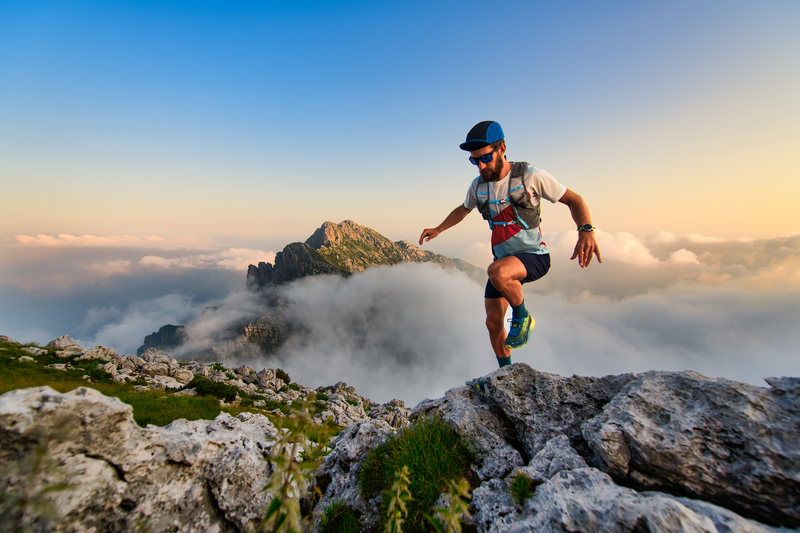
94% of researchers rate our articles as excellent or good
Learn more about the work of our research integrity team to safeguard the quality of each article we publish.
Find out more
ORIGINAL RESEARCH article
Front. Genet. , 02 July 2021
Sec. Genomics of Plants and the Phytoecosystem
Volume 12 - 2021 | https://doi.org/10.3389/fgene.2021.692702
This article is part of the Research Topic The Development and Application of Multi-Omics Integration Approaches to Dissecting Complex Traits in Plants View all 25 articles
Drought stress is a bottleneck factor for plant growth and development, especially in epiphytic orchids that absorb moisture mainly from the air. Recent studies have suggested that there are complex transcriptional regulatory networks related to drought stress in Dendrobium sinense. In this study, the transcription and metabolite alterations involved in drought stress response in D. sinense were investigated through RNA-seq and metabolomics. A total of 856 metabolites were identified from stressed and control samples, with 391 metabolites showing significant differences. With PacBio and Illumina RNA sequencing, 72,969 genes were obtained with a mean length of 2,486 bp, and 622 differentially expressed genes (DEGs) were identified. Correlation analysis showed 7 differential genes, and 39 differential metabolites were involved in interaction networks. The network analysis of differential genes and metabolites suggested that the pathways of purine metabolism and phenylpropanoid biosynthesis may play an important role in drought response in D. sinense. These results provide new insights and reference data for culturally important medicinal plants and the protection of endangered orchids.
Through evolutionary processes, a series of response mechanisms has developed in plants that allow for chemical, physiological, and developmental responses to changes in the external environment (Claeys and Inze, 2013). Drought is one abiotic factor that strongly affects plant growth and development (Singh and Laxmi, 2015; Zhu, 2016). Several studies have elucidated important genes involved in drought stress response, mainly by studying gene expression patterns of drought-stressed plants (Joshi et al., 2016). For example, drought resistance in Triticum aestivum can be improved by TaFBA overexpression that affects the accumulation of sucrose and starch (Zhou et al., 2014). Additionally, aldehyde dehydrogenases (ALDHs) have been considered as general detoxifying enzymes that eliminate abiotic stress in a variety of organisms. Transgenic Arabidopsis plants expressing CsALDH12A1 showed enhanced tolerance to drought stress during plant development (Duan et al., 2015). Under drought stress, the overexpressions of allene oxide synthase (AOS) and allene oxide cyclase (AOC) genes were positively correlated with the levels of major oxylipin metabolites in the AOS branch of the pathway, ultimately leading to the synthesis of jasmonates (Stenzel et al., 2003; Domenico et al., 2012). In CBL-interacting protein kinases (CIPKs) signaling, TaCIPK23 plays an important role in drought stress response in wheat (Cui et al., 2018).
Previous studies have reported that drought stress can induce many metabolites, such as carbohydrates and amino acids (Maruyama et al., 2009, 2014). Sugars serve not only as a solute to regulate cell osmotic pressure but also as primary messengers for signal transduction (Baena-González et al., 2007). Recent research in Arabidopsis indicated that glucose passes through hexokinase-dependent pathways and phytohormone response pathways, which has been proven to associate with participation in adversity (Sharma et al., 2019). OsTF1L overexpression in rice increased drought tolerance by regulating the gene expression involved in lignin biosynthesis (Bang et al., 2018). In addition, drought stress in rice seeds could induce the expression of OsNCED3, promoting the biosynthesis of abscisic acid (ABA) and inhibiting seed germination (Chen et al., 2019). Thus, abundant evidence shows that plants clearly regulate the accumulation of metabolites and gene transcription to respond to drought stress (Morimoto et al., 2017).
In recent years, Illumina sequencing has accelerated research of the transcriptome, which has made great achievements in functional gene verification (Du et al., 2016) and gene mining (Zhang et al., 2019). Although the short reads produced by this second-generation sequencing technology has high accuracy, the short-read length affects assembly and mapping accuracy in the absence of a reference genome, resulting in short transcript splicing and incomplete transcripts (Du and Sun, 2016). In contrast, third-generation long-read sequencing, such as PacBio, can generate full-length transcript sequences but has high error rates. Therefore, the depth of second-generation sequencing can complement the full-length transcript sequencing of third-generation sequencing, identifying subtypes with higher accuracy in transcriptome sequencing, especially for plants without reference genomes (Du and Sun, 2016).
Dendrobium sinense is an endangered epiphytic orchid plant with high medicinal value (Sun et al., 2014). It is well known that epiphytic orchids usually attach to smooth rocks and tree trunks that have low water retention capacity. Compared with terrestrial plants, epiphytes are subjected to moderate drought stress for most of the year (Zhang et al., 2016). As a consequence, moisture stress is the most important abiotic factor limiting the growth and development of epiphytic orchids (Zotz and Bader, 2009). However, few studies have combined the analysis of gene transcripts and metabolites to explore how epiphytic orchids will response to water deficit. In this present work, differential metabolites of D. sinense under drought stress were obtained by metabolome analysis. In addition to greatly increasing molecular resources devoted to D. sinense that lack genome sequence information, the combination of PacBio and Illumina sequencing illustrates gene expression patterns in drought stress. Combined with transcriptome and metabolome data, the importantly metabolic pathways related to drought response were identified in D. sinense. Our data provide new insights and reference data for improving drought resistance in these endangered orchids.
