- 1Division of Human Genetics, Department of Psychiatry, Yale School of Medicine, New Haven, CT, United States
- 2Veteran Affairs Connecticut Healthcare System, West Haven, CT, United States
Angiotensin-converting enzyme-2 (ACE2) receptor has been identified as the key adhesion molecule for the transmission of the SARS-CoV-2. However, there is no evidence that human genetic variation in ACE2 is singularly responsible for COVID-19 susceptibility. Therefore, we performed an integrative multi-level characterization of genes that interact with ACE2 (ACE2-gene network) for their statistically enriched biological properties in the context of COVID-19. The phenome-wide association of 51 genes including ACE2 with 4,756 traits categorized into 26 phenotype categories, showed enrichment of immunological, respiratory, environmental, skeletal, dermatological, and metabolic domains (p < 4e-4). Transcriptomic regulation of ACE2-gene network was enriched for tissue-specificity in kidney, small intestine, and colon (p < 4.7e-4). Leveraging the drug-gene interaction database we identified 47 drugs, including dexamethasone and spironolactone, among others. Considering genetic variants within ± 10 kb of ACE2-network genes we identified miRNAs whose binding sites may be altered as a consequence of genetic variation. The identified miRNAs revealed statistical over-representation of inflammation, aging, diabetes, and heart conditions. The genetic variant associations in RORA, SLC12A6, and SLC6A19 genes were observed in genome-wide association study (GWAS) of COVID-19 susceptibility. We also report the GWAS-identified variant in 3p21.31 locus, serves as trans-QTL for RORA and RORC genes. Overall, functional characterization of ACE2-gene network highlights several potential mechanisms in COVID-19 susceptibility. The data can also be accessed at https://gpwhiz.github.io/ACE2Netlas/.
Introduction
SARS-CoV-2 (severe acute respiratory syndrome coronavirus 2) is the causative agent responsible for recent global spread of COVID-19 (coronavirus disease 2019) (Wu et al., 2020; Zhou et al., 2020). Millions of people have been infected with the virus, which caused global lockdowns and heavily restricted interpersonal contact. These measures were taken to reduce viral spread through respiratory droplet exchange between persons.
SARS-CoV-2 is capable of entering the host cells via ACE2 (angiotensin converting enzyme 2) (Walls et al., 2020; Mercurio et al., 2021). ACE2 is found on many different cell types, which normally helps regulate blood pressure and inflammation through cleavage of angiotensin II (ANG II) (Hamming et al., 2007). The virus occupies cell-surface of ACE2 leading to accumulation of angiotensin (ANGII), inflammation, and cell death (Walls et al., 2020). The interactions between the spike protein and ACE2 trigger pre-/post-fusion conformational changes at the spike protein, following spike cleavage in two main domains. The spike N-terminal domain forms a stable protein-protein complex with ACE2, whereas the C-terminal domain, namely the spike post-fusion protein, favors virus/host-cells membrane fusion (Mercurio et al., 2021). In the lungs, SARS-CoV-2 mediated ANGII accumulation leads to alveolar cell death and a reduction in oxygen uptake (Wang et al., 2020).
Although ACE2 is the cellular entry point, there is little evidence that genetic variation in ACE2 is singularly responsible for COVID-19 susceptibility (Ellinghaus et al., 2020; Pairo-Castineira et al., 2020; Shelton et al., 2020). Due to the functional role of ACE2 in SARS-CoV-2 infection, we hypothesize that genes interacting with ACE2 activity are enriched for molecular pathways relevant for COVID-19 susceptibility. Accordingly, we employed a top-down approach to analyze tissue-specific transcriptomic regulation, drug-gene interactions, and variant prioritization using genetic variants within the ACE2 gene-gene connectome and protein-protein interaction networks. With this approach we identified several biological processes and functional effects of ACE2-gene network relevant for the vast symptoms observed following SARS-CoV-2 infection.
Results
A study overview is presented in Supplementary Figure 1.
The ACE2 Gene Connectome
A total of 60 genes were identified from six network databases that interact with ACE2 (Supplementary Table 1).
Tissue-Specific Transcriptomic Regulation
The differential expression data of 54 tissues (GTEx-v8) was used to identify the tissue specificity of the ACE2 network genes. The ACE2 network genes were enriched for upregulated expression in small intestine (p = 1.07 × 10–16), colon (p = 7.60 × 10–13), kidney (p = 1.93 × 10–8), and liver (p = 4.63 × 10–4) (Figure 1 and Supplementary Table 2). No tissue-specific enrichment was observed for down-regulated expression.
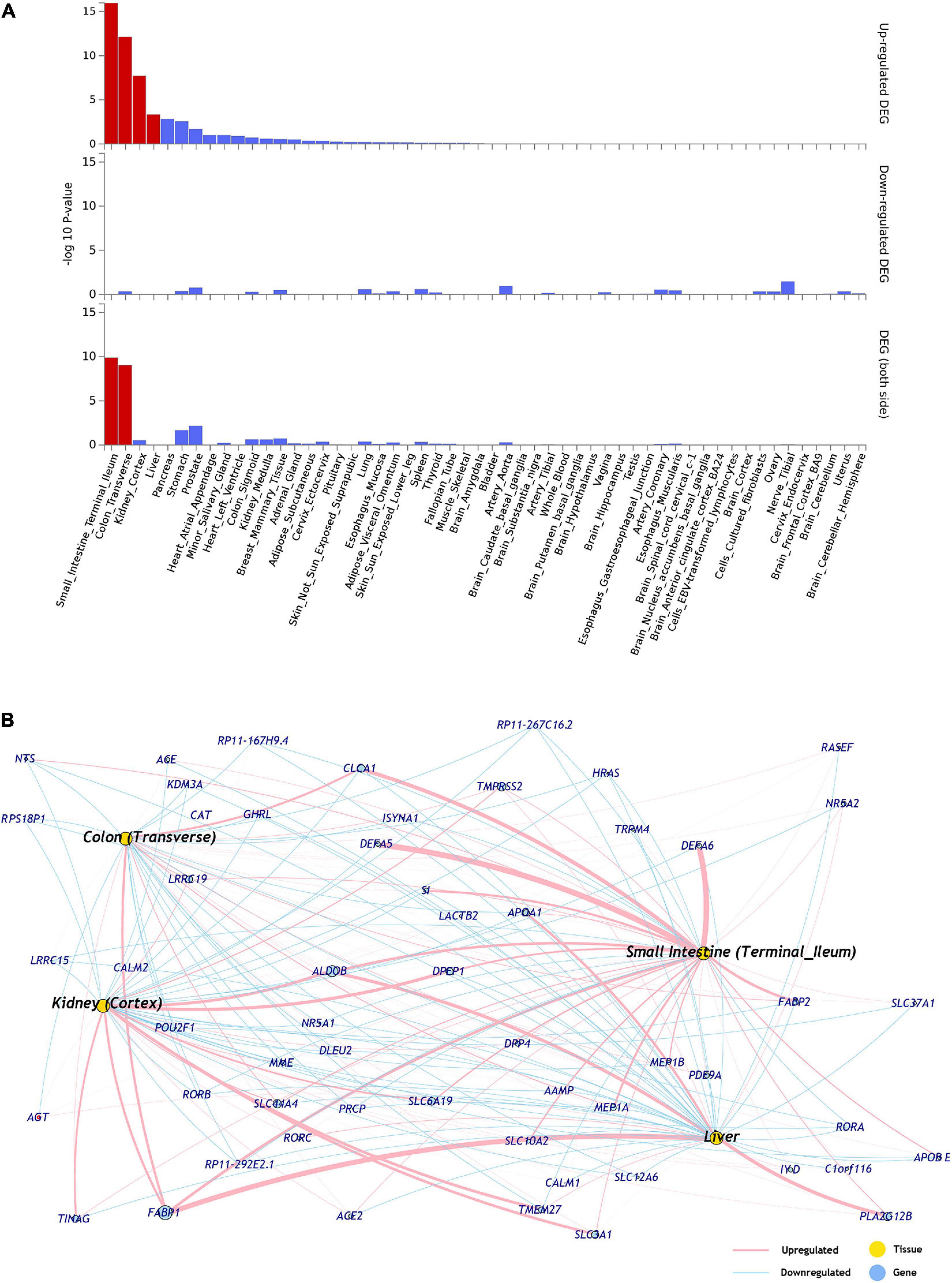
Figure 1. (A) Tissues enriched based on ACE2-network gene expression from GTEx database. The genes from the ACE2-network show over-representation of tissues (x-axis) and –log10 p-value (y-axis). The red bars are significant enrichments. (DEG—Differentially expressed genes). (B) The network of genes with tissue specific expression of overrepresented tissues, pink edges represent upregulation and blue edges represent downregulation. The weight of the edges corresponds to expression values (average transcript per million; TPM).
