred sixty-seven protein-coding genes were found within 200 kb of those SNPs. rs136577145 was significant for both GESS and GED on GL and was about 63 kb upstream of rs41578821, which was detected as significant for GED on CE. The nearby genes mapped with rs136577145 were TFB2M, CNST, SCCPDH, H3-5, and SMYD3. TFB2M, a mitochondrial transcription specificity factor, as well as SMYD3, a histone lysine methyltransferase, were previously linked to bone and skeletal muscle tissue development (Norrbom et al., 2010; Fujii et al., 2011; Sun et al., 2015)—that is, both paternal- and maternal-derived effects on GL were coincident with growth ability. In addition, WWOX overlapped between GESS and GED on other calving traits, though no gene overlapped between GESS and GED on success traits (Figure 5). This is consistent with the genetic correlation estimates observed (Table 2). The low overlap between GESS and GED on success traits demonstrates that different genes are involved in the insemination outcomes from the dam and service sire contributions.
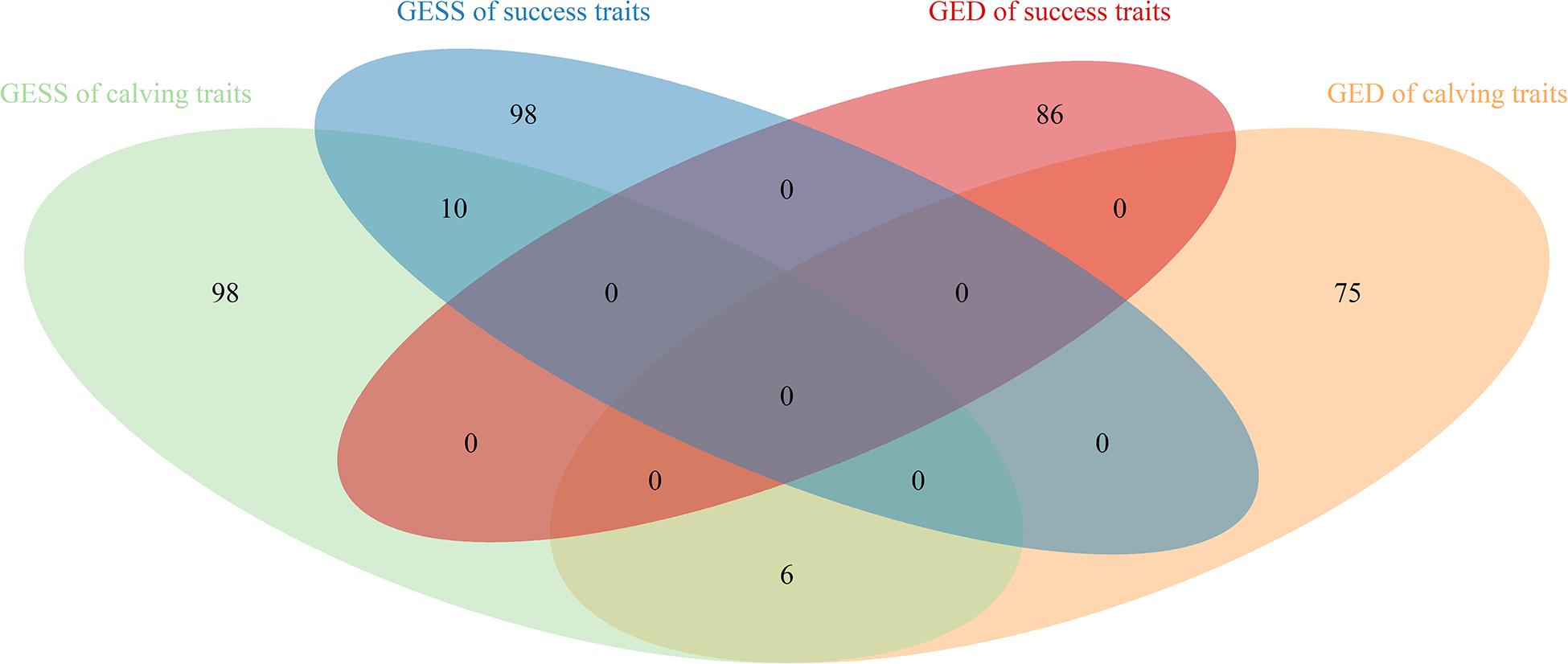
Figure 5. Venn diagram of genes identified for the genetic effect of service sire and genetic effect of dam on success and calving traits in Holstein cattle.
Except for the genes mentioned before, the genomic results of GED are consistent with previous studies (e.g., SMAD4). For GED on success traits, 86 nearby protein-coding genes located 200 kb upstream and downstream of significant SNPs were mapped. Particularly, SMAD4 has been reported to be linked to embryogenesis and folliculogenesis in mice (Chu et al., 2004; Pangas et al., 2006). Lee et al. (2014) reported SMAD4 to be involved in early embryonic development in cattle and to regulate the effects of follistatin, which influences the environment-independent part of the interval from the first to the last insemination in cattle (Zhang et al., 2019). Eighty-one protein-coding genes were mapped for GED on calving traits and previously considered as essential genes for the maternal mechanism during pregnancy, including CXCL10 (Walker et al., 2012), HBEGF (Jessmon et al., 2009), PCDHA13 (Lotfan et al., 2018), TGM2 (Purfield et al., 2019), C1QB (Cochran et al., 2013), CYSTM1 (Purfield et al., 2019), and EPHB2 (Purfield et al., 2015), which were also identified as candidate genes for maternal effect on calving traits in dairy and beef cattle. We also observed that some significant SNPs located in QTLs are associated with related traits (Table 7)—for instance, rs42427669, which was related to GED on NRR56, is located in a QTL region associated with CR (Kiser et al., 2019), while the GL-related marker rs108993952 is located in QTLs associated with CE and SB (Cole et al., 2011).
