- 1School of Public Health, Li Ka Shing Faculty of Medicine, The University of Hong Kong, Hong Kong, China
- 2Graduate School of Public Health and Health Policy, City University of New York, New York, NY, United States
Background: Immune system functioning is relevant to vulnerability to coronavirus disease (COVID-19). Cytokines are important to immunity. To further elucidate the role of the immune system in COVID-19, we used Mendelian randomization (MR) to assess comprehensively and bi-directionally the role of cytokines in COVID-19.
Methods: We assessed primarily whether genetically different levels of 41 cytokines affected risk of any COVID-19 (laboratory confirmed, physician confirmed or self-reported, 36,590 cases, 1,668,938 controls), and conversely if genetic risk of liability to any COVID-19 affected these cytokines (n ≤ 8293) using the most recent genome-wide association studies. We obtained inverse variance weighting (IVW) estimates, conducted sensitivity analyses and used a Benjamini-Hochberg correction to account for multiple comparisons. We also assessed whether any findings were evident for hospitalized COVID-19 (hospitalized laboratory confirmed, 12,888 cases, 1,295,966 controls).
Results: Macrophage inflammatory protein-1β (MIP1b; more commonly known as Chemokine (C-C motif) ligands 4 (CCL4) was inversely associated with COVID-19 [odds ratio (OR) 0.97 per SD, 95% confidence interval (CI) 0.96–0.99] but not after adjustment for multiple comparisons. This finding replicated for hospitalized COVID-19 (OR 0.93, 95% CI 0.89–0.98). Liability to any COVID-19 was nominally associated with several cytokines, such as granulocyte colony-stimulating factor (GCSF) and hepatocyte growth factor (HGF) but not after correction.
Conclusion: A crucial element of immune response to infection (CCL4) was related to COVID-19, whether it is a target of intervention to prevent COVID-19 warrants further investigation.
Background
Coronavirus disease (COVID-19) caused by the severe acute respiratory syndrome coronavirus 2 (SARS-CoV-2) is a serious global pandemic1. Vulnerability to and response to infection with SARS-CoV-2 varies widely2 underscoring the role of the immune response, which is regulated by cytokines (Kany et al., 2019). A “cytokine storm” may occur in severe COVID-19 and results in worse prognosis (Chen et al., 2020; Huang et al., 2020; Sun et al., 2020). Several trials of anti-inflammatory medications, such as tocilizumab and sarilumab targeting the interleukin (IL)-6 receptor, and anakinra, i.e., targeting IL-1 receptor antagonism, are underway3 or completed4,5,6,7,8 (Stone et al., 2020; Hermine et al., 2021; Salvarani et al., 2021). A recent large trial suggested mortality benefits of tocilizumab in some groups of hospitalized patients7, consistent with a previous Mendelian randomization (MR) study suggesting IL-6 blockade might be helpful in COVID-19 hospitalization (Bovijn et al., 2020). In contrast, the wider role of cytokines in COVID-19 has been less investigated, using MR to compare COVID-19 outcomes in people with genetically predicted different levels of cytokines. MR gives less-confounded estimates by making use of the randomization of genetic variants at conception, which is similar to the randomization in randomized controlled trials (RCTs), and has foreshadowed findings from trials9 (Lawlor et al., 2008). MR has previously been used successfully to investigate potential targets for COVID-19 prevention (Leong et al., 2020; Ponsford et al., 2020). Here, we used MR to investigate whether any of 41 cytokines (Ahola-Olli et al., 2017) were associated any COVID-19, and whether liability to COVID-19 affected any of these cytokines. We also assessed whether any findings for any COVID-19 replicated for hospitalized COVID-19.
Materials and Methods
This is a two-sample bi-directional MR study design, using summary statistics from the latest available genome-wide association study (GWAS) for cytokines and COVID-19. MR is an instrumental variable analysis using genetic instruments, which is less confounded due to randomization of genetic variants at conception. MR relies on three assumptions, i.e., the genetic instruments are associated with the exposure (relevance), the genetic instruments are not linked with confounders of exposure on outcome (independence), and the genetic instruments are linked to the outcome only via the exposure (exclusion-restriction) (Davies et al., 2018).