Gene Expression of ACE2-Interacting genes in Upper Respiratory Tissue for SARS-CoV-2 and Other Viruses
Using transcriptomic data related to acute respiratory illnesses for COVID-19 patients (N = 93), other viral (N = 41), or non-viral (N = 100) in the upper respiratory tract tissue (Mick et al., 2020), we found 35 of the 61 ACE2-interacting genes. Of the 35 genes, we found 19 genes that were reported as significant (Benjamini–Hochberg adjusted p-value < 0.05) for any of three comparisons, SARS-CoV-2 vs. no-virus, SARS-CoV-2 vs. other-virus, other-virus vs. no-virus (Supplementary Table 3). For the SARS-CoV-2 vs. no-virus, four of the ACE2-interacting genes were significant (ACE2 logFC = 1.25; CALM2 logFC = 0.36; PRCP logFC = –0.33; RORB logFC = 0.83). For differential gene expression between SARS-CoV-2 vs. other-virus and other-virus vs. no-virus, there were 16 and 11 genes, respectively, that were significant.
Gene-Drug Interaction and Over-Represented Biological Functions
To identify known drugs that interact with the ACE2-gene set, we investigated the drug-gene interaction database (DGIdb) (Griffith et al., 2013). Out of 61 genes, 29 had information about their drug-gene interaction in DGIdb, resulting in 238 unique drug-gene observations (Supplementary Table 4). Some of the notable drugs observed via this approach were spironolactone, dexamethasone, metformin, and hydrocortisone. To understand the role of these drugs in affecting biological processes, we performed drug-set enrichment analysis. DSEA (Napolitano et al., 2016) found gene-ontology mapping for 47 drugs and tested against REACTOME gene ontology database. Although the results did not survive Bonferroni correction, nominally significant enrichments were observed for platelet sensitization by low-density lipoprotein cholesterol (p = 0.003), IL-7 signaling (p = 0.004), glycerophospholipid biosynthesis (p = 0.005), and viral messenger RNA synthesis (p = 0.011) (Figure 2 and Supplementary Table 5).
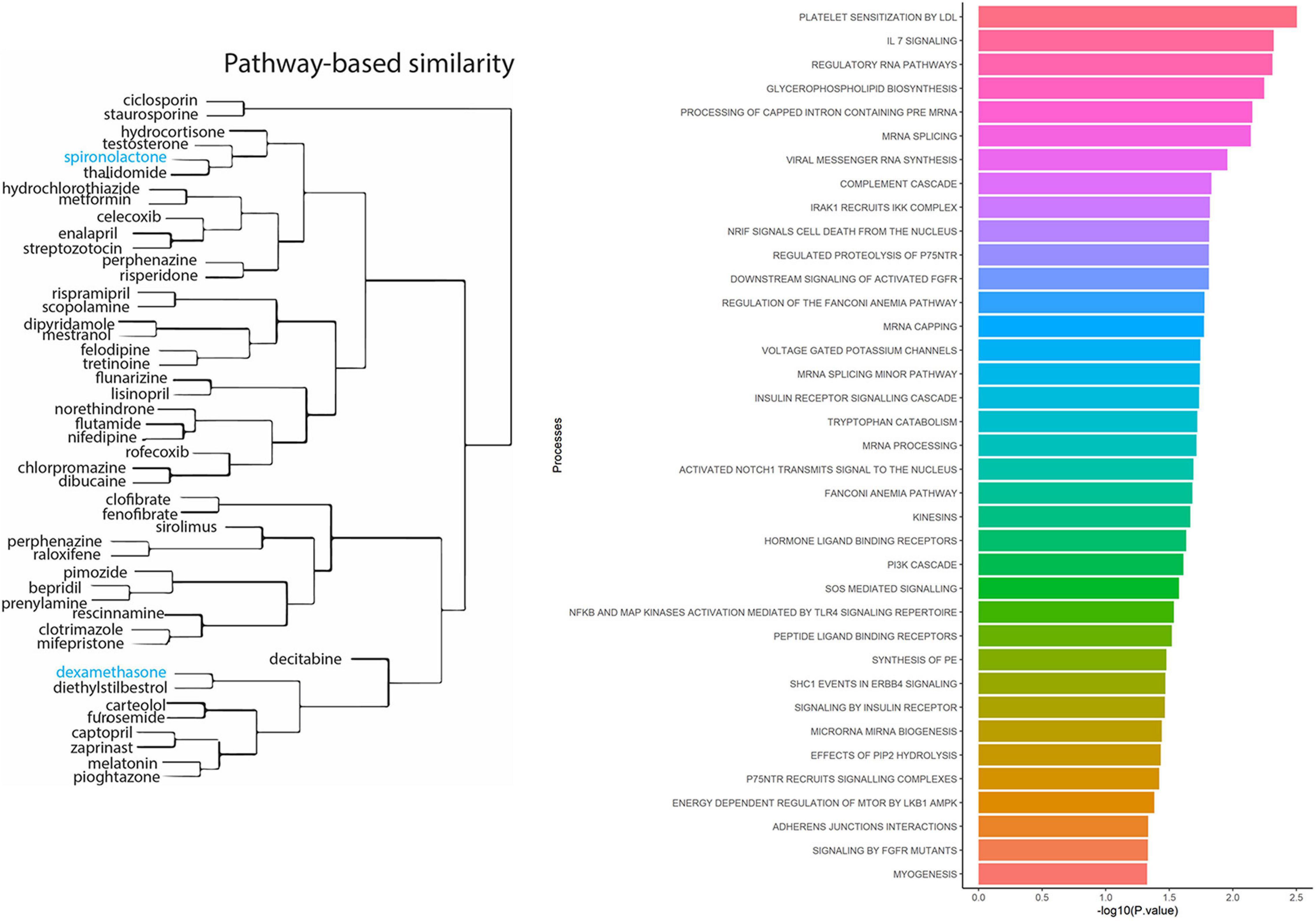
Figure 2. Drug-set enrichment analysis. LEFT: The similarity of drugs based on pathways identified. RIGHT: Biological Processes identified based on drugs that interact with genes from the ACE2-network.
Over-Representation of Phenotypic Domains Within ACE2 Gene Network
To identify the phenotypes/traits associated with the ACE2-genes, we performed a phenome-wide association study (PheWAS). The GWASAtlas (Watanabe et al., 2019) contains 4756 traits categorized into 26 phenotype domains. We found 3983 phenotype associations for 51/61 genes, of which 43 genes with 476/3983 phenotypes were significant (p < 1e-5) (Supplementary Figures 2–52 and Supplementary Table 6). Most significant phenotypes were observed for SLC44A4 with rheumatoid arthritis (p = 1.51 × 10–105) and white blood cell (p = 6.16 × 10–105). Significant phenotypes observed across several genes included body mass index, anthropometric traits, kidney function phenotypes—glomerular filtration rate, renin-angiotensin system, and lung capacity indices, i.e., FEV and FVC. The phenotype domains were tested for enrichment of significant traits vs. non-significant traits (Supplementary Table 7). Six domains were significant: “Immunological” (p = 7.62 × 10–25), “Respiratory” (p = 1.30 × 10–8), “Skeletal” (2.94 × 10–8), “Dermatological” (p = 7.91 × 10–8), “Environmental” (p = 2.21 × 10–7), and “Metabolic” (4.33 × 10–4) (Supplementary Table 8). SLC44A4 had the highest number of associated traits across the significant domains (ntotal = 173) followed by APOA1 had highest number of traits associations, mostly metabolic traits such as triglycerides, cholesterol, lipid measurements and blood cell measurements, i.e., platelet count and mean corpuscle volume (ntotal = 100; metabolic = 71 traits) (Figure 3). SLC44A4, APOA1, and RORA showed associations across all six enriched domains.