Functional Enrichment Analyses
The enriched GO terms and KEGG pathways that passed the criteria of the Benjamini–Hochberg-corrected p-value < 0.05 are summarized in the Supplementary Files, and the genes shared between the main terms or pathways are presented in Supplementary Figures 2–5. Noticeably, both GESS and GED on success traits were enriched in neural development-related terms, such as neural crest cell migration (GO:0001755) for GESS on success traits and positive regulation of neuron projection development (GO:0010976) for GED on success traits. For GESS on calving traits, genes were enriched mainly in the thrombin-activated receptor signaling pathway (GO:0070493), microvillus (GO:0005902), neural precursor cell proliferation (GO:0061351), liver development (GO:0001889), N-methyltransferase activity (GO:0008170), sulfotransferase activity (GO:0008146), and cyclin-dependent protein kinase holoenzyme complex (GO:0000307). These categories include functionable genes named CCNG2 and CKS2 (GO:0000307), LIMS2 (GO:0001889 and GO:0061351), MYO7B, WWOX (GO:0005902), SMYD3, TFB2M (GO:0008170), F2RL1, and F2RL2 (GO:0070493) as previously discussed. In addition, the enrichment analysis for GED showed main terms such as CXCR3 chemokine receptor binding (GO:0048248), homophilic cell adhesion via plasma membrane adhesion molecules (GO:0007156), and synapse pruning (GO:0098883), including CXCL10 (GO:0048248), PCDHA13 (GO:0007156), and C1QB (GO:0098883). Therefore, our findings provide further evidence of the possible genetic mechanism of GESS and GED on reproductive performance in Holstein cattle.
Future Prospects
Generally, only the GED for reproduction performance is analyzed in genetic evaluations, though research including the current study have shown the need to dissect GESS (Tiezzi et al., 2011). Compared to previous studies, we fitted an improved model considering the covariance between GESS and GED and also identified candidate genes associated with GESS and GED. The GESS on reproductive traits is small but significant. The low repeatability estimates indicate the poor consistency among repeated records. Therefore, more accurate records and novel traits are required. With recent improvements in data collection, GESS might become an important factor on the genetic evaluation of reproductive performance. Genomic selection is also expected to contribute to improve the accuracy of breeding value for these lowly heritable traits (Rice and Lipka, 2019). Additional analyses with larger datasets and in independent populations (e.g., different breeds) are recommended.
Conclusion
The GESS on reproductive traits is heritable, with a similar genetic variance to the GED. Moreover, the approximate genetic correlation among the GESS and production, health, and type traits is unfavorable for the success traits (CR and NRR56) but favorable for the calving traits (CE, SB, and GL). A total of 100 and 62 significant SNPs were detected to be associated with GESS and GED on those five reproductive traits, respectively. Among them, five genes (BMP4 and CTCFL for success traits and WWOX, TFB2M, and SMYD3 for calving traits) are suggested as important candidate genes for GESS according to positional and functional analyses. As GESS and GED are lowly heritable, genomic prediction might be a promising alternative for breeding schemes aiming to improve fertility performance in dairy cattle.
Data Availability Statement
The data analyzed in this study is subject to the following licenses/restrictions: this manuscript utilizes proprietary data. Requests to access these datasets should be directed to YW, d2FuZ3lhY2h1bkBjYXUuZWR1LmNu.
Ethics Statement
The studies involving animals were reviewed and approved by the Animal Welfare Committee of the China Agricultural University (Protocol Number: DK996).
Author Contributions
ZC and YW conceived the study. ZC and LB designed the analyses, discussed the results, and drafted the manuscript. ZC performed all the data analyses and the data curation of genotype. ZC, HL, and RS prepared the phenotypic files. YC assisted with the modeling analyses. LL and GG provided support for the collection of raw data. All authors have read and approved the final version of the manuscript.
Funding
This study was supported by the China Agriculture Research System of MOF and MARA, the program for Changjiang Scholar and Innovation Research Team in University (IRT_15R62), and the National Agricultural Genetic Improvement Program (2130135). This study also received funding from Beijing Sanyuan Breeding Technology Ltd., Co. funded project (SYZYZ20190005). The funder had the following involvement with the study: study design. All authors declare no other competing interests.
Conflict of Interest
LL is employed by Beijing Dairy Cattle Center. GG is employed by Beijing Sunlon Livestock Development Company Limited.
The remaining authors declare that the research was conducted in the absence of any commercial or financial relationships that could be construed as a potential conflict of interest.
Publisher’s Note
All claims expressed in this article are solely those of the authors and do not necessarily represent those of their affiliated organizations, or those of the publisher, the editors and the reviewers. Any product that may be evaluated in this article, or claim that may be made by its manufacturer, is not guaranteed or endorsed by the publisher.
Acknowledgments
The authors acknowledge Beijing Sanyuan Breeding Technology Company Limited for providing access to the datasets used. The authors also grateful to Guosheng Su from Aarhus University for providing suggestions about the statistical model of the current study and to Qing Xu from Beijing Jiaotong University for giving advice on the genomic analyses.
Supplementary Material
The Supplementary Material for this article can be found online at: https://www.frontiersin.org/articles/10.3389/fgene.2021.713575/full#supplementary-material
Supplementary Figure 1 | The linkage disequilibrium decay of the population.
Supplementary Figure 2 | The Gene Ontology- and Kyoto Encyclopedia of Genes and Genomes-based network analysis of candidate genes of genetic effect of service sire on success traits using ClueGO application in Cytoscape.
Supplementary Figure 3 | The Gene Ontology- and Kyoto Encyclopedia of Genes and Genomes-based network analysis of candidate genes of genetic effect of service sire on calving traits using ClueGO application in Cytoscape.
