- 1Department of Clinical Epidemiology and Biostatistics, Faculty of Medicine Ramathibodi Hospital, Mahidol University, Bangkok, Thailand
- 2Department of Pharmacology, College of Pharmacy, Rangsit University, Pathum Thani, Thailand
- 3Department of Medicine, Faculty of Medicine Ramathibodi Hospital, Mahidol University, Bangkok, Thailand
- 4Centre for Public Health, School of Medicine, Dentistry and Biomedical Sciences, Queen’s University Belfast, Belfast, United Kingdom
- 5Centre for Clinical Epidemiology and Biostatistics, School of Medicine and Public Health, Faculty of Health and Medicine, University of Newcastle, and Hunter Medical Research Institute, New Lambton, NSW, Australia
Background: Mendelian Randomization (MR) studies show conflicting causal associations of genetically predicted serum urate with cardiovascular risk factors (i.e., hypertension, diabetes, lipid profile, and kidney function). This study aimed to robustly investigate a causal relationship between urate and cardiovascular risk factors considering single nucleotide polymorphisms (SNPs) as instrumental variables using two-sample MR and various sensitivity analyses.
Methods: Data on SNP-urate associations were taken from the Global Urate Genetics Consortium and data on SNP-cardiovascular risk factor associations were taken from various consortia/UK Biobank. SNPs were selected by statistically and biologically driven approaches as instrumental variables. Various sensitivity analyses were performed using different MR methods including inverse variance weighted, MR-Egger, weighted median/mode, MR-PRESSO, and the contamination mixture method.
Results: The statistically driven approach showed significant causal effects of urate on HDL-C and triglycerides using four of the six MR methods, i.e., every 1 mg/dl increase in genetically predicted urate was associated with 0.047 to 0.103 SD decrease in HDL-C and 0.034 to 0.207 SD increase in triglycerides. The biologically driven approach to selection of SNPs from ABCG2, SLC2A9, SLC17A1, SLC22A11, and SLC22A12 showed consistent causal effects of urate on HDL-C from all methods with 0.038 to 0.057 SD decrease in HDL-C per 1 mg/dl increase of urate, and no evidence of horizontal pleiotropy was detected.
Conclusion: Our study suggests a significant and robust causal effect of genetically predicted urate on HDL-C. This finding may explain a small proportion (7%) of the association between increased urate and cardiovascular disease but points to urate being a novel cardiac risk factor.
Introduction
Cardiovascular disease (CVD) carries the greatest global disease burden (World Health Organization, 2017). It can be caused by various risk factors including serum urate (Feig et al., 2008). Previous meta-analyses have shown that elevated urate/hyperuricemia is associated not only with CVD (Kim et al., 2010) but also with CVD risk factors, i.e., hypertension (Grayson et al., 2011), type 2 diabetes mellitus (T2DM) (Kodama et al., 2009), metabolic syndrome (Yuan et al., 2015), and chronic kidney disease (CKD) (Li et al., 2014). Some studies also show that colchicine, a drug used for treatment of gout induced by urate crystal deposition, can reduce the risk of CVD (Tardif et al., 2019; Nidorf et al., 2020). However, it is unclear whether urate is truly causal for CVD, and if it is, whether this is through traditional risk factors or via a novel route.
Mendelian Randomization (MR) has been increasingly used to assess causal inference between gene(s), exposure/phenotype and clinical outcomes, considering that alleles are randomly allocated at meiosis and hence can be used as instrumental variables (IV) to test causation (Lawlor et al., 2008). Meiosis is similar to a randomized trial in that alleles are randomly assigned at conception, and hence unconfounded, except for ethnicity. The effect size of a single nucleotide polymorphism (SNP) is often too weak to serve as a robust IV, and thus genetic risk scores consisting of many SNPs are used to boost the effect size and increase the strength of the IV.
Two-sample MR is commonly used to assess causal association. It requires summary statistic data for SNP-phenotype and SNP-outcome associations that are derived from meta-analyses of genome-wide association studies (GWAS). Various statistical methods have been applied, e.g., inverse variance weighted (IVW) (Lawlor et al., 2008; Burgess et al., 2013), MR-Egger regression (Bowden et al., 2015), weighted median estimator (Bowden et al., 2016), weighted mode estimator (Hartwig et al., 2017), MR-PRESSO (Verbanck et al., 2018), and the contamination mixture method (Burgess et al., 2020b).
Several MR studies have been conducted to assess causal associations of genetic IVs related to urate and coronary heart disease (CHD) (Yang et al., 2010; Kleber et al., 2015; Keenan et al., 2016; White et al., 2016; Efstathiadou et al., 2019), hypertension (Kleber et al., 2015), body mass index (BMI) (White et al., 2016), fasting glucose (Yang et al., 2010; White et al., 2016), T2DM (Sluijs et al., 2015; Keenan et al., 2016; White et al., 2016), estimated glomerular filtration rate (eGFR) (Yang et al., 2010; Jordan et al., 2019), CKD (Yang et al., 2010; Jordan et al., 2019), and lipid profile (White et al., 2016). None suggested a causal association between urate and CHD (Yang et al., 2010; Kleber et al., 2015; Keenan et al., 2016; White et al., 2016), except one study (Efstathiadou et al., 2019) which suggested a modest causal association between urate and CHD, and one study (White et al., 2016) which found causal associations between 31 urate-SNPs and some CVD risk factors using the IVW method, which can be biased in the presence of pleiotropy (i.e., the IVs are associated with the outcome through pathways other than urate). Therefore, sensitivity analyses using other MR methods are recommended (Bowden et al., 2016; Burgess et al., 2020a). This study aimed to investigate a causal relationship between urate and CVD risk factors using genetic variants as the IVs, and two-sample MR with various sensitivity analyses to explore the robustness of the findings.
Materials and Methods
We implemented two-sample MR complying with the STROBE-MR guidelines (Davey Smith et al., 2019) and the guidelines for performing MR (Burgess et al., 2020a). A MR causal-diagram was composed of genetic IVs, urate (as the exposure), and CVD risk factors (as outcomes, see Figure 1). Three IV assumptions were considered (Bowden et al., 2015); genetic IVs are strongly associated with urate; genetic IVs are associated with CVD risk factors only through urate; the associations between genetic IVs and urate and CVD risk factors are unconfounded.
