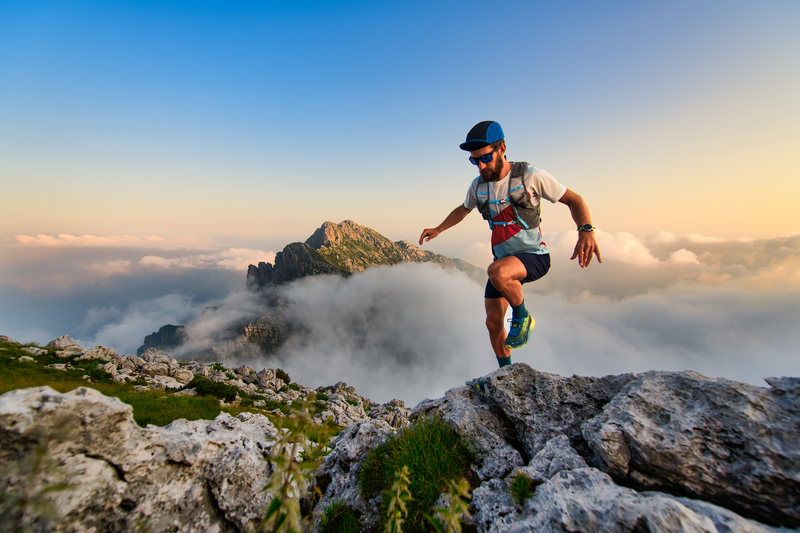
95% of researchers rate our articles as excellent or good
Learn more about the work of our research integrity team to safeguard the quality of each article we publish.
Find out more
ORIGINAL RESEARCH article
Front. Genet. , 19 July 2021
Sec. Computational Genomics
Volume 12 - 2021 | https://doi.org/10.3389/fgene.2021.696836
This article is part of the Research Topic Computational and Experimental Approaches in Exploring the Role of Genetics and Genomics in Multifactorial Diseases View all 6 articles
Background: The development of complex diseases is contributed by the combination of multiple factors and complicated interactions between them. Inflammation has recently been associated with many complex diseases and may cause long-term damage to the human body. In this study, we examined whether two types of complex disease, cerebrovascular disease (CVD) or major depression (MD), systematically altered the transcriptomes of non-diseased human tissues and whether inflammation is linked to identifiable molecular signatures, using post-mortem samples from the Genotype-Tissue Expression (GTEx) project.
Results: Following a series of differential expression analyses, dozens to hundreds of differentially expressed genes (DEGs) were identified in multiple tissues between subjects with and without a history of CVD or MD. DEGs from these disease-associated tissues—the visceral adipose, tibial artery, caudate, and spinal cord for CVD; and the hypothalamus, putamen, and spinal cord for MD—were further analyzed for functional enrichment. Many pathways associated with immunological events were enriched in the upregulated DEGs of the CVD-associated tissues, as were the neurological and metabolic pathways in DEGs of the MD-associated tissues. Eight gene-tissue pairs were found to overlap with those prioritized by our transcriptome-wide association studies, indicating a potential genetic effect on gene expression for circulating cytokine phenotypes.
Conclusion: Cerebrovascular disease and major depression cause detectable changes in the gene expression of non-diseased tissues, suggesting that a possible long-term impact of diseases, lifestyles and environmental factors may together contribute to the appearance of “transcriptomic scars” on the human body. Furthermore, inflammation is probably one of the systemic and long-lasting effects of cerebrovascular events.
Complex diseases are caused by genetic, environmental and lifestyle factors and their interactions, most of which have not yet been identified. Recent studies have revealed that the immune system and inflammatory responses are involved in a wide range of complex diseases, such as cardiovascular disease (Willerson and Ridker, 2004), stroke (Anrather and Iadecola, 2016), cancer (Coussens and Werb, 2002), and psychiatric disorders (Yuan et al., 2019). Inflammation is generally defined as the immune system’s response that defends against injury or stress (Netea et al., 2017). In a normal inflammatory response, the upregulation of inflammatory activity is strictly regulated. However, with psychological, environmental and biological factors (Schneiderman et al., 2005; Sears and Genuis, 2012; Zhu et al., 2014; Furman et al., 2019), the regulated process can become uncontrolled in the resolution phase, causing a systemic chronic inflammation that contributes to damage in all tissues and organs and increases the risk of diseases that remain global leading causes of disability and mortality (Murray and Lopez, 1997; Virani et al., 2020).
The Genotype-Tissue Expression (GTEx) project (Aguet et al., 2020) has established a database of expression data, whole genome sequences, and whole-exome sequences of 54 non-diseased tissue sites across nearly 1,000 individuals (as of the current v8 release). Genotype-Tissue Expression also collected subject phenotype data, including demographic information, general medical histories, histories at the time of death, the circumstances of death, and so on. The medical histories were provided by the hospital systems, which recorded the prior care of deceased donors.
Here we evaluate whether past chronic inflammatory diseases could leave biological alterations (“scars”) in non-diseased tissues of the human. We have focused on two types of complex disease related to the brain, cerebrovascular disease (CVD) and major depression (MD), as they are typical chronic inflammatory diseases with heritable components (Flint and Kendler, 2014; Boehme et al., 2017; Dichgans et al., 2019; Ormel et al., 2019), and there are enough cases of these in the GTEx database. CVD comprises clinical conditions that impair blood flow to the brain, including strokes, transient ischemic attacks, intracranial aneurysms, and other vessel diseases (Goldstein and Lichtman, 2013). Major depression is one of the most common psychiatric illnesses, ranging from 3 to 16.9 percent worldwide (Kessler et al., 2003; Demyttenaere et al., 2004), and has a significant impact on society. It is characterized by a persistent feeling of sadness or a loss of interest or pleasure in outside stimuli. Previous large-scale genome-wide association studies (GWAS) and meta-analyses have identified a large number of genetic loci associated with stroke (Malik et al., 2018; Keene et al., 2020) and depression (Wray et al., 2018; Howard et al., 2019; Ormel et al., 2019) in multi-ancestry groups. However, genetic variability contributing to the susceptibility mechanism underlying CVD and depression as well as their interactions with inflammation remains not fully identified or characterized.
In this study, we aimed to answer the following two questions: (1) is there any significant transcriptomic difference in non-diseased tissues with and without a history of CVD or MD? (2) if yes, is there any evidence to indicate that inflammation may play a role in shaping these transcriptomic landscapes? We performed a differential expression analysis on each GTEx tissue by comparing the expression profiles between subjects with and without the medical history of CVD or MD. Top differentially expressed genes (DEGs) identified in multiple tissues from the series of DE analyses were included in the downstream functional enrichment analysis. We also performed transcriptome-wide association studies (TWAS) on inflammation biomarkers to find any overlaps with the DEGs.