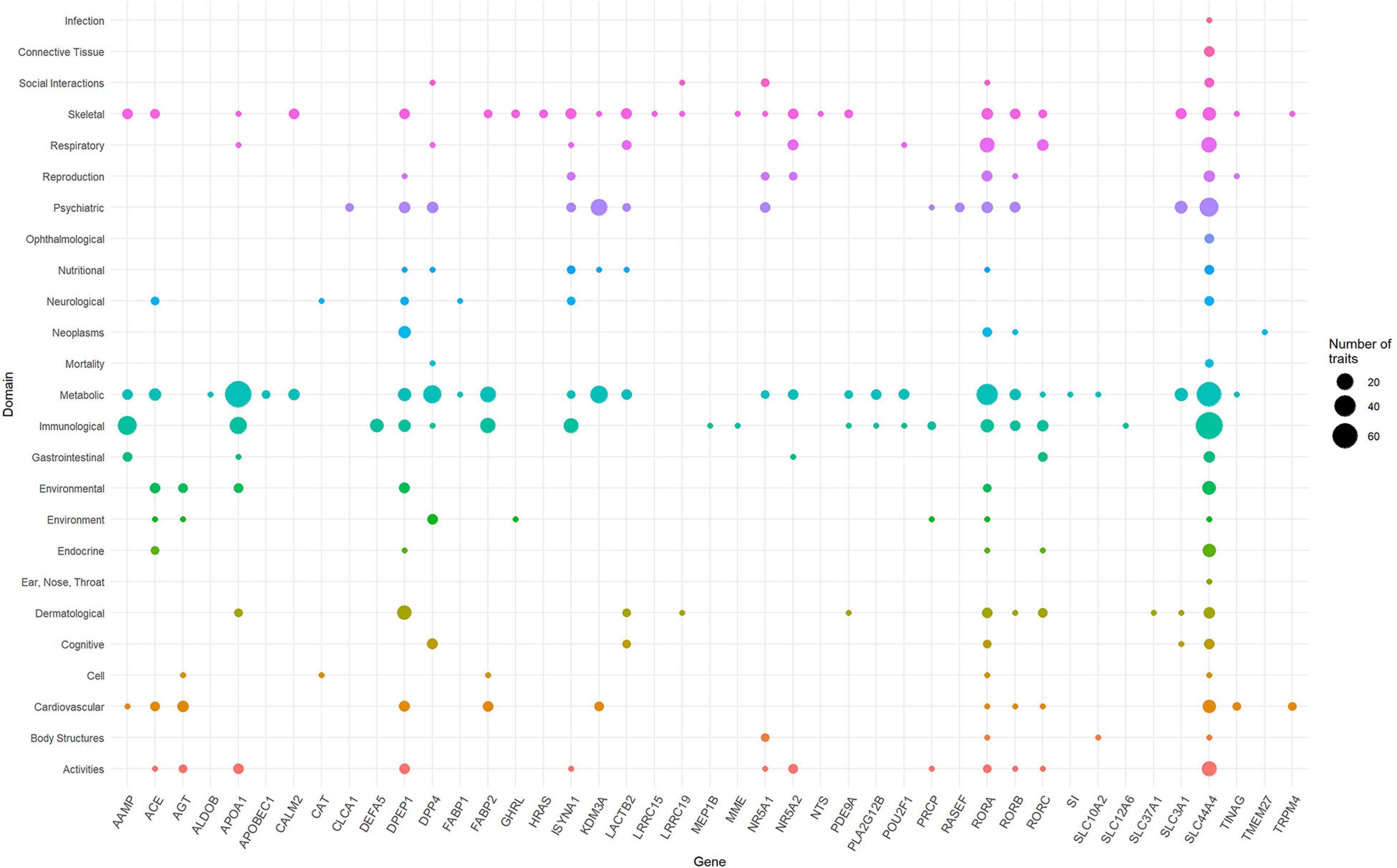
Figure 3. Domain distribution of PheWAS of ACE2-network genes. The ACE2 gene network associations are grouped based on domains (y-axis) and gene names (x-axis). The size of the data points reflects number of phenotypes and corresponding 43 genes surviving multiple testing correction.
Characterization of SNPs
To identify the regulatory role of genetic variations for the ACE2-genes, we report their global allele frequency, functional consequence using pathogenic regulatory score, and disrupting miRNA sites. We extracted all 957,222 SNPs in the ACE2-network and annotated for allele frequency (Supplementary File 3), nearby genes and coordinates (Supplementary File 4), Combined Annotation Dependent Depletion (CADD) (Rentzsch et al., 2019) and DeepSEA (Zhou and Troyanskaya, 2015) scores. There were 98,529 SNPs with CADD score > 10, which corresponds to the top 10% pathogenic variants across the human genome (Supplementary File 5). To identify their regulatory consequences, variants were annotated with DeepSEA which provides functional probability of the SNPs in serving as gene expression, disease and chromatin regulating variants. There were 12,095 SNPs within the ACE2-gene network which had > 50% functional probability (DeepSEA functional score > 0.5) (Supplementary File 6). The miRNAs altered by the SNPs were analyzed for over-represented miRNA-family, biological functions, and diseases considering false discovery rate multiple testing correction (FDR p < 0.05). There were 4 miRNA clusters that were enriched, miR-302b, miR-181d (p = 0.0079), miR-17, and 106a (p = 0.00298). We found 65 biological functions that were significant and the top five significant biological processes were cell death (p = 1.5 × 10–20), inflammation (p = 2.57 × 10–20), cell cycle (p = 2.09 × 10–18), apoptosis (p = 4.15 × 10–18), and immune response (p = 3.17 × 10–17) (Figure 4). We observed a total of 152 significant diseases of which the most significant were diabetes mellitus type 2 (p = 1.55 × 10–22), hepatitis c virus infection (p = 5.56 × 10–21), atherosclerosis (p = 3.08 × 10–19), heart failure (p = 4.22 × 10–19), and Alzheimer’s disease (p = 1.35 × 10–17) (Supplementary Table 8).
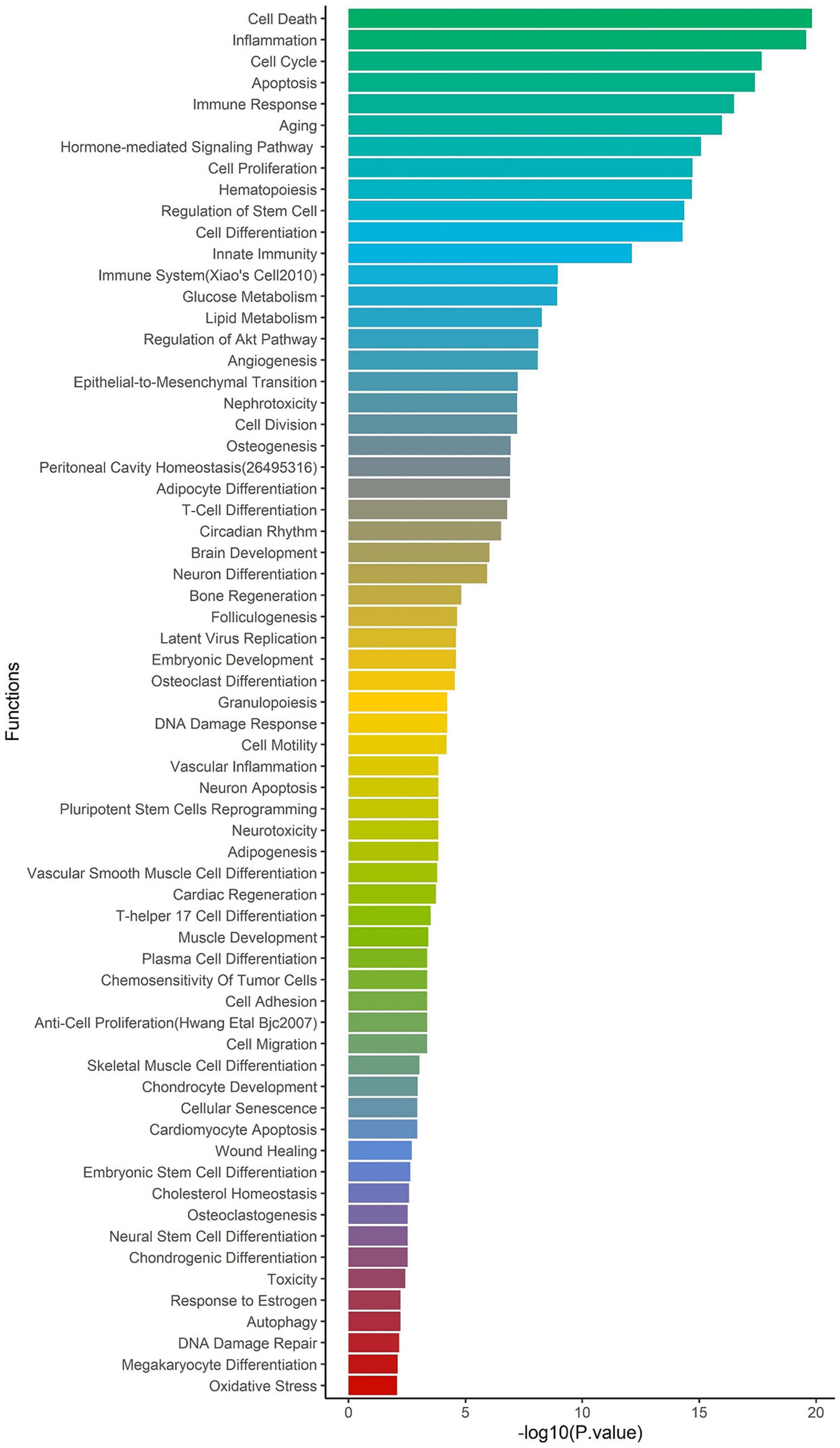
Figure 4. Enrichment of biological functions based on miRNA:SNP annotation. Using miRNAs annotation, over-represented biological processes are shown on y-axis and –log10 p-value on x-axis.
Neanderthal LA Introgression Within ACE2 Network SNPs
Due to the Neanderthal introgression observed in 3p21 locus as risk to COVID-19 (Zeberg and Pääbo, 2020), we compared mean probability of Neanderthal LA between the ACE2-network SNP set (mean = 0.032) and 1,000 randomly selected SNP sets with comparable genomic features (range of Neanderthal LA means = 0.027–0.036). The ACE2-network SNPs did not show evidence of Neanderthal LA introgression significantly different from those expected by chance (p = 0.663) (Supplementary Figure 55).