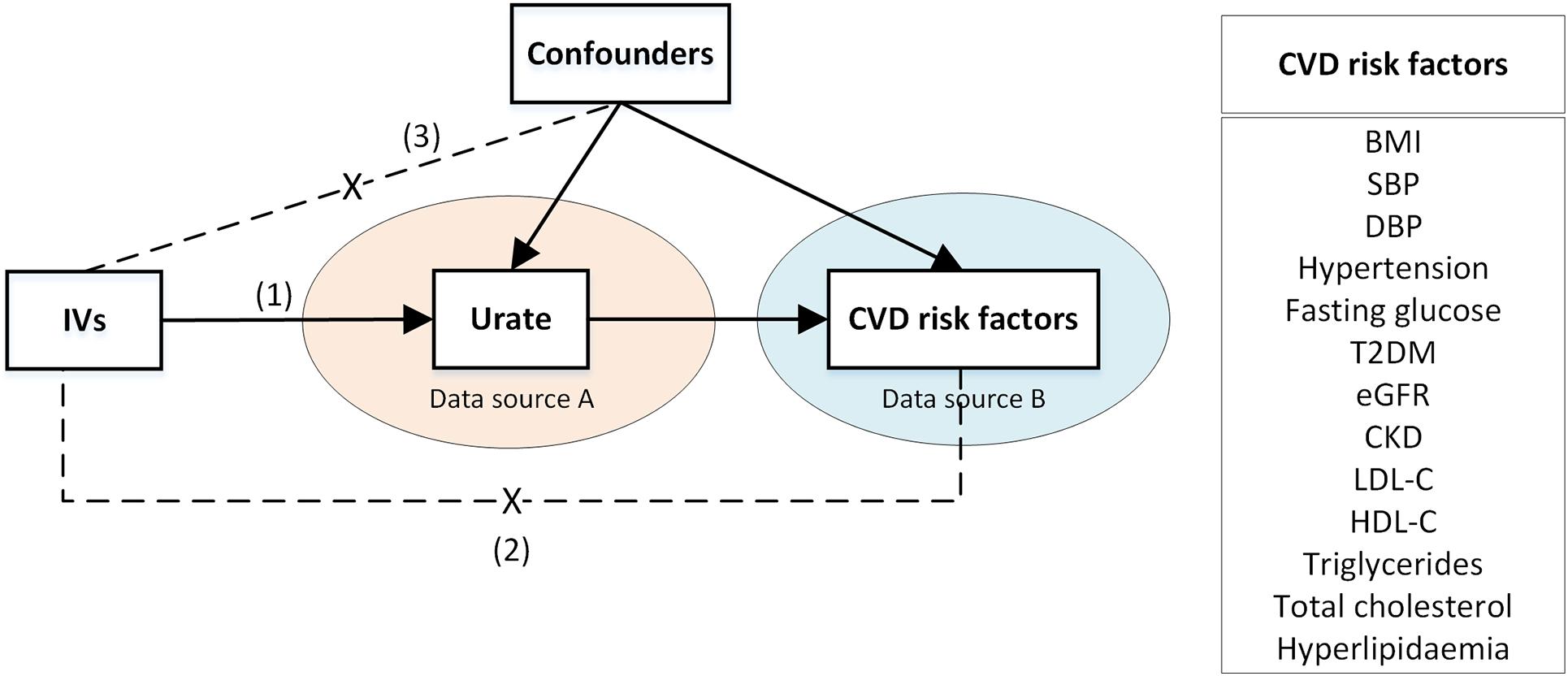
Figure 1. A two-sample Mendelian Randomization causal diagram with three instrumental variable assumptions. (1) IVs are associated with urate; (2) IVs have no direct effect on the CVD risk factors, except through urate; (3) IVs are not associated with confounders of urate -CVD risk factor association. Arrows represent associations. Dashed lines with a cross in the middle represent no associations. BMI, body mass index; CKD, chronic kidney disease; CVD, cardiovascular disease; DBP, diastolic blood pressure; eGFR, estimated glomerular filtration rate; HDL-C, high-density lipoprotein cholesterol; IV, instrumental variable; LDL-C, low-density lipoprotein cholesterol; SBP, systolic blood pressure; T2DM, type 2 diabetes mellitus.
Data Sources
Summary data were retrieved from GWAS datasets via the MR-Base platform developed by the Medical Research Council Integrative Epidemiology Unit (MRC IEU); these datasets have already undergone the recommended quality control processes as previously described (Hemani et al., 2018). The data characteristics and the CVD risk factors considered are described in Table 1. Ethics approval and informed consent were previously obtained for all individual studies included. Data were retrieved for two pathways as follows:
SNP → Urate Association
Single nucleotide polymorphism-urate associations were obtained from the Global Urate Genetics Consortium (GUGC), i.e., a meta-analysis of 48 GWAS in 110,347 Europeans (Kottgen et al., 2013). Mean age and percentage of males were 52.12 years and 45.15%, respectively. Mean (standard deviation; SD) urate ranged from 3.86 (0.92) to 6.10 (1.46) mg/dl; most studies used the uricase method for measuring urate. SNPs were selected as genetic IVs based on two approaches (Burgess et al., 2020a). First, a statistically driven approach was used to select SNPs that were highly associated with urate (P-value < 5 × 10–8) and in low linkage-disequilibrium with other SNPs (r2 < 0.001) within a clumping distance of 10,000 kb. Second, a biologically driven approach was used considering candidate genes ABCG2, SLC2A9, SLC22A12, SLC22A11, SLC17A1, and SLC17A3 which encode urate transporters (Yang et al., 2010; Kottgen et al., 2013; Merriman, 2017); SNPs that were associated with urate (P-value < 5 × 10–8) and independent at r2 < 0.1 were selected. The summary data of the IVs on urate were extracted, F-statistic > 10 was used to evaluate if those SNPs were qualified IVs.
SNP → CVD Risk Factor Associations
Cardiovascular disease risk factors included blood pressure [i.e., systolic blood pressure (SBP)/diastolic blood pressure (DBP) and hypertension], T2DM and fasting glucose, renal outcomes (i.e., CKD and eGFR), lipid profile (i.e., LDL-C, HDL-C, triglycerides, total cholesterol, and hyperlipidemia), and BMI, see Table 1.
Summary data for SNP-BP associations (SBP/DBP and hypertension, defined as clinically diagnosed high blood pressure) were obtained from the MRC-IEU UK Biobank GWAS pipeline (Elsworth et al., 2017), see more details in Supplementary Note 1. Associations of SNP-SBP, SNP-DBP, and SNP-hypertension were obtained from >436,000 European participants.
Summary data for SNP-T2DM associations were obtained from the DIAbetes Genetics Replication And Meta-analysis (DIAGRAM) Consortium (Morris et al., 2012) and SNP-fasting glucose associations were from the Meta-Analyses of Glucose and Insulin-related traits Consortium (MAGIC) (Manning et al., 2012). The DIAGRAM Consortium data was extracted from the DIAGRAMv3 GWAS meta-analysis comprised of 12,171 T2DM cases and 56,862 controls of Europeans from 12 GWAS. T2DM was defined by original studies using several criteria including a fasting glucose ≥ 126 mg/dl, HbA1c ≥ 6.5%, self-report, medications used, etc. For MAGIC, data was included from >58,000 Europeans across 29 cohorts from the discovery stage.
Associations of SNP-CKD and SNP-eGFR were retrieved from the Chronic Kidney Disease Genetics (CKDGen) Consortium consisting of 43 studies for CKD (12,385 CKD cases and 104,780 controls) and 48 studies with 133,814 individuals for creatinine-based eGFR (eGFRcrea) (Pattaro et al., 2016). Participants were mainly of European descent. CKD was defined as eGFRcrea <60 ml/min/1.73m2. SNP-BMI summary data were retrieved from the Genetic Investigation of ANthropometric Traits (GIANT) Consortium (Locke et al., 2015), which included 125 studies from 322,154 European participants.