Multi-tissue RNA-seq data were compiled from the GTEx project, as described in the Materials and Methods section. Subjects with an explicitly reported medical history of cerebrovascular disease or major depression were considered in this study. A total of 16,412 samples across 46 tissues, obtained from 928 subjects, were included in the CVD analysis (Supplementary Figure 1), and 16,221 samples across 45 tissues from 926 subjects in the MD analysis (Supplementary Figure 2).
Risk factors of complex diseases include clinical variables such as age (Niccoli and Partridge, 2012), sex (Ober et al., 2008), and BMI (Knight, 2011). The average age of the cohort with a history of CVD was significantly higher than that of the non-CVD cohort (CVD 52.09 ± 13.09, non-CVD 57.74 ± 10.79 yrs, P = 3.87 × 10–6, t-test), while BMI, sex and race showed no significant differences between the two groups (Supplementary Figure 3). The average age of the MD cohort was younger than that of the non-MD cohort (MD 49.69 ± 13.33, non-MD 53.07 ± 12.91 yrs, P = 1.82 × 10–2, t-test). Moreover, females had a higher incidence of developing depression (P = 0.01, Chi-squared test) (Supplementary Figure 4). This is consistent with the higher prevalence of major depressive disorder in women than in men (Kessler, 2003). To eliminate possible confounding effects from these risk factors, we included them as covariates into our multivariate linear models in the differential expression analysis.
To investigate whether past CVD or MD left “transcriptomic scars” on any tissues or organs, we implemented the voom-limma (Law et al., 2014; Ritchie et al., 2015) pipeline to identify genes differentially expressed between the cohorts with and without a history of CVD or MD, using the linear model described in the Materials and Methods section.
Since the analyzing tissues were defined as non-diseased, we did not expect that there would be a significant number of DEGs identified. To our surprise, 17 out of 46 and 16 out of 45 tissues displayed significant differential expression (false discovery rate <0.05 and absolute fold change >1.5) in the CVD and MD analyses, respectively (Figures 1A,B). The top four tissues with the highest number of significant DEGs were included in the functional enrichment analysis (Adipose – Visceral, Artery – Tibial, Brain – Caudate, and Brain – Spinal cord for CVD), as well as the top three MD tissues (Brain – Hypothalamus, Brain – Putamen, and Brain – Spinal cord).
Figure 1. The number of significantly upregulated and downregulated genes (FDR < 0.05 and absolute log2 fold change > 1.5) identified in GTEx tissues in (A) cerebrovascular diseases and (B) major depression analyses.
There was no common DEG shared by the four CVD or the three MD tissues (Figures 2A,B). A large number of significant DEGs identified by our CVD model (Supplementary Table 1) were associated with inflammation. For instance, the most significantly upregulated gene in the spinal cord, CHI3L1, is related to a variety of inflammatory disorders (Kastrup et al., 2009; Johansen et al., 2010; Im et al., 2020) and coronary artery disease (Rathcke and Vestergaard, 2009). Gene FCGR3A—upregulated in three of the CVD tissues (Brain – Spinal cord adj.P.val = 0.02; Brain – Caudate adj.P.val = 0.03; Artery – Tibial adj.P.val = 0.02)—encodes a receptor that binds the Fc portion of IgG, and it affects the pharmacokinetics in patients with Crohn’s Disease (Termant et al., 2015). LPAR5, which was overexpressed in both the brain caudate and the spinal cord of the subjects with a history of CVD, has been reported to be activated during nerve injury (Santos-Nogueira et al., 2015), and it transmits pro-inflammatory signals (Plastira et al., 2016). DLG2, which was downregulated in hypothalamus tissues with MD (Supplementary Table 2), has been reported to be associated with interferon production (Ali et al., 2018). These results suggest that the systematic effects left by CVD and MD can still be identified in several post-mortem human tissues on the transcriptomic level; among these tissues, top DEGs were reported to have a link with inflammation, indicating that inflammation may play a role.
Figure 2. Overlaps of significantly differentially expressed genes. (A) CVD-associated tissues: Adipose – Visceral, Artery – Tibial, Brain – Caudate, and Brain – Spinal cord. (B) MD-associated tissues: Brain – Hypothalamus, Brain – Putamen, and Brain – Spinal cord, there is no overlap between these three.
A functional overview can be gained through gene set enrichment analysis. CVD DEGs from the Adipose – Visceral, Artery – Tibial, Brain – Caudate, and Brain – Spinal cord; and MD DEGs from the Brain – Hypothalamus, Brain – Putamen, and Brain – Spinal cord were further analyzed using the Gene Set Enrichment Analysis method (Subramanian et al., 2005). A broad spectrum of Gene Ontology (GO) terms, with the top significantly enriched GO terms in the CVD spinal cord, is presented as an example in Figure 3.
Figure 3. Cytoscape network image for GO terms significantly enriched in DEGs from the spinal cord in the CVD analysis. Similar GO terms (inner circles) were combined into groups (outer circles) by AutoAnnotate, summarizing labels generated from the app were further improved manually. Circles in blue (left) are GO terms enriched for downregulated DEGs, those in red (right) are GO terms enriched for upregulated DEGs (significant GO term’s cutoff: q-value < 0.1).
Strikingly, upregulated genes in all four CVD tissues were significantly enriched in immunological events, for instance antigen binding, T cell proliferation, the interferon-gamma-mediated signaling pathway (Figure 3, Table 1), although the visceral adipose had only one significantly upregulated gene. This is consistent with a large body of evidence showing that inflammation plays a crucial role in cerebrovascular diseases. Inflammation can rupture the intracranial aneurysm wall (Tulamo et al., 2010), lead to secondary injury after an ischemic stroke (Ahmad and Graham, 2010), and impact the progression of symptomatic intracranial atherosclerosis (Arenillas et al., 2008). Inflammation has also been linked to blood–brain barrier dysfunction (de Vries et al., 2012) and tissue injury (Jin et al., 2010) in cerebrovascular diseases.
Table 1. Top three Gene Ontology (GO) terms with the highest normalized enrichment scores in tissues with many immunological terms.
For the MD tissues, inflammatory events only mainly enriched in downregulated genes only in the spinal cord (Table 1) and a few in upregulated genes in the hypothalamus (Supplementary Data Sheet 2). Likewise, depression has been associated with increased inflammatory activation in both the periphery and the central nervous system. Many antidepressant agents reduce inflammatory activation in immune cells and lower circulating inflammatory cytokine levels, supporting this association (Lee and Giuliani, 2019). Furthermore, it is worth mentioning that mitochondrial events and cellular respiration were significantly upregulated in the putamen. Since mitochondrial energy metabolism in the putamen has been reported to be highly correlated with emotional and intellectual impairment in Schizophrenics (Prince et al., 2000), it might also have some hidden links with depression as well. Another interesting result is that hypothalamus upregulated genes were mapped to terms related to cilia (Supplementary Data Sheet 1). There is still no obvious evidence connecting cilia with depression so far, but it is an underexplored area worth investigating (Pruski and Lang, 2019).