Functional Annotation of Network SNPs Using the COVID-19 GWAS
We tested ACE2-network SNPs with respect to six COVID-19-related phenotypes (Freeze 3) released by the COVID-19 Host Genetics Initiative (Covid-19 Host Genetics Initiative (HGI), 2020). To identify independent variants, the variants were pruned for linkage disequilibrium (LD < 0.1 within 250 kb genomic size) and clumped for p-value < 0.01. Variants surviving multiple testing were annotated for eQTLs, and mQTLs. Three genes—RORA, SLC12A6, and SLC6A19—showed associations with multiple COVID-19 phenotypes (Supplementary Tables 9–14 and Supplementary Figures 56–61). RORA SNPs were associated with COVID-19 positive status (rs17303202, p = 2.35e-5), laboratory-confirmed positive COVID-19 status (rs4774377, p = 8.25e-5), hospitalized COVID-19 (rs17303202, p = 2.76e-05), and COVID-19 with very severe respiratory symptoms (rs341419, p = 8.13e-4). The SNPs (rs12912196) in RORA gene are also associated with gene expression (eQTL) of RORA gene (p = 3.9e-5) and mQTL (cg00930615, p = 7.84e-7) in ANXA2. SLC12A6 associations were observed with respect to COVID-19 (rs145719616, p = 1.19e-4), hospitalized COVID-19 (rs192235418, p = 4.42e-4), COVID-19 with very severe respiratory (rs2705343, p = 1.86e-3), and. SLC6A19 SNPs were associated with severe COVID-19 phenotype definitions, i.e., COVID-19 with very severe respiratory confirmed (rs76067074, p = 2.65e-3) and hospitalized COVID-19 (rs76067074, p = 2.52e-4). Furthermore, the GWAS of hospitalized COVID-19 identified 3p21.31 locus, wherein the genome-wide significant variant—rs13325613 (chr3:46298373bp; p = 1.17e-08) is a trans-QTL for genes RORA (p = 7.7e-7) and RORC (p = 1.3e-31).
We further created a unique comprehensive network by prioritizing genes from the ACE2-network using transcriptomic profile specific to SARS-CoV-2, over-represented domains for traits associated with ACE2-network genes, and the gene-drug targets that were overrepresented for biological functions (Figure 5).
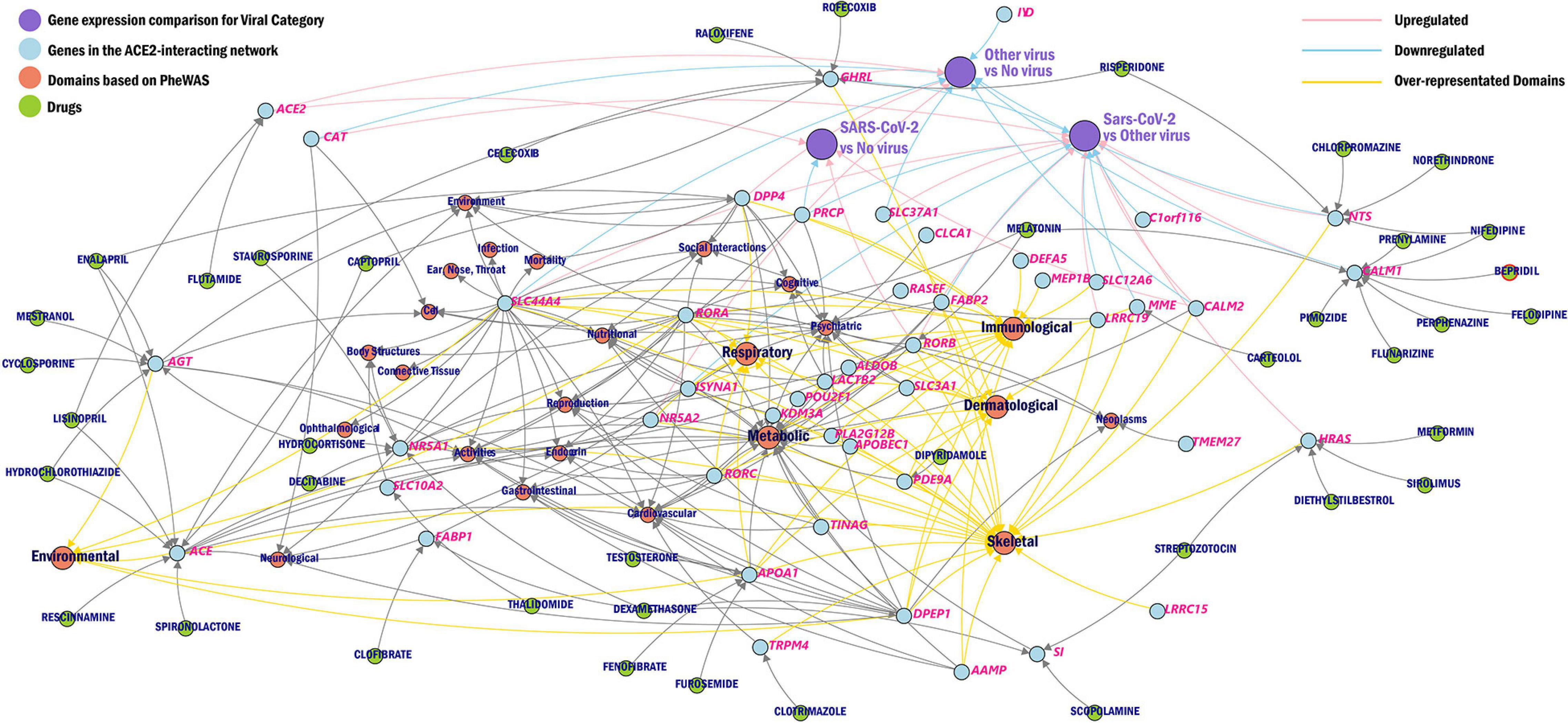
Figure 5. Network of ACE2 interacting genes. The overview of ACE2-network genes (blue) as drug targets (green), phenotype domains (red), and gene expression category (purple) wherein the genes are significant from the CZBioHub (see text for details). The pink edges represent upregulation and blue edges represent downregulation, and yellow edges connect genes to overrepresented phenotype domains.
Discussion
ACE2 is expressed in several tissues and plays a key role in host-entry of SARS-CoV-2 (Hoffmann et al., 2020). However, the genomic profile of ACE2 is limited in explaining the vast symptomology observed for COVID-19. Understanding ACE2 associated molecular networks presents several functional insights between genetic targets based on gene expression, topology, and protein and signaling relationships (Huang et al., 2018). Due to the well-characterized role of ACE2 in SARS-CoV-2 infection, we generated novel information regarding the molecular and phenotypic characteristics of ACE gene network in the context of their potential involvement in COVID-19 susceptibility. Our PheWAS-based analysis showed that genetic variation within ACE2 gene network is associated with immunity (white blood cell, neutrophil count, lymphocyte count), respiratory (FVC, asthma, DVT), and metabolic traits (BMI, cholesterol, body measurements). This is in line with known epidemiology of COVID-19 and its comorbidities (Ejaz et al., 2020; Gardinassi et al., 2020).
The expression of ACE2-network genes was enriched for regulatory mechanisms related to small intestine, colon, kidney, and liver. It is hypothesized that furin, a serine protease present in lungs but also highly expressed in small intestine, and is involved in the cleavage of S-spike for attachment of the ACE2 receptor (Mönkemüller et al., 2020). Patients with kidney disease have higher risk for COVID-19 severe symptoms (Ajaimy and Melamed, 2020). Additionally, the inflammation and cytokine storm from COVID-19 is observed to damage kidney tissues (Gao et al., 2020). Lastly, modest increase in liver enzymes has been associated with COVID-19, and returning to baseline during the recovery phase (Pawlotsky, 2020).
Understanding the genes that interact with ACE2 receptor has potential to understand drug-targets and molecular processes that might play a role in susceptibility and treatment response of COVID-19. The drug-gene interaction analysis within ACE2 network identified dexamethasone, reported to lower mortality in COVID-19 cases requiring mechanical ventilation (RECOVERY Collaborative Group et al., 2020). Drugs—spironolactone and hydrocortisone target the androgen system. Androgen signaling modulates ACE2 expression and elevated androgen levels have been associated with severe symptomology of COVID-19 (Samuel et al., 2020). Spironolactone is a diuretic and alleviates respiratory symptoms by reducing fluid from the lungs (Cadegiani et al., 2020). The use of spironolactone is currently being tested for acute respiratory distress syndrome in COVID-19 patients (Dumanlı et al., 2020). Hydrocortisone is currently under clinical trials for treating COVID-19 related hypoxia symptoms (Petersen et al., 2020). Among the other compounds identified, metformin, a known drug for treating diabetes, can also affect respiratory outcomes (Yen et al., 2020). A recent study reported protective effects of metformin in women with diabetes and obesity who were admitted with COVID-19 diagnosis (Bramante et al., 2020). Lastly, melatonin has been hypothesized to improve general immunity and lower oxidative stress generated from SARS-CoV-2 infection (Shneider et al., 2020).
