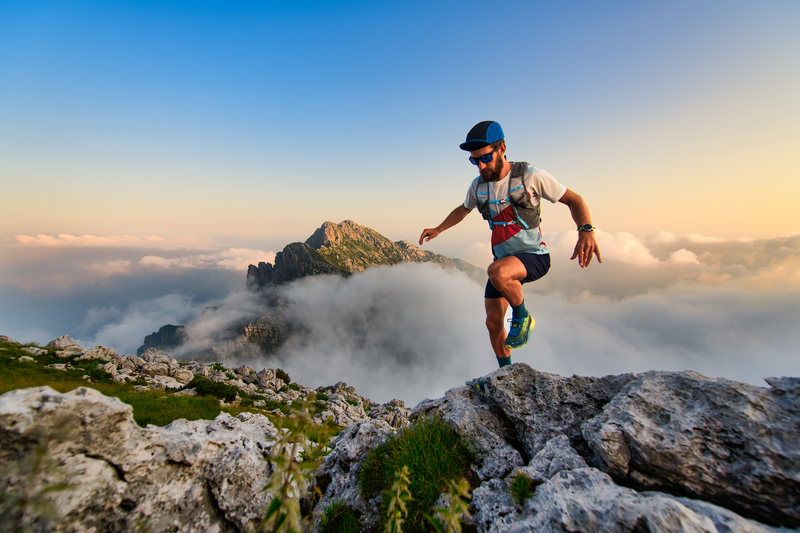
94% of researchers rate our articles as excellent or good
Learn more about the work of our research integrity team to safeguard the quality of each article we publish.
Find out more
ORIGINAL RESEARCH article
Front. Genet. , 13 July 2021
Sec. Cancer Genetics and Oncogenomics
Volume 12 - 2021 | https://doi.org/10.3389/fgene.2021.698595
Background: Hereditary cancer predisposition syndromes account for approximately 10% of cancer cases. Next generation sequencing (NGS) based multi-gene targeted panels is now a frontline approach to identify pathogenic mutations in cancer predisposition genes in high-risk families. Recent evolvement of NGS technologies have allowed simultaneous detection of sequence and copy number variants (CNVs) using a single platform. In this study, we have analyzed frequency and nature of sequence variants and CNVs, in a Canadian cohort of patients, suspected with hereditary cancer syndrome, referred for genetic testing following specific genetic testing guidelines based on patient’s personal and/or family history of cancer.
Methods: A 2870 patients were subjected to a single NGS based multi-gene targeted hereditary cancer panel testing algorithm to identify sequence variants and CNVs in cancer predisposition genes at our reference laboratory in Southwestern Ontario. CNVs identified by NGS were confirmed by alternative techniques like Multiplex ligation-dependent probe amplification (MLPA).
Results: A 15% (431/2870) patients had a pathogenic variant and 36% (1032/2870) had a variant of unknown significance (VUS), in a cancer susceptibility gene. A total of 287 unique pathogenic variant were identified, out of which 23 (8%) were novel. CNVs identified by NGS based approach accounted for 9.5% (27/287) of pathogenic variants, confirmed by alternate techniques with high accuracy.
Conclusion: This study emphasizes the utility of NGS based targeted testing approach to identify both sequence and CNVs in patients suspected with hereditary cancer syndromes in clinical setting and expands the mutational spectrum of high and moderate penetrance cancer predisposition genes.
Hereditary cancer predisposition syndromes account for up to 10% of all diagnosed cancer cases. Initially, primary genetic testing was limited to the high penetrance genes BRCA1 and/or BRCA2, which account for approximately 3–5% of breast cancers and 12–15% of ovarian cancers in most populations (Balmaña et al., 2011; Lang et al., 2017; Li et al., 2019). However, it is now established that additional hereditary cancer predisposition syndromes are linked to an ever increasing number of genes, including but not limited to TP53, CDH1, SKT11, PTEN, PALB2, MLH1, MSH2, PMS1, PMS2, and MSH6, which have also been associated with increased risk of breast, ovarian, and other cancers, often as part of more complex family histories including colon, endometrial, gastric, brain, and/or other cancers (Rahman et al., 2007; Roberts et al., 2018; Schon and Tischkowitz, 2018). Advances in next generation sequencing (NGS) technologies have uncovered a variety of new genes conferring variable levels of cancer risk. Ease of testing of multiple genes simultaneously, along with reduced cost and rapid turnaround times has enabled implementation of this technique as a frontline clinical test for individuals suspected to have a familial cancer syndrome (Okur and Chung, 2017). NGS technologies have also been implemented in rapid, cost-effective and high-throughput identification of copy number variants (CNVs) along with sequence variants. CNVs constitute a class of structural genetic variant involving increase or decrease in the number of copies of specific regions of DNA. CNVs in cancer susceptibility genes constitute for a significant number of pathogenic variants (PVs) (approximately 7%) and can be identified using NGS based algorithms with significant reduction in turnaround times and cost (Mancini-DiNardo et al., 2019).
Our laboratory has previously validated a unique NGS-based pipeline for detection of sequence and copy number variants (CNVs), in a panel of known cancer susceptibility genes and have been serving as a reference laboratory for genetic susceptibility testing for cancer within the province of Ontario, Canada for the past two decades (Schenkel et al., 2016; Kerkhof et al., 2017). In the present study we have analyzed genetic test results in 2870 patients analyzed using this NGS pipeline for hereditary cancer gene predisposition, referred for genetic testing to Molecular Diagnostics Laboratory the London Health Sciences Centre (LHSC), a provincial testing center in Southwestern Ontario. This testing is publicly funded for patients who meet eligibility criteria specified by the Ministry of Health and Long-term Care (MOHLTC).
The largest subset of these patients had been referred due to a personal or family history of hereditary breast and/or ovarian cancer, and were typically identified using provincial [Ontario Breast Screening Program (OBSP)]1 guidelines for referral, using a similar approach to that described by the National Comprehensive Cancer Network (NCCN)2. However significant subset of our patients was referred due to a personal and/or family history of other types of cancers, including uterine, colorectal, gastric, and/or other cancers, as well as colonic polyposis.
In this study we have described the spectrum of reportable genetic sequence variants identified, which include a significant proportion of deleterious and previously unreported sequence variants and CNVs which by using this approach can be identified routinely at a sub-exon resolution for multiple genes, in parallel, with high accuracy. We have analyzed frequency of variants in moderate and high penetrance gene among patients presenting with various cancer types.