Wild D. sinense plants were collected at the national nature reserves of Bawangling, Changjiang County, Hainan Province, China. Individuals were cultured at room conditions [25°C, relative humidity (RH) ≥ 95%] for 2 months. Then, we simulated a range of drought conditions by adjusting relative air humidity (RH) in separate growing containers for 7 days. Based on our previous investigation (Yang, 2009), D. sinense was assigned to grow under conditions for group A (RH ≥ 95%), group B (45% ≤ RH ≤ 50%), or group C (RH ≤ 5%), which were named D. sinense A, B, and C (DSA, DSB, and DSC), respectively. After 7 days of growth in these conditions, leaves and pseudo-bulbs were collected for further metabolome and transcriptome studies, immediately frozen in liquid nitrogen and stored at –80°C.
For each treatment experiment, 100 mg sample was fully ground in liquid nitrogen for physiological test. The contents of catalase (CAT), peroxidase isoenzyme (POD), superoxide dismutase (SOD), and ascorbate peroxidase (APX) were determined using the respective Solarbio detection kits. The experimental data were analyzed by SPSS software.
DSA, DSB, and DSC samples were crushed using a mixer mill (MM 400, Retsch, Shanghai, China) with a zirconia bead for 1.5 min at 30 Hz. One hundred milligrams powder was dissolved in 1.0 ml 70% aqueous methanol and incubated overnight at 4°C. Following centrifugation (10,000g for 10 min), the extracts were absorbed by CNWBOND Carbon-GCB SPE Cartridge (ANPEL, Shanghai, China) and filtered by SCAA-104 of 0.22 μm pore size (ANPEL, Shanghai, China). The data of high-performance liquid chromatography (HPLC) was analyzed by Shim-pack UFLC SHIMADZU CBM30A system (Applied Biosystems 6500 QTRAP, Thermo Fisher Scientific, Waltham, United States).
The extracted samples were analyzed using a liquid chromatography electrospray ionization tandem mass spectrometry (LC-ESI-MS/MS) system (UPLC, Shim-pack UFLC SHIMADZU CBM30A system; MS/MS, Applied Biosystems 6500 QTRAP). The conditions for liquid phase analysis were as follows: chromatographic column, Waters ACQUITY UPLC HSS T3 C18 (1.8 μm, 2.1 mm × 100 mm); solvent system, water (0.04% acetic acid)/acetonitrile (0.04% acetic acid); gradient program, 95:5 V/V at 0 min, 5:95 V/V at 11.0 min, 5:95 V/V at 12.0 min, 95:5 V/V at 12.1 min, 95:5 V/V at 15.0 min; flowrate, 0.40 ml/min; temperature, 40°C; and injection volume, 2 μl. The effluent was alternatively connected to an ESI-triple quadrupole-linear ion trap (QTRAP)-MS.
The ESI source operation parameters were as follows: electrospray ionization, source temperature 500°C; ion spray voltage (IS), 5,500 V; curtain gas (CUR) at 25.0 psi; and the collision gas (CAD), high. Instrument tuning and mass calibration were performed with 10 and 100 μmol/L polypropylene glycol solutions in QQQ and LIT modes, respectively. QQQ scans were acquired as multiple reaction monitoring (MRM) experiments with collision gas (nitrogen) set to 5 psi. Declustering potential (DP) and collision energy (CE) for individual MRM transitions were done with further DP and CE optimization. A specific set of MRM transitions were monitored for each period according to the metabolites eluted within this period (Chen et al., 2013).
The software analyst 1.6.3 was used to process the mass spectrometry data, and the metabolites of the samples were qualitatively and quantitatively analyzed based on Met Ware metabolic database (Wuhan, China). Orthogonal partial least-squares discriminant analysis (OPLS-DA) with supervised mode was used to analyze the differences within and among experimental groups. The metabolic contents were normalized by R software1, through which an accumulation-mode clustering analysis [hierarchical clustering analysis (HCA)] of metabolites was performed to test for differences among samples (Chong and Xia, 2018). The pathway-based Kyoto Encyclopedia of Genes and Genomes (KEGG) database was used for the functional annotation of differential metabolites (Kanehisa and Goto, 2000).
Total RNA was extracted from mature non-aging leaves and pseudo-bulbs with the Quick RNA Isolation Kit (Waryong, Beijing, China). RNA quality and concentration of each sample were measured using agarose gel electrophoresis, Nanodrop 2000 (Thermo Fisher Scientific, Waltham, United States), and Agilent Bioanalyzer 2100 (Agilent Technologies, Santa Clara, United States). Subsequently, an Iso-Seq library was prepared using the Clontech SMARTer PCR cDNA Synthesis Kit and sequenced on a PacBio Sequel (PN 100-092-800-03).
SMRTlink 6.0 software was used to process raw sequence data, and circular consensus sequences (CCS) were obtained by subread BAM files. The CCS BAM files were then classified as full-length non-chimera (FLNC) or non-full length (nFL) according to 5′-primer, 3′-primer and poly-A. Consensus sequences were obtained by clustering full-length sequences with isoform-level clustering (ICE), and additional nucleotide errors in consensus reads were corrected using the Illumina RNA-seq data using the LoRDEC software (Salmela and Rivals, 2014). The consensus sequences were corrected with non-full-length and non-chimeric sequences, resulting in polished consensus sequences (Fu et al., 2012). Finally, any redundancy in corrected consensus reads was removed by CD-HIT to obtain transcripts for subsequent analyses and as reference sequences (Fu et al., 2012). The RSEM software was used to estimate gene expression levels for each sample by mapping the transcript sequences to obtain the read count of each transcript (Li and Dewey, 2011). Considering the sequence depth and gene length of fragments, the gene expression level was calculated with the fragments per kilobase million (FPKM) method.
DESeq-R package (1.10.1) was used to analyze the differential expression between two groups. The differentially expressed genes (DEGs) were selected by | log2 (fold change)| > 1 and false discovery rate (FDR) < 0.05 (Storey and Tibshirani, 2003). Gene annotations and gene ontology (GO) enrichment analyses were implemented with the Go seq-R package. GO terms with FDR ≤ 0.05 were considered significantly enriched by DEGs. The KEGG database was used as a resource for understanding high-level functions and utilities of the biological system2. The KOBAS (3.0) software was used to test the statistical enrichment of DEGs in KEGG pathways.
The Pearson coefficient between metabolome and transcriptome data were calculated using R (cor test) software. Correlations corresponding to R2 > 0.8 and FDR < 0.05 were selected. The correlated data were visualized using Cytoscape software and KEGG database. Differences between samples were tested for statistical significance using the Duncan MRT method. Statistical analysis was implemented by SPSS software (version 19.0).
In order to explore the physiological mechanism of D. sinense response to drought stress, three treated experiments were conducted with different RH gradients sustained for 7 days. The color of DSA leaves was healthy green, while DSB and DSC leaves showed a noticeably yellow color with the increase in stress degree (Supplementary Figure 1). There are protective enzymes to scavenging reactive oxygen species (ROS) in plants, and the high activity of the enzyme is closely related to the degree of stress. Catalase and POD activities displayed a significant gradual increase (p < 0.05) with the intensification of drought stress (Figure 1). Superoxide dismutase and APX protective enzyme activities were only significantly different (p < 0.05) between DSA and DSC (Figure 1). The results showed that the activity of protective enzymes in D. sinense was increased, and the scavenging ability of reactive oxygen species was enhanced after drought stress.