The SNPs-lipid profile data (i.e., LDL-C, HDL-C, triglycerides, and total cholesterol) were obtained from the Global Lipids Genetics Consortium (GLGC) (Willer et al., 2013), which pooled 23 GWAS from 94,595 Europeans. In addition, summary statistics for hyperlipidemia (3,439 cases and 459,571 controls), defined by ICD-10, were also retrieved from the MRC-IEU UK Biobank.
The effects of the genetic IVs on each CVD risk factor were extracted from source data as described above. Where specific SNP data was missing, a proxy SNP in linkage disequilibrium (r2 ≥ 0.8) was used instead (Hemani et al., 2018). All data were aligned to the Genome Reference Consortium Human Build 37 (GRCh37) and SNP identifiers were mapped to dbSNP build 144.
Data Harmonization
Summary data for the SNP-urate and SNP-CVD risk factor associations [beta coefficient (β) and standard error (SE)] were retrieved and harmonized for comparisons of minor versus major (reference) alleles across databases. Palindromic SNPs (i.e., SNPs with A/T or G/C) with a minor allele frequency above 0.42 were excluded given the difficulty in identifying the reference strand when the minor allele frequency was close to 0.5 (Hemani et al., 2018).
Statistical Analysis
The two-sample MR analyses were performed according to the guidelines (Burgess et al., 2020a). The main causal effect was estimated by a ratio of beta-coefficients of SNP-CVD risk factors to SNP-urate, and this was combined across all genetic IVs using the IVW method (Burgess et al., 2013) with a random-effect model. Five additional sensitivity analyses [i.e., MR-Egger (Bowden et al., 2015), weighted median (Bowden et al., 2016) and mode (Hartwig et al., 2017), MR-PRESSO (Verbanck et al., 2018), and the contamination mixture methods (Burgess et al., 2020b)] were performed to assess whether the causal estimates were robust to potential horizontal pleiotropy, see more details in Supplementary Note 2. Furthermore, leave-one-out analysis was conducted by removing one SNP at a time to observe the individual contributions to the IVW causal effects. In addition, bidirectional MR analysis was performed to identify the direction of any causal association. Steiger filtering was used to remove SNPs that were more correlated to the outcome than the exposure. Power calculations were performed, see Supplementary Tables 1A,B. R software version 3.6.3 was used for all analyses.
Results
Selection of Genetic IVs Based on the Statistically Driven Approach
There were 2,450,547 autosomal SNP-urate associations in the GUGC, of which 27 were significantly associated with urate with F statistics ranging from 35.39 to 1406.25 with corresponding P-values of 2.36 × 10–8 to 1.00 × 10–200; the proportion of phenotypic variance explained by each SNP (R2) ranged from 0.00032 to 0.01262, see Supplementary Table 2. Two palindromic SNPs (rs17632159 and rs6830367) were removed leaving 25 SNPs for SNP-urate and SNP-outcome associations, except for hyperlipidemia, where an additional two SNPs (rs7654258 and rs1825043) did not have outcome data, leaving 23 SNPs in total.
Mendelian Randomization results are described in Figures 2A,B. Between 15 and 25 SNPs were significantly associated with continuous outcomes through urate. Results from the six different MR methods were largely consistent for BMI, LDL-C, triglycerides, and total cholesterol with inconsistent causal effects for SBP, DBP, and HDL-C. For instance, two or three of the six MR methods showed significant causal effects of urate on SBP (i.e., MR-Egger, MR-PRESSO, and the contamination mixture method) and DBP (i.e., MR-Egger and MR-PRESSO) but the direction of the effects differed depending on the MR approach used. Four MR methods (i.e., IVW, weighted median, weighted mode, and MR-PRESSO) consistently showed significant causal effects of urate on HDL-C, i.e., for each 1 mg/dl increase in urate determined by genetic variants, there was a 0.047 to 0.103 SD decrease in HDL-C. In addition, the IVW method, weighted median estimator, MR-PRESSO, and the contamination mixture methods also demonstrated that for each 1 mg/dl increase in urate determined by genetic variants, there was a 0.034 to 0.207 SD increase in triglycerides, see Figure 2A. No causal effects of urate on fasting glucose and eGFR were observed.
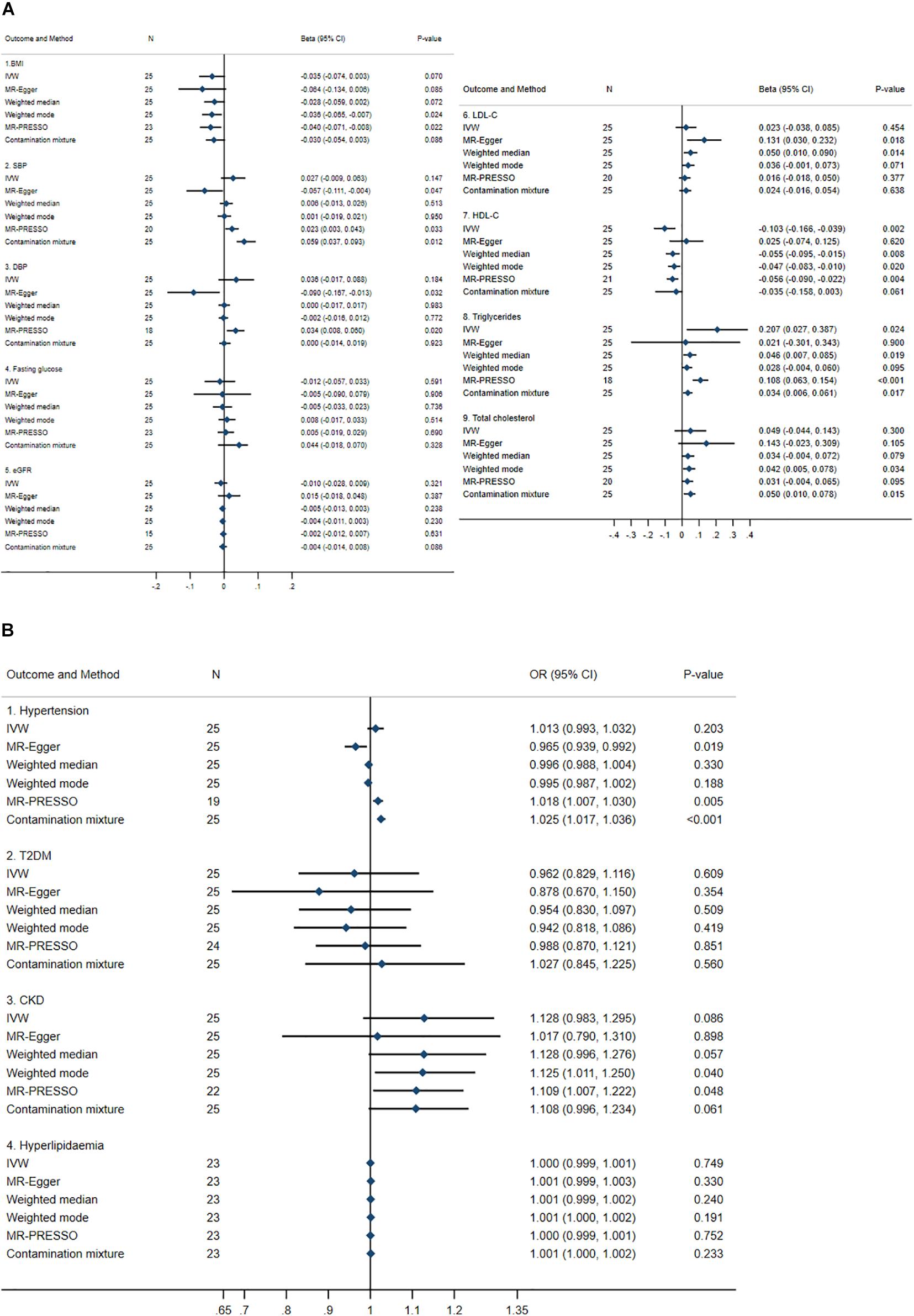
Figure 2. Summary causal effects of genetically predicted urate on cardiovascular risk factors by a statistically driven approach. (A) Continuous outcomes. (B) Binary outcomes. Refer to the unit of continuous outcomes in Table 1. Confidence interval (CI) from the contamination mixture method for LDL-C and T2DM contained multiple ranges of values (not shown in the figure). IVW, inverse variance weighted; MR-PRESSO, Mendelian Randomization Pleiotropy Residual Sum and Outlier; OR, odds ratio. For abbreviated outcomes, refer to abbreviation lists in Figure 1.