Supplementary Figure 4 | The Gene Ontology- and Kyoto Encyclopedia of Genes and Genomes-based network analysis of candidate genes of genetic effects of the dam on success traits using ClueGO application in Cytoscape.
Supplementary Figure 5 | The Gene Ontology- and Kyoto Encyclopedia of Genes and Genomes-based network analysis of candidate genes of genetic effects of the dam on calving traits using ClueGO application in Cytoscape.
Abbreviations
GED, genetic effects of the dam; SCR, sire conception rate; GESS, genetic effect of service sire; GWAS, genome-wide association study; CR, conception rate; NRR56, 56-day non-return rate; CE, calving ease; SB, stillbirth; GL, gestation length; SNP, single-nucleotide polymorphism; MAF, minor allele frequency; FDR, false discovery rate; QTL, quantitative trait locus.
Footnotes
- ^ www.afimilk.com.cn
- ^ http://genome.ucsc.edu/cgi-bin/hgLiftOver
- ^ www.animalgenome.org/cgi-bin/QTLdb/BT/index
References
Albrecht, E., Komolka, K., Ponsuksili, S., Gotoh, T., Wimmers, K., and Maak, S. (2016). Transcriptome profiling of Musculus longissimus dorsi in two cattle breeds with different intramuscular fat deposition. Genomics Data 7, 109–111. doi: 10.1016/j.gdata.2015.12.014
Averill, T. A., Rekaya, R., and Weigel, K. (2004). Genetic analysis of male and female fertility using longitudinal binary data. J. Dairy Sci. 87, 3947–3952. doi: 10.3168/jds.s0022-0302(04)73534-1
Azzam, S., Keele, J., and Nielsen, M. (1988). Expectations of heritability estimates for non-return rate of bulls and conception rate of cows. J. Anim. Sci. 66, 2767–2783.
Bagnato, A., and Oltenacu, P. (1993). Genetic study of fertility traits and production in different parities in Italian Friesian cattle. J. Anim. Breed. Genet. 110, 126–134.
Barton, S. C., Surani, M., and Norris, M. (1984). Role of paternal and maternal genomes in mouse development. Nature 311, 374–376. doi: 10.1038/311374a0
Bell, A., Ferrell, C., and Freetly, H. (1993). “Pregnancy and fetal metabolism,” in Quantitative Aspects of Ruminant Digestion and Metabolism, eds J. Dijkstra, J. M. Forbes, and J. France (Ithaca, NY: Department of Animal Science, Cornell University), 405–431.
Benjamini, Y., Drai, D., Elmer, G., Kafkafi, N., and Golani, I. (2001). Controlling the false discovery rate in behavior genetics research. Behav. Brain Res. 125, 279–284. doi: 10.1016/s0166-4328(01)00297-2
Bennett, G., and Gregory, K. (2001). Genetic (co) variances for calving difficulty score in composite and parental populations of beef cattle: II. Reproductive, skeletal, and carcass traits. J. Anim. Sci. 79, 52–59. doi: 10.2527/2001.79152x
Berry, D., Evans, R., and Mc Parland, S. (2011). Evaluation of bull fertility in dairy and beef cattle using cow field data. Theriogenology 75, 172–181. doi: 10.1016/j.theriogenology.2010.08.002
Bindea, G., Mlecnik, B., Hackl, H., Charoentong, P., Tosolini, M., Kirilovsky, A., et al. (2009). ClueGO: a Cytoscape plug-in to decipher functionally grouped gene ontology and pathway annotation networks. Bioinformatics 25, 1091–1093. doi: 10.1093/bioinformatics/btp101
Boichard, D., and Manfredi, E. (1994). Genetic analysis of conception rate in French Holstein cattle. Acta Agric. Scand. A Anim. Sci. 44, 138–145. doi: 10.1080/09064709409410890
Bourdon, R., and Brinks, J. (1982). Genetic, environmental and phenotypic relationships among gestation length, birth weight, growth traits and age at first calving in beef cattle. J. Anim. Sci. 55, 543–553. doi: 10.2527/jas1982.553543x
Browning, B. L., Zhou, Y., and Browning, S. R. (2018). A one-penny imputed genome from next-generation reference panels. Am. J. Hum. Genet. 103, 338–348. doi: 10.1016/j.ajhg.2018.07.015
Buzanskas, M. E., do Amaral Grossi, D., Ventura, R. V., Schenkel, F. S., Chud, T. C. S., Stafuzza, N. B., et al. (2017). Candidate genes for male and female reproductive traits in Canchim beef cattle. J. Anim.l Sci. Biotechnol. 8:67.
Calo, L., McDowell, R., VanVleck, L. D., and Miller, P. (1973). Genetic aspects of beef production among Holstein-Friesians pedigree selected for milk production. J. Anim. Sci. 37, 676–682. doi: 10.2527/jas1973.373676x
Cervantes, I., Gutiérrez, J. P., Fernández, I., and Goyache, F. (2010). Genetic relationships among calving ease, gestation length, and calf survival to weaning in the Asturiana de los Valles beef cattle breed. J. Anim. Sci. 88, 96–101. doi: 10.2527/jas.2009-2066
Chu, G. C., Dunn, N. R., Anderson, D. C., Oxburgh, L., and Robertson, E. J. (2004). Differential requirements for Smad4 in TGFβ-dependent patterning of the early mouse embryo. Development 131, 3501–3512. doi: 10.1242/dev.01248
Cochran, S. D., Cole, J. B., Null, D. J., and Hansen, P. J. (2013). Single nucleotide polymorphisms in candidate genes associated with fertilizing ability of sperm and subsequent embryonic development in cattle. Biol. Reprod. 89:69.