Data Sources
Genetic Associations With Cytokines
Genetic associations with 41 plasma/serum cytokines (in standard deviations) were obtained from a meta-analysis of up to 8,293 individuals from two studies in Finland, the Cardiovascular Risk in Young Finns Study (n = 2,019, mean age 37.4 years in men, 37.5 in women) and the FINRISK survey (n = 6,313, survey 1997: mean age 48.3 years in men, 47.3 in women; survey 2002: 60.4 in men, 60.1 in women) (Ahola-Olli et al., 2017). Genetic associations were adjusted for age, sex, body mass index and the first 10 genetic principal components, and used genomic control to correct for population stratification and cryptic relatedness (Ahola-Olli et al., 2017).
Genetic Associations With COVID-19
Genetic associations with any COVID-19 compared to the population (36,590 cases and 1,668,938 controls) were obtained from the COVID19-hg GWAS meta-analyses round five summary statistics (updated January 2021, leaving out 23andMe) initiated by the COVID-19 Host Genetics Initiative (COVID-19 Host Genetics Initiative, 2020). Any COVID-19 was defined as laboratory confirmation of SARS-CoV-2 infection (RNA and/or serology based), EHR/ICD coding/physician confirmed COVID-19, or self-reported COVID-19 positive (36,590 cases compared to 1,668,938 population controls). Genetic associations with hospitalized COVID-19 (hospitalized laboratory confirmed) (12,888 cases) compared to the population (1,295,966 controls) were obtained from the same study. Summary statistics leaving out the UK Biobank [any COVID-19 (42,557 cases and 1,424,707 controls), hospitalized COVID-19 (11,829 cases and 1,725,210 controls)] were used as sensitivity analyses as the study had fewer genetic variants. The participants are largely drawn from on-going cohort studies (Supplementary Table 1), mostly from Europe and the United States. The analyses controlled for age, sex, and ancestry (COVID-19 Host Genetics Initiative, 2020).
Mendelian Randomization Analysis
Selection of Genetic Predictors
We used single-nucleotide polymorphisms (SNPs) strongly (P < 5 × 10–8) and independently (r2 < 0.05) associated with the exposures (cytokine or the COVID-19 phenotype) as genetic predictors. Given such genetic predictors were not available for all exposures, we also used a less stringent selection criteria (P < 5 × 10–6) for exposures without predictors at genome-wide significance. Potentially palindromic SNPs (allele pairs A/T or G/C) were removed before SNP selection because allele frequency is not available for the cytokine GWAS precluding unequivocal allele alignment across both studies. Independent genetic predictors (r2 < 0.05) available for both cytokines and COVID-19 were used in the MR analysis.
Statistical Analysis
The F-statistic was used as an indicator of instrument strength, obtained by averaging the SNP specific F-statistics (square of beta for exposure divided by its variance) (Bowden et al., 2016b). To test independence, we checked whether any of the genetic instruments were associated with several common potential confounders including tobacco smoking status, frequency of alcohol intake and frequency of walking at genome-wide significance, using UK Biobank genetic summary associations10.
Estimates were obtained by meta-analyzing SNP-specific Wald estimates (genetic association with outcome divided by association with exposure) using inverse variance weighting (IVW) with fixed (≤3 SNPs) or multiplicative random effects (4 + SNPs), which assumes balanced pleiotropy (Burgess et al., 2013). Given we assessed associations primarily between cytokines and any COVID-19 and the two COVID-19 phenotypes are correlated, we used a Benjamini-Hochberg false discovery rate (FDR)-control to correct for multiple hypotheses testing for 41 cytokines versus each COVID-19 phenotype (Benjamini and Hochberg, 1995).
Sensitivity Analysis
MR-Egger was used to identify potentially invalid estimates from IVW (indicated by a non-zero intercept), although it has less statistical power (Bowden et al., 2015). Weighted median (WM) gives robust estimates even when some invalid instruments (<50% weight) are used (Bowden et al., 2016a).
Statistical analyses were conducted using R version 3.6.2 (The R Foundation for Statistical Computing, Vienna, Austria). The “ld_clump” function (“ieugwasr” package) was used to select the independent genetic variants. The MendelianRandomization R package was used to obtain MR estimates. This study only uses published or publicly available data. Ethical approval for each of the studies included in the investigation can be found in the original publications (including informed consent from each participant).
Results
Genetic Predictors for Circulating Cytokines and COVID-19
Of the 41 circulating cytokines 19 had genome-wide significant predictors for any COVID-19 (Supplementary Table 2), all the 41 cytokines considered had 2 or more non-palindromic independent genetic predictors with p-values smaller than 5 × 10–6. The F-statistics ranged from 18.7 to 169.8 (Supplementary Table 2). Both COVID-19 phenotypes considered had 2 or more non-palindromic independent genetic predictors with p-value < 5 × 10–8 (Supplementary Table 3). F-statistics ranged from 60.5 to 64.8 (Supplementary Table 3).