For dichotomous outcomes, no causal effects of urate on T2DM or hyperlipidemia were identified but inconsistent causal effects on hypertension were observed. For hypertension, two of the six MR methods (i.e., MR-PRESSO and the contamination mixture method) detected causal risk effects with odds ratio (ORs) between 1.018 and 1.025, whereas MR-Egger detected an OR of 0.965. For CKD, two of the six MR methods (i.e., weighted-mode and MR-PRESSO) identified significant causal effects of urate with ORs of 1.109 and 1.125, respectively.
Heterogeneity was detected for all outcomes, see Supplementary Table 3. In addition, the pleiotropy test using the MR-Egger method identified significant pleiotropy with SBP, DBP, hypertension, LDL-C, and HDL-C, see Supplementary Table 3. It was noted that the contamination mixture method identified bimodal causal estimates of urate on LDL-C and T2DM, see Supplementary Table 4.
Additional leave-one-out plots were constructed, see Supplementary Figures 1A–M. Omitting a single SNP at a time did not significantly change the IVW causal estimates for any outcome, except BMI, SBP, CKD, and triglycerides, where the confidence intervals shifted from/to the null indicating non-robust causal estimates potentially due to outliers; scatter plots between genetically predicted urate and BMI, SBP, CKD, and triglycerides are shown in Supplementary Figures 2–5, respectively.
Selection of Genetic IVs Based on the Biologically Driven Approach
Single nucleotide polymorphisms in six genes regulating urate transport were considered. Of these, 15 SNPs were significantly associated with urate (i.e., three SNPs in ABCG2, six SNPs in SLC2A9, four SNPs in SLC17A1, one SNP in SLC22A11, and one SNP in SLC22A12) with F-statistics of 49.00 to 2243.77 and corresponding P-values of 1.30 × 10–10 to 1.00 × 10–200 and R2 of 0.00045 to 0.01999, see Supplementary Table 5. Three SNPs in SLC2A9 (rs10516194, rs13128385, and rs16891971) and rs10498730 in SLC17A1 were removed due either to outcome data not being available or SNPs being palindromic; therefore, 11–14 SNPs remained in the analyses.
Mendelian Randomization results from the biologically driven approach are summarized (see Figures 3A,B), and were largely similar to those from the statistically driven approach but were more precise. For example, the causal estimates of urate on HDL-C were significant for all methods, i.e., for each 1 mg/dl increase in genetically predicted urate, HDL-C decreased by 0.038 to 0.057 SD. Sensitivity analysis to determine the validity of the genetic IVs using the contamination mixture method by varying SD (i.e., ψ) yielded consistent results, see Supplementary Table 6. Leave-one-out analysis also showed robust results for all outcomes, see Supplementary Figures 2A–M.
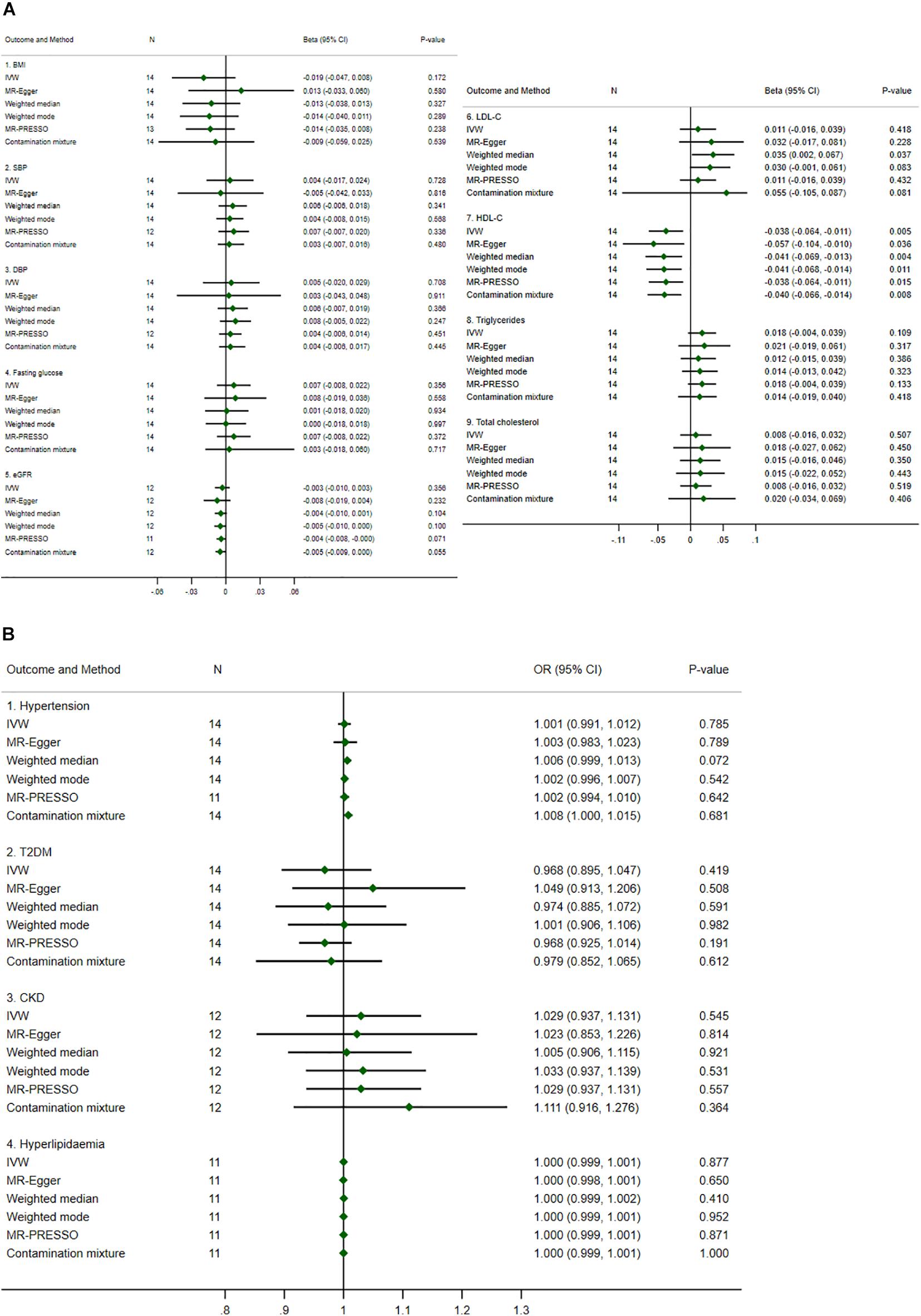
Figure 3. Summary causal effects of genetically predicted urate on cardiovascular risk factors by a biologically driven approach. (A) Continuous outcomes. (B) Binary outcomes. Refer to the unit of continuous outcomes in Table 1. Confidence interval (CI) from the contamination mixture method for hypertension contained multiple ranges of values (not shown in the figure). IVW, inverse variance weighted; MR-PRESSO, Mendelian Randomization Pleiotropy Residual Sum and Outlier; OR, odds ratio. For abbreviated outcomes, refer to abbreviation lists in Figure 1.