Figure 1. Effects of drought stress on physiological and biochemical parameters in pseudobulb of D. sinense. Data are means ± SE of three separate measurements. The significance of differences was analyzed using Duncan method.
A total of 856 metabolites were detected, belonging to 16 categories (Supplementary Table 1). Among them, the most abundant were flavonoids (240; 28.04%), organic acids and derivatives (106; 12.38%), and amino acids and derivatives (93; 10.86%). Differential abundance of metabolites indicated 391 differential metabolites related to drought stress (Figure 2), mainly including flavonoids (116, 29.67%), phenylpropanoids (38, 9.72%), amino acids and derivatives (37, 9.46%), and lipids (35, 8.95%) (Supplementary Table 1). Interestingly, upregulated metabolites were more common than downregulated metabolites under drought stress, as observed in the comparisons of DSA vs. DSB and DSA vs. DSC groups (Supplementary Figure 2). Additionally, when comparing DSA (control) to DSB (moderate drought stress), 32 of the 151 identified upregulated metabolites were increased by more than 2.5-fold with a variable importance in project (VIP) score > 1.5. Of these, acetosyringone, 4-methylcatechol, pyrocatechol, apigenin 6-C-hexosyl-8-C-hexosyl-O-hexoside, and parthenolide increased most dramatically by more than >2,000-fold change and VIP > 1.7 (Supplementary Table 2). DSA vs. DSC (extreme drought stress) also included 32 upregulated metabolites with more than 2.5-fold change and VIP > 1.5. Of these, c-hexosyl-apigenin c-pentoside, acetosyringone, magnolol, 4-methylcatechol, pyrocatechol, and catechol increased by more than 2,000-fold (Supplementary Table 2). Intriguingly, 13 of the same metabolites with significant differences were detected in DSA vs. DSB and DSA vs. DSC, such as, L-tryptamine, S-(5′-adenosy)-L-homocysteine, p-coumaric acid, and 4-methylcatechol (Supplementary Table 2).
To further study the response to drought stress, KEGG enrichment pathway analysis was performed (Supplementary Table 3). The differential metabolites in the significant enrichment pathway were analyzed by cluster analysis. Under moderate drought stress, organic acids and their derivatives and procyanidin metabolites were significantly enriched. Under more extreme drought stress (RH ≤ 5%), flavonoids and carbohydrates were significantly enriched (Figure 3 and Supplementary Table 3).
Figure 3. Metabolites on metabolic pathways with significantly different enrichment. (A) Cluster analysis of DSB vs. DSA differential metabolites. (B) Cluster analysis of DSC vs. DSA differential metabolites. The analysis of hierarchical clustering for differential metabolism calculated by complete linkage approach. These metabolites were derived from metabolic pathways (p < 0.05) in DSA vs. DSB and DSA vs. DSC. Red denotes metabolites with high content, and blue means low content. The agglomeration method to be used by hclust function.
To obtain a more reliable transcriptome library, different treatments and organs of D. sinense were prepared for third-generation sequencing. 18.41G polymerase read bases (504,082 polymerase read) were obtained on the PacBio Sequel platform. Additionally, 84.47G clean bases were obtained on an Illumina platform (Supplementary Table 4). A total of 72,797 unigenes with a mean length of 2,486 bp were obtained (Table 1). We found that 62,038 (85.02%) unigenes had been previously annotated at least in one database (Supplementary Figure 3). Through GO analysis, we categorized these unigenes into 56 pathways, with “metabolic process,” “cell,” and “catalytic activity” having high ranking in biological processes, cellular components, and molecular functions, respectively (Supplementary Figure 3). A total of 38,037 unigenes were divided into 25 groups using the KOG database. “General function prediction only” was the most significant category, follow by “Posttranslational modification protein turnover chaperones” and “Signal transduction mechanisms” (Supplementary Figure 3).
We compared transcript counts across treatments to identify DEGs. Three hundred seventy-four DEGs were identified in DSA vs. DSB (151 upregulated and 223 downregulated genes), 130 DEGs in DSA vs. DSC (53 upregulated and 77 downregulated genes), and 256 DEGs in DSB vs. DSC (129 upregulated and 127 downregulated genes) (Supplementary Figure 4). Statistically, a total of 622 DEGs were characterized in differently treated D. sinense (Supplementary Figure 4). Surprisingly, DEGs were not found to be shared among different comparison groups (Supplementary Figure 4). To further explore the expression patterns of DEGs in response to drought stress, 622 DEGs were grouped into six clusters by k-means (Figure 4). Compared with control (DSA), the DEGs from class 1 showed a positive response, while those of class 6 showed a negative response (Figure 4). In DSC, the class 2 DEGS were upregulated, and the class 4 DEGs were downregulated (Figure 4). On the other hand, in DSB, the class 3 DEGs were upregulated, but the class 5 DEGs were downregulated (Figure 4). In the GO analysis, the functions of class 1 were related to “carbohydrate derivative binding,” “adenyl nucleotide binding,” “ATPase activity,” and “nucleotide binding” (Supplementary Table 5). “Transcription factor activity,” “oxidoreductase activity,” and “pectin metabolic process” were identified from class 2 of DEGs (Supplementary Table 5).
Figure 4. Results of the k-means clustering of 622 DEGs identified by PacBio Iso-Seq based on their expression patterns.
Transcription factors (TFs) are involved in regulating the biosynthesis of secondary metabolites in plants. According to the plant transcription family database, 3,284 TFs were identified in our data and distributed across 89 families using iTAK software (Figure 5A). Importantly, 27 differentially expressed TFs, classified into 21 families, were identified (Figure 5B). When combined with the analysis of expression trends of DEGs, the TFs were distributed across different classes. Class 3 contained the most differentially expressed TFs (ARID4, BIM2, C3H50, RR22, MYB-like, BT1, and BPM3). Class 5 contained the second most differentially expressed TFs (IDD4, C3H17, FRS6, HMG-like, PIE1, and pyrH). Class 6 consisted of five differentially expressed TFs (BBRD, ABI5, GATA26, BLH1, and NLP3). The ABI5 TF is involved in ABA signal transduction through a negative regulatory relationship. Classes 1 and 4 have the same number of differentially expressed TFs: FRS3, ATXR7, and PIE1 from class 1 and FRS6, NAC017, and CIA2 from class 4. Additionally, the TFs of PRR95 and ALY3 were upregulated after extreme drought stress (RH ≤ 5%). These results suggested that TFs may regulate the metabolite biosynthesis pathway of D. sinense in response to drought stress.
Figure 5. TFs involved in response to drought stress. (A) Number of TFs in different families. (B) Heat map presenting the expression patterns of different TFs in response to drought treatments. Red denotes TFs with high expression levels, and blue means low expression. The color ranges from red to blue represents the log2 (FPKM + 0.01) value from large too small.