This study involved assessment of anonymized and de-identified data from a clinical laboratory database, including genetic test findings along with key clinical and demographic features, at the Molecular Diagnostics Laboratory at London Health Sciences Centre during time period from January 2016 to May 2018. All clinical and demographic details in the laboratory database were obtained from the Test Request Forms (TRFs), submitted by the ordering physician or healthcare provider, that is kept as part of routine laboratory quality control protocols. The guidelines for referral for genetic testing have been described in Supplementary Table 1. Blood samples were received by the laboratory as part of routine clinical diagnostic testing, for which patients received counseling and provided informed consent in reference cancer genetics clinics in Ontario. Anonymized summary data in the laboratory Quality Management database was presented in compliance with the lab Quality Improvement protocol. The genomic DNA was isolated by standard protocols using the MagNA Pure system (Roche Diagnostics, Laval, QC, Canada).
A custom in-house developed Comprehensive Cancer Panel initially included 26 known cancer predisposition genes (comprehensive version v1), while subsequent updates and expansions of this panel are designated as v2 and v3 included 31 and 38 genes, respectively (Genes listed in Supplementary Table 2). Two subpanels (for which genes were bioinformatically filtered and reported from the comprehensive panel), included genes associated with hereditary breast and ovarian cancer syndrome (24 genes in v.3), and hereditary gastric and/or colorectal cancer syndrome (24 genes in v.3). All samples underwent target enrichment and sequencing for the genes included in the comprehensive panel, however analysis and reporting was carried out according to the predefined subset of genes as per the ordering physician’s submitted requisition form (Comprehensive; Breast and Ovarian Cancer; Gastric and Colorectal Cancer; HP16). Genes included in each panel and subsequent versions are described in Supplementary Table 2 and number of patients tested on each panel is shown in Figure 1. Our panel content was designed to meet the requirements set forth by the Ministry of Health and Long term Care of Ontario and is based on the clinical guidelines and recommendations including Cancer Care Ontario’s OBSP (see text footnote 1) and NIH ClinGen expert recommendations3 and National Centre for Biotechnology Information (NCBI) Gene Reviews4 along with genes reported in literature (Lee et al., 2019; Seifert et al., 2019).
Figure 1. Number of patients tested for hereditary cancer predisposition with multi gene targeted panel test: This chart describes number of patients tested with each sub-panel and versions. Each sub-panel and its respective version constitute of a set of genes associated with increased predisposition to specific cancers (List of genes included is described in Supplementary Table 2).
Custom sequence capture probes were designed using the SeqCap EZ Choice Library system (Roche NimbleGen, Inc., Madison, WI, United States). The design included enrichment for all coding exons as well as 20 bp of the flanking intronic regions. Normally, 100 ng of genomic DNA was used for library preparations. Library preparation and target capturing sequencing steps were performed as previously described (Schenkel et al., 2016; Kerkhof et al., 2017).
Sequence alignment, coverage distribution and variant identification were performed with NextGene software version 2.4.1 (SoftGenetics, LLC) using standard alignment settings. BAM and VCF files were imported into Geneticist Assistant version1.1.5 (SoftGenetics, LLC) for quality control assessment as previously described (Schenkel et al., 2016; Kerkhof et al., 2017). Variants were analyzed by a clinical molecular geneticist and classified for pathogenicity as per published American College of Medical Genetics (ACMG) guidelines (Richards et al., 2015). All of the variants classified as ACMG 1 (pathogenic), 2 (Likely pathogenic), or 3 [variant of unknown significance (VUS)] were confirmed by sanger sequencing or targeted molecular assessment as appropriate.
CNV analysis was performed following methods previously validated in our laboratory (Susswein et al., 2016). Briefly, coverage distribution reports were generated using NextGene software v2.4.1 (SoftGenetics). The normalization factor for each sample included in the capture was identified based on total coverage and then used to normalize each nucleotide of the entire panel. The normalized nucleotide value was then graphed using Excel v.14.0.6129.5000 (Microsoft Corporation) to identify exon as well as sub-exon variants. Deletions were identified by a ratio of ≤0.65 and duplications were identified by a ratio of ≥1.35. These cutoff values were calculated based on internal laboratory reference analysis as previously described. A four-allele normalization method was adapted to address pseudogene involvement (or another homologous region) for example PMS2 gene. The coverage for the four alleles was totaled at each nucleotide position and normalization algorithm was performed. Deletions were defined by a mean ratio of ≤0.8 (3/4 alleles), whereas duplications were defined by a ratio of ≥1.2 (5/4 alleles). CNVs identified by this NGS analysis pipeline were confirmed and characterized by a second clinically validated method, including Multiplex Ligation-dependent Probe Amplification (MLPA), which was used to confirm CNVs in 35 patients. In three patients, breakpoints were identified using Long Range PCR (LR-PCR) followed by Sanger sequencing. All variants are reported using HGVS nomenclature (den Dunnen et al., 2016).
Demographic details of the 2870 patients assessed in the study are included in Table 1. The number of patients tested on each subpanel (as per referring physician) is shown in Figure 1. Genetic variants were analyzed and classified according to criteria described by ACMG in five specific categories: ACMG 1 (pathogenic), ACMG 1 (likely pathogenic), ACMG 3 (variant of uncertain significance), ACMG 4 (likely benign), and ACMG 5 (Benign) (Richards et al., 2015). Genetic variants classified as ACMG 1, 2, and 3 were included in the patient’s test reports. For further analysis in this study, all variants categorized as ACMG 1 and 2 are referred to as PV and ACMG 3 variants are referred to as VUSs. Majority of patients in the cohort were female (90.3%; 2593/2870). Although a direct comparison is not possible due to a marked difference in sample size, higher frequency of PV was seen in males (21.3% vs. 14.3%). More than 50% of patients were in the age group of 50–70 years at the time of testing, and PV detection rates were slightly higher in younger age groups (<60 years). Ancestral origin or ethnicity information was available for 2181/2870 (76%) patients. Of these, approximately 84% (1828/2181) were of European ancestry, and 16% were from other ethnicities, including Asian, African, Indigenous Canadian, South American and mixed race (Table 1).