Heterogeneity was identified as borderline for HDL-C (P-value = 0.073 and 0.077 for IVW and MR-Egger methods, respectively), see Supplementary Table 7. The pleiotropy test assessed by the MR-Egger method was not significant for any causal estimates, see Supplementary Table 7. The confidence intervals for the causal estimates of urate on hypertension using the contamination mixture method did not converge and showed multiple ranges of values, see Supplementary Table 8.
We further performed bidirectional MR analysis to explore if genetically predicted HDL-C was causally associated with urate. A total 87 SNPs were highly associated with HDL-C with F-statistics ranging from 29.95 to 1749.13. Two palindromic SNPs were removed leaving 85 SNPs as genetic IVs. It was found that all MR methods except MR-Egger showed significant causal effects of HDL-C on urate, i.e., for each one SD increase in genetically predicted HDL-C, there was a 0.066 to 0.115 mg/dl decrease in urate, see Supplementary Note 3 and Supplementary Table 9.
Discussion
We conducted two-sample MR assessing causal associations between genetically predicted urate and CVD risk factors using six analysis methods. Our results show robust causal associations between genetically predicted urate and HDL-C from the biologically driven approach. In addition, from the statistically driven approach, leave-one-out analyses suggested that rs1260326 in GCKR was the main genetic driver of urate on increased triglycerides and removing it resulted in a loss of significance. This finding is consistent with previous reported associations between rs1260326 and increased plasma triglycerides (Vaxillaire et al., 2008).
Six genes encoding urate transporters were considered in the biologically driven approach, i.e., ABCG2, SLC17A1, and SLC17A3 involved in urate excretion whereas SLC2A9, SLC22A12, and SLC22A11 regulated urate reabsorption in the renal proximal tubule (Merriman, 2017). The results showed significant causal estimates of these genetically predicted urate levels on decreased HDL-C, which were consistent across different MR methods. Sensitivity analyses using the leave-one-out method and varying the SD of invalid IVs using the contamination mixture method indicated robust results. In addition, the MR-Lasso and MR-RAPS methods also showed consistent significant results, see Supplementary Tables 10, 11. Furthermore, the causal estimates showed low heterogeneity and no evidence of pleiotropy, indicating valid genetic IVs. Our findings suggested that a genetically predicted increase of one unit in urate would decrease HDL-C by approximately 0.05 SD. A previous MR study (Allara et al., 2019) suggested that 1 SD increase in HDL-C would result in OR of CHD of 0.91. In other words, the OR for 1 SD decrease in HDL-C on CHD is about 1.10. The causal effects of 28 SNPs on CHD through urate was reported with an OR (estimated by MR-PRESSO) of 1.07 (Efstathiadou et al., 2019). If these causal effects are truly present, combining these figures using a mediation framework (Burgess et al., 2015), i.e., HDL-C mediates the effect of urate on CHD, leads to the estimate that 7% of the causal effect of genetically predicted urate on CHD is through HDL-C, see Supplementary Note 4. This would indicate that urate may be a novel risk factor for CVD and does not mediate its effect simply through other traditional risk factors. Inflammation plays a crucial role in CVD progression and emerging evidence suggests that colchicine, an anti-inflammatory medication used to treat gout, may also prove beneficial for the treatment of chronic coronary artery disease and the progression of atherosclerosis (Opstal et al., 2020).
Several studies previously conducted two-sample MR analysis with a statistically driven approach to assess causal relationships between urate and CVD risk factors. One study reported small causal effects of urate on HDL-C, triglycerides, SBP, and DBP, and no significant causal effects of urate on LDL-C, total cholesterol, fasting glucose, T2DM, and BMI (White et al., 2016). However, their results were based solely on the IVW method with no other MR methods to evaluate pleiotropy, so the causal effects may have been biased.
Another study identified inconsistent causal estimates of urate on SBP using different MR methods (Efstathiadou et al., 2019). Based on our statistically driven approach, only MR-PRESSO and the contamination mixture methods were able to identify the causal estimates of urate on SBP but these may have been biased as heterogeneity and pleiotropy were significantly present. In addition, a sensitivity analysis by varying the SD of invalid genetic IVs (ψ) in the contamination mixture method shifted the lower confidence interval toward the null, questioning the validity of the instruments. This method also detected multiple ranges across the confidence intervals of the causal estimates on both SBP and hypertension, implicating more than one biological mechanism linking urate and SBP or hypertension (data not shown). Our leave-one-out analyses also showed that rs2231142 in ABCG2 was influential on the effect of urate on SBP.
Another recent study revealed no evidence of causal effects of urate on either eGFR or CKD from different MR methods (Jordan et al., 2019), which was similar to our statistically driven approach by the contamination mixture method. Furthermore, we found no causal effects of urate on fasting glucose and T2DM in line with other MR studies using individual level data and different MR approaches (Kleber et al., 2015; Sluijs et al., 2015).