Cole, J. B., Wiggans, G. R., Ma, L., Sonstegard, T. S., Lawlor, T. J., Crooker, B. A., et al. (2011). Genome-wide association analysis of thirty one production, health, reproduction and body conformation traits in contemporary US Holstein cows. BMC Genomics 12:408. doi: 10.1111/j.1365-2052.2005.01337.x
Cole, J., and VanRaden, P. (2018). Symposium review: possibilities in an age of genomics: the future of selection indices. J. Dairy Sci. 101, 3686–3701. doi: 10.3168/jds.2017-13335
Cole, J., Wiggans, G., VanRaden, P., and Miller, R. (2007). Stillbirth (co) variance components for a sire-maternal grandsire threshold model and development of a calving ability index for sire selection. J. Dairy Sci. 90, 2489–2496. doi: 10.3168/jds.2006-436
Crews, D. Jr. (2006). Age of dam and sex of calf adjustments and genetic parameters for gestation length in Charolais cattle. J. Anim. Sci. 84, 25–31. doi: 10.2527/2006.84125x
Cubas, A., Berger, P., and Healey, M. (1991). Genetic parameters for calving ease and survival at birth in Angus field data. J. Anim. Sci. 69, 3952–3958. doi: 10.2527/1991.69103952x
Dadati, E., Kennedy, B., and Burnside, E. (1985). Relationships between conformation and reproduction in Holstein cows: type and calving performance. J. Dairy Sci. 68, 2639–2645. doi: 10.3168/jds.s0022-0302(85)81148-6
De Vos, J. A. (2018). Genome Wide Association Study of Carcass Traits Based on Real Time Ultrasound in South African Nguni Cattle. Hatfield: University of Pretoria.
Devlin, B., and Roeder, K. (1999). Genomic control for association studies. Biometrics 55, 997–1004. doi: 10.1111/j.0006-341x.1999.00997.x
Doyle, J. L., Berry, D. P., Veerkamp, R. F., Carthy, T. R., Evans, R. D., Walsh, S. W., et al. (2020). Genomic regions associated with muscularity in beef cattle differ in five contrasting cattle breeds. Genet. Sel. Evol. 52:2.
Durinck, S., Moreau, Y., Kasprzyk, A., Davis, S., De Moor, B., Brazma, A., et al. (2005). BioMart and Bioconductor: a powerful link between biological databases and microarray data analysis. Bioinformatics 21, 3439–3440. doi: 10.1093/bioinformatics/bti525
Eaglen, S., Coffey, M., Woolliams, J., and Wall, E. (2013). Direct and maternal genetic relationships between calving ease, gestation length, milk production, fertility, type, and lifespan of Holstein-Friesian primiparous cows. J. Dairy Sci. 96, 4015–4025. doi: 10.3168/jds.2012-6229
Egger-Danner, C., Cole, J., Pryce, J., Gengler, N., Heringstad, B., Bradley, A., et al. (2015). Invited review: overview of new traits and phenotyping strategies in dairy cattle with a focus on functional traits. Animal 9, 191–207. doi: 10.1017/s1751731114002614
Eghbalsaied, S. (2011). Estimation of genetic parameters for 13 female fertility indices in Holstein dairy cows. Trop. Anim. Health Prod. 43, 811–816. doi: 10.1007/s11250-010-9767-z
Fang, L., Jiang, J., Li, B., Zhou, Y., Freebern, E., Vanraden, P. M., et al. (2019). Genetic and epigenetic architecture of paternal origin contribute to gestation length in cattle. Commun. Biol. 2:100.
Fatehi, A., Van Den Hurk, R., Colenbrander, B., Daemen, A., Van Tol, H., Monteiro, R., et al. (2005). Expression of bone morphogenetic protein2 (BMP2), BMP4 and BMP receptors in the bovine ovary but absence of effects of BMP2 and BMP4 during IVM on bovine oocyte nuclear maturation and subsequent embryo development. Theriogenology 63, 872–889. doi: 10.1016/j.theriogenology.2004.05.013
Fleming, A., Baes, C. F., Martin, A., Chud, T., Malchiodi, F., Brito, L. F., et al. (2019). Symposium review: the choice and collection of new relevant phenotypes for fertility selection. J. Dairy Sci. 102, 3722–3734. doi: 10.3168/jds.2018-15470
Forde, N., Duffy, G. B., McGettigan, P. A., Browne, J. A., Mehta, J. P., Kelly, A. K., et al. (2012). Evidence for an early endometrial response to pregnancy in cattle: both dependent upon and independent of interferon tau. Physiol. Genomics 44, 799–810. doi: 10.1152/physiolgenomics.00067.2012
Fujii, T., Tsunesumi, S.-I., Yamaguchi, K., Watanabe, S., and Furukawa, Y. (2011). Smyd3 is required for the development of cardiac and skeletal muscle in zebrafish. PLoS One 6:e23491. doi: 10.1371/journal.pone.0023491
Ga, O. J., Schenkel, F., Alcantara, L., Houlahan, K., Lynch, C., and Baes, C. (2021). Estimated genetic parameters for all genetically evaluated traits in Canadian Holsteins. J. Dairy Sci. 104, 9002–9015. doi: 10.3168/jds.2021-20227
Gao, N., Chen, Y., Liu, X., Zhao, Y., Zhu, L., Liu, A., et al. (2019). Weighted single-step GWAS identified candidate genes associated with semen traits in a Duroc boar population. BMC Genomics 20:797.
Grigoletto, L., Ferraz, J., Oliveira, H. R., Eler, J. P., Bussiman, F. O., Abreu Silva, B. C., et al. (2020). Genetic architecture of carcass and meat quality traits in Montana tropical® composite beef cattle. Front. Genet. 11:123.