Out of 2870 patients, 431 tested positive for a PV, accounting for a 15% overall detection rate (including MUTYH mono-allelic and bi-allelic variants). Patients tested with the comprehensive panel had a slightly higher rate of PVs (16.2%) compared to other sub-panels, 13.6% for the Breast and Ovarian Cancer sub-panel (Supplementary Table 3). Although the number of patients analyzed with the Gastric and Colorectal Cancer sub-panel was relatively less, the PV detection rates were highest for this sub-panel (22%; 24 out of 109 tested positive for a PV). A total of 1285 reportable variants were identified; 287 (22%) were pathogenic (PV), and 998 (78%) were VUSs, (Figure 2). All variants identified in the study are listed in Supplementary Table 4. Ninety-two percent of PVs detected in this study have previously been described in the literature and/or ClinVar database, and 23 (8%) were novel, i.e., did not appear to be reported elsewhere (listed in Supplementary Table 5).
Figure 2. Frequency of pathogenic variants and variants of unknown significance identified in the study. This figure gives information about frequency of different types of variants (A) Pie chart showing number of pathogenic variants (ACMG 1 and 2) and ACMG 3 (variants of unknown significance), (B) Pie chart showing frequency of different types of pathogenic variants (ACMG 1 and 2), (C) Table showing frequency of copy number variants (CNVs) and sequence variants.
The number of patients with a PV in a high or moderate penetrance gene (as defined by NCCN; see text footnote 2), presenting with specific cancer phenotype is listed in Tables 2, 3. Overall the major cancer types seen in patients were breast, ovarian, colorectal, gastric, uterine, pancreas, prostate, brain and colonic polyposis. BRCA1 and BRCA2 genes had highest frequency of PVs among patients with ovarian cancer (5.4% in BRCA1 and 4% in BRCA2) followed by patients with breast cancer (2.1% in BRCA1 and 1.7% in BRCA2). Mismatch repair (MMR), genes had the highest frequency of PVs in the sub-group of patients presenting with colorectal cancer, (MLH1; 3%), MSH2; 1.8%, MSH6; 3%, PMS2; 1.1%) or uterine cancer (MLH1; 2.6%) and MSH2, MSH6, PMS2 with1.3% each). Among patients presenting with colonic polyposis, APC gene had highest frequency of PVs (APC;4%) (Table 2). Out of 2870 patients tested, 2730 were diagnosed with cancer and 409 (14.9%) of these had a PV. About 140 patients were not diagnosed with any cancer, and had been referred for genetic testing based on criteria involving their family cancer history; 22 of these patients (15.7%) were positive for a PV in a cancer susceptibility gene.
Table 3. Frequency of pathogenic variants in BRCA1 and 2 genes, other high and moderate penetrance genes in various high-risk categories of patients with breast and/or ovarian cancer.
Majority of this patient cohort (2003 out of 2870) patients presented with breast and/or ovarian cancer, and the frequency of patients with a PV in a cancer susceptibility gene is shown in Figure 3. Out of these patients the majority had been diagnosed with breast cancer (1548/2870; 54%: including 49 patients diagnosed with both breast and ovarian cancer) followed by patients with isolated ovarian cancer, (455/2870; 16%). PVs were detected in 12.5% of breast cancer patients, and 18% of ovarian cancer patients; the major genes and frequency of PVs are described in Figures 3, 4. Approximately 5% of patients presenting with breast cancer had a PV in one of the high penetrance genes, (BRCA1, BRCA2, TP53, PALB2, CDH1, and PTEN), and an additional 7.5% patients had a PV in one of the moderate penetrance genes (Table 2). Among ovarian cancer patients more than 9% harbored PVs in BRCA1 and BRCA2 genes. Notably, in patients with ovarian cancer, a significant number of PVs were identified in the moderate penetrance RAD51 genes; RAD51C (1.5%) and RAD51D (1%), followed by the MMR and ATM genes (Table 2).
Figure 3. Graph showing distribution of pathogenic variants (PVs) in patients with breast and/or ovarian cancer: Patients presenting with Breast and/or Ovarian cancer (n = 2003). 13.7% (276/2003) patients tested positive for a PV. Genes with PVs are listed on the X-axis; Number of patients positive for a pathogenic variant is shown on Y-axis.
Figure 4. Graph showing distribution of pathogenic variants (PVs) in patients with ovarian cancer: Patients presenting with Ovarian cancer (n = 455). 18%(82/455) patients tested positive for a PV. Genes with PVs are listed on the X-axis; Number of patients positive for a pathogenic variant is shown on Y-axis.
Approximately 11% of patients presented with gastrointestinal (GI), tract related disease; 6% with colorectal cancer, 3.4% with polyposis, and 1.7% with gastric and/or other GI tract cancers, (Table 2). Overall the PV detection rate was highest in this group: i.e., 19% with colorectal cancer, 21% with polyposis, and 21.5% with gastric cancer (Table 2). The major genes with PVs in patients with colorectal cancer included the MMR genes MLH1, MSH2, PMS2, and MSH6, (accounting for 9% of patients with a PV), followed by APC, TP53, MUTYH, BARD1, ATM, and BRIP1. Patients presenting with polyposis tested positive for PVs in APC (4%), MUTYH (2%), PMS2 (2%), MLH1 (2%), TP53 (2%), and to a lesser extent in PTEN, SMAD4, and ATM. Among patients with gastric and other GI tract cancer, PV’s were found in CDH1, TP53, ATM, MSH2, and PMS2 genes (Table 2).
Cancer types seen less frequently in our study included uterine (76 patients; 2.6%), pancreatic (24 patients; 0.8%), and prostate cancer (29 patients; 1%). Among the sub-group of patients with uterine cancer, PVs were identified in (12/76; 15.7%) MMR genes, as well as BRCA2, ATM, RAD51C, and NTHL1. Out of 24 patients with pancreatic cancer, two patients tested positive for a PV in the PALB2 gene accounting for an 8.3% PV detection rate. Among 29 patients presenting with prostate cancer, only three patients had a PV (two in CHEK2 and one in MLH1) (Table 2).