We then explored correlations between the DEG and metabolites by calculating the Pearson correlation coefficients between all DEGs and differential metabolites. One hundred twenty-one DEGs and 239 differential metabolites had a strong correlation (R2 > 0.8, FDR < 0.05) (Supplementary Table 6). We then screened for extremely significantly DEGs (p < 0.01), which resulted in a set of DEGs that were enriched for genes relating to redox process, metabolic process, and phospholipid biosynthesis process (Supplementary Table 7). Nine extremely significantly DEGs were involved in microtubule organizing center and nuclear cell components, and 24 extremely significantly DEGs related to molecular functions were involved in molecular processes such as 6-phosphofructokinase activity, methionine adenosyltransferase, and GTP binding (Supplementary Table 7).
We also constructed a coexpression network of 33 extremely significantly DEGs and 11 differential metabolites (Figure 6). The result showed that extremely significant DEGs may play an important role in the response of D. sinense to drought stress. Further analysis on the relationship between genes and metabolites found five metabolic pathways in the coexpression network, such as glycerophospholipid metabolism pathway (ko0564), arginine and proline metabolism pathway (ko0330), purine metabolism pathway (ko0230), tryptophan metabolism pathway (ko0380), and phenylpropanoid biosynthesis pathway (ko0940).
Figure 6. Coexpression network of DEGs and differentially expressed metabolites. These DEGs were correlated with metabolites (R > 0.8). The networks were visualized with the Cytoscape software. The purple denotes represent DEGs, and blue means differential metabolites.
By combining the correlation analysis of transcriptome and metabolome in D. sinense, we found that purine metabolism and phenylpropanol biosynthesis were the main metabolic pathways in response to drought stress. The analysis found 7 DEGs and 39 differential metabolites from the purine metabolic pathway, such as phosphatidylglycamine synthase (purL), adenylate kinase (AK), and ribonucleoside diphosphate reductase subunit M1 (RRM1). In addition, the expression of inosine-5′-monophosphate dehydrogenase (IMPDH) gene was upregulated specifically in DSB (the moderate drought conditions) (Figure 7). Compared with DSA, the metabolite accumulation of 3,4-dihydroxy-DL-phenylalanine in DSB and DSC was downregulated, and the contents of guanine, xanthine, and hypoxanthine were upregulated. For example, xanthines accumulated significantly in DSB (DSB > DSA > DSC). Therefore, the biomass accumulation of xanthine, hypoxanthine, and guanine was consistent with the expression trend of IMPDH gene under drought stress. In addition, five PAL, four 4CL, seven CAD, two CCR, and two cytochrome P450 genes were found in the phenylpropanoid biosynthesis pathway. Compared with DSA, these genes did not show significant differences in DSB and DSC. However, the CAD gene was downregulated in DSB vs. DSC. Surprisingly, the phenylpropanol biosynthetic pathway of downstream metabolites [coumaric acid, conibenol, (E)-p-coumaric acid, and 4-hydroxy-3-methoxy cinnamaldehyde] was upregulated compared with DSA. In addition, the glycolysis pathway was also involved in response to drought stress (Figure 7). These results indicate that D. sinense improves its ability to resist drought stress by regulating the metabolism of genetic material and promoting the accumulation of antioxidant products and cytoplasmic material under drought stress.
Figure 7. Pathway diagram of major metabolic pathways. This pathway is constructed based on the KEGG pathway. The color denotes the different expression levels. Dark red denotes upregulation in DSB vs. DSC, blue shows that DEGs or metabolites are upregulated in DSA vs. DSB and downregulation in DSB vs. DSC, strong green denotes downregulation in DSA vs. DSB and DSB vs. DSC. Blue denotes downregulation in DSA vs. DSB or DSA vs. DSC or DSB vs. DSC, red denotes upregulation in DSA vs. DSB and DSB vs. DSC, and purple denotes with upregulation in DSA vs. DSC and DSB vs. DSC.
Compared to genomic DNA-seq, RNA-seq is often faster, simpler, and more scalable and can reveal important information about gene transcription and expression (Trapnell et al., 2010; José and Marta, 2014). Extensive transcriptomic studies have shown that RNA-seq technology is a useful tool for studying adaption of plants to different environmental stress (Brenner and Schmülling, 2012; José and Marta, 2014; Begara-Morales et al., 2014). However, many studies had found that second-generation RNA-seq technology is imperfect, and short reads can reduce sequence and assembly accuracy (Haas and Zody, 2010; Grabherr et al., 2013). With the development of newer sequencing technologies, full-length transcript sequences can be obtained using the PacBio Iso-Seq technology. This technology solves the inherent limitations of Illumina high-throughput sequencing and identifies new gene subtypes with alternatively spliced transcript variants (Roberts et al., 2013; Du and Sun, 2016; An et al., 2018). Previous Illumina-based RNA-seq studies in orchids found the average transcript length to be 764.3 bp in Dendrobium huoshanense (Yuan et al., 2018) and 505 bp in Dendrobium officinale (He et al., 2017). In this present work, the full-length transcripts had an average length of 2,244 bp, similar to previous results of Dendrobium candidum (Xu et al., 2017). These results support the viewpoint that the third-generation sequencing technique can obtain longer transcripts than Illumina-based RNA-seq. To obtain a complete and accurate transcriptome library, short-reads generated from Illumina sequencing were used to correct long reads from third-generation sequencing. Previous studies have used this strategy to generate high-throughput sequencing data, providing reference transcriptomes for plant species that lack genome sequence information (Sharon et al., 2013; Grabherr et al., 2013; Huddleston et al., 2014). To our knowledge, this is the first report of transcriptome sequencing using RNA-seq and PacBio SMRT in D. sinense. This full-length reference transcriptome will be helpful to future genetic studies of D. sinense.
It is well known that multiple adaptative mechanisms have evolved in plants to reduce the effects of stress. Metabolites appear to be the key to these adaptations, with many studies revealing significant differences in the accumulation of metabolites under drought stress (Georgii et al., 2017; Navarro-Cerrillo et al., 2019). In this study, flavonoids, amino acids and derivatives, organic acids and derivatives, and phenylpropanoids were identified as accumulating at significantly different rates across drought conditions, showing upregulation under drought stress in both DSA vs. DSB and DSA vs. DSC. These results are similar to previous studies that also found that the content of flavonoids, amino acids, and other metabolites were increased in drought stress (Georgii et al., 2017; Navarro-Cerrillo et al., 2019). A previous study in Dendrobium moniliforme also indicated that the accumulation of flavonoids and alkaloid was induced by drought stress (Wu et al., 2016). Physiologically, these secondary metabolites in plants can prevent the accumulation of reactive oxygen species from damaging proteins and cell membranes, thereby improving drought resistance (Mutwakil et al., 2017; Šircelj et al., 2017). Specifically, organic acids and small molecule compounds may be involved in carbon metabolism (López-Bucio et al., 2000). From a physiological and biochemical perspective, the increase in flavonoid content in the pseudobulbs of D. sinense appears to be related to adaptability to water (Zhu, 2010; Šircelj et al., 2017). The extremely significant accumulations of amino acids, organic acids, and derivatives in drought-stressed D. sinense further suggest that these are important metabolites in response to drought stress (López-Bucio et al., 2000). In addition, only a few secondary metabolites showed a strong response to drought stress, such as four phenylpropanoids, two flavone, one polyphenol, and one parthenolide. Importantly, acyl syringone, 4-methylcatechol, catechol, apigenin6-C-hexosyl-8-C-hexosyl-O-hexoside, parthenolide, magnolol, and pyrocatechol may be key molecular components of drought stress response in D. sinense. Importantly, strong drought tolerance in D. sinense may in fact be related to its high flavonoid content in the body. This strongly suggests that drought stress induces the accumulation of metabolites that may improve drought tolerance of D. sinense.