Guo, G., Guo, X., Wang, Y., Zhang, X., Zhang, S., Li, X., et al. (2014). Estimation of genetic parameters of fertility traits in Chinese Holstein cattle. Can. J. Anim. Sci. 94, 281–285. doi: 10.4141/cjas2013-113
Han, Y., and Peñagaricano, F. (2016). Unravelling the genomic architecture of bull fertility in Holstein cattle. BMC Genet. 17:143.
Hansen, M., Lund, M. S., Pedersen, J., and Christensen, L. (2004). Gestation length in Danish Holsteins has weak genetic associations with stillbirth, calving difficulty, and calf size. Livest. Prod. Sci. 91, 23–33. doi: 10.1016/j.livprodsci.2004.06.007
Heidari, S., Taromchi, A., Nejatbakhsh, R., and Shokri, S. (2018). Expression and localisation of RXFP 3 in human spermatozoa and impact of INSL 7 on sperm functions. Andrologia 50, e12928. doi: 10.1111/and.12928
Heringstad, B., Chang, Y., Svendsen, M., and Gianola, D. (2007). Genetic analysis of calving difficulty and stillbirth in Norwegian Red cows. J. Dairy Sci. 90, 3500–3507. doi: 10.3168/jds.2006-792
Hoekstra, J., Van der Lugt, A., Van der Werf, J., and Ouweltjes, W. (1994). Genetic and phenotypic parameters for milk production and fertility traits in upgraded dairy cattle. Livest. Prod. Sci. 40, 225–232. doi: 10.1016/0301-6226(94)90090-6
Hu, J., Chen, Y.-X., Wang, D., Qi, X., Li, T.-G., Hao, J., et al. (2004). Developmental expression and function of Bmp4 in spermatogenesis and in maintaining epididymal integrity. Dev. Biol. 276, 158–171. doi: 10.1016/j.ydbio.2004.08.034
Huang, J., Guo, F., Zhang, Z., Zhang, Y., Wang, X., Ju, Z., et al. (2016). PCK1 is negatively regulated by bta-miR-26a, and a single-nucleotide polymorphism in the 3’ untranslated region is involved in semen quality and longevity of Holstein bulls. Mol. Reprod. Dev. 83, 217–225. doi: 10.1002/mrd.22613
Jamrozik, J., Fatehi, J., Kistemaker, G., and Schaeffer, L. (2005). Estimates of genetic parameters for Canadian Holstein female reproduction traits. J. Dairy Sci. 88, 2199–2208. doi: 10.3168/jds.s0022-0302(05)72895-2
Jansen, J. (1985). Genetic aspects of fertility in dairy cattle based on analysis of AI data—a review with emphasis on areas for further research. Livest. Prod. Sci. 12, 1–12. doi: 10.1016/0301-6226(85)90036-3
Jaton, C., Schenkel, F., Malchiodi, F., Sargolzaei, M., Price, C., Baes, C., et al. (2017). Genetic analysis for quality of frozen embryos produced by Holstein cattle donors in Canada. J. Dairy Sci. 100, 7320–7329. doi: 10.3168/jds.2017-12851
Jessmon, P., Leach, R. E., and Armant, D. R. (2009). Diverse functions of HBEGF during pregnancy. Mol. Reprod. Dev. 76, 1116–1127. doi: 10.1002/mrd.21066
Jia, C., Li, C., Fu, D., Chu, M., Zan, L., Wang, H., et al. (2020). Identification of genetic loci associated with growth traits at weaning in yak through a genome-wide association study. Anim. Genet. 51, 300–305. doi: 10.1111/age.12897
Jiang, J., Cole, J. B., Da, Y., VanRaden, P. M., and Ma, L. (2018). Fast Bayesian fine-mapping of 35 production, reproduction and body conformation traits with imputed sequences of 27K Holstein bulls. BioRxiv [Preprint]. BioRxiv: 428227,
Jiang, Z., Kunej, T., Wibowo, T. A., Michal, J. J., Zhang, Z., Gaskins, C. T., et al. (2006). “The basal nucleus-encoded mitochondrial transcription genes and meat quality in beef cattle,” in Proceedings of the 8th World Congress on Genetics Applied to Livestock Production (Belo Horizonte: Instituto Prociencia).
Johnson, T., Keehan, M., Harland, C., Lopdell, T., Spelman, R., Davis, S., et al. (2019). Identification of the pseudoautosomal region in the Hereford bovine reference genome assembly ARS-UCD1. 2. J. Dairy Sci. 102, 3254–3258. doi: 10.3168/jds.2018-15638
King, K., Seidel, G. Jr., and Elsden, R. (1985). Bovine embryo transfer pregnancies. II. Lengths of gestation. J. Anim. Sci. 61, 758–762. doi: 10.2527/jas1985.614758x
Kiser, J. N., Keuter, E. M., Seabury, C. M., Neupane, M., Moraes, J. G., Dalton, J., et al. (2019). Validation of 46 loci associated with female fertility traits in cattle. BMC Genomics 20:576.
Koide, Y., Kiyota, T., Tonganunt, M., Pinkaew, D., Liu, Z., Kato, Y., et al. (2009). Embryonic lethality of fortilin-null mutant mice by BMP-pathway overactivation. Biochim. Biophys. Acta (BBA) General Subjects 1790, 326–338. doi: 10.1016/j.bbagen.2009.01.012
Kuhn, M., and Hutchison, J. (2008). Prediction of dairy bull fertility from field data: use of multiple services and identification and utilization of factors affecting bull fertility. J. Dairy Sci. 91, 2481–2492. doi: 10.3168/jds.2007-0743
Lee, K.-B., Zhang, K., Folger, J. K., Knott, J. G., and Smith, G. W. (2014). Evidence supporting a functional requirement of SMAD4 for bovine preimplantation embryonic development: a potential link to embryotrophic actions of follistatin. Biol. Reprod. 91:62.