We further explored our differential expression results using Disease Ontology (Schriml et al., 2019) and Human Phenotype Ontology (Köhler et al., 2019) annotations. Top enriched diseases and human phenotypes are similar to the biological phenotypes found by our GO analysis (Supplementary Data Sheet 1). Immune responses and cerebrovascular lesions were significantly enriched in the upregulated genes of the CVD tissues. For example, six Disease Ontology terms—human immunodeficiency virus infectious diseases, temporal arteritis, alopecia areata, autoimmune thrombocytopenic purpura, intracranial aneurysms, and primary immunodeficiency diseases—were shared by all four CVD tissues’ overexpressed genes (Supplementary Figure 5), and they are all diseases associated with inflammation and cerebrovascular accidents.
Our enrichment results reinforce the strong evidence linking inflammation to CVD, as well as other interesting biological phenomena that probably have associations with CVD and MD in the analyzing tissues.
We then enquired whether the “transcriptomic scars” were associated with the subject’s genotype. TWAS is a powerful approach to prioritize target genes by combining genetic variants identified in GWAS with transcriptome data, and can help shed light on possible associations between genetic loci and human complex diseases. Here, we carried out TWAS with S-PrediXcan (Barbeira et al., 2018) on four public GWAS summary statistics datasets, in which human circulating levels of C-reactive protein (CRP) (Han et al., 2020), monocyte chemotactic protein-1 (MCP1), interleukin-6 (IL-6), and interferon-gamma (Ahola-Olli et al., 2017) were evaluated (Table 2). C-reactive protein is known as a systemic biomarker of inflammation and has been shown to be a CVD risk biomarker (Di Napoli et al., 2011) and to increase in patients with MD (Miller and Raison, 2016). Cytokines MCP1, IL-6 and interferon gamma have been reported to have a great probability of contributing to both CVD (Moreno et al., 2008; Seifert et al., 2014; Georgakis et al., 2019) and MD (Eyre et al., 2016; Franscina Pinto and Andrade, 2016; Hodes et al., 2016). Our aim was to find any overlaps between gene–tissue pairs identified from TWAS and from our DE analyses amongst the tissues with expression variance (including Adipose – Visceral, Artery – Tibial, Brain – Caudate, Brain – Hypothalamus, Brain – Putamen, and Brain – Spinal cord), plus whole blood, since circulating cytokine levels were measured in serum or plasma samples. There were 1,575 and 25 significant gene–tissue pairs passing the adjusted P-value threshold (P-value/number of genes) found in CRP and MCP1 data, respectively, regardless of tissue types (Supplementary Table 3). There were eight overlapping gene–tissue pairs (Table 3), amongst which PPP1R18, RP11-238F2.1, FRK had the same direction of variations but others had the opposite direction. All seven protein-coding genes were more or less related to immune responses. Specifically, it was demonstrated that complement gene C2 was expressed in human post-mortem brain-derived cerebrovascular smooth muscle cells and may amplify the pro-inflammatory effects in brain vessels (Walker et al., 2008). The major histocompatibility complex class I chain-related gene A MICA is a highly polymorphic gene that encodes protein variants functioning in immune activation and surveillance; our results therefore indicate that there may be a link between MICA and depression.
Most CVD and MD transcriptome analyses (Grond-Ginsbach et al., 2008; Pantazatos et al., 2017; Kim et al., 2018; Cai et al., 2019; Zhu et al., 2019; Androvic et al., 2020; Wittenberg et al., 2020) are restricted to mouse models or a limited sample size of human expression data. In this study, we systematically analyzed expression data for over 16,000 non-diseased human samples across multiple tissues from GTEx, investigating global transcriptomic alterations on the human body in cases with a history of CVD or MD. We first built a linear mixed model and applied it to the expression data. Dozens to hundreds of differentially expressed genes were identified in the visceral adipose, tibial artery, caudate, and spinal cord for CVD, and in the hypothalamus, putamen, and spinal cord for MD. Furthermore, functional enrichment analysis showed that a large number of annotations pertaining to inflammatory responses were enriched in upregulated CVD DEGs from all four tissues, and that MD DEGs were mostly associated with neurological and metabolic events. Our results suggest that the long-term sequelae of cerebrovascular accidents and depressive symptoms can still be reflected in post-mortem samples, and that inflammation may be maintained for a period of time after CVD onset.
A growing body of evidence indicates that inflammation not only contributes to the initiation and development of CVD (Lakhan et al., 2009; Gu et al., 2019), it also persists globally in the brain for the long-term after CVD (Shi et al., 2019). Neuroinflammation followed by cerebrovascular accidents may promote recovery and further injury, playing both beneficial and detrimental roles (Jayaraj et al., 2019). A large-scale GWAS discovered one genetic variant (rs1842681) in the gene LOC105372028 associated with post-stroke outcomes (Söderholm et al., 2019). Furthermore, proteomic studies of post-stroke depression (PSD) reveal that immune dysfunction in stroke survivors is associated with PSD (Zhan et al., 2014; Nguyen et al., 2016). The connection between inflammation and depression is undeniable (Dantzer et al., 2008; Miller and Raison, 2016). Unlike the CVD results, only a small portion of the MD DEGs were enriched in immune responses, while the top ones are mostly non-coding RNAs (Supplementary Table 2). This is reasonable since MD is highly heterogeneous (Goldberg, 2011) and not all individuals exposed to inflammatory challenges develop depression. Still, inflammatory responses that occur before and after cerebrovascular accidents or depression are very complicated, and the underlying mechanism is yet to be elucidated.
Our study also provides evidence of the general and potential long-term effects left by cerebrovascular events and depression from the transcriptomic aspect. A great number of DEGs were identified in some of the tissues, especially the brain, indicating that gene expression levels were alternated since CVD/MD onset. Non-brain tissues with significant CVD DEGs are related to vascular diseases and may pose risks to CVD. To be more specific, adipose tissue and its secreted inflammatory proteins contributed to obesity-associated vasculopathy and cardiovascular risk (Berg and Scherer, 2005), and they may contribute to CVD as well. Peripheral arterial disease occurring in the tibial artery shared similar risk factors with CVD (Banerjee et al., 2010). Moreover, DEGs such as CHI3L1 and LPAR5 may reveal possible mechanisms for post-CVD outcomes, but further experiments are necessary for validation. Interestingly, the hypothalamus had the highest number of MD DEGs, which is compatible with one of the most enduring and replicated findings in psychiatry — the activation of the hypothalamic-pituitary-adrenal axis in a subset of MD patients. The identified DEGs may play a role in the neuroendocrine function of the hypothalamus. The pathways enriched in putamen positive DEGs were mainly about mitochondrial functions and the electric transport chain, which replicates previous results (Sacchet et al., 2017) and provides new insights into the effects of depression. Apart from these, other tissues have a relatively small number of DEGs reported. The reasons could be (1) small sample sizes of tissues that limit the statistical power to detect any differences; (2) tissue specificity, in other words, the studied diseases (CVD and MD) may raise only a modest effect on the gene expression of those tissues. In addition, the effect may get compensated gradually or last not long enough to be detectable at the end of life. Further studies are needed to elucidate these points.