Genetically Predicted Circulating Cytokines on Any COVID-19
Macrophage inflammatory protein-1β (MIP1b) was nominally inversely associated with any COVID-19 using IVW and WM but not after correcting for multiple comparisons (Figure 1 and Supplementary Table 4). These nominal associations replicated for hospitalized COVID-19 using all methods but not after correcting for multiple comparisons (Supplementary Figure 1 and Supplementary Table 5). MIP1b was also found associated with very severe respiratory confirmed COVID-19 (death or respiratory support, 5,582 cases versus 7,09,010 controls) (OR 0.92, 95% CI 0.84–1.00). The other cytokines considered, including IL-6, were not associated with either COVID-19 phenotype (any or hospitalized). In sensitivity analysis using genetic associations leaving out the UK Biobank rather than 23andMe, monocyte chemotactic protein-1 (MCP1; CCL2) was nominally positively associated with any COVID-19 (Supplementary Table 6) and MIP1b was also nominally inversely associated with hospitalized COVID-19 (Supplementary Table 7).
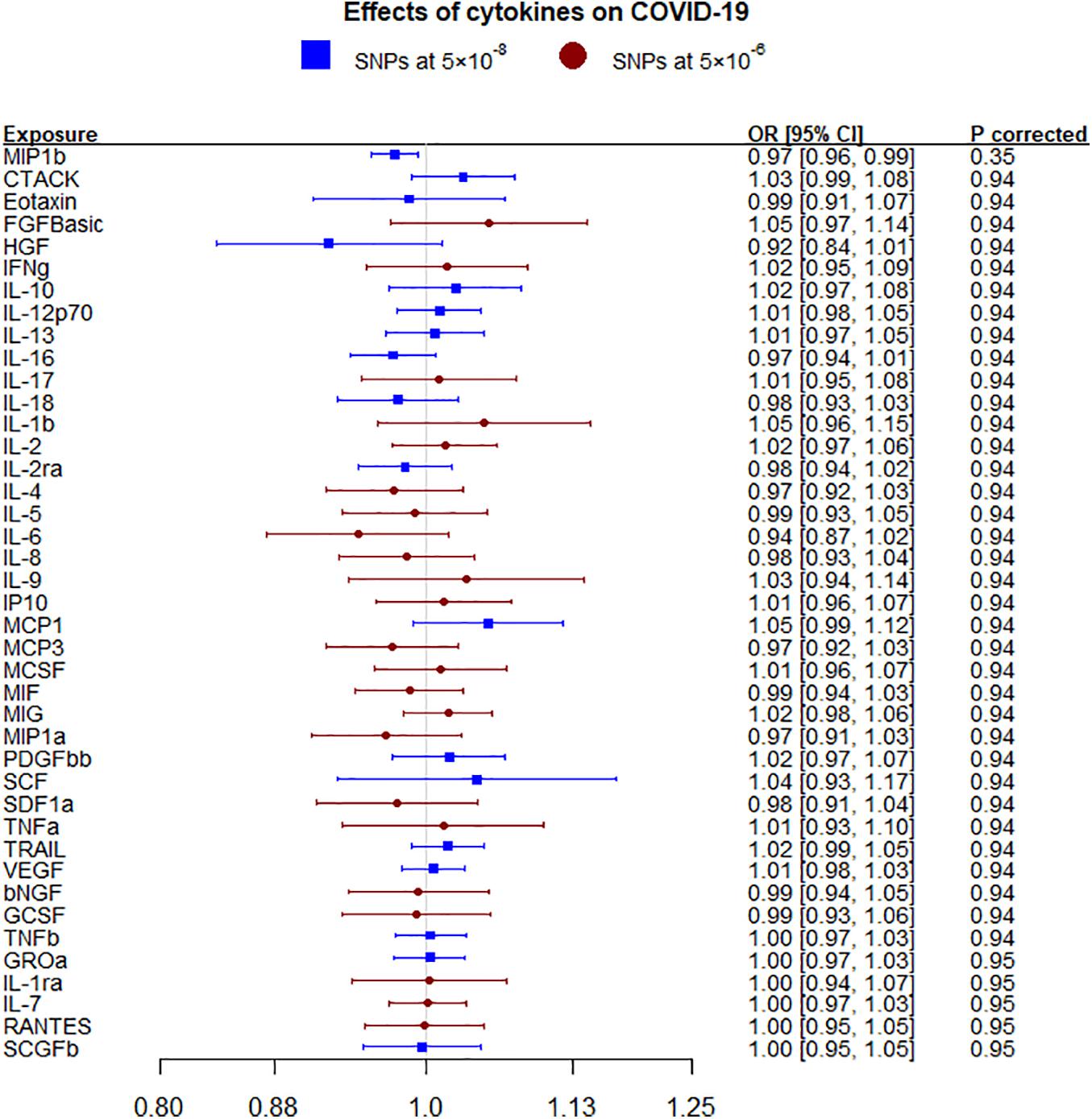
Figure 1. Effects of 41 circulating cytokines on COVID-19 using inverse variance weighting with p-values using a Benjamini-Hochberg correction.