Lemos, M. V., Chiaia, H. L. J., Berton, M. P., Feitosa, F. L., Aboujaoud, C., Camargo, G. M., et al. (2016). Genome-wide association between single nucleotide polymorphisms with beef fatty acid profile in Nellore cattle using the single step procedure. BMC Genomics 17:213.
Li, C. W., and Ge, W. (2011). Spatiotemporal expression of bone morphogenetic protein family ligands and receptors in the zebrafish ovary: a potential paracrine-signaling mechanism for oocyte-follicle cell communication. Biol. Reprod. 85, 977–986. doi: 10.1095/biolreprod.111.092239
Li, G., Khateeb, K., Schaeffer, E., Zhang, B., and Khatib, H. (2012a). Genes of the transforming growth factor-beta signalling pathway are associated with pre-implantation embryonic development in cattle. J. Dairy Res. 79:310. doi: 10.1017/s0022029912000210
Li, G., Peñagaricano, F., Weigel, K., Zhang, Y., Rosa, G., and Khatib, H. (2012b). Comparative genomics between fly, mouse, and cattle identifies genes associated with sire conception rate. J. Dairy Sci. 95, 6122–6129. doi: 10.3168/jds.2012-5591
Li, N., Pan, S., Zhu, H., Mu, H., Liu, W., and Hua, J. (2014). BMP4 promotes SSEA-1+ hUC-MSC differentiation into male germ-like cells in vitro. Cell Prolif. 47, 299–309. doi: 10.1111/cpr.12115
Lillehammer, M., Meuwissen, T., and Sonesson, A. (2011). A comparison of dairy cattle breeding designs that use genomic selection. J. Dairy Sci. 94, 493–500. doi: 10.3168/jds.2010-3518
Liu, J., Luo, X., Xu, Y., Gu, J., Tang, F., Jin, Y., et al. (2016). Single-stranded DNA binding protein Ssbp3 induces differentiation of mouse embryonic stem cells into trophoblast-like cells. Stem Cell Res. Ther. 7:79.
Liu, X., Huang, M., Fan, B., Buckler, E. S., and Zhang, Z. (2016). Iterative usage of fixed and random effect models for powerful and efficient genome-wide association studies. PLoS Genet. 12:e1005767. doi: 10.1371/journal.pgen.1005767
Lotfan, M., Ali, S. A., Yadav, M. L., Choudhary, S., Jena, M. K., Kumar, S., et al. (2018). Genome-wide gene expression analysis of 45 days pregnant fetal cotyledons vis-a-vis non-pregnant caruncles in buffalo (Bubalus bubalis). Gene 654, 127–137. doi: 10.1016/j.gene.2018.02.038
Luo, M., Boettcher, P., Dekkers, J., and Schaeffer, L. (1999). Bayesian analysis for estimation of genetic parameters of calving ease and stillbirth for Canadian Holsteins. J. Dairy Sci. 82, 1848. e1-e11.
Luo, M., Boettcher, P., Schaeffer, L., and Dekkers, J. (2002). Estimation of genetic parameters of calving ease in first and second parities of Canadian Holsteins using Bayesian methods. Livest. Prod. Sci. 74, 175–184. doi: 10.1016/s0301-6226(01)00294-9
Ma, L., Liu, X., Wang, F., He, X., Chen, S., and Li, W. (2015). Different donor cell culture methods can influence the developmental ability of cloned sheep embryos. PLoS One 10:e0135344. doi: 10.1371/journal.pone.0135344
Madsen, P., Sørensen, P., Su, G., Damgaard, L. H., Thomsen, H., and Labouriau, R, eds (2006). “DMU-a package for analyzing multivariate mixed models,” in Proceedings of the 8th World Congress on Genetics Applied to Livestock Production, Belo Horizonte.
Martinsson-Ahlzén, H.-S., Liberal, V., Grünenfelder, B., Chaves, S. R., Spruck, C. H., and Reed, S. I. (2008). Cyclin-dependent kinase-associated proteins Cks1 and Cks2 are essential during early embryogenesis and for cell cycle progression in somatic cells. Mol. Cell Biol. 28, 5698–5709. doi: 10.1128/mcb.01833-07
Masuda, K., Furuyama, T., Takahara, M., Fujioka, S., Kurinami, H., and Inagaki, S. (2004). Sema4D stimulates axonal outgrowth of embryonic DRG sensory neurones. Genes Cells 9, 821–829. doi: 10.1111/j.1365-2443.2004.00766.x
McClintock, S., Beard, K., Gilmour, A., and Goddard, M. (2003). Relationships between calving traits in heifers and mature cows in Australia. Interbull. Bull. 31:102.
Meijering, A. (1985). Sire evaluation for calving traits by Best Linear Unbiased Prediction and nonlinear methodology. J. Anim. Breed. Genet. 102, 95–105. doi: 10.1111/j.1439-0388.1985.tb00677.x
Miglior, F., Gong, W., Wang, Y., Kistemaker, G., Sewalem, A., and Jamrozik, J. (2009). Genetic parameters of production traits in Chinese Holsteins using a random regression test-day model. J. Dairy Sci. 92, 4697–4706. doi: 10.3168/jds.2009-2212
Mujibi, F., and Crews, D. Jr. (2009). Genetic parameters for calving ease, gestation length, and birth weight in Charolais cattle. J Anim Sci 87, 2759–2766. doi: 10.2527/jas.2008-1141
Müller, M.-P., Rothammer, S., Seichter, D., Russ, I., Hinrichs, D., Tetens, J., et al. (2017). Genome-wide mapping of 10 calving and fertility traits in Holstein dairy cattle with special regard to chromosome 18. J. Dairy Sci. 100, 1987–2006. doi: 10.3168/jds.2016-11506
Murray, B., Schaeffer, L., and Burnside, E. (1977). Estimation of breeding values for non return rates of Canadian Holstein Friesian sires. Dairy Ind. Res. Rep. 1977, 30–33.