The cancer type was not defined for 219 patients (7.6%), and 42 (19%) of these patients had a PV (Table 2). A total of 61 patients were reported to have rare cancer types including brain cancer, (12 patients; 1 with PV in the MSH2 gene), thyroid cancer, (9 patients; 1 with PV in the BRCA2 gene), melanoma (25 patients; 4 patients with PV in PALB2, BRCA2 or the CHEK2 gene), sarcoma (12 patients; 2 with a PV in MLH1 and CHEK2 gene, respectively) and hematological cancer (three patients).
We have analyzed pathogenic variant detection rates in 6 actionable high penetrance genes recommended by NCCN (see text footnote 2: BRCA1, BRCA2, PALB2, TP53, PTEN, and CDH1) in patients with breast and/or ovarian cancer, categorized in sub-groups based on specific clinical presentation (Table 3). It was observed that PV detection rates were higher in certain clinically categorized sub groups These include: (1) breast and ovarian cancer (both diagnosed in one patient at any age), 11/49 (22.4%), (2) breast cancer <45 years of age, 38/482 (8%), (3) breast cancer <35 year of age, 20/186 (11%), (4) ovarian cancer at any age, 49/455 (11%), (5) triple negative (ER/PR/HER-2/neu negative) breast cancer, (TNBC), 21/218 (9.6%), (6) breast cancer with pancreatic or prostate at any age, 2/10 (20%). Since these sub-groups of patients have higher PV detection rates in high risk genes, they can be defined as high- risk group of patients. It can be inferred that these high-risk sub-groups are candidates for immediate referral for genetic testing at initial clinical contact, to avoid any delay in obtaining genetic test results for these high-risk sub groups as many medical and/or surgical management options for these patients can be driven by these genetic test results.
Whole gene or intragenic copy number variants were detected in 32 of 2870 (1.1%) patients, with confirmation by alternate techniques as shown in Supplementary Table 6. CNVs constituted 2.5% (33/1285) of total variants, and almost one in ten (9.5%; 27/287) of all PVs (Figure 2). 37% (10/27) of the pathogenic CNVs identified were in three (BRCA1, BRCA2, and PALB2) of the six high penetrance breast and/or ovarian cancer genes (BRCA1, BRCA2, PALB2, TP53, PTEN, and CDH1) identified by the NCCN (see text footnote 2). BRCA1 and BRCA2 deletions and duplications accounted for the majority, i.e., 33% (9/27) of the pathogenic CNVs detected. Normalized CNV plots showing CNVs identified in this study are represented in Figure 5. Frequency of different types of PVs (CNVs, nonsense, missense, deletion, insertions) is depicted in Figure 2. Detection of 25–150 bp “Mid-size” insertions and deletions can be challenging for NGS-based CNV algorithm. NGS alignment parameters require 80% match of a 150 bp reads for a successful alignment. Hence, the NGS alignment algorithm is normally limited to detection of in/dels <30 bp. However, the CNV-calling algorithm which is performed in parallel using the read depth data in a 10 bp sliding window is designed to enable detection of CNV >30 bp. As part of our standard clinical testing protocol all variants detected by NGS (sequence or CNV) are confirmed by and alternate methodology before a clinical report is issued. This protocol is designed to rule out have excluded the possibility of any technical artifacts that may result from NGS analysis and include standard clinically validated methodologies such as MLPA, LR-PCR, and Sanger sequencing (Supplementary Table 6).
Figure 5. Normalized copy number variant (CNV) plots demonstrating deletions and duplications in genes on the hereditary cancer panel. Y-axis represents quantile normalized copy number data (for unique autosomal genes, 0.5 indicates 1 copy; 1 indicates 2 copies; and 1.5, 3 copies; for homologous autosomal genes with their pseudogene, 0.75 indicates 3 copies; 1, 4 copies; and 1.25, 5 copies). Constitutional deletions are defined by a mean ratio of ≤0.65, and duplications are defined by a ratio of ≥1.35. Homologous region PMS2/PMS2CL deletions and duplications are assessed by a ratio of <0.8 and >1.2, respectively. X-axis indicates gene locations. From left to right, arrows represent patients with the following CNVs. (A) single patient with deletions in MSH2 and MSH6 genes [MSH2:c.(?_-21)_(*21_?)del/MSH6:c.(?_-21)_(*21_?)del] (represented by 2 arrows), pathogenic deletions of MLH1, PMS2, and BRCA2 [MLH1:c.(116 + 21_11721)_(545 + 21_546-21)del, PMS2:c.(537 + 21_538-21)_(903 + 21_904-21)del, BRCA2:c.(?_-21)_(67 + 21_68-21)del], novel VUS with four copies of a region of ATM [ATM:c.(8850 + 21_8851-21)_(*21_?) (Schon and Tischkowitz, 2018)], pathogenic deletions of PALB2 and BRCA1 [PALB2:c.(2586 + 21_2587-21)_(2748 + 21_2749-21)del, BRCA1:c.(5406 + 21_5407-21)_(*21_?)del, BRCA1:c.(5332 + 21_5333-21)_(5406 + 21_5407-21)del, BRCA1:c.(4357 + 21_4358-21)_(4484 + 21_4485-21)del], duplication of unknown significance in BRCA1 [BRCA1:c.(?_-21)_(80 + 21_81-21) (Li et al., 2019)], novel pathogenic deletion in RAD51C [RAD51C: c.(571 + 21_572-21)_(*21_?)del], pathogenic deletion and duplication of CHEK2 [CHEK2:c.(908 + 21_909-21)_(1095 + 21_1096-21)del, CHEK2:c.(319 + 21_320-21)_(592 + 21_593-21)dup], respectively. (B) Pathogenic deletions in MSH2, MSH6, BARD1, and APC genes [MSH2:c.(366 + 21_367-21)_(1076 + 21_1077-21)del, MSH6:c.(?_-21)_(260 + 21_261-21)del, BARD1:c.(?_-21)_(*21_?)del, APC:c.1958 + 241_4457del], duplications of unknown significance in PMS2 and ATM (novel variant) [PMS2:c.(2006 + 21_2007-21)_(*21_?)dup, ATM:c.(662 + 21_663-21)_(9171 + 21_9172-21)dup], pathogenic deletions in BRCA2, RAD51D (novel variant) and BRCA1 [BRCA2:c.(8487 + 21_8488-21)_(8632 + 21_8633-21)del, RAD51D:c.(?_-21)_(*21_?)del, BRCA1:c.(5277 + 21_5278-21)_(*21_?)del, BRCA1:c.(4986 + 21_4987-21)_(5074 + 21_5075-21)del], pathogenic duplication of BRCA1 [BRCA1:c.(4185 + 21_4186-21)_(4357 + 21_4358-21)dup], pathogenic deletion in BRCA1 [BRCA1:c.(?_-21)_(80 + 21_81-21)del], pathogenic duplication of RAD51C [RAD51C:c.(837 + 21_838-21)_(965 + 21_966-21)dup], pathogenic deletion of CHEK2 [CHEK2:c.(1461 + 21_1462-21)_(*21_?)del].