Only a few DEGs identified by our linear model overlapped with genes prioritized by TWAS for selected cytokine phenotypes. This was expected and is probably due to the small fraction of genetic risk factors shared by complex diseases and these circulating cytokine levels. Additionally, only about 11 percent of heritability was explained by bulk tissue expression quantitative trait loci, according to this study (Yao et al., 2020). Therefore, possible long-term transcriptomic alterations across tissues and organs are probably caused by external factors such as lifestyle and the social and physical environment. Nevertheless, we used bulk RNA-seq for our analyses, and further utilizing techniques with higher resolution, such as single-cell sequencing and cell-type decomposition from bulk sequencing, could reveal more precise signals on specific cell types.
This study reveals molecular signatures of chronic effects and damage on multiple tissues potentially contributed by two types of complex diseases (CVD and MD) and associated factors. These signatures may be linked to inflammation and other disease-related pathways. Together, these results indicate that suffering from a complex disease can cause a tissue-wide impact on the transcriptomes, and they also suggest that treatment to attenuate inflammation may improve the body’s health in patients recovering from CVD. Our study not only provides insights into these disease mechanisms but also offers a possible route to studying the long-lasting changes caused by chronic diseases on multiple tissues or organs.
Multi-tissue RNA-seq data were collected from the GTEx project (Aguet et al., 2020) v8 release (dbGaP: phs000424.v8.p2). The genes and samples were filtered and quantile-normalized in a tissue-aware manner, as described in the Yet Another RNA Normalization (YARN) pipeline paper (Paulson et al., 2017).
Subjects with an explicitly reported medical history of cerebrovascular disease (MHCVD, phv00169142.v8.p2) or major depression (MHDPRSSN, phv00169145.v8.p2) were considered in this study. We removed subjects with missing values in their Hardy scale (DTHHRDY), ischemic time (SMTSISCH), or batch ID (SMNABTCH). All cell lines and tissues with less than 12 samples with a history of CVD or less than 10 samples with a history of MD were excluded from our analyses. Finally, we used a total of 16,412 human post-mortem samples (1,498 with and 14,914 without a history of CVD), covering 46 tissues from 928 subjects (99 with and 829 without a history of CVD) in the CVD analysis (Supplementary Figure 1); and a total of 16,221 samples (1,602 with and 14,619 without a history of depression) across 45 tissues, including at least 10 samples with a history of MD, from 926 subjects (91 with and 835 without a history of MD) (Supplementary Figure 2).
Differential expression analysis between the samples with and without a history of CVD/MD was conducted using the voom-limma pipeline (Law et al., 2014; Ritchie et al., 2015). Briefly, RNA-seq read counts were transformed to log counts per million (log-cpm) with associated precision weights to stabilize the variance in the data using the voom function, followed by linear model fitting and the empirical Bayes procedure. According to the paper (Somekh et al., 2019), the multivariate linear regression model that adjusted for known confounders outperforms other methods correcting for hidden confounders, which may remove some of the desired biological signals. Hence, we adopted the linear regression model but replaced the experimental batch (SMGEBTCH) with another batch information (SMNABTCH). This model fits for gender (GENDER), the interval between the time of the donor’s death and the sample collection (SMTSISCH), age (AGE), the type of nucleic acid isolation batch (SMNABTCH), the type of death (DTHHRDY), as well as the variables of our interest—the medical history of CVD (MHCVD) and MD (MHDPRSSN)—for the gene expression data (Y):
Y ∼β1GENDER + β2SMTSISCH + β3AGE + β4SMNABTCH + β5DTHHRDY + β6MHCVD + ε
Y ∼β1GENDER + β2SMTSISCH + β3AGE + β4SMNABTCH + β5DTHHRDY + β6MHDPRSSN + ε
The GENDER term was removed from sex-specific tissues, and the SMNABTCH term was removed from tissues in only one batch. P-values from the regression model were adjusted for multiple testing using the Benjamini-Hochberg method (Benjamini and Hochberg, 1995).
Pre-ranked Gene Set Enrichment Analysis (GSEA) (Subramanian et al., 2005) was conducted with gene lists ranked by the t-statistics from the results of our DE analyses, with default program parameters and a default background set on GSEA v4.0.1. The Gene Matrix Transposed (GMT) files of Gene Ontology were obtained from the Molecular Signatures Database v7.1. Disease Ontology (Schriml et al., 2019) data were downloaded from the Alliance of Genome Resources1. Human Phenotype Ontology (Köhler et al., 2019) annotations were acquired from the website2. The Cytoscape (Shannon et al., 2003) figure (Figure 3) was generated with the AutoAnnotate application (Kucera et al., 2016), and auto-generated summarizing labels were further improved manually.
GWAS summary statistics datasets were downloaded from the NHGRI-EBI GWAS Catalog (Buniello et al., 2019) for study GCST009777 (Han et al., 2020) and study GCST004421 (Ahola-Olli et al., 2017) on 19/10/2020. These GWAS datasets examined biomarkers of inflammatory responses, and they were obtained from Caucasian subjects (Table 2). Gene expression variation was inferred using S-PrediXcan (Barbeira et al., 2018) with GTEx v8 elastic-net prediction models3 for the four tissues with expression variation between CVD and non-CVD cohorts: Adipose – Visceral, Artery – Tibial, Brain – Caudate, and Brain – Spinal cord; and the three tissues with expression variation between MD and non-MD cohorts: Brain – Hypothalamus, Brain – Putamen, and Brain – Spinal cord. We ran S-PrediXcan on these tissues one by one in each phenotype. Tissue–gene pairs with P-value < 0.05/(number of tested genes) were considered as significant.
This project is under the approval of access request #84958 for the dataset General Research Use in Genotype-Tissue Expression (dbGaP: phs000424). The GTEx data were downloaded from dbGaP.
GitHub repository: https://github.com/cyclab/GTEx-Complex-Diseases.
GTEx open-access data can be found on the GTEx Portal (https://gtexportal.org/home/datasets). GTEx protected data are available via dbGaP (accession phs000424.v8).
This study involving reanalysis of the GTEx data was reviewed and approved by NCBI dbGaP (Project #22839).
C-LP and C-YC designed the study, wrote, and reviewed the manuscript. C-LP analyzed the data and drafted the manuscript. C-YC acquired the funding. Both contributed to the article and approved the submitted version.
This work was financially supported by the Young Scholar Fellowship Program by the Ministry of Science and Technology (MOST) in Taiwan, under Grant MOST 110-2636-E-010-001, MOST109-2636-E-010-003, and MOST110-2811-B-010-507. This work was also supported by the Sustainable Translational Research Orchestrated Knowledge Ecosystem (STROKE) and by the Brain Research Center, National Yang Ming Chiao Tung University, from The Featured Areas Research Center Program within the framework of the Higher Education Sprout Project by the Ministry of Education (MOE) in Taiwan.