Genetically Predicted Liability to COVID-19 on Circulating Cytokines
Liability to any COVID-19 was nominally inversely associated with granulocyte colony-stimulating factor (GCSF), hepatocyte growth factor (HGF), IL-2 receptor alpha subunit (IL2ra), macrophage colony-stimulating factor (MCSF), tumor necrosis factor-beta (TNFb) and TNF-related apoptosis inducing ligand (TRAIL) but positively associated with monocyte chemotactic protein-3 (MCP3) but not after correction for multiple comparisons (Figure 2 and Supplementary Table 8). The association of liability to hospitalized COVID-19 with IL2ra and TNFb were similar to those for liability to any COVID-19 (Supplementary Figure 2 and Supplementary Table 9). Most results were similar using genetic associations leaving out the UK Biobank rather than 23andMe (Supplementary Tables 10, 11).
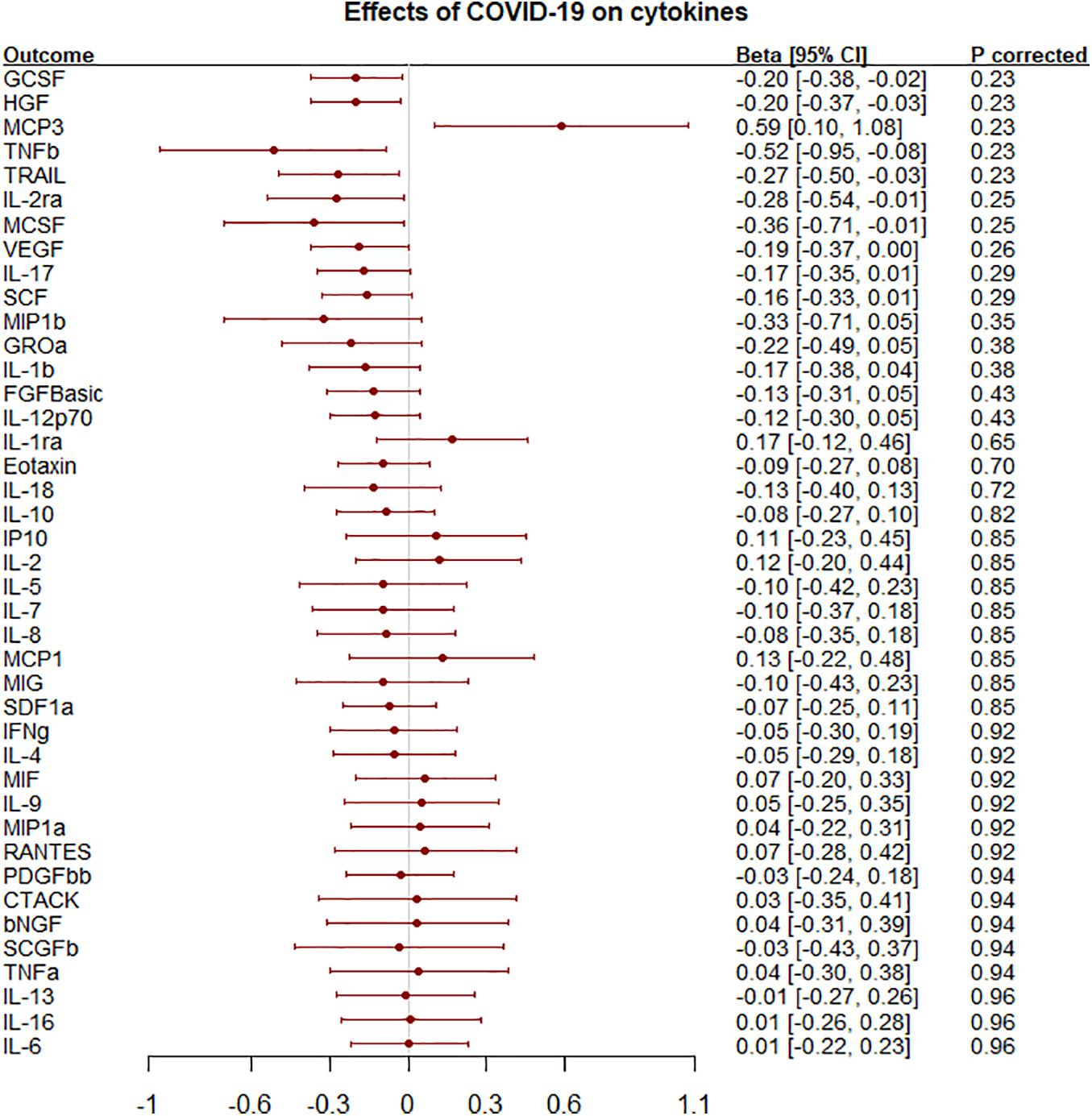
Figure 2. Effects of liability to COVID-19 on 41 circulating cytokines using inverse variance weighting with p-values using a Benjamini-Hochberg correction.