An increasingly complex pattern of germline mutations is being detected in patients presenting with various types of cancers following the almost universal adoption of NGS-based genetic test protocols in diagnostic laboratories. Many new cancer susceptibility genes have been identified, and the frequency of mutations and associated clinical cancer phenotypes continue to be described in a wide variety of different ethnic groups across the world. Methods for detection of PVs in cancer predisposition genes have evolved significantly from the gold standard sanger sequencing and MLPA to most advanced NGS based massive parallel sequencing methods (Susswein et al., 2016; Okur and Chung, 2017; Rosenthal et al., 2017; Neben et al., 2019; Oliver et al., 2019; Tsaousis et al., 2019; LaDuca et al., 2020; Yadav et al., 2020). We have implemented a NGS-based approach that we have developed and optimized for detection of sequence variants and CNVs, in our laboratory, to test families suspected with familial cancer syndromes (Schenkel et al., 2016; Kerkhof et al., 2017; Volodarsky et al., 2020). The work flow and analysis algorithm is unique and highly accurate. Here we describe the outcome of a large cohort of patients referred to be tested using this approach at the Molecular Diagnostic Laboratory, London Health Sciences Centre (LHSC), an academic health care center serving a catchment area of approximately 2 million people in Southwestern Ontario, Canada. The distribution and prevalence of clinically relevant variants has been analyzed among patients tested with different panels of genes as described above, based on their personal and family history of cancer.
We report an overall detection rate of 15% for pathogenic/likely pathogenic mutations. Previous studies have reported comparable diagnostic yield, though a direct comparison has not been possible due to variability in patient inclusion criteria, number of genes on the variant detection and classification criteria (Susswein et al., 2016; Rosenthal et al., 2017; Neben et al., 2019; Oliver et al., 2019; Tsaousis et al., 2019; LaDuca et al., 2020; Yadav et al., 2020). Our panel content was designed to meet the requirements set forth by the Ministry of Health and Long term Care of Ontario and is based on the clinical guidelines and recommendations including Cancer Care Ontario’s OBSP (see text footnote 1) and NIH ClinGen expert recommendations (see text footnote 3) and NCBI Gene Reviews (see text footnote 4) along with genes reported in literature (Lee et al., 2019; Seifert et al., 2019). The panel content and gene number has expanded over the years based on these requirements. As a comparison in a recent study with an extended targeted panel, including 64 hereditary cancer predisposition genes, PV detection rate of 19.2% was reported in 496 patients with hereditary breast and ovarian cancer and 12% patients were positive for a PV in BRCA1 and BRCA2 genes (Shin et al., 2020). Another study with 143 gene targeted panel showed a PV detection rate of 17% (Oliver et al., 2019). Extended panels with more genes can yields increased PV detection rates but patient selection criteria and PV detection rates for individual high/low/unknown penetrance differ between the labs and are often variably reported in the overall detection rates. Also, the supporting evidence for clinical impact of variants in newly identified genes included in larger panels is often limited (Seifert et al., 2019). However, a recent hospital–based study has reported a 5.7% mutation detection rate in 6 actionable high penetrance breast cancer genes in a subset of patients presenting with breast cancer who were selected by NCCN clinical criteria for testing (Yadav et al., 2020). In our center, for similar patients selected by the equivalent OBSP clinical criteria, the mutation detection rate of the same subpanel of genes was 6.5% (Table 3). A recent prospective multi-center study has shown very high germline pathogenic variant detection rates (13.3%) in 2984 cancer patients not selected based on cancer type, disease stage, family history of cancer ethnicity, or age, tested by 80 gene NGS panel with nearly 30% of patients had their treatments impacted due to this information (Samadder et al., 2020). These results are definitely suggestive of the fact that guidelines used for selecting patients for genetic testing should be re-evaluated as the number of genes on NGS based targeted panels are expanding or approaches like universal multigene panel testing may be implemented in near future.
Intragenic copy number changes have been identified in hereditary cancer and account for a small, but clinically significant, proportion of cases. While the majority of BRCA1 and BRCA2 variants are loss of function sequence changes, intragenic large genomic rearrangements have been reported to account for 3–15% (Vasickova et al., 2007; Hansen et al., 2009) of all BRCA1 and BRCA2 mutations. Until more recently, copy number variant detection in breast and ovarian cancer has been limited to studies of BRCA1 and BRCA2 genes using approaches such as MLPA, long-range and/or quantitative PCR in the primary screen, and the contribution of CNVs in other hereditary cancer predisposition genes was relatively unknown. Our laboratory has been among the first few in the world to report a clinically validated copy number variant detection algorithm that could be applied to NGS panel testing, and which was initially applied to our hereditary breast cancer panel (Schenkel et al., 2016; Kerkhof et al., 2017). These computational methods have been demonstrated to accurately detect intragenic copy number alterations of >50 bp, such as exon deletions and/or duplications, and have enabled us to detect PVs across an entire panel of genes while decreasing costs through avoidance of parallel testing, an approach, which has led to decreased test turnaround times. Our analysis pipeline is designed to circumvent challenges of detecting intermediate in/del variants using a combination of sequence alignment and high-resolution CNV calling. Sequence alignment is based on the random DNA fragmentation resulting in a staggered sequence alignment with a deep sequence coverage (>200×). This enables the CNV calling algorithm to use a sliding scale window which is designed to asses for loss or gain of sequence coverage at a 10 bp resolution. This analysis is dependent on high quality of DNA and inter/intra run sequence uniformity, and absolute sensitivity for the complex variants cannot be guaranteed in specimens not meeting the specific quality control parameters. In our experience, in peripheral blood DNA, this applies to less than 2% of specimens where the data quality is suboptimal. Similar limitation for detection of such variants is common even with targeted orthogonal methods such as Sanger sequencing and MLPA analysis. CNV detection using NGS data increases the diagnostic yield of this assay, while decreasing the need for the orthogonal testing modalities, hence increasing the TAT and overall costs to the system. However, it also must be recognized that it is possible that some more complex rearrangements, low-level mosaicism, balanced translocations and other complex genomic features may have a limited detection using this methodology and result in false negatives. This however is not unlike other genomics testing methods including the classic techniques such as MLPA5 and copy number microarrays as well as other NGS-based CNV detection algorithms (Park and Mori, 2010; Zhao et al., 2013). This study has identified pathogenic (ACMG 1 and ACMG 2) CNVs in 32 of 2870 (1.1%) patients, or 32 of 431 (7.4%) of all PVs identified, a proportion roughly in line with few previous reports (Mancini-DiNardo et al., 2019; Tsaousis et al., 2019; Zeng et al., 2020). A significant subset of these CNVs have been identified in some of the less well characterized cancer predisposition genes and adds to the spectrum of variants reported in these genes (Table 2). These findings emphasize the benefit of routinely incorporating CNV analysis into NGS panel testing, an approach that will significantly improve the efficiency of screening for hereditary cancers. Moving forward, this type of CNV analysis pipeline will become indispensable as further studies suggest expanding the gene testing criteria (Yadav et al., 2020; Zeng et al., 2020) and will serve to highlight the cost-effectiveness of panel-based testing for all cancer patients and/or population screening for cancer prevention (Manchanda et al., 2018; Sun et al., 2019).