The miRNA target sites altered by ACE2-network SNPs identified miR-302b and miR-181d as over-represented miRNA clusters. The downregulated expression of miR-302b has been observed to reduce survival rates in chronic obstructive pulmonary disease (COPD) patients (Keller et al., 2019). A meta-analysis showed that COPD diagnosis increased susceptibility to COVID-19 (Lippi and Henry, 2020). The miRNA-181 cluster has been associated with regulation of TNF-alpha (Zhu et al., 2017), T-cell aging (Ye et al., 2018) and emphysema (Osei et al., 2015). miRNA-17 and 106 belong to same miRNA family, miRNA-17 is upregulated in bronchoalveolar stem cells to lower SARS-CoV replication (Mallick et al., 2009). An in silico study of miRNA targets for SARS-CoV-2 genomic sequence found miRNA-17 as one of the targets with experimental evidence of its upregulation in H7N9 Influenza virus infection (Khan M.A.A.K. et al., 2020). The top over-represented diseases in miRNA-ACE2-network-SNPs were diabetes, hepatitis C viral infection, heart failure and Alzheimer’s disease. A greater number of diabetic individuals with COVID-19 have been reported to require hospitalization than non-diabetic individuals (Apicella et al., 2020). Furthermore, SARS-CoV-2 infection contributes in the development of ketosis in diabetic individuals resulting in longer length of hospitalization stay (Li et al., 2020). Triglyceride and glucose index was associated with severity of COVID-19 (Ren et al., 2020). While there are limited studies about hepatitis C in COVID-19 patients (Richardson et al., 2020), heart failure was reported by multiple studies as being associated with COVID-19 severity (Hanley et al., 2020; Yancy and Fonarow, 2020). Alzheimer’s disease is another condition associated with COVID-19 susceptibility (Chang et al., 2020), including APOE4 carrier status with increased risk of severe COVID-19 (Kuo et al., 2020).
In contrast to specific enrichment of Neanderthal LA in a COVID-19 risk locus on chromosome 3 (Zeberg and Pääbo, 2020), there is no evidence of increased Neanderthal LA in the ACE2 network investigated here. This suggests that, although some loci conferring risk for COVID-19 severity, such as the one identified on chromosome 3, may have originated from Neanderthal admixture events, this mechanism did not shape the genetic architecture of the ACE2 network responsible for entry of SARS-CoV-2 into host cellular machinery.
Lastly, among ACE2-network-SNPs, potential COVID-19 risk alleles were observed in RORA gene with respect to multiple COVID-19 phenotypes. RORA protein product is involved in immune response, cancer and metabolism (Cook et al., 2015). RORA plays a role in the activation of T helper cells during lung inflammation by regulating tumor necrosis factor and interleukins (Nejati Moharrami et al., 2018; Haim-Vilmovsky et al., 2019), and was upregulated in cardiomyocytes infected with SARS-CoV-2 (Hachim et al., 2020). The hypothesis-free approach of genome-wide association of hospitalized COVID-19 vs. the population highlighted SLC6A20 with genome-wide significance on chromosome 3 locus. The SLC12 (SLC12A6) class is responsible for transport of inorganic ions such as sodium and chloride while the SLC6 class (SLC6A19, identified via network approach and SLC6A20, identified via genome-wide approach) are responsible for transport of amino acids such as glutamate and glycine which are important for neurotransmitter activity (Lin et al., 2015). SLC6A19 (among other SLC-class genes) serves similar function to SLC6A20, both are expressed in the intestinal tissue and contingent upon ACE2 expression (Vuille-Dit-Bille et al., 2020). Multiple studies report more than 10% of the COVID-19 confirmed patients exhibit gastrointestinal symptoms (Jin et al., 2020; Khan M. et al., 2020; Lian et al., 2020).
Although we provided a wide range of information highlighting the molecular and phenotypic characteristics of ACE2 gene network and their putative implications with COVID-19 risk, the findings reported have to be considered exploratory. We used appropriate computational methods and statistical approaches to generate reliable evidence useful to open new directions in COVID-19 research. We also highlighted when the results reported did not survive stringent multiple testing correction. This limitation is particularly relevant with respect to the ACE2 network genetic associations. Due to the limited statistical power of the genome-wide data available for the Freeze 3 data from the COVID-19 Host Genetics Initiative, none of the risk alleles identified as functionally relevant survive genome-wide testing correction. Future work from the HGI will potentially lead to more risk loci being identified. Further analyses will be needed to validate our current findings.
Conclusion
ACE2 is one of the few molecular targets recognized to play a key role in the COVID-19 pathogenesis. We conducted a comprehensive analysis leveraging multiple resources (e.g., drug-gene interactions, tissue-specific transcriptomic profile, and phenome-wide and genome-wide datasets) to expand our understanding of the genomic characteristics of the host ACE2 gene network. Overall, our findings incorporate multi-tiered epigenomic, transcriptomic, and genomics of the known ACE2-network which highlight the potential mechanisms linking ACE2 systems biology to COVID-19 susceptibility and its possible comorbidities.
Methods
Gene Network Collection
Information regarding ACE2 gene network was mined from GeneMANIA (Franz et al., 2018), Stringdb (Szklarczyk et al., 2017), Agile Protein Interactomes Database (APID) (Prieto and De Las Rivas, 2006), GeneNetwork (Deelen et al., 2019), Biogrid (Oughtred et al., 2019), and FunctionalNet (Lee et al., 2011) for Homo sapiens organism, last searched on June 27, 2020. Since all resources use different algorithms, using ACE2 as query gene, immediate genes connections that were available in each databank were retrieved with their default settings. Removing overlapping genes across the six databases, resulted in 61 unique genes (60 genes plus ACE2) (Supplementary Figure 1 and Supplementary Table 1). Specifically, GeneMANIA uses automatically selected weighting method for 20 max resultant genes with 10 max resultant attributes. In Stringdb, the ACE2 gene query with medium confidence interaction score, and including all active interaction source except text mining. For APID, single query of ACE2 generated a network of 11 interacting genes. In GeneNetwork, we used ACE2 as query genes and selected co-regulated genes with evidence of p < 2e-12. In Biogrid, we used ACE2 in Homo Sapiens as query, and used the network information. In FunctionalNet, the ACE2 was searched using its ENTREZ id (59272) and selected the genelist that interact with ACE2. We focused on the genes that interact immediately with ACE2 because including more genes that interact through intermediate genes will result in large volume data and difficult to interpret. Several genes were identified by multiple sources listed in Supplementary Table 1, and a total of 61 genes including ACE2 were investigated for their characteristics. The genomic coordinates for the genes were annotated using biomart (Durinck et al., 2009), ensemble GRCh37/hg19. The analysis and visualization were performed in R 3.6.
Tissue-Specific Transcriptomic Regulation
The tissue specificity was tested for 60 ACE2-interacting genes in FUMA (Watanabe et al., 2017). The input genes were tested for pre-calculated tissue-specific differentially expressed genes from the GTEx v8 (Aguet et al., 2019). We also considered the t-statistic sign for up and down-regulated genes against protein coding genes as background. Enrichments were performed using hypergeometric tests and significant enrichments were defined according to Bonferroni corrected p-value < 0.05.
Gene Expression of ACE2-Interacting Genes in Upper Respiratory Tissue for SARS-CoV-2 and Other Viruses
To understand which genes from the ACE2-interacting genes are differentially expressed in SARS-CoV-2 and other viruses, we extracted these genes from transcriptomic study of acute respiratory illnesses for COVID-19 patients (N = 93), other viral (N = 41) or non-viral (N = 100) in the upper respiratory tract tissue (Mick et al., 2020). Their data was extracted from https://github.com/czbiohub/covid19-transcriptomics-pathogenesis-diagnostics-results. We used ENSEMBL identifiers of 61 ACE2 interacting genes and were able to extract 35/61 genes. The study performed three gene expression comparisons, SARS-CoV-2 vs. no-virus, SARS-CoV-2 vs. other-virus, other-virus vs. no-virus, and genes with Benjamin-Hochberg adjusted p-value < 0.05 were assigned as significant to the respective comparison.
Phenome-Wide Analysis of ACE2 Gene Network
A phenome-wide association study (PheWAS) was performed for 51 of 61 genes that were present in GWASAtlas (Watanabe et al., 2019) using all traits available per gene. Statistical significance was determined by applying a Bonferroni multiple-testing correction accounting for the number of GWAS traits (4,765 traits) available in the GWASAtlas (p < 1.05 × 10–5). Each trait was grouped into a domain (Supplementary Table 5) which was tested for enrichment using one-sided Fisher’s exact test for high proportion of significant traits vs. all others tested. A significant domain enrichment was defined considering a Bonferroni-corrected threshold accounting for the number of domains tested (p-value < 0.0019; 0.05/26).
Gene-Drug Interactions and Biological Functions
Information on drugs that interact with ACE2 network genes were extracted from The Drug-Gene Interaction database (DGIdb) (Griffith et al., 2013) followed by drug-set enrichment for over represented biological functions using DSEA (Drug-Set Enrichment Analysis) (Napolitano et al., 2016).
Characterization of SNPs
Single nucleotide polymorphism (SNPs) were extracted based on the genomic coordinates of the genes (± 10 kb) for GrCh37; dbSNP153 from the UCSC browser (Haeussler et al., 2019) using bigbed utilities (Karolchik et al., 2004), and referred to as “ACE2-network SNPs.” ACE2-network SNPs were annotated for global allele frequency, Combined Annotation-Dependent Depletion (CADD) score (Rentzsch et al., 2019), deep learning based algorithm framework (DeepSEA) (Zhou and Troyanskaya, 2015), and target miRNAs using SNPnexus (Dayem Ullah et al., 2018). DeepSEA is a deep learning-based algorithmic framework for predicting the chromatin effects of sequence alterations with single nucleotide sensitivity (Zhou and Troyanskaya, 2015). The identified miRNAs were tested for over-represented miRNA clusters, functions, and diseases using TAM 2.0 (Li et al., 2018).