Previous work has shown that plants adapt to drought stress by responding to the accumulation of metabolites and altering gene expression (Georgii et al., 2017; Acevedo et al., 2019). Although many drought-resistant genes have been found in model plants, few investigations have been done on Dendrobium. Our study presents a systematic analysis of drought-resistance genes, shedding light on the mechanisms of drought response in Dendrobium. In D. sinense, 622 DEGs were identified as potentially involved in drought stress. Through GO and KEGG enrichment analyses, we found that these DEGs were significantly enriched in ATPase activity, hydrolase activity, acting on acid anhydrides, pyrophosphatase activity, ion binding, and RNA helicase activity. Moreover, many DEGs encoding protein kinases, lipoxygenase, phospholipase, antioxidant enzymes, transporters, ubiquitin-protein ligase, and TFs were identified, such as FBA1, CIPK32, PBL27, LOX2, PLC4, GAD, ABCF1, KEG, GATA26, and C3H. Notably, LOX2 and KEG are involved in the biosynthesis and signal transduction of plant hormones (Wasternack, 2007; Wasternack and Hause, 2013, 2019). ABI5 and EGS are related to ABA biosynthesis (Joshi et al., 2016; Zhu, 2016). These results clearly suggest that hormone signaling may play an important role in the response of D. sinense to drought stress.
Studies in model plants have revealed that nucleotide biosynthesis and degradation are two of the metabolic pathways involved in drought response in Arabidopsis (Watanabe et al., 2014) and rice (Boo and Jung, 1998). Recent work indicated that the nucleotide metabolism pathway was an important pathway for D. wangliangii to adapt to drought stress (Zhao et al., 2019). The IMPHD gene was reported as the rate-limiting enzyme of guanine nucleoside biosynthesis, directly affecting the biosynthesis of guanine, xanthine, and hypoxanthine (Mercati et al., 2019). In DSA vs. DSB, the IMPHD gene was upregulated, and the content of guanine, xanthine, and hypoxanthine increased. In DSB vs. DSC, the expression level of IMPHD gene was downregulated and the metabolites guanine, xanthine, and hypoxanthine were also downregulated. These data indicated that drought stress affected the biosynthesis of purines pathway.
Previous work showed that when guanine is deficient, guanine is replaced by xanthine and hypoxanthine in RNA and DNA synthesis, leading to disorders of the metabolic system (Zoref-Shani et al., 2010). Our results showed that guanine biosynthesis was promoted in response to drought stress in D. sinense, while xanthine and hypoxanthine bioaccumulation was reduced under severe drought stress. This allowed for the maintenance of normal metabolic processes to reduce stress-induced damage. In addition, previous studies have reported that nucleotide metabolism may increase drought tolerance in Hylocereus undatus (Fan et al., 2014) and Triticum boeoticum (Liu et al., 2015). This suggested that purine metabolism may increased the drought tolerance of D. sinense. This result therefore supports findings from previous studies and provides a new insight to explore drought tolerance of epiphytes.
PAL, C4H, and 4CL are key genes in the early stages of lignin and flavonoid biosynthesis (Martens and Mithöfer, 2005). In Fagopyrum esculentum Moench, lignin increases cell wall thickness to prevent water loss and improve drought resistance (Hou et al., 2019). In our study, we found that coumaric acid, coniferol, (E)-p-coumaric acid, and 4-hydroxy-3-methoxy cinnamaldehyde were upregulated in DSB vs. DSC, and five genes related to lignin were also identified. For these genes, only the CAD gene was downregulated in DSA vs. DSB, and no other genes were significantly different in DSB vs. DSC. This negative correlation between lignin biosynthesis and gene expression under drought stress is consistent with results from previous studies (Hu, 2008; Liu, 2019). Reports have also suggested that the root tissue thickness of D. sinense roots increased under drought stress (Wang, 2017). Our results suggest that when D. sinense experience drought stress, lignin accumulation is promoted, thereby increasing cell wall thickness to prevent water loss. In addition, most flavonoids were significantly upregulated in DSB vs. DSC even though we did not identify DEGs associated with flavonoid biosynthesis. However, previous research has shown that genes related to flavonoid biosynthesis were upregulated in buckwheat under drought stress (Hou et al., 2019). It is also suspected that flavonoids have antioxidant properties (Sarker and Oba, 2018). In light of this, our results suggest that D. sinense responds to water deficit in the air by increasing its antioxidant capacity, but the pathway for regulating flavonoid biosynthesis under drought stress needs further exploration.
The datasets presented in this study can be found in online repositories. The names of the repository/repositories and accession number(s) can be found below: NCBI BioProject Accession PRJNA723915.
XS, JN, and CZ conceived the project and designed the experiments. CZ, WH, and JC collected the datasets. CZ and JC plotted the figures. CZ, JC, XS, and JN wrote the manuscript. All authors read and approved the final manuscript.
This study was supported by the Innovative Research Team Program of Hainan Natural Science Fund (2018CXTD331) and Hainan University [KYQD(ZR)1701].
The authors declare that the research was conducted in the absence of any commercial or financial relationships that could be construed as a potential conflict of interest.
We would like to thank Stephen Gaughran at Princeton University for his assistance with English language and grammatical editing.
The Supplementary Material for this article can be found online at: https://www.frontiersin.org/articles/10.3389/fgene.2021.692702/full#supplementary-material
Supplementary Figure 1 | The phenotypes of D. sinense under drought stress. (A,D) were growth in RH ≥ 95%, (B,E) were growth in 45% ≤ RH ≤ 50%, (C,F) were growth in RH ≤ 5%
Supplementary Figure 2 | Different metabolites up- or down-regulated in different groups. (A) DSA vs. DSB, (B) DSA vs. DSC, (C) DSB vs. DSC.