We have also observed variants in few genes that have a less well-established association with certain cancer types previously reported in the literature. PVs in the ATM gene have been reported to have an increased predisposition to develop a wide range of cancers including breast, ovarian, pancreatic, colorectal, uterine, gastric, and/or prostate cancers as well as colorectal polyps consistent with our own findings (Supplementary Table 7) (Thompson et al., 2005; Sriramulu et al., 2019). A PV in the APC gene, (c.3920T > A, p.(Ile1307Lys) was detected in 5 unrelated patients with breast cancer. This sequence variant has been reported as a low penetrance allele common in the Ashkenazi Jewish population, and its association with breast cancer risk has been controversial (Redston et al., 1998; Woodage et al., 1998). However, this association is supported by our findings which also suggest increased breast cancer risk in patients carrying this variant. We have also identified a PV in BRIP1 gene in a patient presenting with colorectal cancer (Supplementary Table 7). BRIP1 gene is reported to moderately increase the risk of breast and/or ovarian cancer, (OMIM: #605882) though its association with colorectal cancer is sparsely reported in the literature and exact risks are not known (Ali et al., 2019). As the list of genes, associated with an increased predisposition to cancer increases, more extensive studies will be needed to obtain a better understanding of the cancer risk patterns for these genes.
This study correlates clinical presentations and associated PVs in a large anonymized data set of patients with personal and/or family history of cancer, referred for genetic testing over a 2-year period to a provincial genetic testing laboratory in Southwestern Ontario, Canada. It is recognized that that there are some limitations to this study in that in this anonymized data set we were not able to confirm clinical information provided on the test requisition, and in a minority of cases this information was incomplete. However, this study has provided an overview of the pattern of DNA sequence variants and genomic rearrangements identified in variety of cancers using a unique NGS based algorithm in a clinical setting. In addition, our findings do serve to highlight some key aspects related to the genetic testing offered in the province that may help to provide a more effective approach to identify and immediately refer for testing higher risk clinical subgroups who would benefit from more timely accession to their genetic test results, which will serve to better manage their ongoing clinical care.
The original contributions presented in the study are included in the article/Supplementary Material, further inquiries can be directed to the corresponding author/s.
Ethical review and approval was not required for the study on human participants in accordance with the local legislation and institutional requirements. Written informed consent to participate in this study was provided by the participants’ legal guardian/next of kin.
PB did data curation, wrote original draft, performed data analysis, and conceptualization. ML did data curation, analysis, manuscript reviewing, and editing. KR contributed in writing original draft and data analysis. DC contributed in conceptualization and reviewing manuscript. JR did data curation and analysis. JK contributed in data curation, method validation, and analysis. MV contributed to the manuscript review and editing. AS did data curation and validation of methods. MK contributed to the conceptualization, arrange resources, and manuscript review and editing. KP contributed to the manuscript review and writing. LS and HL did validation of methods, manuscript review, and writing. PA contributed to the supervision, visualization, and writing original manuscript draft. BS contributed to the investigation, supervision, visualization, conceptualization, resources, funding acquisition, writing–reviewing and editing. All authors contributed to the article and approved the submitted version.
This study was supported by London Health Sciences Centre Molecular Diagnostics Innovation and Development Fund.
The authors declare that the research was conducted in the absence of any commercial or financial relationships that could be construed as a potential conflict of interest.
The Supplementary Material for this article can be found online at: https://www.frontiersin.org/articles/10.3389/fgene.2021.698595/full#supplementary-material
Supplementary Table 1 | Guidelines for referral for hereditary cancer predisposition genetic testing.
Supplementary Table 2 | List of genes included in different subpanels and respective versions.
Supplementary Table 3 | Number of patients tested on each sub-panel (Panel name/no of genes; genes listed in Supplementary Table 2).
Supplementary Table 4 | List of all patients tested and genetic variants (ACMG 1, 2, and 3) identified in genes included on the panel.
Supplementary Table 5 | Novel Pathogenic Variants (PVs) identified in the study.
Supplementary Table 6 | Copy Number Variants identified in this study using our NGS algorithm and validation using alternate techniques.
Supplementary Table 7 | Number of patients with each cancer type positive for a pathogenic variant in genes across all sub-panels.