Muuttoranta, K., Tyrisevä, A.-M., Mäntysaari, E. A., Pösö, J., Aamand, G. P., and Lidauer, M. H. (2019). Genetic parameters for female fertility in Nordic Holstein and Red Cattle dairy breeds. J. Dairy Sci. 102, 8184–8196. doi: 10.3168/jds.2018-15858
Nilsson, E. E., and Skinner, M. K. (2003). Bone morphogenetic protein-4 acts as an ovarian follicle survival factor and promotes primordial follicle development. Biol. Reprod. 69, 1265–1272. doi: 10.1095/biolreprod.103.018671
Norman, H., Hutchison, J., and Wright, J. (2008). Sire Conception Rate: New National Ai Bull Fertility Evaluation. AIPL Res Report SCR1 (7-08). Washington, DC: USDA.
Norrbom, J., Wallman, S., Gustafsson, T., Rundqvist, H., Jansson, E., and Sundberg, C. (2010). Training response of mitochondrial transcription factors in human skeletal muscle. Acta Physiol. 198, 71–79. doi: 10.1111/j.1748-1716.2009.02030.x
Ou, J., Li, Y., Wang, Z., Jin, C., Li, K., Lu, Y., et al. (2020). Lrrc34 is highly expressed in sscs and is necessary for SSC expansion in vitro. Chin. Med. Sci. J. 35, 20–30.
Pangas, S. A., Li, X., Robertson, E. J., and Matzuk, M. M. (2006). Premature luteinization and cumulus cell defects in ovarian-specific Smad4 knockout mice. Mol. Endocrinol. 20, 1406–1422. doi: 10.1210/me.2005-0462
Peddinti, D., Memili, E., and Burgess, S. C. (2010). Proteomics-based systems biology modeling of bovine germinal vesicle stage oocyte and cumulus cell interaction. PLos One 5:e11240. doi: 10.1371/journal.pone.0011240
Perkins, A., Wolfe, C., Eben, F., Soothill, P., and Linton, E. (1995). Corticotrophin-releasing hormone-binding protein in human fetal plasma. J. Endocrinol. 146, 395–401. doi: 10.1677/joe.0.1460395
Purfield, D., Bradley, D., Kearney, J., Evans, R., and Berry, D. (2015). Genome-wide association using high density genotypes for calving difficulty in dairy and beef cattle. Genet. Sel. Evol. 47:47.
Purfield, P. C., Evans, R. E., Carthy, T. R., and Berry, D. P. (2019). Genomic regions associated with gestation length detected using whole-genome sequence data differ between dairy and beef cattle. Front. Genet. 10:1068.
Raheja, K., Nadarajah, K., and Burnside, E. (1989). Relationship of bull fertility with daughter fertility and production traits in Holstein dairy cattle. J. Dairy Sci. 72, 2679–2682. doi: 10.3168/jds.s0022-0302(89)79409-1
Ramal-Sanchez, M., Bernabo, N., Tsikis, G., Blache, M.-C., Labas, V., Druart, X., et al. (2020). Progesterone induces sperm release from oviductal epithelial cells by modifying sperm proteomics, lipidomics and membrane fluidity. Mol. Cell Endocrinol. 504:110723. doi: 10.1016/j.mce.2020.110723
Rangaswami, H., Bulbule, A., and Kundu, G. C. (2006). Osteopontin: role in cell signaling and cancer progression. Trends Cell Biol. 16, 79–87. doi: 10.1016/j.tcb.2005.12.005
Rice, B., and Lipka, A. E. (2019). Evaluation of RR-BLUP genomic selection models that incorporate peak genome-wide association study signals in maize and sorghum. Plant Genome 12, 1–14.
Shimizu, T., Yokoo, M., Miyake, Y., Sasada, H., and Sato, E. (2004). Differential expression of bone morphogenetic protein 4–6 (BMP-4,-5, and-6) and growth differentiation factor-9 (GDF-9) during ovarian development in neonatal pigs. Domest. Anim. Endocrinol. 27, 397–405. doi: 10.1016/j.domaniend.2004.04.001
Silvia, W., Hatler, T., Nugent, A., and Da Fonseca, L. L. (2002). Ovarian follicular cysts in dairy cows: an abnormality in folliculogenesis. Domest. Anim. Endocrinol. 23, 167–177. doi: 10.1016/s0739-7240(02)00154-6
Sokal, R. R., and Rohlf, F. J. (1981). Biometry: The Principles And Practice of Statistics in Biological Research. San Francisco, CA: W.H. Freeman.
Song, Q., Zhang, W., Wu, F., Zhang, J., Xu, M., Li, H., et al. (2019). Cloning and expression levels of TFAM and TFB2M genes and their correlation with meat and CarCass quality traits in Jiaxing BlaCk pig. Ann. Anim. Sci. 19, 327–341. doi: 10.2478/aoas-2018-0056
Srikanth, K., Lee, S.-H., Chung, K.-Y., Park, J.-E., Jang, G.-W., Park, M.-R., et al. (2020). A gene-set enrichment and protein–protein interaction network-based GWAS with regulatory SNPs identifies candidate genes and pathways associated with carcass traits in Hanwoo cattle. Genes 11:316. doi: 10.3390/genes11030316
Steinbock, L., Näsholm, A., Berglund, B., Johansson, K., and Philipsson, J. (2003). Genetic effects on stillbirth and calving difficulty in Swedish Holsteins at first and second calving. J. Dairy Sci. 86, 2228–2235. doi: 10.3168/jds.s0022-0302(03)73813-2
Su, G., Lund, M. S., and Sorensen, D. (2007). Selection for litter size at day five to improve litter size at weaning and piglet survival rate. J. Dairy Sci. 85, 1385–1392. doi: 10.2527/jas.2006-631
Sun, C., and Su, G. (2010). Comparison on models for genetic evaluation of non-return rate and success in first insemination of the Danish Holstein cows. Livest. Sci. 127, 205–210. doi: 10.1016/j.livsci.2009.09.015
Sun, Y., Luo, W., Xie, H., Zhang, Y., and Cai, H. (2015). Analysis of association between polymorphism of TFB2M gene and meat quality, growth and slaughter traits in Guizhou White Goat, a well-known Chinese indigenous goat breed. Pak. J. Zool. 47, 1605–1610.