Neanderthal Introgression
Motivated by evidence of a chromosome 3 COVID-19 risk locus enriched of Neanderthal local ancestry (LA) (Zeberg and Pääbo, 2020), we compared the distribution of probability of Neanderthal LA in our COVID-19 ACE2-network SNP set and 1,000 randomly sampled SNP sets comprised on SNPs across the genome with comparable genomic features. ACE2-network SNPs were mapped using previously defined Neanderthal LA data (Sankararaman et al., 2014; Durvasula and Sankararaman, 2019). A total of 6,822 LD-independent pairwise SNPs (r2 = 0.1 and p = 0.1 in 250 kb window size) were used as standard input for SNPsnap (Pers et al., 2015). In SNPsnap, 1,249/6,822 independent ACE2 network SNPs could be matched based on the following genomic features relative to the input SNP list: minor allele frequency within 2%, gene density within 50%, nearest gene within 50%, and number of linkage disequilibrium groups within 50%. SNPsnap was instructed to exclude the ACE2-network SNP list from the pool of eligible feature-matched SNPs. Non-parametric Wilcoxon rank sum tests were used to compare the Neanderthal LA of our ACE2 network SNP list to that of all 1,000 random SNP sets and multiple testing correction was applied to adjust for a false discovery rate of 5%.
Association Statistics of ACE2 Network SNPs From the COVID-19 Host Genetics Initiative (HGI)
The ACE2-network SNPs were extracted from association statistics released by the COVID-19 HGI (COVID-19 Host Genetics Initiative [HGI], 2020) for six phenotypes describing COVID-19 susceptibility. These phenotypes were A2_V2 [very severe respiratory confirmed COVID-19 cases (N = 536) vs. population (N = 329391)], B1_V2 [hospitalized COVID-19 cases (N = 928) vs. not hospitalized COVID-19 cases (N = 2028)], B2_V2 [hospitalized COVID-19 cases (N = 3199) vs. population (N = 897488)], C1_V2 [COVID-19 cases (N = 3523) vs. lab/self-reported negative (N = 36634)], C2_V2 [COVID-19 cases (N = 6696) vs. population (N = 1073072)], and D1_V2 [predicted COVID-19 cases from self-reported symptoms (N = 1865) vs. predicted or self-reported non-COVID-19 cases (N = 29174)]. The SNPs of the ACE2 network were extracted and pruned for LD and p-value using plink 1.9. The multiple testing correction was applied using Bonferroni p-value < 0.05. These significant SNPs were annotated further for pathogenicity using Combined Annotation Dependent Depletion (CADD) score and their role as quantitative trait loci (QTL) for gene expression using GTEx, and methylation using QTLbase (Zheng et al., 2020). The trans-eQTL relationship of GWAS-reported locus-3p21.31 were identified from eQTLgen (Võsa et al., 2018).
Data Availability Statement
The raw data supporting the conclusions of this article will be made available by the authors, without undue reservation, to any qualified researcher. The data presented is available in Supplementary Files and also on https://gpwhiz.github.io/ACE2Netlas/.
Ethics Statement
Ethical review and approval was not required for the study on human participants in accordance with the local legislation and institutional requirements. The patients/participants provided their written informed consent to participate in their original studies reported.
Author Contributions
GP conceptualized the study design, analyzed, and drafted the manuscript. FW contributed to analysis and manuscript writing. AG, DK, FD, and RP contributed to result interpretation, manuscript drafting and revision. RP supervised the study and finalized the manuscript. All authors contributed to the article and approved the submitted version.
Funding
We would like to acknowledge support from the National Institutes of Health (R21 DC018098, R21 DA047527 and F32 MH122058).
Conflict of Interest
The authors declare that the research was conducted in the absence of any commercial or financial relationships that could be construed as a potential conflict of interest.
Publisher’s Note
All claims expressed in this article are solely those of the authors and do not necessarily represent those of their affiliated organizations, or those of the publisher, the editors and the reviewers. Any product that may be evaluated in this article, or claim that may be made by its manufacturer, is not guaranteed or endorsed by the publisher.
Acknowledgments
We thank the COVID-19 Host Genetics Initiative (https://www.covid19hg.org/acknowledgements/) for providing open access to genetic association data. The content of this manuscript has been presented at the American Society of Human Genetics (Virtual) 2020 (https://www.abstractsonline.com/pp8/#!/9070/presentation/1977).
Supplementary Material
The Supplementary Material for this article can be found online at: https://www.frontiersin.org/articles/10.3389/fgene.2021.698033/full#supplementary-material
References
Aguet, F., Barbeira, A. N., Bonazzola, R., Brown, A., Castel, S. E., Jo, B., et al. (2019). The GTEx Consortium atlas of genetic regulatory effects across human tissues. bioRxiv [Preprint]. doi: 10.1101/787903
Ajaimy, M., and Melamed, M. L. (2020). COVID-19 in Patients with Kidney Disease. Clin. J. Am. Soc. Nephrol. 15, 1087–1089. doi: 10.2215/CJN.09730620
Apicella, M., Campopiano, M. C., Mantuano, M., Mazoni, L., Coppelli, A., and Del Prato, S. (2020). COVID-19 in people with diabetes: understanding the reasons for worse outcomes. Lancet Diabetes Endocrinol. 8, 782–792. doi: 10.1016/S2213-8587(20)30238-2
Bramante, C., Ingraham, N., Murray, T., Marmor, S., Hoversten, S., Gronski, J., et al. (2020). Observational Study of Metformin and Risk of Mortality in Patients Hospitalized with Covid-19. medRxiv [Preprint]. doi: 10.1101/2020.06.19.20135095
Cadegiani, F. A., Goren, A., and Wambier, C. G. (2020). Spironolactone may provide protection from SARS-CoV-2: targeting androgens, angiotensin converting enzyme 2 (ACE2), and renin-angiotensin-aldosterone system (RAAS). Med. Hypotheses 143:110112. doi: 10.1016/j.mehy.2020.110112
Chang, T. S., Ding, Y., Freund, M. K., Johnson, R., Schwarz, T., Yabu, J. M., et al. (2020). Prior diagnoses and medications as risk factors for COVID-19 in a Los Angeles Health System. medRxiv [Preprint]. doi: 10.1101/2020.07.03.20145581
Cook, D. N., Kang, H. S., and Jetten, A. M. (2015). Retinoic Acid-Related Orphan Receptors (RORs): regulatory Functions in Immunity, Development, Circadian Rhythm, and Metabolism. Nucl. Receptor Res. 2:101185. doi: 10.11131/2015/101185
Covid-19 Host Genetics Initiative (HGI). (2020). The COVID-19 Host Genetics Initiative, a global initiative to elucidate the role of host genetic factors in susceptibility and severity of the SARS-CoV-2 virus pandemic. Eur. J. Hum. Genet. 28, 715–718. doi: 10.1038/s41431-020-0636-6
Dayem Ullah, A. Z., Oscanoa, J., Wang, J., Nagano, A., Lemoine, N. R., and Chelala, C. (2018). SNPnexus: assessing the functional relevance of genetic variation to facilitate the promise of precision medicine. Nucleic Acids Res. 46, W109–W113. doi: 10.1093/nar/gky399
Deelen, P., van Dam, S., Herkert, J. C., Karjalainen, J. M., Brugge, H., Abbott, K. M., et al. (2019). Improving the diagnostic yield of exome- sequencing by predicting gene-phenotype associations using large-scale gene expression analysis. Nat. Commun. 10:2837. doi: 10.1038/s41467-019-10649-4
Dumanlı, G. Y., Dilken, O., and Ürkmez, S. (2020). Use of Spironolactone in SARS-CoV-2 ARDS Patients. Turk. J. Anaesthesiol. Reanim. 48, 254–255. doi: 10.5152/TJAR.2020.569
Durinck, S., Spellman, P. T., Birney, E., and Huber, W. (2009). Mapping identifiers for the integration of genomic datasets with the R/Bioconductor package biomaRt. Nat. Protoc. 4, 1184–1191. doi: 10.1038/nprot.2009.97