Ali, M., Delozier, C. D., and Chaudhary, U. (2019). BRIP-1 germline mutation and its role in colon cancer: presentation of two case reports and review of literature. BMC Med. Genet. 20:75. doi: 10.1186/s12881-019-0812-0
Balmaña, J., Díez, O., Rubio, I. T., and Cardoso, F. (2011). ESMO guidelines working group. BRCA in breast cancer: ESMO clinical practice guidelines. Ann. Oncol. 22, vi31–vi34.
den Dunnen, J. T., Dalgleish, R., Maglott, D. R., Hart, R. K., Greenblatt, M. S., McGowan-Jordan, J., et al. (2016). HGVS recommendations for the description of sequence variants: 2016 Update. Hum. Mutat. 37, 564–569. doi: 10.1002/humu.22981
Hansen, T. O., Jønson, L., Albrechtsen, A., Andersen, M. K., Ejlertsen, B., Nielsen, F. C., et al. (2009). Large BRCA1 and BRCA2 genomic rearrangements in Danish high risk breast-ovarian cancer families. Breast Cancer Res. Treat. 115, 315–323. doi: 10.1007/s10549-008-0088-0
Kerkhof, J., Schenkel, L. C., Reilly, J., McRobbie, S., Aref-Eshghi, E., Stuart, A., et al. (2017). Clinical validation of copy number variant detection from targeted next-generation sequencing panels. J. Mol. Diagn. 19, 905–920. doi: 10.1016/j.jmoldx.2017.07.004
LaDuca, H., Polley, E. C., Yussuf, A., Hoang, L., Gutierrez, S., Hart, S. N., et al. (2020). A clinical guide to hereditary cancer panel testing: evaluation of gene-specific cancer associations and sensitivity of genetic testing criteria in a cohort of 165,000 high-risk patients. Genet. Med. 22, 407–415. doi: 10.1038/s41436-019-0633-8
Lang, G. T., Shi, J. X., Hu, X., Zhang, C. H., Shan, L., Song, C. G., et al. (2017). The spectrum of BRCA mutations and characteristics of BRCA-associated breast cancers in China: screening of 2,991 patients and 1,043 controls by next-generation sequencing. Int. J. Cancer. 141, 129–142. doi: 10.1002/ijc.30692
Lee, K., Seifert, B. A., Shimelis, H., Ghosh, R., Crowley, S. B., Carter, N. J., et al. (2019). Clinical validity assessment of genes frequently tested on hereditary breast and ovarian cancer susceptibility sequencing panels. Genet. Med. 21, 1497–1506. doi: 10.1038/s41436-018-0361-5
Li, J., Wen, W. X., Eklund, M., Kvist, A., Eriksson, M., Christensen, H. N., et al. (2019). Prevalence of BRCA1 and BRCA2 pathogenic variants in a large, unselected breast cancer cohort. Int. J. Cancer. 144, 1195–1204. doi: 10.1002/ijc.31841
Manchanda, R., Patel, S., Gordeev, V. S., Antoniou, A. C., Smith, S., Lee, A., et al. (2018). Cost-effectiveness of population-based BRCA1, BRCA2, RAD51C, RAD51D, BRIP1, PALB2 mutation testing in unselected general population women. J. Natl. Cancer Inst. 110, 714–725. doi: 10.1093/jnci/djx265
Mancini-DiNardo, D., Judkins, T., Kidd, J., Bernhisel, R., Daniels, C., Brown, K., et al. (2019). Detection of large rearrangements in a hereditary pan-cancer panel using next-generation sequencing. BMC Med. Genom. 12:138. doi: 10.1186/s12920-019-0587-3
Neben, C. L., Zimmer, A. D., Stedden, W., van den Akker, J., O’Connor, R., Chan, R. C., et al. (2019). Multi-gene panel testing of 23,179 individuals for hereditary cancer risk identifies pathogenic variant carriers missed by current genetic testing guidelines. J. Mol. Diagn. 21, 646–657. doi: 10.1016/j.jmoldx.2019.03.001
Okur, V., and Chung, W. K. (2017). The impact of hereditary cancer gene panels on clinical care and lessons learned. Cold Spring Harb. Mol. Case Stud. 3:a002154. doi: 10.1101/mcs.a002154
Oliver, J., Quezada Urban, R., Franco Cortés, C. A., Díaz Velásquez, C. E., Montealegre Paez, A. L., Pacheco-Orozco, R. A., et al. (2019). Latin american study of hereditary breast and ovarian cancer LACAM: A genomic epidemiology approach. Front. Oncol. 9:1429. doi: 10.3389/fonc.2019.01429
Park, B. S., and Mori, M. (2010). Balancing false discovery and false negative rates in selection of differentially expressed genes in microarrays. Open Access Bioinform. 2010, 1–9. doi: 10.2147/oab.s7181
Rahman, N., Seal, S., Thompson, D., Kelly, P., Renwick, A., Elliott, A., et al. (2007). PALB2, which encodes a BRCA2-interacting protein, is a breast cancer susceptibility gene. Nat. Genet. 39, 165–167. doi: 10.1038/ng1959
Redston, M., Nathanson, K. L., Yuan, Z. Q., Neuhausen, S. L., Satagopan, J., Wong, N., et al. (1998). The APCI1307K allele and breast cancer risk. Nat. Genet. 20, 13–14.
Richards, S., Aziz, N., Bale, S., Bick, D., Das, S., Gastier-Foster, J., et al. (2015). Standards and guidelines for the interpretation of sequence variants: a joint consensus recommendation of the American College of medical genetics and genomics and the association for molecular pathology. Genet. Med. 17, 405–424. doi: 10.1038/gim.2015.30
Roberts, M. E., Jackson, S. A., Susswein, L. R., Zeinomar, N., Ma, X., Marshall, M. L., et al. (2018). MSH6 and PMS2 germ-line pathogenic variants implicated in Lynch syndrome are associated with breast cancer. Genet. Med. 20, 1167–1174. doi: 10.1038/gim.2017.254
Rosenthal, E. T., Bernhisel, R., Brown, K., Kidd, J., and Manley, S. (2017). Clinical testing with a panel of 25 genes associated with increased cancer risk results in a significant increase in clinically significant findings across a broad range of cancer histories. Cancer Genet. 218, 58–68. doi: 10.1016/j.cancergen.2017.09.003
Samadder, N. J., Riegert-Johnson, D., Boardman, L., Rhodes, D., Wick, M., Okuno, S., et al. (2020). Comparison of universal genetic testing vs guideline-directed targeted testing for patients with hereditary cancer syndrome. JAMA Oncol. 7, 230–237. doi: 10.1001/jamaoncol.2020.6252
Schenkel, L. C., Kerkhof, J., Stuart, A., Reilly, J., Eng, B., Woodside, C., et al. (2016). Clinical next-generation sequencing pipeline outperforms a combined approach using sanger sequencing and multiplex ligation-dependent probe amplification in targeted gene panel analysis. J. Mol. Diagn. 18, 657–667. doi: 10.1016/j.jmoldx.2016.04.002
Schon, K., and Tischkowitz, M. (2018). Clinical implications of germline mutations in breast cancer: TP53. Breast Cancer Res. Treat. 167, 417–423. doi: 10.1007/s10549-017-4531-y
Seifert, B. A., McGlaughon, J. L., Jackson, S. A., Ritter, D. I., Roberts, M. E., Schmidt, R. J., et al. (2019). Determining the clinical validity of hereditary colorectal cancer and polyposis susceptibility genes using the clinical genome resource clinical validity framework. Genet. Med. 21, 1507–1516. doi: 10.1038/s41436-018-0373-1
Shin, H. C., Lee, H. B., Yoo, T. K., Lee, E. S., Kim, R. N., Park, B., et al. (2020). Detection of germline mutations in breast cancer patients with clinical features of hereditary cancer syndrome using a multi-gene panel test. Cancer Res. Treat. 52, 697–713. doi: 10.4143/crt.2019.559
Sriramulu, S., Ramachandran, M., Subramanian, S., Kannan, R., Gopinath, M., Sollano, J., et al. (2019). A review on role of ATM gene in hereditary transfer of colorectal cancer. Acta Biomed. 89, 463–469.