Taylor, J. F., Schnabel, R. D., and Sutovsky, P. (2018). Genomics of bull fertility. Animal 12, s172–s183.
Tiezzi, F., Maltecca, C., Penasa, M., Cecchinato, A., and Bittante, G. (2013). Genetic analysis of dairy bull fertility from field data of Brown Swiss cattle. J. Dairy Sci. 96, 7325–7328. doi: 10.3168/jds.2013-6885
Tiezzi, F., Penasa, M., Maltecca, C., Cecchinato, A., and Bittante, G. (2011). Exploring different model structures for the genetic evaluation of dairy bull fertility. Agric. Conspec. Sci. 76, 239–243.
Van Tassell, C., Wiggans, G., and Misztal, I. (2003). Implementation of a sire-maternal grandsire model for evaluation of calving ease in the United States. J. Dairy Sci. 86, 3366–3373. doi: 10.3168/jds.s0022-0302(03)73940-x
Vanderick, S., Troch, T., Gillon, A., Glorieux, G., and Gengler, N. (2014). Genetic parameters for direct and maternal calving ease in W alloon dairy cattle based on linear and threshold models. J. Anim. Breed. Genet. 131, 513–521. doi: 10.1111/jbg.12105
VanRaden, P., Sanders, A., Tooker, M., Miller, R., Norman, H., Kuhn, M., et al. (2004). Development of a national genetic evaluation for cow fertility. J. Dairy Sci. 87, 2285–2292. doi: 10.3168/jds.s0022-0302(04)70049-1
Vieira-Neto, A., Galvão, K., Thatcher, W., and Santos, J. (2017). Association among gestation length and health, production, and reproduction in Holstein cows and implications for their offspring. J. Dairy Sci. 100, 3166–3181. doi: 10.3168/jds.2016-11867
Walker, C. G., Littlejohn, M. D., Mitchell, M. D., Roche, J. R., and Meier, S. (2012). Endometrial gene expression during early pregnancy differs between fertile and subfertile dairy cow strains. Physiol. Genomics 44, 47–58. doi: 10.1152/physiolgenomics.00254.2010
Weigel, K., and Rekaya, R. (2000). Genetic parameters for reproductive traits of Holstein cattle in California and Minnesota. J. Dairy Sci. 83, 1072–1080. doi: 10.3168/jds.s0022-0302(00)74971-x
Weintraub, A. S., Lin, X., Itskovich, V. V., Aguinaldo, J. G. S., Chaplin, W. F., Denhardt, D. T., et al. (2004). Prenatal detection of embryo resorption in osteopontin-deficient mice using serial noninvasive magnetic resonance microscopy. Pediatric Res. 55, 419–424. doi: 10.1203/01.pdr.0000112034.98387.b2
Wiggans, G., Cooper, T., VanRaden, P., and Cole, J. (2011). Adjustment of traditional cow evaluations to improve accuracy of genomic predictions. J. Dairy Sci. 94, 6188–6193. doi: 10.3168/jds.2011-4481
Wiggans, G., Misztal, I., and Van Tassell, C. (2003). Calving ease (co) variance components for a sire-maternal grandsire threshold model. J. Dairy Sci. 86, 1845–1848. doi: 10.3168/jds.s0022-0302(03)73771-0
Wu, X., Fang, M., Liu, L., Wang, S., Liu, J., Ding, X., et al. (2013). Genome wide association studies for body conformation traits in the Chinese Holstein cattle population. BMC Genomics 14:897.
Zhang, X., Chu, Q., Guo, G., Dong, G., Li, X., Zhang, Q., et al. (2017). Genome-wide association studies identified multiple genetic loci for body size at four growth stages in Chinese Holstein cattle. PLoS One 12:e0175971.
Keywords: dairy cattle, genetic evaluation, genome-wide association study, paternal effect, reproductive traits, service sire
Citation: Chen Z, Brito LF, Luo H, Shi R, Chang Y, Liu L, Guo G and Wang Y (2021) Genetic and Genomic Analyses of Service Sire Effect on Female Reproductive Traits in Holstein Cattle. Front. Genet. 12:713575. doi: 10.3389/fgene.2021.713575
Received: 23 May 2021; Accepted: 03 August 2021;
Published: 03 September 2021.
Edited by:
Lingzhao Fang, The University of Edinburgh, United KingdomReviewed by:
Lubos Vostry, Czech University of Life Sciences Prague, CzechiaChao Ning, Shandong Agricultural University, China
Jicai Jiang, North Carolina State University, United States
Copyright © 2021 Chen, Brito, Luo, Shi, Chang, Liu, Guo and Wang. This is an open-access article distributed under the terms of the Creative Commons Attribution License (CC BY). The use, distribution or reproduction in other forums is permitted, provided the original author(s) and the copyright owner(s) are credited and that the original publication in this journal is cited, in accordance with accepted academic practice. No use, distribution or reproduction is permitted which does not comply with these terms.
*Correspondence: Gang Guo, Z3VvZ2FuZzIxODBAMTI2LmNvbQ==; Yachun Wang, d2FuZ3lhY2h1bkBjYXUuZWR1LmNu