Discussion
In this bi-directional MR study of circulating cytokines and COVID-19, we found some associations in both directions. For associations of cytokines with COVID-19, lower MIP1b was nominally and consistently associated with COVID-19 phenotypes, which has rarely been studied previously. In the other direction, we found liability to COVID-19 was inversely associated with several cytokines, such as GCSF and HGF.
Few previous studies have investigated the role of MIP1b in COVID-19, although higher MIP1b in patients than controls has been observed (De Biasi et al., 2020; Hachim et al., 2020). This study showed internally consistent associations of MIP1b with COVID-19, indicating MIP1b may play a protective role in both COVID-19 vulnerability and hospitalization. MIP1b is a chemoattractant for several immune cells, particularly T cells (Schall et al., 1993; Tedla et al., 1998; Bystry et al., 2001), whose function is increasingly being recognized in COVID-19 (Chen and John Wherry, 2020). T cells are pivotal to the anti-viral immune response, and may be low and/or functionally exhausted in COVID-19 (Mathew et al., 2020) especially severe COVID-19 (Diao et al., 2020; Giamarellos-Bourboulis et al., 2020). In this study, we also found liability to COVID-19 might reduce MIP1b levels using MR-Egger but not any other methods, whether it is relevant in the T cell exhaustion in COVID-19 needs further investigation. Given the cytokines were obtained from studies conducted before the COVID-19 pandemic, any associations of liability to COVID-19 with cytokines indicate that people more vulnerable to COVID-19 have a somewhat different cytokine profile possibly reflecting differences in immune functioning.
Although the evidence concerning the role of MIP1b in COVID-19 is inevitably limited, MIP1b has been extensively investigated in other viral diseases, such as HIV (Cocchi et al., 2000) and hepatitis A (Sung et al., 2017). MIP1b is a ligand of CCR5, which has also been extensively investigated in the context of HIV (Qi et al., 2020). Relevance of IL-6 to specifically COVID-19 has been more intensively investigated. Observationally associations of IL-6 with COVID-19 have been seen (Chen et al., 2020; Gao et al., 2020; Ruan et al., 2020). Genetically mimicking IL6R blockade using genetic instruments from the IL6R gene strongly associated with c-reactive protein was associated with lower risk of hospitalized COVID-19 (Bovijn et al., 2020), but whether these associations are due to IL-6, IL-6R, or pleiotropic effects via other mechanisms is unclear. Genetically mimicking IL6R blockers, such as tocilizumab, suggest a very wide range of effects (Rosa et al., 2019).
MR studies rely on three important assumptions, i.e., relevance, independence and exclusion restriction. We used several methods to detect violation of these assumptions. First, to ensure relevance we selected genetic predictors strongly (genome-wide significant) associated with the exposures, but also included less significant predictors 5 × 10–6 for some exposures because predictors at genome-wide significance were not available for all. The F-statistics did not indicate weak instruments. Second, to check independence, we assessed associations of the genetic predictors with several common confounders, and found no associations with these confounders (Supplementary Tables 12–17), except one SNP rs3748034 predicting HGF was associated with alcohol intake. Third, to test the exclusion restriction assumption, we used sensitivity analyses with different assumptions including MR-Egger and WM to detect pleiotropy and give robust estimates even if invalid SNPs are included, but might lack of power (such as MR-Egger).