Sun, L., Brentnall, A., Patel, S., Buist, D. S. M., Bowles, E. J. A., Evans, D. G. R., et al. (2019). A cost-effectiveness analysis of multigene testing for all patients with breast cancer. JAMA Oncol. 5, 1718–1730. doi: 10.1001/jamaoncol.2019.3323
Susswein, L. R., Marshall, M. L., Nusbaum, R., Vogel Postula, K. J., Weissman, S. M., Yackowski, L., et al. (2016). Pathogenic and likely pathogenic variant prevalence among the first 10,000 patients referred for next-generation cancer panel testing. Genet. Med. 18, 823–832. doi: 10.1038/gim.2015.166
Thompson, D., Duedal, S., Kirner, J., McGuffog, L., Last, J., Reiman, A., et al. (2005). Cancer risks and mortality in heterozygous ATM mutation carriers. J. Natl. Cancer Inst. 97, 813–822. doi: 10.1093/jnci/dji141
Tsaousis, G. N., Papadopoulou, E., Apessos, A., Agiannitopoulos, K., Pepe, G., Kampouri, S., et al. (2019). Analysis of hereditary cancer syndromes by using a panel of genes: novel and multiple pathogenic mutations. BMC Cancer 19:535. doi: 10.1186/s12885-019-5756-4
Vasickova, P., Machackova, E., Lukesova, M., Damborsky, J., Horky, O., Pavlu, H., et al. (2007). High occurrence of BRCA1 intragenic rearrangements in hereditary breast and ovarian cancer syndrome in the Czech Republic. BMC Med. Genet. 8:32. doi: 10.1186/1471-2350-8-32
Volodarsky, M., Kerkhof, J., Stuart, A., Levy, M., Brady, L. I., Tarnopolsky, M., et al. (2020). Comprehensive genetic sequence and copy number analysis for Charcot-Marie-Tooth disease in a Canadian cohort of 2517 patients. J. Med. Genet. 8, 284–288. doi: 10.1136/jmedgenet-2019-106641
Woodage, T., King, S. M., Wacholder, S., Hartge, P., Struewing, J. P., McAdams, M., et al. (1998). The APCI1307K allele and cancer risk in a community-based study of Ashkenazi Jews. Nat. Genet. 20, 62–65. doi: 10.1038/1722
Yadav, S., Hu, C., Hart, S. N., Boddicker, N., Polley, E. C., Na, J., et al. (2020). Evaluation of germline genetic testing criteria in a hospital based series of women with breast cancer. J. Clin. Oncol. 38, 1409–1418.
Zeng, C., Guo, X., Wen, W., Shi, J., Long, J., Cai, Q., et al. (2020). Evaluation of pathogenetic mutations in breast cancer predisposition genes in population-based studies conducted among Chinese women. Breast Cancer Res. Treat. 181, 465–473. doi: 10.1007/s10549-020-05643-0
Keywords: next generation sequencing, copy number variants, familial cancer syndromes, breast cancer, colorectal cancer
Citation: Bhai P, Levy MA, Rooney K, Carere DA, Reilly J, Kerkhof J, Volodarsky M, Stuart A, Kadour M, Panabaker K, Schenkel LC, Lin H, Ainsworth P and Sadikovic B (2021) Analysis of Sequence and Copy Number Variants in Canadian Patient Cohort With Familial Cancer Syndromes Using a Unique Next Generation Sequencing Based Approach. Front. Genet. 12:698595. doi: 10.3389/fgene.2021.698595
Received: 21 April 2021; Accepted: 07 June 2021;
Published: 13 July 2021.
Edited by:
Mehdi Pirooznia, National Heart, Lung, and Blood Institute (NHLBI), United StatesReviewed by:
Elizabeth Chao, University of California, Irvine, United StatesCopyright © 2021 Bhai, Levy, Rooney, Carere, Reilly, Kerkhof, Volodarsky, Stuart, Kadour, Panabaker, Schenkel, Lin, Ainsworth and Sadikovic. This is an open-access article distributed under the terms of the Creative Commons Attribution License (CC BY). The use, distribution or reproduction in other forums is permitted, provided the original author(s) and the copyright owner(s) are credited and that the original publication in this journal is cited, in accordance with accepted academic practice. No use, distribution or reproduction is permitted which does not comply with these terms.
*Correspondence: Peter Ainsworth, cGV0ZXIuYWluc3dvcnRoQGxoc2Mub24uY2E=; Bekim Sadikovic, YmVraW0uc2FkaWtvdmljQGxoc2Mub24uY2E=
Disclaimer: All claims expressed in this article are solely those of the authors and do not necessarily represent those of their affiliated organizations, or those of the publisher, the editors and the reviewers. Any product that may be evaluated in this article or claim that may be made by its manufacturer is not guaranteed or endorsed by the publisher.
Research integrity at Frontiers
Learn more about the work of our research integrity team to safeguard the quality of each article we publish.