Our findings suggest that urate may be causally related to HDL-C levels. This supports a previous meta-analysis demonstrating a significant inverse relationship between urate and HDL-C (Chen et al., 2020b), although longitudinal studies reported inconsistent findings (Gonçalves et al., 2012; Babio et al., 2015). One small study observed significantly higher HDL-C after 3 months of allopurinol administration, a xanthine oxidase inhibitor used for hyperuricemia treatment (Ziga and Becic, 2013). However, randomized controlled trials did not find a significant change in HDL-C levels in CKD and CVD patients receiving xanthine oxidase inhibitors (Bowden et al., 2013; Nakagomi et al., 2015). Nevertheless, there are a number of biological mechanisms suggesting that urate may reduce HDL-C. It has been shown that higher fructose consumption leads to increased circulating urate and metabolic syndrome, and, by reducing urate through allopurinol, the derangements in the metabolic features can be alleviated (Nakagawa et al., 2006). Another potential mechanism may be mediated through fibroblast growth factor 21 (FGF21), a metabolic regulator that demonstrates glucose and lipid-lowering effects in various animal models (Xie and Leung, 2017). In diabetic monkeys, the administration of recombinant FGF21 improves the blood lipid profile including increasing HDL-C in a dose-dependent manner (Kharitonenkov et al., 2007). Recently, miR-149-5p, which targets FGF21, was shown to be significantly up-regulated in uric acid-stimulated hepatocytes, leading to aggregated uric acid-induced triglyceride accumulation (Chen et al., 2020a). Taken together, it is probable that urate is causally related to reduced HDL-C. Surprisingly, bidirectional MR indicated that a reverse causal association between genetically predicted HDL-C and urate may also be present; the mechanism for this may be that an increase in apolipoprotein-A1 (the main component of HDL-C) is associated with high eGFR (Goek et al., 2012), which consequently increases uric acid excretion through urine, thus lowering serum urate level as previously proposed (Peng et al., 2015).
Our study has several strengths. Two-sample MR analysis increases study power through the inclusion of larger sample sizes from GWAS consortia. MR is also less influenced by potential confounding due to the random allocation of alleles at conception. The genetic IVs were carefully selected by the statistically and biologically driven hypotheses to strengthen the robustness of the results. Our findings favor the biologically driven approach, where SNPs were selected from genes with biological plausibility, leading to lower heterogeneity and pleiotropy than the statistically driven approach. The data sources used were mostly selected from populations of European ancestry, therefore, population stratification is much less likely. These data also originate from public sources, so our approach is transparent and reproducible. We have comprehensively performed six different MR methods to account for pleiotropy and observed robust results, including the contamination mixture method, a novel MR method that has been proposed to better address the issue of invalid instruments (Burgess et al., 2020b). The urate and HDL-C causal relationship was supported by the robust identification of genetic variants in urate transporters.
Limitations
Some limitations could be not avoided. We used two-sample MR methods, where datasets for SNP-urate and SNP-outcomes were derived from different individuals. A degree of overlap in data sources for SNP-urate (i.e., GUGC) and the outcome consortia (i.e., CKDgen, DIAGRAM, MAGIC, GIANT, and GLGC) might also affect causal estimates. The results of LD score regression analysis provided in LD Hub (Bulik-Sullivan et al., 2015; Zheng et al., 2017) indicate that the intercept of genetic correlations between urate and HDL-C was 1.057, indicating some degree of sample overlap. Lastly, some outcome data (e.g., T2DM and hypertension) were defined slightly differently across GWAS, making absolute standardization impossible based on summary-aggregated data.
Conclusion
Our data indicates a significant causal effect of genetically predicted urate on HDL-C and supports recent randomized controlled trial data suggesting that colchicine, a commonly prescribed drug for the treatment of gout, reduces the risk of CVD. Furthermore, our results suggest that only a small component (7%) of this association may be mediated by known risk factors, especially HDL, and that the majority of this effect is likely mediated by other, novel routes.
Data Availability Statement
Publicly available datasets were analyzed in this study. This data can be found here: https://www.mrbase.org.
Ethics Statement
This study involving human participants was reviewed and approved by Institutional Review Board of the Faculty of Medicine, Ramathibodi Hospital, Mahidol University. Written informed consent for participation was not required for this study in accordance with the national legislation and the institutional requirements.
Author Contributions
TL designed the study, performed data acquisition, analysis, interpretation, and drafted the manuscript. SR performed data acquisition and analysis. BO, GJM, and JA performed data interpretation. AT designed the study and performed data interpretation. All authors read and approved the final manuscript.
Conflict of Interest
The authors declare that the research was conducted in the absence of any commercial or financial relationships that could be construed as a potential conflict of interest.
Acknowledgments
We thank the MRC-IEU who developed the MR-Base platform, the GUGC, the CKDgen consortium, the DIAGRAM consortium, the GIANT consortium, the GLGC, the MAGIC, and the MRC-IEU UK Biobank GWAS pipeline for providing the summary data used in this study. This work is a part of the thesis of TL in Doctor of Philosophy Program in Clinical Epidemiology, Department of Clinical Epidemiology and Biostatistics, Faculty of Medicine Ramathibodi Hospital and Faculty of Graduate Studies, Mahidol University.
Supplementary Material
The Supplementary Material for this article can be found online at: https://www.frontiersin.org/articles/10.3389/fgene.2021.687279/full#supplementary-material
References
Allara, E., Morani, G., Carter, P., Gkatzionis, A., Zuber, V., Foley, C. N., et al. (2019). Genetic determinants of lipids and cardiovascular disease outcomes: a wide-angled mendelian randomization investigation. Circulat. Genomic Precision Med. 12:e002711. doi: 10.1161/circgen.119.002711
Babio, N., Martínez-González, M. A., Estruch, R., Wärnberg, J., Recondo, J., Ortega-Calvo, M., et al. (2015). Associations between serum uric acid concentrations and metabolic syndrome and its components in the PREDIMED study. Nutr. Metab. Cardiovasc. Dis. 25, 173–180. doi: 10.1016/j.numecd.2014.10.006
Bowden, J., Davey Smith, G., and Burgess, S. (2015). Mendelian randomization with invalid instruments: effect estimation and bias detection through egger regression. Int. J. Epidemiol. 44, 512–525. doi: 10.1093/ije/dyv080
Bowden, J., Davey Smith, G., Haycock, P. C., and Burgess, S. (2016). Consistent estimation in mendelian randomization with some invalid instruments using a weighted median estimator. Genet. Epidemiol. 40, 304–314. doi: 10.1002/gepi.21965
Bowden, R. G., Shelmadine, B. D., Moreillon, J. J., Deike, E., Griggs, J. O., and Wilson, R. L. (2013). Effects of uric acid on lipid levels in CKD patients in a randomized controlled trial. Cardiol. Res. 4, 56–63. doi: 10.4021/cr263w
Bulik-Sullivan, B., Finucane, H. K., Anttila, V., Gusev, A., Day, F. R., Loh, P. R., et al. (2015). An atlas of genetic correlations across human diseases and traits. Nat. Genet. 47, 1236–1241. doi: 10.1038/ng.3406
Burgess, S., Butterworth, A., and Thompson, S. G. (2013). Mendelian randomization analysis with multiple genetic variants using summarized data. Genet. Epidemiol. 37, 658–665. doi: 10.1002/gepi.21758
Burgess, S., Daniel, R. M., Butterworth, A. S., and Thompson, S. G. (2015). Network Mendelian randomization: using genetic variants as instrumental variables to investigate mediation in causal pathways. Int. J. Epidemiol. 44, 484–495. doi: 10.1093/ije/dyu176
Burgess, S., Davey Smith, G., Davies, N., Dudbridge, F., Gill, D., Glymour, M., et al. (2020a). Guidelines for performing mendelian randomization investigations [version 2; peer review: 2 approved]. Wellcome Open Res. 4:186. doi: 10.12688/wellcomeopenres.15555.2
Burgess, S., Foley, C. N., Allara, E., Staley, J. R., and Howson, J. M. M. (2020b). A robust and efficient method for mendelian randomization with hundreds of genetic variants. Nat. Commun. 11:376. doi: 10.1038/s41467-019-14156-4
Chen, S., Chen, D., Yang, H., Wang, X., Wang, J., and Xu, C. (2020a). Uric acid induced hepatocytes lipid accumulation through regulation of miR-149-5p/FGF21 axis. BMC Gastroenterol. 20:39. doi: 10.1186/s12876-020-01189-z
Chen, S., Yang, H., Chen, Y., Wang, J., Xu, L., Miao, M., et al. (2020b). Association between serum uric acid levels and dyslipidemia in Chinese adults: a cross-sectional study and further meta-analysis. Medicine (Baltimore) 99:e19088. doi: 10.1097/md.0000000000019088
Davey Smith, G., Davies, N. M., Dimou, N., Egger, M., Gallo, V., Golub, R., et al. (2019). STROBE-MR: guidelines for strengthening the reporting of Mendelian randomization studies. PeerJ Preprints 7:e27857v1.