Supplementary Figure 3 | Functional prediction and annotation of transcriptome genes in D. sinense. (A) D. sinense GO enrichment function analysis. The abscissa is the GO term of the next level in the three major categories of GO, and the ordinate is the number of genes annotated to the term (including its sub-term). The three different classifications represent the three basic classifications of GO term from left to right (i.e., biological processes, cell components, and molecular functions). (B) D. sinense gene function prediction. The abscis-axis is the names of the 26 groups of KOG, and the ordinate is the number of genes annotated to the group. (C) D. sinense gene functional annotation Venn diagram. The sum of the numbers in each large circle represents the number of transcripts for the database annotation, and the overlapping parts of the circles represent the transcript annotation results that are common between databases.
Supplementary Figure 4 | Number and distribution of up-regulated and down-regulated genes. (A) The distribution of DEGs in different sample, (B) Summary of D. sinense DEGs under drought stress.
Supplementary Table 1 | Detection of all metabolic quantities and species.
Supplementary Table 2 | Significantly different metabolites between stressed and control samples.
Supplementary Table 3 | Significantly enriched KEGG pathways.
Supplementary Table 4 | Summary of RNA-seq for DSA, DSB, and DSC.
Supplementary Table 5 | GO enrichment result in DEGs.
Supplementary Table 6 | Differential genes and differential metabolites with a correlation coefficient R2 ≥ 0.8.
Supplementary Table 7 | Extremely significantly DEGs GO function annotation.
Acevedo, R. M., Avicol, E. H., Gonzalez, S., Salvador, A. R., Rivarola, M., Paniego, N., et al. (2019). Transcript and metabolic adjustments triggered by drought in Ilex paraguariensis leaves. Planta 250, 445–462. doi: 10.1007/s00425-019-03178-3
An, D., Cao, H., Li, C., Humbeck, K., and Wang, W. (2018). Isoform sequencing and state-of-art applications for unravelling complexity of plant transcriptomes. Genes 9:43. doi: 10.3390/genes9010043
Baena-González, E., Rolland, F., Thevelein, J. M., and Sheen, J. (2007). A central integrator of transcription networks in plant stress and energy signaling. Nature 448, 938–942. doi: 10.1038/nature06069
Bang, S. W., Lee, D. K., Jung, H., Chung, P. J., Kim, Y. S., Choi, Y. D., et al. (2018). Overexpression of OsTF1L, a rice HD-Zip transcription factor, promotes lignin biosynthesis and stomatal closure that improves drought tolerance. Plant Biotechnol. J. 17, 118–131. doi: 10.1111/pbi.12951
Begara-Morales, J. C., Sanchez-Calvo, B., Luque, F., Leyva-Perez, M. O., Leterrier, M., Corpas, F. J., et al. (2014). Differential transcriptomic analysis by RNA-Seq of GSNO-responsive genes between Arabidopsis roots and leaves. Plant Cell Physiol. 55, 1080–1095. doi: 10.1093/pcp/pcu044
Boo, Y. C., and Jung, J. (1998). Alteration in pyridine nucleotide status in cells as an adaptive response to water stress in Rice (Oryza sativa L.) seedlings. Appl. Biol. Chem. 41, 228–234.
Brenner, W. G., and Schmülling, T. (2012). Transcript profiling of cytokinin action in Arabidopsis roots and shoots discovers largely similar but also organ-specific responses. BMC Plant Biol. 12:112. doi: 10.1186/1471-2229-12-112
Chen, B. X., Peng, Y. X., Gao, J. D., Zhang, Q., Liu, Q. J., Fu, H., et al. (2019). Coumarin-induced delay of rice seed germination is mediated by suppression of abscisic acid catabolism and reactive oxygen species production. Front. Plant Sci. 10:828. doi: 10.3389/fpls.2019.00828
Chen, W., Gong, L., Guo, Z., Wang, W. S., Zhang, H. Y., Liu, X. Q., et al. (2013). A novel integrated method for large-scale detection, identification, and quantification of widely targeted metabolites: application in the study of rice metabolomics. Mol. Plant 6, 1769–1780. doi: 10.1093/mp/sst080
Chong, J., and Xia, J. G. (2018). MetaboAnalystR: an R package for flexible and reproducible analysis of metabolomics data. Bioinformatics 34, 4313–4314. doi: 10.1093/bioinformatics/bty528
Claeys, H., and Inze, D. (2013). The agony of choice: how plants balance growth and survival under water-limiting conditions. Plant Physiol. 162, 1768–1779. doi: 10.1104/pp.113.220921
Cui, X. Y., Du, Y. T., Fu, J. D., Yu, T. F., Wang, C. T., Chen, M., et al. (2018). Wheat CBL-interacting protein kinase 23 positively regulates drought stress and ABA responses. BMC Plant Biol. 18:93. doi: 10.1186/s12870-018-1306-5
Domenico, S. D., Bonsegna, S., Horres, R., Pastor, V., Taurino, M., Poltronieri, P., et al. (2012). Transcriptomic analysis of oxylipin biosynthesis genes and chemical profiling reveal an early induction of jasmonates in chickpea roots under drought stress. Plant Physiol. Biochem. 61, 115–122. doi: 10.1016/j.plaphy.2012.09.009
Du, N., and Sun, Y. (2016). Improve homology search sensitivity of PacBio data by correcting frameshifts. Bioinformatics 32, 529–537.
Du, X. L., Zhang, X. F., Nie, H., Liu, M. L., and Cheng, J. L. (2016). Transcript profiling analysis reveals crucial genes regulating main metabolism during adventitious root formation in cuttings of Morus alba L. Plant Growth Regul. 79, 251–262. doi: 10.1007/s10725-015-0130-2
Duan, Z., Zhang, D. Y., Zhang, J. Q., Di, H. Y., Wu, F., Hu, X. W., et al. (2015). Co-transforming bar and CsALDH genes enhanced resistance to herbicide and drought and salt stress in transgenic alfalfa (Medicago sativa L.). Front. Plant Sci. 6:1115. doi: 10.3389/fpls.2015.01115
Fan, J. Q., Yan, X. F., Qiao, G., Zhang, X. B., and Wen, P. X. (2014). Identification of differentially-expressed genes potentially implicated in drought response in pitaya (Hylocereus undatus) by suppression subtractive hybridization and cDNA microarray analysis. Gene 553, 322–331. doi: 10.1016/j.gene.2013.08.098
Fu, L. M., Niu, B. F., Zhu, Z. W., Wu, S. T., and Li, W. Z. (2012). CD-HIT: accelerated for clustering the next-generation sequencing data. Bioinformatics 28, 3150–3152. doi: 10.1093/bioinformatics/bts565
Georgii, E., Jin, M., Zhao, J., Kanawati, B., Schmitt-Kopplin, P., Albert, A., et al. (2017). Relationships between drought, heat and air humidity responses revealed by transcriptome-metabolome co-analysis. BMC Plant Biol. 17:120. doi: 10.1186/s12870-017-1062-y
Grabherr, M. G., Haas, B. J., Yassour, M., Levin, J. Z., and Amit, I. (2013). Trinity: reconstructing a full-length transcriptome without a genome from RNA-Seq data. Nat. Biotechnol. 29, 644–652.