This large bi-directional MR study used the largest available GWASs for cytokines and COVID-19. However, limitations still exist. First, we assumed genetic associations in one sample could be replicated in the other sample (Burgess et al., 2015). Both GWAS mainly concern people of European ancestry. Second, we assumed linear associations in this study, while the associations of cytokines and COVID-19 could be complex and change over time. Third, given most participants were from Europe and the United States, whether the associations are applicable to other populations, such as Asians, is uncertain. However, causal factors should be consistent across different populations although the underlying mechanism might not always be relevant11. Fourth, covariable adjusted summary statistics might bias the MR results in some situations (Hartwig et al., 2019). Here, the summary statistics for cytokines have adjusted for body mass index, which is the common cause of COVID-19 (Mark et al., 2020) and survival (Sun et al., 2019). As such, the adjustment is more likely to correct for selection bias more than induce it (Schooling et al., 2020). Fifth, the case definition is essentially nested meaning the phenotypes are not completely independent. We focused on any COVID-19 to maximize power and comprehensiveness, particularly given COVID-19 can have long-term consequences even if the initial disease is not severe12. Sixth, the case definition for any COVID-19 reflects disease rather than infection with SARS-CoV-2, so our findings may represent the role of MIP1b in preventing disease on infection or in protecting against infection. Seventh, there were suggestive associations of liability to COVID-19 with MIP1b using MR-Egger. We used Steiger filtering to indicate whether there were any invalid genetic instruments that predicted the outcomes more than the exposures by comparing the variance in exposures and outcomes explained by each genetic variant (r2). For the associations of MIP1b with COVID-19, Steiger filtering did not indicate any invalid genetic instruments. However, Steiger filtering did suggest two genetic instruments might be predictors of MIP1b more than of COVID-19. After removing them, the observed association of liability to COVID-19 with MIP1b was not evident using any method (Supplementary Table 18). Last, but not least, we did not consider the relations among cytokines and the role of the cytokine cascade in immune response.
Conclusion
This large bi-directional MR study suggests MIP1b may protect against any COVID-19 and also hospitalized COVID-19. MIP1b has previously been investigated as a target in other infectious diseases, whether it could be a potential target for prevention and treatment of COVID-19 as well as its exact role in immune response needs further investigation.
Data Availability Statement
The datasets generated and/or analysed during the current study are available in Supplementary Files.
Ethics Statement
This study only uses published or publicly available data. Ethical approval for each of the studies included in the investigation can be found in the original publications (including informed consent from each participant).
Author Contributions
CS and ML designed the study. ML conducted the analysis and drafted the manuscript. CS directed the analytic strategy and supervised the study from conception to completion. CY checked the data analyses. ML, CY, and CS revised the drafts of the manuscript. All authors contributed to the interpretation of the data and critically revising the manuscript.
Conflict of Interest
The authors declare that the research was conducted in the absence of any commercial or financial relationships that could be construed as a potential conflict of interest.
Acknowledgments
Data on COVID-19 have been contributed by the COVID-19 host genetics initiative and can be downloaded from https://www.covid19hg.org/results/. Data on cytokines have been contributed by The Computational Medicine Research Team.
Supplementary Material
The Supplementary Material for this article can be found online at: https://www.frontiersin.org/articles/10.3389/fgene.2021.680646/full#supplementary-material
Footnotes
- ^ https://www.who.int/emergencies/diseases/novel-coronavirus-2019/situation-reports
- ^ https://www.nidirect.gov.uk/articles/coronavirus-covid-19-definitions-clinically-extremely-vulnerable-and-vulnerable
- ^ https://clinicaltrials.gov/ct2/show/NCT04443881
- ^ https://www.reuters.com/article/us-health-coronavirus-roche-hldg/roche-rheumatoid-arthritis-drug-fails-to-help-covid-19-patients-in-italian-study-idUSKBN23O3GG
- ^ https://pharmaphorum.com/news/il-6-hypothesis-looking-shaky-as-kevzara-fails-covid-19-trial/
- ^ https://pharmaphorum.com/news/jury-still-out-on-il-6-drugs-in-covid-19-following-preliminary-trial-results/
- ^ https://www.roche.com/investors/updates/inv-update-2020-07-29.htm
- ^ https://www.roche.com/investors/updates/inv-update-2020-09-18.htm
- ^ https://pubmed.ncbi.nlm.nih.gov/28569269/
- ^ http://www.nealelab.is/uk-biobank/
- ^ https://pubmed.ncbi.nlm.nih.gov/31121303/
- ^ https://covid.joinzoe.com/post/long-covid
References
Ahola-Olli, A. V., Würtz, P., Havulinna, A. S., Aalto, K., Pitkänen, N., Lehtimäki, T., et al. (2017). Genome-wide Association Study Identifies 27 Loci Influencing Concentrations of Circulating Cytokines and Growth Factors. Am. J. Hum. Genet. 100, 40–50. doi: 10.1016/j.ajhg.2016.11.007
Benjamini, Y., and Hochberg, Y. (1995). Controlling the False Discovery Rate: a Practical and Powerful Approach to Multiple Testing. J. R. Stat. Soc. Series B 57, 289–300.