The authors declare that the research was conducted in the absence of any commercial or financial relationships that could be construed as a potential conflict of interest.
We thank Yi-Sian Lin, Pin-Jou Wu, Yao-Chung Chen, and Hao-Jen Deng for their valuable discussions. We thank the National Center for High-performance Computing (NCHC) for providing computational and storage resources.
The Supplementary Material for this article can be found online at: https://www.frontiersin.org/articles/10.3389/fgene.2021.696836/full#supplementary-material
Supplementary Figure 1 | The number of samples with (MHCVD = 1) and without (MHCVD = 0) cerebrovascular disease history in each selected GTEx tissue.
Supplementary Figure 2 | The number of samples with (MHDPRSSN = 1) and without (MHDPRSSN = 0) major depression history in each selected GTEx tissue.
Supplementary Figure 3 | The distribution of age, BMI, sex, race in cohorts with (MHCVD = 1) and without (MHCVD = 0) cerebrovascular disease history.
Supplementary Figure 4 | The distribution of age, BMI, sex, race in cohorts with (MHDPRSSN = 1) and without (MHDPRSSN = 0) major depression history.
Supplementary Figure 5 | Venn diagram of (A) significant Disease Ontology (DO) terms enriched by upregulated DEGs, (B) significant DO terms enriched by downregulated DEGs, (C) significant Human Phenotype Ontology (HPO) terms enriched by upregulated DEGs, (D) significant HPO terms enriched by downregulated DEGs in four CVD tissues. The threshold is FDR.q.val < 0.05.
Supplementary Table 1 | Significantly differentially expressed genes in CVD analysis.
Supplementary Table 2 | Significantly differentially expressed genes in MD analysis. All limma-voom differential expression results, including limma reports, MA plots, and volcano plots, can be found at: https://doi.org/10.5281/zenodo.5075921.
Supplementary Table 3 | Significant TWAS associations.
Supplementary Data Sheet 1 | All GSEA results.
Supplementary Data Sheet 2 | All TWAS results.
Aguet, F., Barbeira, A. N., Bonazzola, R., Brown, A., Castel, S. E., Jo, B., et al. (2020). The GTEx consortium atlas of genetic regulatory effects across human tissues. Science 369, 1318–1330. doi: 10.1126/SCIENCE.AAZ1776
Ahmad, M., and Graham, S. H. (2010). Inflammation after stroke: mechanisms and therapeutic approaches. Transl. Stroke Res. 1, 74–84. doi: 10.1007/s12975-010-0023-7
Ahola-Olli, A. V., Würtz, P., Havulinna, A. S., Aalto, K., Pitkänen, N., Lehtimäki, T., et al. (2017). Genome-wide association study identifies 27 loci influencing concentrations of circulating cytokines and growth factors. Am. J. Hum. Genet. 100, 40–50. doi: 10.1016/j.ajhg.2016.11.007
Ali, S., Hoven, A., Dress, R. J., Schaal, H., Alferink, J., and Scheu, S. (2018). Identification of a novel Dlg2 isoform differentially expressed in IFNβ-producing plasmacytoid dendritic cells. BMC Genomics 19:194. doi: 10.1186/s12864-018-4573-5
Androvic, P., Kirdajova, D., Tureckova, J., Zucha, D., Rohlova, E., Abaffy, P., et al. (2020). Decoding the transcriptional response to ischemic stroke in young and aged mouse brain. Cell Rep. 31:107777. doi: 10.1016/j.celrep.2020.107777
Anrather, J., and Iadecola, C. (2016). Inflammation and stroke: an overview. Neurotherapeutics 13, 661–670. doi: 10.1007/s13311-016-0483-x
Arenillas, J. F., Álvarez-Sabín, J., Molina, C. A., Chacón, P., Fernández-Cadenas, I., Ribó, M., et al. (2008). Progression of symptomatic intracranial large artery atherosclerosis is associated with a proinflammatory state and impaired fibrinolysis. Stroke 39, 1456–1463. doi: 10.1161/STROKEAHA.107.498600
Banerjee, A., Fowkes, F. G., and Rothwell, P. M. (2010). Associations between peripheral artery disease and ischemic stroke: implications for primary and secondary prevention. Stroke 41, 2102–2107. doi: 10.1161/STROKEAHA.110.582627
Barbeira, A. N., Dickinson, S. P., Bonazzola, R., Zheng, J., Wheeler, H. E., Torres, J. M., et al. (2018). Exploring the phenotypic consequences of tissue specific gene expression variation inferred from GWAS summary statistics. Nat. Commun. 9:1825. doi: 10.1038/s41467-018-03621-1
Benjamini, Y., and Hochberg, Y. (1995). Controlling the False discovery rate: a practical and powerful approach to multiple testing. J. R. Stat. Soc. Ser. B 57, 289–300. doi: 10.1111/j.2517-6161.1995.tb02031.x
Berg, A. H., and Scherer, P. E. (2005). Adipose tissue, inflammation, and cardiovascular disease. Circ. Res. 96, 939–949. doi: 10.1161/01.RES.0000163635.62927.34
Boehme, A. K., Esenwa, C., and Elkind, M. S. V. (2017). Stroke risk factors, genetics, and prevention. Circ. Res. 120, 472–495. doi: 10.1161/CIRCRESAHA.116.308398
Buniello, A., Macarthur, J. A. L., Cerezo, M., Harris, L. W., Hayhurst, J., Malangone, C., et al. (2019). The NHGRI-EBI GWAS catalog of published genome-wide association studies, targeted arrays and summary statistics 2019. Nucleic Acids Res. 47, D1005–D1012. doi: 10.1093/nar/gky1120
Cai, Y., Zhang, Y., Ke, X., Guo, Y., Yao, C., Tang, N., et al. (2019). Transcriptome sequencing unravels potential biomarkers at different stages of cerebral ischemic stroke. Front. Genet. 10:814. doi: 10.3389/fgene.2019.00814
Coussens, L. M., and Werb, Z. (2002). Inflammation and cancer. Nature 420, 860–867. doi: 10.1038/nature01322
Dantzer, R., O’Connor, J. C., Freund, G. G., Johnson, R. W., and Kelley, K. W. (2008). From inflammation to sickness and depression: when the immune system subjugates the brain. Nat. Rev. Neurosci. 9, 46–56. doi: 10.1038/nrn2297
de Vries, H. E., Kooij, G., Frenkel, D., Georgopoulos, S., Monsonego, A., and Janigro, D. (2012). Inflammatory events at blood-brain barrier in neuroinflammatory and neurodegenerative disorders: implications for clinical disease. Epilepsia 53(Suppl. 6), 45–52. doi: 10.1111/j.1528-1167.2012.03702.x