Efstathiadou, A., Gill, D., McGrane, F., Quinn, T., and Dawson, J. (2019). Genetically determined uric acid and the risk of cardiovascular and neurovascular diseases: a mendelian randomization study of outcomes investigated in randomized trials. J. Am. Heart Assoc. 8:e012738. doi: 10.1161/jaha.119.012738
Elsworth, B. L., Mitchell, R., Raistrick, C. A., Paternoster, L., Hemani, G., and Gaunt, T. R. (2017). MRC IEU UK Biobank GWAS Pipeline Version 1. Bristol: University of Bristol.
Feig, D. I., Kang, D. H., and Johnson, R. J. (2008). Uric acid and cardiovascular risk. N Engl. J. Med. 359, 1811–1821. doi: 10.1056/NEJMra0800885
Goek, O. N., Köttgen, A., Hoogeveen, R. C., Ballantyne, C. M., Coresh, J., and Astor, B. C. (2012). Association of apolipoprotein A1 and B with kidney function and chronic kidney disease in two multiethnic population samples. Nephrol. Dial. Transplant. 27, 2839–2847. doi: 10.1093/ndt/gfr795
Gonçalves, J. P., Oliveira, A., Severo, M., Santos, A. C., and Lopes, C. (2012). Cross-sectional and longitudinal associations between serum uric acid and metabolic syndrome. Endocrine 41, 450–457. doi: 10.1007/s12020-012-9629-8
Grayson, P. C., Kim, S. Y., LaValley, M., and Choi, H. K. (2011). Hyperuricemia and incident hypertension: a systematic review and meta-analysis. Arthritis Care Res. (Hoboken) 63, 102–110. doi: 10.1002/acr.20344
Hartwig, F. P., Davey Smith, G., and Bowden, J. (2017). Robust inference in summary data Mendelian randomization via the zero modal pleiotropy assumption. Int. J. Epidemiol. 46, 1985–1998. doi: 10.1093/ije/dyx102
Hemani, G., Zheng, J., Elsworth, B., Wade, K. H., Haberland, V., Baird, D., et al. (2018). The MR-Base platform supports systematic causal inference across the human phenome. eLife 7:e34408. doi: 10.7554/eLife.34408
Jordan, D. M., Choi, H. K., Verbanck, M., Topless, R., Won, H. H., Nadkarni, G., et al. (2019). No causal effects of serum urate levels on the risk of chronic kidney disease: a Mendelian randomization study. PLoS Med. 16:e1002725. doi: 10.1371/journal.pmed.1002725
Keenan, T., Zhao, W., Rasheed, A., Ho, W. K., Malik, R., Felix, J. F., et al. (2016). Causal assessment of serum urate levels in cardiometabolic diseases through a mendelian randomization study. J. Am. Coll. Cardiol. 67, 407–416. doi: 10.1016/j.jacc.2015.10.086
Kharitonenkov, A., Wroblewski, V. J., Koester, A., Chen, Y. F., Clutinger, C. K., Tigno, X. T., et al. (2007). The metabolic state of diabetic monkeys is regulated by fibroblast growth factor-21. Endocrinology 148, 774–781. doi: 10.1210/en.2006-1168
Kim, S. Y., Guevara, J. P., Kim, K. M., Choi, H. K., Heitjan, D. F., and Albert, D. A. (2010). Hyperuricemia and coronary heart disease: a systematic review and meta-analysis. Arthritis Care Res. (Hoboken) 62, 170–180. doi: 10.1002/acr.20065
Kleber, M. E., Delgado, G., Grammer, T. B., Silbernagel, G., Huang, J., Krämer, B. K., et al. (2015). Uric acid and cardiovascular events: a mendelian randomization study. J. Am. Soc. Nephrol. 26, 2831–2838. doi: 10.1681/asn.2014070660
Kodama, S., Saito, K., Yachi, Y., Asumi, M., Sugawara, A., Totsuka, K., et al. (2009). Association between serum uric acid and development of type 2 diabetes. Diabetes Care 32, 1737–1742. doi: 10.2337/dc09-0288
Kottgen, A., Albrecht, E., Teumer, A., Vitart, V., Krumsiek, J., Hundertmark, C., et al. (2013). Genome-wide association analyses identify 18 new loci associated with serum urate concentrations. Nat. Genet. 45, 145–154. doi: 10.1038/ng.2500
Lawlor, D. A., Harbord, R. M., Sterne, J. A., Timpson, N., and Davey Smith, G. (2008). Mendelian randomization: using genes as instruments for making causal inferences in epidemiology. Stat. Med. 27, 1133–1163. doi: 10.1002/sim.3034
Li, L., Yang, C., Zhao, Y., Zeng, X., Liu, F., and Fu, P. (2014). Is hyperuricemia an independent risk factor for new-onset chronic kidney disease?: A systematic review and meta-analysis based on observational cohort studies. BMC Nephrol. 15:122. doi: 10.1186/1471-2369-15-122
Locke, A. E., Kahali, B., Berndt, S. I., Justice, A. E., Pers, T. H., Day, F. R., et al. (2015). Genetic studies of body mass index yield new insights for obesity biology. Nature 518, 197–206. doi: 10.1038/nature14177
Manning, A. K., Hivert, M. F., Scott, R. A., Grimsby, J. L., Bouatia-Naji, N., Chen, H., et al. (2012). A genome-wide approach accounting for body mass index identifies genetic variants influencing fasting glycemic traits and insulin resistance. Nat. Genet. 44, 659–669. doi: 10.1038/ng.2274
Merriman, T. (2017). Genomic influences on hyperuricemia and gout. Rheum Dis. Clin. North Am. 43, 389–399. doi: 10.1016/j.rdc.2017.04.004
Morris, A. P., Voight, B. F., Teslovich, T. M., Ferreira, T., Segre, A. V., Steinthorsdottir, V., et al. (2012). Large-scale association analysis provides insights into the genetic architecture and pathophysiology of type 2 diabetes. Nat. Genet. 44, 981–990. doi: 10.1038/ng.2383
Nakagawa, T., Hu, H., Zharikov, S., Tuttle, K. R., Short, R. A., Glushakova, O., et al. (2006). A causal role for uric acid in fructose-induced metabolic syndrome. Am. J. Physiol. Renal Physiol. 290, F625–F631. doi: 10.1152/ajprenal.00140.2005