Bovijn, J., Lindgren, C. M., and Holmes, M. V. (2020). Genetic variants mimicking therapeutic inhibition of IL-6 receptor signaling and risk of COVID-19. Lancet Rheumatol. 2:e658–e659. doi: 10.1016/S2665-9913(20)30345-3
Bowden, J., Davey Smith, G., and Burgess, S. (2015). Mendelian randomization with invalid instruments: effect estimation and bias detection through Egger regression. Int. J. Epidemiol. 44, 512–525. doi: 10.1093/ije/dyv080
Bowden, J., Davey Smith, G., Haycock, P. C., and Burgess, S. (2016a). Consistent Estimation in Mendelian Randomization with Some Invalid Instruments Using a Weighted Median Estimator. Genet. Epidemiol. 40, 304–314. doi: 10.1002/gepi.21965
Bowden, J., Del Greco, M. F., Minelli, C., Davey Smith, G., Sheehan, N. A., and Thompson, J. R. (2016b). Assessing the suitability of summary data for two-sample Mendelian randomization analyses using MR-Egger regression: the role of the I2 statistic. Int. J. Epidemiol. 45, 1961–1974. doi: 10.1093/ije/dyw220
Burgess, S., Butterworth, A., and Thompson, S. G. (2013). Mendelian randomization analysis with multiple genetic variants using summarized data. Genet Epidemiol 37, 658–665. doi: 10.1002/gepi.21758
Burgess, S., Scott, R. A., Timpson, N. J., Davey Smith, G., and Thompson, S. G. (2015). Using published data in Mendelian randomization: a blueprint for efficient identification of causal risk factors. Eur. J. Epidemiol. 30, 543–552. doi: 10.1007/s10654-015-0011-z
Bystry, R. S., Aluvihare, V., Welch, K. A., Kallikourdis, M., and Betz, A. G. (2001). B cells and professional APCs recruit regulatory T cells via CCL4. Nat. Immun. 2, 1126–1132. doi: 10.1038/ni735
Chen, G., Wu, D., Guo, W., Cao, Y., Huang, D., Wang, H., et al. (2020). Clinical and immunological features of severe and moderate coronavirus disease 2019. J. Clin. Invest. 130, 2620–2629. doi: 10.1172/jci137244
Chen, Z., and John Wherry, E. (2020). T cell responses in patients with COVID-19. Nat. Rev. Immunol. 20, 529–536. doi: 10.1038/s41577-020-0402-6
Cocchi, F., DeVico, A. L., Yarchoan, R., Redfield, R., Cleghorn, F., Blattner, W. A., et al. (2000). Higher macrophage inflammatory protein (MIP)-1alpha and MIP-1beta levels from CD8+ T cells are associated with asymptomatic HIV-1 infection. Proc. Natl. Acad. Sci. U. S. A. 97, 13812–13817. doi: 10.1073/pnas.240469997
COVID-19 Host Genetics Initiative. (2020). The COVID-19 Host Genetics Initiative, a global initiative to elucidate the role of host genetic factors in susceptibility and severity of the SARS-CoV-2 virus pandemic. Eur. J. Hum. Genet. 28, 715–718. doi: 10.1038/s41431-020-0636-6
Davies, N. M., Holmes, M. V., and Davey Smith, G. (2018). Reading Mendelian randomisation studies: a guide, glossary, and checklist for clinicians. BMJ 362:k601. doi: 10.1136/bmj.k601
De Biasi, S., Meschiari, M., Gibellini, L., Bellinazzi, C., Borella, R., Fidanza, L., et al. (2020). Marked T cell activation, senescence, exhaustion and skewing towards TH17 in patients with COVID-19 pneumonia. Nat. Commun. 11:3434. doi: 10.1038/s41467-020-17292-4
Diao, B., Wang, C., Tan, Y., Chen, X., Liu, Y., Ning, L., et al. (2020). Reduction and Functional Exhaustion of T Cells in Patients With Coronavirus Disease 2019 (COVID-19). Front. Immunol. 11:827. doi: 10.3389/fimmu.2020.00827