Demyttenaere, K., Bruffaerts, R., Posada-Villa, J., Gasquet, I., Kovess, V., Lepine, J. P., et al. (2004). Prevalence, severity, and unmet need for treatment of mental disorders in the World Health Organization world mental health surveys. J. Am. Med. Assoc. 291, 2581–2590. doi: 10.1001/jama.291.21.2581
Di Napoli, M., Elkind, M. S., Godoy, D. A., Singh, P., Papa, F., and Popa-Wagner, A. (2011). Role of C-reactive protein in cerebrovascular disease: a critical review. Expert Rev. Cardiovasc. Ther. 9, 1565–1584. doi: 10.1586/erc.11.159
Dichgans, M., Pulit, S. L., and Rosand, J. (2019). Stroke genetics: discovery, biology, and clinical applications. Lancet Neurol. 18, 587–599. doi: 10.1016/S1474-4422(19)30043-2
Eyre, H. A., Air, T., Pradhan, A., Johnston, J., Lavretsky, H., Stuart, M. J., et al. (2016). A meta-analysis of chemokines in major depression. Prog. NeuroPsychopharmacol. Biol. Psychiatry 68, 1–8. doi: 10.1016/j.pnpbp.2016.02.006
Flint, J., and Kendler, K. S. (2014). The genetics of major depression. Neuron 81, 484–503. doi: 10.1016/j.neuron.2014.01.027
Franscina Pinto, E., and Andrade, C. (2016). Interferon-related depression: a primer on mechanisms, treatment, and prevention of a common clinical problem. Curr. Neuropharmacol. 14, 743–748. doi: 10.2174/1570159x14666160106155129
Furman, D., Campisi, J., Verdin, E., Carrera-Bastos, P., Targ, S., Franceschi, C., et al. (2019). Chronic inflammation in the etiology of disease across the life span. Nat. Med. 25, 1822–1832. doi: 10.1038/s41591-019-0675-0
Georgakis, M. K., Malik, R., Björkbacka, H., Pana, T. A., Demissie, S., Ayers, C., et al. (2019). Circulating monocyte chemoattractant protein-1 and risk of stroke meta-analysis of population-based studies involving 17180 individuals. Circ. Res. 125, 773–782. doi: 10.1161/CIRCRESAHA.119.315380
Goldberg, D. (2011). The heterogeneity of “major depression.”. World Psychiatry 10, 226–228. doi: 10.1002/j.2051-5545.2011.tb00061.x
Goldstein, L. B., and Lichtman, J. H. (2013). “Epidemiology of cerebrovascular disease,” in Vascular Medicine: A Companion to Braunwald’s Heart Disease, Second Edn, eds M. Creager, J. Beckman, and J. Loscalzo (Amsterdam: Elsevier), 349–360. doi: 10.1016/B978-1-4377-2930-6.00029-X
Grond-Ginsbach, C., Hummel, M., Wiest, T., Horstmann, S., Pfleger, K., Hergenhahn, M., et al. (2008). Gene expression in human peripheral blood mononuclear cells upon acute ischemic stroke. J. Neurol. 255, 723–731. doi: 10.1007/s00415-008-0784-z
Gu, Y., Gutierrez, J., Meier, I. B., Guzman, V. A., Manly, J. J., Schupf, N., et al. (2019). Circulating inflammatory biomarkers are related to cerebrovascular disease in older adults. Neurol. Neuroimmunol. NeuroInflammation 6:e521. doi: 10.1212/NXI.0000000000000521
Han, X., Ong, J. S., An, J., Hewitt, A. W., Gharahkhani, P., and MacGregor, S. (2020). Using Mendelian randomization to evaluate the causal relationship between serum C-reactive protein levels and age-related macular degeneration. Eur. J. Epidemiol. 35, 139–146. doi: 10.1007/s10654-019-00598-z
Hodes, G. E., Ménard, C., and Russo, S. J. (2016). Integrating Interleukin-6 into depression diagnosis and treatment. Neurobiol. Stress 4, 15–22. doi: 10.1016/j.ynstr.2016.03.003
Howard, D. M., Adams, M. J., Clarke, T. K., Hafferty, J. D., Gibson, J., Shirali, M., et al. (2019). Genome-wide meta-analysis of depression identifies 102 independent variants and highlights the importance of the prefrontal brain regions. Nat. Neurosci. 22, 343–352. doi: 10.1038/s41593-018-0326-7
Im, J. H., Yeo, I. J., Park, P. H., Choi, D. Y., Han, S. B., Yun, J., et al. (2020). Deletion of chitinase-3-like 1 accelerates stroke development through enhancement of Neuroinflammation by STAT6-dependent M2 microglial inactivation in Chitinase-3-like 1 knockout mice. Exp. Neurol. 323:113082. doi: 10.1016/j.expneurol.2019.113082
Jayaraj, R. L., Azimullah, S., Beiram, R., Jalal, F. Y., and Rosenberg, G. A. (2019). Neuroinflammation: friend and foe for ischemic stroke. J. Neuroinflamm. 16:142. doi: 10.1186/s12974-019-1516-2
Jin, R., Yang, G., and Li, G. (2010). Inflammatory mechanisms in ischemic stroke: role of inflammatory cells. J. Leukoc. Biol. 87, 779–789. doi: 10.1189/jlb.1109766
Johansen, J. S., Bojesen, S. E., Tybjærg-Hansen, A., Mylin, A. K., Price, P. A., and Nordestgaard, B. G. (2010). Plasma YKL-40 and total and disease-specific mortality in the general population. Clin. Chem. 56, 1580–1591. doi: 10.1373/clinchem.2010.146530
Kastrup, J., Johansen, J. S., Winkel, P., Hansen, J. F., Hildebrandt, P., Jensen, G. B., et al. (2009). High serum YKL-40 concentration is associated with cardiovascular and all-cause mortality in patients with stable coronary artery disease. Eur. Heart J. 30, 1066–1072. doi: 10.1093/eurheartj/ehp049
Keene, K. L., Hyacinth, H. I., Bis, J. C., Kittner, S. J., Mitchell, B. D., Cheng, Y. C., et al. (2020). Genome-wide association study meta-analysis of stroke in 22 000 individuals of african descent identifies novel associations with stroke. Stroke 51, 2454–2463. doi: 10.1161/STROKEAHA.120.029123
Kessler, R. C. (2003). Epidemiology of women and depression. J. Affect. Disord. 74, 5–13. doi: 10.1016/S0165-0327(02)00426-3
Kessler, R. C., Berglund, P., Demler, O., Jin, R., Koretz, D., Merikangas, K. R., et al. (2003). The epidemiology of major depressive disorder: results from the national comorbidity survey replication (NCS-R). J. Am. Med. Assoc. 289, 3095–3105. doi: 10.1001/jama.289.23.3095
Kim, J., Kang, S. W., Mallilankaraman, K., Baik, S. H., Lim, J. C., Balaganapathy, P., et al. (2018). Transcriptome analysis reveals intermittent fasting-induced genetic changes in ischemic stroke. Hum. Mol. Genet. 27, 1497–1513. doi: 10.1093/hmg/ddy057
Knight, J. A. (2011). Diseases and disorders associated with excess body weight. Ann. Clin. Lab. Sci. 41, 107–121.