Durvasula, A., and Sankararaman, S. (2019). A statistical model for reference-free inference of archaic local ancestry. PLoS Genet. 15:e1008175. doi: 10.1371/journal.pgen.1008175
Ejaz, H., Alsrhani, A., Zafar, A., Javed, H., Junaid, K., Abdalla, A. E., et al. (2020). COVID-19 and comorbidities: deleterious impact on infected patients. J. Infect. Public Health 13, 1833–1839. doi: 10.1016/j.jiph.2020.07.014
Ellinghaus, D., Degenhardt, F., Bujanda, L., Buti, M., Albillos, A., Invernizzi, P., et al. (2020). Genomewide Association Study of Severe Covid-19 with Respiratory Failure. N. Engl. J. Med. 383, 1522–1534. doi: 10.1056/NEJMoa2020283
Franz, M., Rodriguez, H., Lopes, C., Zuberi, K., Montojo, J., Bader, G. D., et al. (2018). GeneMANIA update 2018. Nucleic Acids Res. 46, W60–W64. doi: 10.1093/nar/gky311
Gao, M., Wang, Q., Wei, J., Zhu, Z., and Li, H. (2020). Severe Coronavirus disease 2019 pneumonia patients showed signs of aggravated renal impairment. J. Clin. Lab. Anal. 34:e23535. doi: 10.1002/jcla.23535
Gardinassi, L. G., Souza, C. O. S., Sales-Campos, H., and Fonseca, S. G. (2020). Immune and Metabolic Signatures of COVID-19 Revealed by Transcriptomics Data Reuse. Front. Immunol. 11:1636. doi: 10.3389/fimmu.2020.01636
Griffith, M., Griffith, O. L., Coffman, A. C., Weible, J. V., McMichael, J. F., Spies, N. C., et al. (2013). DGIdb: mining the druggable genome. Nat. Methods 10, 1209–1210. doi: 10.1038/nmeth.2689
Hachim, M. Y., Al Heialy, S., Senok, A., Hamid, Q., and Alsheikh-Ali, A. (2020). Molecular Basis of Cardiac and Vascular Injuries Associated With COVID-19. Front. Cardiovasc. Med. 7:582399. doi: 10.3389/fcvm.2020.582399
Haeussler, M., Zweig, A. S., Tyner, C., Speir, M. L., Rosenbloom, K. R., Raney, B. J., et al. (2019). The UCSC Genome Browser database: 2019 update. Nucleic Acids Res. 47, D853–D858. doi: 10.1093/nar/gky1095
Haim-Vilmovsky, L., Walker, J. A., Henriksson, J., Miao, Z., Natan, E., Kar, G., et al. (2019). Rora regulates activated T helper cells during inflammation. bioRxiv [Preprint]. doi: 10.1101/709998
Hamming, I., Cooper, M. E., Haagmans, B. L., Hooper, N. M., Korstanje, R., Osterhaus, A. D. M. E., et al. (2007). The emerging role of ACE2 in physiology and disease. J. Pathol. 212, 1–11. doi: 10.1002/path.2162
Hanley, B., Naresh, K. N., Roufosse, C., Nicholson, A. G., Weir, J., Cooke, G. S., et al. (2020). Histopathological findings and viral tropism in UK patients with severe fatal COVID-19: a post-mortem study. Lancet Microbe 1, E245–E253. doi: 10.1016/S2666-5247(20)30115-4
Hoffmann, M., Kleine-Weber, H., Schroeder, S., Krüger, N., Herrler, T., Erichsen, S., et al. (2020). SARS-CoV-2 Cell Entry Depends on ACE2 and TMPRSS2 and Is Blocked by a Clinically Proven Protease Inhibitor. Cell 181, 271–280.e8. doi: 10.1016/j.cell.2020.02.052
Huang, J. K., Carlin, D. E., Yu, M. K., Zhang, W., Kreisberg, J. F., Tamayo, P., et al. (2018). Systematic evaluation of molecular networks for discovery of disease genes. Cell Syst. 6, 484–495.e5. doi: 10.1016/j.cels.2018.03.001
Jin, X., Lian, J.-S., Hu, J.-H., Gao, J., Zheng, L., Zhang, Y.-M., et al. (2020). Epidemiological, clinical and virological characteristics of 74 cases of coronavirus-infected disease 2019 (COVID-19) with gastrointestinal symptoms. Gut 69, 1002–1009. doi: 10.1136/gutjnl-2020-320926
Karolchik, D., Hinrichs, A. S., Furey, T. S., Roskin, K. M., Sugnet, C. W., Haussler, D., et al. (2004). The UCSC Table Browser data retrieval tool. Nucleic Acids Res. 32, D493–D496. doi: 10.1093/nar/gkh103
Keller, A., Ludwig, N., Fehlmann, T., Kahraman, M., Backes, C., Kern, F., et al. (2019). Low miR-150-5p and miR-320b Expression Predicts Reduced Survival of COPD Patients. Cells 8:1162. doi: 10.3390/cells8101162
Khan, M. A. A. K., Sany, M. R. U., Islam, M. S., Mehebub, M. S., and Islam, A. B. M. M. K. (2020). Epigenetic regulator miRNA pattern differences among SARS-CoV, SARS-CoV-2 and SARS-CoV-2 world-wide isolates delineated the mystery behind the epic pathogenicity and distinct clinical characteristics of pandemic COVID-19. bioRxiv [Preprint]. doi: 10.1101/2020.05.06.081026
Khan, M., Khan, H., Khan, S., and Nawaz, M. (2020). Epidemiological and clinical characteristics of coronavirus disease (COVID-19) cases at a screening clinic during the early outbreak period: a single-centre study. J. Med. Microbiol. 69, 1114–1123. doi: 10.1099/jmm.0.001231
Kuo, C.-L., Pilling, L. C., Atkins, J. L., Masoli, J. A. H., Delgado, J., Kuchel, G. A., et al. (2020). APOE e4 genotype predicts severe COVID-19 in the UK Biobank community cohort. J. Gerontol. A Biol. Sci. Med. Sci. 75, 2231–2232. doi: 10.1093/gerona/glaa131
Lee, I., Blom, U. M., Wang, P. I., Shim, J. E., and Marcotte, E. M. (2011). Prioritizing candidate disease genes by network-based boosting of genome-wide association data. Genome Res. 21, 1109–1121. doi: 10.1101/gr.118992.110
Li, J., Han, X., Wan, Y., Zhang, S., Zhao, Y., Fan, R., et al. (2018). TAM 2.0: tool for MicroRNA set analysis. Nucleic Acids Res. 46, W180–W185. doi: 10.1093/nar/gky509
Li, J., Wang, X., Chen, J., Zuo, X., Zhang, H., and Deng, A. (2020). COVID-19 infection may cause ketosis and ketoacidosis. Diabetes Obes. Metab. 22, 1935–1941. doi: 10.1111/dom.14057
Lian, J., Jin, X., Hao, S., Jia, H., Cai, H., Zhang, X., et al. (2020). Epidemiological, clinical, and virological characteristics of 465 hospitalized cases of coronavirus disease 2019 (COVID-19) from Zhejiang province in China. Influenza Other Respir. Viruses 14, 564–574. doi: 10.1111/irv.12758
Lin, L., Yee, S. W., Kim, R. B., and Giacomini, K. M. (2015). SLC transporters as therapeutic targets: emerging opportunities. Nat. Rev. Drug Discov. 14, 543–560. doi: 10.1038/nrd4626
Lippi, G., and Henry, B. M. (2020). Chronic obstructive pulmonary disease is associated with severe coronavirus disease 2019 (COVID-19). Respir. Med. 167:105941. doi: 10.1016/j.rmed.2020.105941
Mallick, B., Ghosh, Z., and Chakrabarti, J. (2009). MicroRNome analysis unravels the molecular basis of SARS infection in bronchoalveolar stem cells. PLoS One 4:e7837. doi: 10.1371/journal.pone.0007837
Mercurio, I., Tragni, V., Busto, F., De Grassi, A., and Pierri, C. L. (2021). Protein structure analysis of the interactions between SARS-CoV-2 spike protein and the human ACE2 receptor: from conformational changes to novel neutralizing antibodies. Cell Mol. Life Sci. 78, 1501–1522. doi: 10.1007/s00018-020-03580-1
Mick, E., Kamm, J., Pisco, A. O., Ratnasiri, K., Babik, J. M., Castañeda, G., et al. (2020). Upper airway gene expression reveals suppressed immune responses to SARS-CoV-2 compared with other respiratory viruses. Nat. Commun. 11:5854. doi: 10.1038/s41467-020-19587-y
Mönkemüller, K., Fry, L., and Rickes, S. (2020). COVID-19, coronavirus, SARS-CoV-2 and the small bowel. Rev. Esp. Enferm. Dig. 112, 383–388. doi: 10.17235/reed.2020.7137/2020
Napolitano, F., Sirci, F., Carrella, D., and di Bernardo, D. (2016). Drug-set enrichment analysis: a novel tool to investigate drug mode of action. Bioinformatics 32, 235–241. doi: 10.1093/bioinformatics/btv536
Nejati Moharrami, N., Bjørkøy Tande, E., Ryan, L., Espevik, T., and Boyartchuk, V. (2018). RORα controls inflammatory state of human macrophages. PLoS One 13:e0207374. doi: 10.1371/journal.pone.0207374
Osei, E. T., Florez-Sampedro, L., Timens, W., Postma, D. S., Heijink, I. H., and Brandsma, C.-A. (2015). Unravelling the complexity of COPD by microRNAs: it’s a small world after all. Eur. Respir. J. 46, 807–818. doi: 10.1183/13993003.02139-2014