Haas, B. J., and Zody, M. C. (2010). Advancing RNA-Seq analysis. Nat. Biotechnol. 28, 421–423. doi: 10.1038/nbt0510-421
He, L., Fu, S., Xu, Z., Yan, J., Xu, J., Hong, Z., et al. (2017). Hybrid sequencing of full-length cDNA transcripts of stems and leaves in Dendrobium officinale. Genes 8:257. doi: 10.3390/genes8100257
Hou, Z. H., Yin, J. L., Lu, Y. F., Song, J. H., Wang, S. P., Wei, S. D., et al. (2019). Transcriptomic analysis reveals the temporal and spatial changes in physiological process and gene expression in common buckwheat (Fagopyrum esculentum Moench) grown under drought stress. Agronomy 9:569. doi: 10.3390/agronomy9100569
Hu, Y. (2008). Differential Expression of Candidate Genes for Lignin Biosynthesis Under Drought Stress in Maize. Ya’an: Sichuan Agricultural University.
Huddleston, J., Ranade, S., Malig, M., Antonacci, F., Chaisson, M., Hon, L., et al. (2014). Reconstructing complex regions of genomes using long-read sequencing technology. Genome Res. 24, 688–696. doi: 10.1101/gr.168450.113
José, G., and Marta, F. (2014). RNA-Seq applications for plant transcriptome analysis in response to cold acclimation by Ion Torrent technology. Technol. Health Care 12, 125–127.
Joshi, R., Wani, S. H., Singh, B., Bohra, A., Dar, Z. A., Lone, A. A., et al. (2016). Transcription factors and plants response to drought stress: current understanding and future directions. Front. Plant Sci. 7:1029. doi: 10.3389/fpls.2016.01029
Kanehisa, M., and Goto, S. (2000). KEGG: kyoto encyclopedia of genes and genomes. Nucleic Acids Res. 28, 27–30. doi: 10.1093/nar/28.1.27
Li, B., and Dewey, C. N. (2011). RSEM: accurate transcript quantification from RNA-Seq data with or without a reference genome. BMC Bioinformatics 12:323. doi: 10.1186/1471-2105-12-323
Liu, H., Sultan, M. A. R. F., Liu, X. L., Zhang, J., Yu, F., and Zhao, H. X. (2015). Physiological and comparative proteomic analysis reveals different drought responses in roots and leaves of drought-tolerant wild wheat (Triticum boeoticum). PLoS One 10:e0121852. doi: 10.1371/journal.pone.0121852
Liu, W. (2019). Functional Research of CmCADs in Lignin Biosynthesis in Oriental Melon (Cucumis melo L.) Under Abiotic Stresses. Shenyang: Shenyang Agricultural University.
López-Bucio, J., Nieto-Jacobo, M. F., Ramirez-Rodriguez, V. V., and Herrera-Estrella, L. (2000). Organic acid metabolism in plants: from adaptive physiology to transgenic varieties for cultivation in extreme soils. Plant Sci. 160, 1–13. doi: 10.1016/s0168-9452(00)00347-2
Martens, S., and Mithöfer, A. (2005). Flavones and flavone synthases. Phytochemistry 66, 2399–2407. doi: 10.1016/j.phytochem.2005.07.013
Maruyama, K., Takeda, M., Kidokoro, S., Yamada, K., Sakuma, Y., Urano, K., et al. (2009). Metabolic pathways involved in cold acclimation identified by integrated analysis of metabolites and transcripts regulated by DREB1A and DREB2A. Plant Physiol. 150, 1972–1980. doi: 10.1104/pp.109.135327
Maruyama, K., Urano, K., Yoshiwara, K., Morishita, Y., Sakurai, N., Suzuki, H., et al. (2014). Integrated analysis of the effects of cold and dehydration on rice metabolites, phytohormones, and gene transcripts. Plant Physiol. 164, 1759–1771. doi: 10.1104/pp.113.231720
Mercati, F., Fontana, I., Gristina, A. S., Martorana, A., El Nagar, M., De Michele, R., et al. (2019). Transcriptome analysis and codominant markers development in caper, a drought tolerant orphan crop with medicinal value. Sci. REP U. K. 9:10411. doi: 10.1038/s41598-019-46613-x
Morimoto, K., Ohama, N., Kidokoro, S., Mizoi, J., Takahashi, J., Todaka, D., et al. (2017). BPM-CUL3 E3 ligase modulates thermotolerance by facilitating negative regulatory domain-mediated degradation of DREB2A in Arabidopsis. Proc. Natl. Acad. Sci. U.S.A. 114, 8528–8536.
Mutwakil, M. Z., Hajrah, N. H., Atef, A., Edris, S., Sabir, M. J., Al-Ghamdi, A. K., et al. (2017). Transcriptomic and metabolic responses of Calotropis procera to salt and drought stress. BMC Plant Biol. 17:231. doi: 10.1186/s12870-017-1155-7
Navarro-Cerrillo, R. M., Sanchez-Salguero, R., Rodriguez, C., Lazo, J. D., Moreno-Rojas, J. M., Palacios-Rodriguez, G., et al. (2019). Is thinning an alternative when trees could die in response to drought? The case of planted Pinus nigra and P. Sylvestris stands in southern Spain. For. Ecol. Manag. 433, 313–324. doi: 10.1016/j.foreco.2018.11.006
Roberts, R. J., Carneiro, M. O., and Schatz, M. C. (2013). The advantages of SMRT sequencing. Genome Biol. 14:405.
Salmela, L., and Rivals, E. (2014). LoRDEC: accurate and efficient long read error correction. Bioinformatics 30, 3506–3514. doi: 10.1093/bioinformatics/btu538
Sarker, U., and Oba, S. (2018). Drought stress effects on growth, ROS markers, compatible solutes, phenolics, flavonoids, and antioxidant activity in Amaranthus tricolor. Appl. Biochem. Biotech. 186, 999–1016. doi: 10.1007/s12010-018-2784-5
Sharma, M., Banday, Z. Z., Shukla, B. N., and Laxmi, A. (2019). Glucose-regulated HLP1 acts as a key molecule in governing thermomemory. Plant Physiol. 180, 1081–1100. doi: 10.1104/pp.18.01371
Sharon, D., Tilgner, H., Grubert, F., and Snyder, M. (2013). A single-molecule long-read survey of the human transcriptome. Nat. Biotechnol. 31, 1009–1014. doi: 10.1038/nbt.2705
Singh, D., and Laxmi, A. (2015). Transcriptional regulation of drought response: a tortuous network of transcriptional factors. Front. Plant Sci. 6:895. doi: 10.3389/fpls.2015.00895
Šircelj, H., Tausz, M., Grill, D., and Batiča, F. (2017). Detecting different levels of drought stress in apple trees (Malus domestica Borkh.) with selected biochemical and physiological parameters. Sci. Hortic. 113, 362–369. doi: 10.1016/j.scienta.2007.04.012
Stenzel, I., Hause, B., Miersch, O., Kurz, T., Maucher, H., Weichert, H., et al. (2003). Jasmonate biosynthesis and the allene oxide cyclase family of Arabidopsis thaliana. Plant Mol. Biol. 51, 895–911.