Köhler, S., Carmody, L., Vasilevsky, N., Jacobsen, J. O. B., Danis, D., Gourdine, J. P., et al. (2019). Expansion of the Human Phenotype Ontology (HPO) knowledge base and resources. Nucleic Acids Res. 47, D1018–D1027. doi: 10.1093/nar/gky1105
Kucera, M., Isserlin, R., Arkhangorodsky, A., and Bader, G. D. (2016). AutoAnnotate: a cytoscape app for summarizing networks with semantic annotations [version 1; referees: 2 approved]. F1000Research 5:1717. doi: 10.12688/F1000RESEARCH.9090.1
Lakhan, S. E., Kirchgessner, A., and Hofer, M. (2009). Inflammatory mechanisms in ischemic stroke: therapeutic approaches. J. Transl. Med. 7:97. doi: 10.1186/1479-5876-7-97
Law, C. W., Chen, Y., Shi, W., and Smyth, G. K. (2014). Voom: precision weights unlock linear model analysis tools for RNA-seq read counts. Genome Biol. 15:R29. doi: 10.1186/gb-2014-15-2-r29
Lee, C. H., and Giuliani, F. (2019). The role of inflammation in depression and fatigue. Front. Immunol. 10:1696. doi: 10.3389/fimmu.2019.01696
Malik, R., Chauhan, G., Traylor, M., Sargurupremraj, M., Okada, Y., Mishra, A., et al. (2018). Multiancestry genome-wide association study of 520,000 subjects identifies 32 loci associated with stroke and stroke subtypes. Nat. Genet. 50, 524–537. doi: 10.1038/s41588-018-0058-3
Miller, A. H., and Raison, C. L. (2016). The role of inflammation in depression: from evolutionary imperative to modern treatment target. Nat. Rev. Immunol. 16, 22–34. doi: 10.1038/nri.2015.5
Moreno, V. P., Subirá, D., Meseguer, E., and Llamas, P. (2008). IL-6 as a biomarker of ischemic cerebrovascular disease. Biomark. Med. 2, 125–136. doi: 10.2217/17520363.2.2.125
Murray, C. J. L., and Lopez, A. D. (1997). Alternative projections of mortality and disability by cause 1990-2020: global burden of disease study. Lancet 349, 1498–1504. doi: 10.1016/S0140-6736(96)07492-2
Netea, M. G., Balkwill, F., Chonchol, M., Cominelli, F., Donath, M. Y., Giamarellos-Bourboulis, E. J., et al. (2017). A guiding map for inflammation. Nat. Immunol. 18, 826–831. doi: 10.1038/ni.3790
Nguyen, V. A., Carey, L. M., Giummarra, L., Faou, P., Cooke, I., Howells, D. W., et al. (2016). A pathway proteomic profile of ischemic stroke survivors reveals innate immune dysfunction in association with mild symptoms of depression - a pilot study. Front. Neurol. 7:85. doi: 10.3389/fneur.2016.00085
Niccoli, T., and Partridge, L. (2012). Ageing as a risk factor for disease. Curr. Biol. 22, R741–R752. doi: 10.1016/j.cub.2012.07.024
Ober, C., Loisel, D. A., and Gilad, Y. (2008). Sex-specific genetic architecture of human disease. Nat. Rev. Genet. 9, 911–922. doi: 10.1038/nrg2415
Ormel, J., Hartman, C. A., and Snieder, H. (2019). The genetics of depression: successful genome-wide association studies introduce new challenges. Transl. Psychiatry 9:114. doi: 10.1038/s41398-019-0450-5
Pantazatos, S. P., Huang, Y. Y., Rosoklija, G. B., Dwork, A. J., Arango, V., and Mann, J. J. (2017). Whole-transcriptome brain expression and exon-usage profiling in major depression and suicide: evidence for altered glial, endothelial and ATPase activity. Mol. Psychiatry 22, 760–773. doi: 10.1038/mp.2016.130
Paulson, J. N., Chen, C. Y., Lopes-Ramos, C. M., Kuijjer, M. L., Platig, J., Sonawane, A. R., et al. (2017). Tissue-aware RNA-Seq processing and normalization for heterogeneous and sparse data. BMC Bioinformatics 18:437. doi: 10.1186/s12859-017-1847-x
Plastira, I., Bernhart, E., Goeritzer, M., Reicher, H., Kumble, V. B., Kogelnik, N., et al. (2016). 1-Oleyl-lysophosphatidic acid (LPA) promotes polarization of BV-2 and primary murine microglia towards an M1-like phenotype. J. Neuroinflamm. 13:205. doi: 10.1186/s12974-016-0701-9
Prince, J. A., Harro, J., Blennow, K., Gottfries, C. G., and Oreland, L. (2000). Putamen mitochondrial energy metabolism is highly correlated to emotional and intellectual impairment in schizophrenics. Neuropsychopharmacology 22, 284–292. doi: 10.1016/S0893-133X(99)00111-6
Pruski, M., and Lang, B. (2019). Primary cilia–an underexplored topic in major mental illness. Front. Psychiatry 10:104. doi: 10.3389/fpsyt.2019.00104
Rathcke, C. N., and Vestergaard, H. (2009). YKL-40 - an emerging biomarker in cardiovascular disease and diabetes. Cardiovasc. Diabetol. 8:61. doi: 10.1186/1475-2840-8-61
Ritchie, M. E., Phipson, B., Wu, D., Hu, Y., Law, C. W., Shi, W., et al. (2015). Limma powers differential expression analyses for RNA-sequencing and microarray studies. Nucleic Acids Res. 43:e47. doi: 10.1093/nar/gkv007
Sacchet, M. D., Camacho, M. C., Livermore, E. E., Thomas, E. A. C., and Gotlib, I. H. (2017). Accelerated aging of the putamen in patients with major depressive disorder. J. Psychiatry Neurosci. 42, 164–171. doi: 10.1503/jpn.160010
Santos-Nogueira, E., López-Serrano, C., Hernández, J., Lago, N., Astudillo, A. M., Balsinde, J., et al. (2015). Activation of lysophosphatidic acid receptor type 1 contributes to pathophysiology of spinal cord injury. J. Neurosci. 35, 10224–10235. doi: 10.1523/JNEUROSCI.4703-14.2015
Schneiderman, N., Ironson, G., and Siegel, S. D. (2005). Stress and health: psychological, behavioral, and biological determinants. Annu. Rev. Clin. Psychol. 1, 607–628. doi: 10.1146/annurev.clinpsy.1.102803.144141
Schriml, L. M., Mitraka, E., Munro, J., Tauber, B., Schor, M., Nickle, L., et al. (2019). Human disease ontology 2018 update: classification, content and workflow expansion. Nucleic Acids Res. 47, D955–D962. doi: 10.1093/nar/gky1032
Sears, M. E., and Genuis, S. J. (2012). Environmental determinants of chronic disease and medical approaches: recognition, avoidance, supportive therapy, and detoxification. J. Environ. Public Health 2012:356798. doi: 10.1155/2012/356798