Oughtred, R., Stark, C., Breitkreutz, B.-J., Rust, J., Boucher, L., Chang, C., et al. (2019). The BioGRID interaction database: 2019 update. Nucleic Acids Res. 47, D529–D541. doi: 10.1093/nar/gky1079
Pairo-Castineira, E., Clohisey, S., Klaric, L., Bretherick, A., Rawlik, K., Parkinson, N., et al. (2020). Genetic mechanisms of critical illness in Covid-19. medRxiv [Preprint]. doi: 10.1101/2020.09.24.20200048
Pawlotsky, J.-M. (2020). COVID-19 and the liver-related deaths to come. Nat. Rev. Gastroenterol. Hepatol. 17, 523–525. doi: 10.1038/s41575-020-0328-2
Pers, T. H., Timshel, P., and Hirschhorn, J. N. (2015). SNPsnap: a Web-based tool for identification and annotation of matched SNPs. Bioinformatics 31, 418–420. doi: 10.1093/bioinformatics/btu655
Petersen, M. W., Meyhoff, T. S., Helleberg, M., Kjær, M.-B. N., Granholm, A., Hjortsø, C. J. S., et al. (2020). Low-dose hydrocortisone in patients with COVID-19 and severe hypoxia (COVID STEROID) trial-Protocol and statistical analysis plan. Acta Anaesthesiol. Scand. 64, 1365–1375. doi: 10.1111/aas.13673
Prieto, C., and De Las Rivas, J. (2006). APID: agile protein interaction dataanalyzer. Nucleic Acids Res. 34, W298–W302. doi: 10.1093/nar/gkl128
RECOVERY Collaborative Group, P., Lim, W. S., Emberson, J. R., Mafham, M., Bell, J. L., et al. (2020). Dexamethasone in Hospitalized Patients with Covid-19 - Preliminary Report. N. Engl. J. Med. 384, 693–704. doi: 10.1056/NEJMoa2021436
Ren, H., Yang, Y., Wang, F., Yan, Y., Shi, X., Dong, K., et al. (2020). Association of the insulin resistance marker TyG index with the severity and mortality of COVID-19. Cardiovasc. Diabetol. 19:58. doi: 10.1186/s12933-020-01035-2
Rentzsch, P., Witten, D., Cooper, G. M., Shendure, J., and Kircher, M. (2019). CADD: predicting the deleteriousness of variants throughout the human genome. Nucleic Acids Res. 47, D886–D894. doi: 10.1093/nar/gky1016
Richardson, S., Hirsch, J. S., Narasimhan, M., Crawford, J. M., McGinn, T., Davidson, K. W., et al. (2020). Presenting Characteristics, Comorbidities, and Outcomes Among 5700 Patients Hospitalized With COVID-19 in the New York City Area. JAMA 323, 2052–2059. doi: 10.1001/jama.2020.6775
Samuel, R. M., Majd, H., Richter, M. N., Ghazizadeh, Z., Zekavat, S. M., Navickas, A., et al. (2020). Androgen Signaling Regulates SARS-CoV-2 Receptor Levels and Is Associated with Severe COVID-19 Symptoms in Men. Cell Stem Cell 27, 876–889.e12. doi: 10.1016/j.stem.2020.11.009
Sankararaman, S., Mallick, S., Dannemann, M., Prüfer, K., Kelso, J., Pääbo, S., et al. (2014). The genomic landscape of Neanderthal ancestry in present-day humans. Nature 507, 354–357. doi: 10.1038/nature12961
Shelton, J. F., Shastri, A. J., Ye, C., Weldon, C. H., Filshtein-Somnez, T., Coker, D., et al. (2020). Trans-ethnic analysis reveals genetic and non-genetic associations with COVID-19 susceptibility and severity. medRxiv [Preprint]. doi: 10.1101/2020.09.04.20188318
Shneider, A., Kudriavtsev, A., and Vakhrusheva, A. (2020). Can melatonin reduce the severity of COVID-19 pandemic? Int. Rev. Immunol. 39, 153–162. doi: 10.1080/08830185.2020.1756284
Szklarczyk, D., Morris, J. H., Cook, H., Kuhn, M., Wyder, S., Simonovic, M., et al. (2017). The STRING database in 2017: quality-controlled protein-protein association networks, made broadly accessible. Nucleic Acids Res. 45, D362–D368. doi: 10.1093/nar/gkw937
Võsa, U., Claringbould, A., Westra, H. J., and Bonder, M. J. (2018). Unraveling the polygenic architecture of complex traits using blood eQTL metaanalysis. bioRxiv [Preprint]. doi: 10.1101/447367
Vuille-Dit-Bille, R. N., Liechty, K. W., Verrey, F., and Guglielmetti, L. C. (2020). SARS-CoV-2 receptor ACE2 gene expression in small intestine correlates with age. Amino Acids 52, 1063–1065. doi: 10.1007/s00726-020-02870-z
Walls, A. C., Park, Y.-J., Tortorici, M. A., Wall, A., McGuire, A. T., and Veesler, D. (2020). Structure, Function, and Antigenicity of the SARS-CoV-2 Spike Glycoprotein. Cell 181, 281–292.e6. doi: 10.1016/j.cell.2020.02.058
Wang, D., Hu, B., Hu, C., Zhu, F., Liu, X., Zhang, J., et al. (2020). Clinical Characteristics of 138 Hospitalized Patients With 2019 Novel Coronavirus-Infected Pneumonia in Wuhan, China. JAMA 323, 1061–1069. doi: 10.1001/jama.2020.1585
Watanabe, K., Stringer, S., Frei, O., Umićević Mirkov, M., de Leeuw, C., Polderman, T. J. C., et al. (2019). A global overview of pleiotropy and genetic architecture in complex traits. Nat. Genet. 51, 1339–1348. doi: 10.1038/s41588-019-0481-0
Watanabe, K., Taskesen, E., van Bochoven, A., and Posthuma, D. (2017). Functional mapping and annotation of genetic associations with FUMA. Nat. Commun. 8:1826. doi: 10.1038/s41467-017-01261-5
Wu, F., Zhao, S., Yu, B., Chen, Y.-M., Wang, W., Song, Z.-G., et al. (2020). A new coronavirus associated with human respiratory disease in China. Nature 579, 265–269. doi: 10.1038/s41586-020-2008-3
Yancy, C. W., and Fonarow, G. C. (2020). Coronavirus Disease 2019 (COVID-19) and the Heart-Is Heart Failure the Next Chapter? JAMA Cardiol. 5, 1216–1217. doi: 10.1001/jamacardio.2020.3575
Ye, Z., Li, G., Kim, C., Hu, B., Jadhav, R. R., Weyand, C. M., et al. (2018). Regulation of miR-181a expression in T cell aging. Nat. Commun. 9:3060. doi: 10.1038/s41467-018-05552-3
Yen, F.-S., Wei, J. C.-C., Yang, Y.-C., Hsu, C.-C., and Hwu, C.-M. (2020). Respiratory outcomes of metformin use in patients with type 2 diabetes and chronic obstructive pulmonary disease. Sci. Rep. 10:10298. doi: 10.1038/s41598-020-67338-2
Zeberg, H., and Pääbo, S. (2020). The major genetic risk factor for severe COVID-19 is inherited from Neanderthals. Nature 587, 610–612. doi: 10.1038/s41586-020-2818-3
Zheng, Z., Huang, D., Wang, J., Zhao, K., Zhou, Y., Guo, Z., et al. (2020). QTLbase: an integrative resource for quantitative trait loci across multiple human molecular phenotypes. Nucleic Acids Res. 48, D983–D991. doi: 10.1093/nar/gkz888
Zhou, J., and Troyanskaya, O. G. (2015). Predicting effects of noncoding variants with deep learning-based sequence model. Nat. Methods 12, 931–934. doi: 10.1038/nmeth.3547
Zhou, P., Yang, X.-L., Wang, X.-G., Hu, B., Zhang, L., Zhang, W., et al. (2020). A pneumonia outbreak associated with a new coronavirus of probable bat origin. Nature 579, 270–273. doi: 10.1038/s41586-020-2012-7
Keywords: ACE2, COVID-19, miRNA, immune response, network
Citation: Pathak GA, Wendt FR, Goswami A, Koller D, De Angelis F, COVID-19 Host Genetics Initiative and Polimanti R (2021) ACE2 Netlas: In silico Functional Characterization and Drug-Gene Interactions of ACE2 Gene Network to Understand Its Potential Involvement in COVID-19 Susceptibility. Front. Genet. 12:698033. doi: 10.3389/fgene.2021.698033
Received: 07 May 2021; Accepted: 29 July 2021;
Published: 27 August 2021.
Edited by:
Kristina Allen-Brady, The University of Utah, United StatesReviewed by:
Ana Hernandez Cordero, University of British Columbia, CanadaNasser Pouladi, Azarbaijan Shahid Madani University, Iran
Ciro Leonardo Pierri, University of Bari Aldo Moro, Italy
Copyright © 2021 Pathak, Wendt, Goswami, Koller, De Angelis, COVID-19 Host Genetics Initiative and Polimanti. This is an open-access article distributed under the terms of the Creative Commons Attribution License (CC BY). The use, distribution or reproduction in other forums is permitted, provided the original author(s) and the copyright owner(s) are credited and that the original publication in this journal is cited, in accordance with accepted academic practice. No use, distribution or reproduction is permitted which does not comply with these terms.
*Correspondence: Gita A. Pathak, Z2l0YS5wYXRoYWtAeWFsZS5lZHU=; Renato Polimanti, cmVuYXRvLnBvbGltYW50aUB5YWxlLmVkdQ==