Nakagomi, A., Saiki, Y., Noma, S., Kohashi, K., Morisawa, T., Kosugi, M., et al. (2015). Effects of febuxostat and allopurinol on the inflammation and cardiac function in chronic heart failure patients with hyperuricemia. IJC Metab. Endocrine 8, 46–55. doi: 10.1016/j.ijcme.2015.07.001
Nidorf, S. M., Fiolet, A. T. L., Mosterd, A., Eikelboom, J. W., Schut, A., Opstal, T. S. J., et al. (2020). Colchicine in patients with chronic coronary disease. N Engl. J. Med. 383, 1838–1847. doi: 10.1056/NEJMoa2021372
Opstal, T. S. J., Hoogeveen, R. M., Fiolet, A. T. L., Silvis, M. J. M., The, S. H. K., Bax, W. A., et al. (2020). Colchicine attenuates inflammation beyond the inflammasome in chronic coronary artery disease: a LoDoCo2 proteomic substudy. Circulation 142, 1996–1998. doi: 10.1161/circulationaha.120.050560
Pattaro, C., Teumer, A., Gorski, M., Chu, A. Y., Li, M., Mijatovic, V., et al. (2016). Genetic associations at 53 loci highlight cell types and biological pathways relevant for kidney function. Nat. Commun. 7:10023. doi: 10.1038/ncomms10023
Peng, T. C., Wang, C. C., Kao, T. W., Chan, J. Y., Yang, Y. H., Chang, Y. W., et al. (2015). Relationship between hyperuricemia and lipid profiles in US adults. BioMed Res. Int. 2015:127596. doi: 10.1155/2015/127596
Sluijs, I., Holmes, M. V., van der Schouw, Y. T., Beulens, J. W., Asselbergs, F. W., Huerta, J. M., et al. (2015). A mendelian randomization study of circulating uric acid and type 2 diabetes. Diabetes Metab. Res. Rev. 64, 3028–3036. doi: 10.2337/db14-0742
Tardif, J.-C., Kouz, S., Waters, D. D., Bertrand, O. F., Diaz, R., Maggioni, A. P., et al. (2019). Efficacy and safety of low-dose colchicine after myocardial infarction. N. Engl. J. Med. 381, 2497–2505. doi: 10.1056/NEJMoa1912388
Vaxillaire, M., Cavalcanti-Proença, C., Dechaume, A., Tichet, J., Marre, M., Balkau, B., et al. (2008). The common P446L polymorphism in GCKR inversely modulates fasting glucose and triglyceride levels and reduces type 2 diabetes risk in the DESIR prospective general French population. Diabetes Metab. Res. Rev. 57, 2253–2257. doi: 10.2337/db07-1807
Verbanck, M., Chen, C. Y., Neale, B., and Do, R. (2018). Detection of widespread horizontal pleiotropy in causal relationships inferred from Mendelian randomization between complex traits and diseases. Nat. Genet. 50, 693–698. doi: 10.1038/s41588-018-0099-7
White, J., Sofat, R., Hemani, G., Shah, T., Engmann, J., Dale, C., et al. (2016). Plasma urate concentration and risk of coronary heart disease: a Mendelian randomisation analysis. Lancet Diab. Endocrinol. 4, 327–336. doi: 10.1016/s2213-8587(15)00386-1
Willer, C. J., Schmidt, E. M., Sengupta, S., Peloso, G. M., Gustafsson, S., Kanoni, S., et al. (2013). Discovery and refinement of loci associated with lipid levels. Nat. Genet. 45, 1274–1283. doi: 10.1038/ng.2797
World Health Organization (2017). Cardiovascular Diseases (CVDs). Geneva: World Health Organization.
Xie, T., and Leung, P. S. (2017). Fibroblast growth factor 21: a regulator of metabolic disease and health span. Am. J. Physiol. Endocrinol. Metab. 313, E292–E302. doi: 10.1152/ajpendo.00101.2017
Yang, Q., Kottgen, A., Dehghan, A., Smith, A. V., Glazer, N. L., Chen, M. H., et al. (2010). Multiple genetic loci influence serum urate levels and their relationship with gout and cardiovascular disease risk factors. Circ. Cardiovasc. Genet. 3, 523–530. doi: 10.1161/circgenetics.109.934455
Yuan, H., Yu, C., Li, X., Sun, L., Zhu, X., Zhao, C., et al. (2015). Serum URIC ACID levels and risk of metabolic syndrome: a dose-response meta-analysis of prospective studies. J. Clin. Endocrinol. Metab. 100, 4198–4207. doi: 10.1210/jc.2015-2527
Zheng, J., Erzurumluoglu, A. M., Elsworth, B. L., Kemp, J. P., Howe, L., Haycock, P. C., et al. (2017). LD Hub: a centralized database and web interface to perform LD score regression that maximizes the potential of summary level GWAS data for SNP heritability and genetic correlation analysis. Bioinformatics 33, 272–279. doi: 10.1093/bioinformatics/btw613
Keywords: cardiovascular risk factor, instrumental variable, Mendelian Randomization, urate, urate transporter gene
Citation: Lukkunaprasit T, Rattanasiri S, Ongphiphadhanakul B, McKay GJ, Attia J and Thakkinstian A (2021) Causal Associations of Urate With Cardiovascular Risk Factors: Two-Sample Mendelian Randomization. Front. Genet. 12:687279. doi: 10.3389/fgene.2021.687279
Received: 29 March 2021; Accepted: 10 June 2021;
Published: 08 July 2021.
Edited by:
Guolian Kang, St. Jude Children’s Research Hospital, United StatesReviewed by:
Ping Zeng, Xuzhou Medical University, ChinaZhongshang Yuan, Shandong University, China
Copyright © 2021 Lukkunaprasit, Rattanasiri, Ongphiphadhanakul, McKay, Attia and Thakkinstian. This is an open-access article distributed under the terms of the Creative Commons Attribution License (CC BY). The use, distribution or reproduction in other forums is permitted, provided the original author(s) and the copyright owner(s) are credited and that the original publication in this journal is cited, in accordance with accepted academic practice. No use, distribution or reproduction is permitted which does not comply with these terms.
*Correspondence: Sasivimol Rattanasiri, c2FzaXZpbW9sLnJhdEBtYWhpZG9sLmVkdQ==; Ammarin Thakkinstian, YW1tYXJpbi50aGFAbWFoaWRvbC5hYy50aA==