Storey, J. D., and Tibshirani, R. (2003). Statistical significance for genome wide studies. Proc. Natl. Acad. Sci. U.S.A. 100, 9440–9445.
Sun, M., Yang, S. J., Zhang, J. L., Bartlett, M., and Zhang, S. B. (2014). Correlated evolution in traits influencing leaf water balance in Dendrobium (Orchidaceae). Plant Ecol. 215, 1255–1267. doi: 10.1007/s11258-014-0383-2
Trapnell, C., Williams, B. A., Pertea, G., Mortazavi, A., Kwan, G., Baren, M. J. V., et al. (2010). Transcript assembly and quantification by RNA-Seq reveals unannotated transcripts and isoform switching during cell differentiation. Nat. Biotechnol. 28, 511–515. doi: 10.1038/nbt.1621
Wang, X. M. (2017). Mycorrhiza Biology of Dendrobium sinense (Orchidaceae), An Endemic Species in Hannan Island. Hai Kou: Han Nan University.
Wasternack, C. (2007). Jasmonates: an update on biosynthesis, signal transduction and action in plant stress response, growth and development. Ann. Bot. 100, 681–697. doi: 10.1093/aob/mcm079
Wasternack, C., and Hause, B. (2013). Jasmonates: biosynthesis, perception, signal transduction and action in plant stress response, growth and development. an update to the 2007 review in Annals of Botany. Ann. Bot. 111, 1021–1058. doi: 10.1093/aob/mct067
Wasternack, C., and Hause, B. (2019). The missing link in jasmonic acid biosynthesis. Nat. Plants 5, 776–777. doi: 10.1038/s41477-019-0492-y
Watanabe, S., Kounosu, Y., Shimada, H., and Sakamoto, A. (2014) Arabidopsis xanthine dehydrogenase mutants defective in purine degradation show a compromised protective response to drought and oxidative stress. Plant Biotechnol. 31, 173–178. doi: 10.5511/plantbiotechnology.14.0117a
Wu, X., Yuan, J., Luo, A., Chen, Y., and Fan, Y. (2016). Drought stress and re-watering increase secondary metabolites and enzyme activity in Dendrobium moniliforme. Ind. Crops Prod. 94, 385–393. doi: 10.1016/j.indcrop.2016.08.041
Xu, M., Liu, X., Wang, J. W., Teng, S. Y., Shi, J. Q., Li, Y. Y., et al. (2017). Transcriptome sequencing and development of novel genic SSR markers for Dendrobium officinale. Mol. Breed. 37:18.
Yang, F. S. (2009). Conservation Biology on the Base of Endophytic Mycorrhizal Fungi of an Endangered Orchid Dendrobium sinense. Matsubara: Han Nan University.
Yuan, Y. D., Yu, M. Y., Jia, Z. H., Song, X. E., Liang, Y. Q., and Zhang, J. C. (2018). Analysis of Dendrobium huoshanense transcriptome unveils putative genes associated with active ingredients synthesis. BMC Genomics 19:978. doi: 10.1186/s12864-018-5305-6
Zhang, W., Hu, H., and Zhang, S. B. (2016). Divergent adaptive strategies by two co-occurring epiphytic Orchids to water stress: escape or avoidance? Front. Plant Sci. 7:588. doi: 10.3389/fpls.2016.00588
Zhang, X. Y., Yang, Z. R., Li, Z., Zhang, F. L., and Hao, L. Z. (2019). De novo transcriptome assembly and co-expression network analysis of Cynanchum thesioides: identification of genes involved in resistance to drought stress. Gene 710, 375–386. doi: 10.1016/j.gene.2019.05.055
Zhao, D. K., Shi, Y. N., Senthilkumar, H. A., Qiao, Q., Wang, Q. X., Shen, Y., et al. (2019). Enriched networks ‘nucleoside/nucleotide and ribonucleoside/ribonucleotide metabolic processes’ and ‘response to stimulus’ potentially conferred to drought adaptation of the epiphytic orchid Dendrobium wangliangii. Physiol. Mol. Biol. Plants 25, 31–45. doi: 10.1007/s12298-018-0607-3
Zhou, S. M., Sun, X. D., Yin, S. H., Kong, X. Z., Zhou, S., Xu, Y., et al. (2014). The role of the F-box gene TaFBA1 from wheat (Triticum aestivum L.) in drought tolerance. Plant Physiol. Biochem. 84, 213–223. doi: 10.1016/j.plaphy.2014.09.017
Zhu, C. C. (2010). Effects of Environmental Factors on the Growth and Principal Medicinal Compositions of Ginkgo Biloba Seedlings. Nanjing: Nanjing Forestry University.
Zhu, J. K. (2016). Abiotic stress signaling and responses in plants. Cell 167, 313–324. doi: 10.1016/j.cell.2016.08.029
Zoref-Shani, E., Bromberg, Y., Brosh, S., Sidi, Y., and Sperling, O. (2010). Characterization of the alterations in purine nucleotide metabolism in hypoxanthine-guanine phosphoribosyltransferase-deficient rat neuroma cell line. J. Neurochem. 61, 457–463. doi: 10.1111/j.1471-4159.1993.tb02146.x
Keywords: Dendrobium sinense, drought stress, metabolomics, transcriptomics, third-generation sequencing, purine metabolism, phenylpropanoid biosynthesis
Citation: Zhang C, Chen J, Huang W, Song X and Niu J (2021) Transcriptomics and Metabolomics Reveal Purine and Phenylpropanoid Metabolism Response to Drought Stress in Dendrobium sinense, an Endemic Orchid Species in Hainan Island. Front. Genet. 12:692702. doi: 10.3389/fgene.2021.692702
Received: 09 April 2021; Accepted: 24 May 2021;
Published: 02 July 2021.
Edited by:
Lidan Sun, Beijing Forestry University, ChinaReviewed by:
Chunyu Zhang, Beijing Forestry University, ChinaCopyright © 2021 Zhang, Chen, Huang, Song and Niu. This is an open-access article distributed under the terms of the Creative Commons Attribution License (CC BY). The use, distribution or reproduction in other forums is permitted, provided the original author(s) and the copyright owner(s) are credited and that the original publication in this journal is cited, in accordance with accepted academic practice. No use, distribution or reproduction is permitted which does not comply with these terms.
*Correspondence: Xiqiang Song, c29uZ3N0cm9uZ0BoYWluYW51LmVkdS5jbg==; Jun Niu, bml1anVuQGhhaW5hbnUuZWR1LmNu
†These authors have contributed equally to this work
Disclaimer: All claims expressed in this article are solely those of the authors and do not necessarily represent those of their affiliated organizations, or those of the publisher, the editors and the reviewers. Any product that may be evaluated in this article or claim that may be made by its manufacturer is not guaranteed or endorsed by the publisher.
Research integrity at Frontiers
Learn more about the work of our research integrity team to safeguard the quality of each article we publish.