Seifert, H. A., Collier, L. A., Chapman, C. B., Benkovic, S. A., Willing, A. E., and Pennypacker, K. R. (2014). Pro-inflammatory interferon gamma signaling is directly associated with stroke induced neurodegeneration. J. Neuroimmune Pharmacol. 9, 679–689. doi: 10.1007/s11481-014-9560-2
Shannon, P., Markiel, A., Ozier, O., Baliga, N. S., Wang, J. T., Ramage, D., et al. (2003). Cytoscape: a software environment for integrated models of biomolecular interaction networks. Genome Res. 13, 2498–2504. doi: 10.1101/gr.1239303
Shi, K., Tian, D. C., Li, Z. G., Ducruet, A. F., Lawton, M. T., and Shi, F. D. (2019). Global brain inflammation in stroke. Lancet Neurol. 18, 1058–1066. doi: 10.1016/S1474-4422(19)30078-X
Söderholm, M., Pedersen, A., Lorentzen, E., Stanne, T. M., Bevan, S., Olsson, M., et al. (2019). Genome-wide association meta-analysis of functional outcome after ischemic stroke. Neurology 92, E1271–E1283. doi: 10.1212/WNL.0000000000007138
Somekh, J., Shen-Orr, S. S., and Kohane, I. S. (2019). Batch correction evaluation framework using a-priori gene-gene associations: applied to the GTEx dataset. BMC Bioinformatics 20:268. doi: 10.1186/s12859-019-2855-9
Subramanian, A., Tamayo, P., Mootha, V. K., Mukherjee, S., Ebert, B. L., Gillette, M. A., et al. (2005). Gene set enrichment analysis: a knowledge-based approach for interpreting genome-wide expression profiles. Proc. Natl. Acad. Sci. U.S.A. 102, 15545–15550. doi: 10.1073/pnas.0506580102
Termant, D., Berkane, Z., Picon, L., Gouilleux-Gruart, V., Colombel, J. F., Allez, M., et al. (2015). Assessment of the influence of inflammation and FCGR3A Genotype on infliximab pharmacokinetics and time to relapse in patients with crohn’s disease. Clin. Pharmacokinet. 54, 551–562. doi: 10.1007/s40262-014-0225-3
Tulamo, R., Frösen, J., Hernesniemi, J., and Niemelä, M. (2010). Inflammatory changes in the aneurysm wall: a review. J. Neurointerv. Surg. 2, 120–130. doi: 10.1136/jnis.2009.002055
Virani, S. S., Alonso, A., Benjamin, E. J., Bittencourt, M. S., Callaway, C. W., Carson, A. P., et al. (2020). Heart disease and stroke statistics—2020 update: a report from the american heart association. Circulation 141, e139–e596. doi: 10.1161/CIR.0000000000000757
Walker, D. G., Dalsing-Hernandez, J. E., and Lue, L. F. (2008). Human postmortem brain-derived cerebrovascular smooth muscle cells express all genes of the classical complement pathway: a potential mechanism for vascular damage in cerebral amyloid angiopathy and Alzheimer’s disease. Microvasc. Res. 75, 411–419. doi: 10.1016/j.mvr.2007.10.004
Willerson, J. T., and Ridker, P. M. (2004). Inflammation as a cardiovascular risk factor. Circulation 109, II-2–II-10. doi: 10.1161/01.cir.0000129535.04194.38
Wittenberg, G. M., Greene, J., Vértes, P. E., Drevets, W. C., and Bullmore, E. T. (2020). Major depressive disorder is associated with differential expression of innate immune and neutrophil-related gene networks in peripheral blood: a quantitative review of whole-genome transcriptional data from case-control studies. Biol. Psychiatry 88, 625–637. doi: 10.1016/j.biopsych.2020.05.006
Wray, N. R., Ripke, S., Mattheisen, M., Trzaskowski, M., Byrne, E. M., Abdellaoui, A., et al. (2018). Genome-wide association analyses identify 44 risk variants and refine the genetic architecture of major depression. Nat. Genet. 50, 668–681. doi: 10.1038/s41588-018-0090-3
Yao, D. W., O’Connor, L. J., Price, A. L., and Gusev, A. (2020). Quantifying genetic effects on disease mediated by assayed gene expression levels. Nat. Genet. 52, 626–633. doi: 10.1038/s41588-020-0625-2
Yuan, N., Chen, Y., Xia, Y., Dai, J., and Liu, C. (2019). Inflammation-related biomarkers in major psychiatric disorders: a cross-disorder assessment of reproducibility and specificity in 43 meta-analyses. Transl. Psychiatry 9:233. doi: 10.1038/s41398-019-0570-y
Zhan, Y., Yang, Y. T., You, H. M., Cao, D., Liu, C. Y., Zhou, C. J., et al. (2014). Plasma-based proteomics reveals lipid metabolic and immunoregulatory dysregulation in post-stroke depression. Eur. Psychiatry 29, 307–315. doi: 10.1016/j.eurpsy.2014.03.004
Zhu, Y., Armstrong, J. L., Tchkonia, T., and Kirkland, J. L. (2014). Cellular senescence and the senescent secretory phenotype in age-related chronic diseases. Curr. Opin. Clin. Nutr. Metab. Care 17, 324–328. doi: 10.1097/MCO.0000000000000065
Keywords: complex disease, cerebrovascular disease, major depression, inflammation, transcriptome, GTEx
Citation: Poon C-L and Chen C-Y (2021) Exploring the Impact of Cerebrovascular Disease and Major Depression on Non-diseased Human Tissue Transcriptomes. Front. Genet. 12:696836. doi: 10.3389/fgene.2021.696836
Received: 17 April 2021; Accepted: 21 June 2021;
Published: 19 July 2021.
Edited by:
Duc-Hau Le, Vingroup Big Data Institute, VietnamReviewed by:
Qingnan Liang, Baylor College of Medicine, United StatesCopyright © 2021 Poon and Chen. This is an open-access article distributed under the terms of the Creative Commons Attribution License (CC BY). The use, distribution or reproduction in other forums is permitted, provided the original author(s) and the copyright owner(s) are credited and that the original publication in this journal is cited, in accordance with accepted academic practice. No use, distribution or reproduction is permitted which does not comply with these terms.
*Correspondence: Cho-Yi Chen, Y2hveWljaGVuQG55Y3UuZWR1LnR3
Disclaimer: All claims expressed in this article are solely those of the authors and do not necessarily represent those of their affiliated organizations, or those of the publisher, the editors and the reviewers. Any product that may be evaluated in this article or claim that may be made by its manufacturer is not guaranteed or endorsed by the publisher.
Research integrity at Frontiers
Learn more about the work of our research integrity team to safeguard the quality of each article we publish.