Gao, Y., Li, T., Han, M., Li, X., Wu, D., Xu, Y., et al. (2020). Diagnostic utility of clinical laboratory data determinations for patients with the severe COVID-19. J. Med. Virol. 92, 791–796. doi: 10.1002/jmv.25770
Giamarellos-Bourboulis, E. J., Netea, M. G., Rovina, N., Akinosoglou, K., Antoniadou, A., Antonakos, N., et al. (2020). Complex Immune Dysregulation in COVID-19 Patients with Severe Respiratory Failure. Cell Host Microbe 27, 992–1000.e3. doi: 10.1016/j.chom.2020.04.009
Hachim, M. Y., Hachim, I. Y., Naeem, K. B., Hannawi, H., Al Salmi, I., and Hannawi, S. (2020). C-C chemokine receptor type 5 links COVID-19, rheumatoid arthritis, and Hydroxychloroquine: in silico analysis. Transl. Med. Commun. 5:14. doi: 10.1186/s41231-020-00066-x
Hartwig, F. P., Tilling, K., Davey Smith, G., Lawlor, D. A., and Borges, M. C. (2019). Bias in two-sample Mendelian randomization by using covariable-adjusted summary associations. bioRxiv 816363. doi: 10.1101/816363
Hermine, O., Mariette, X., Tharaux, P.-L., Resche-Rigon, M., Porcher, R., Ravaud, P., et al. (2021). Effect of Tocilizumab vs Usual Care in Adults Hospitalized With COVID-19 and Moderate or Severe Pneumonia: a Randomized Clinical Trial. JAMA Intern. Med. 181, 32–40. doi: 10.1001/jamainternmed.2020.6820
Howrey, B., Goodwin, J. S., Eschbach, K., and Freeman, J. (2010). Lower stroke mortality among Hispanics: an exploration of potential methodological confounders. Med. Care 48, 534–539. doi: 10.1097/MLR.0b013e3181d686cf
Huang, C., Wang, Y., Li, X., Ren, L., Zhao, J., Hu, Y., et al. (2020). Clinical features of patients infected with 2019 novel coronavirus in Wuhan, China. Lancet 395, 497–506. doi: 10.1016/S0140-6736(20)30183-5
Kany, S., Vollrath, J. T., and Relja, B. (2019). Cytokines in Inflammatory Disease. Int. J. Mol. Sci. 20:6008. doi: 10.3390/ijms20236008
Lawlor, D. A., Harbord, R. M., Sterne, J. A., Timpson, N., and Davey Smith, G. (2008). Mendelian randomization: using genes as instruments for making causal inferences in epidemiology. Stat. Med. 27, 1133–1163. doi: 10.1002/sim.3034
Leong, A., Cole, J., Brenner, L. N., Meigs, J. B., Florez, J. C., and Mercader, J. M. (2020). Cardiometabolic Risk Factors for COVID-19 Susceptibility and Severity: a Mendelian Randomization Analysis. medRxiv. [Preprint]. doi: 10.1101/2020.08.26.20182709
Mark, P. J., Gkatzionis, A., Walker, V., Grant, A., Wootton, R. E., Moore, L. S. P., et al. (2020). Cardiometabolic traits, sepsis and severe covid-19 with respiratory failure: a Mendelian randomization investigation. medRxiv 142, 1791–1793. doi: 10.1101/2020.06.18.20134676
Mathew, D., Giles, J. R., Baxter, A. E., Oldridge, D. A., Greenplate, A. R., Wu, J. E., et al. (2020). Deep immune profiling of COVID-19 patients reveals distinct immunotypes with therapeutic implications. Science 369:eabc8511. doi: 10.1126/science.abc8511
Ponsford, M. J., Gkatzionis, A., Walker, V. M., Grant, A. J., Wootton, R. E., Moore, L. S. P., et al. (2020). Cardiometabolic Traits, Sepsis, and Severe COVID-19: a Mendelian Randomization Investigation. Circulation 142, 1791–1793. doi: 10.1161/circulationaha.120.050753
Qi, B., Fang, Q., Liu, S., Hou, W., Li, J., Huang, Y., et al. (2020). Advances of CCR5 antagonists: from small molecules to macromolecules. Eur. J. Med. Chem. 208:112819. doi: 10.1016/j.ejmech.2020.112819
Rosa, M., Chignon, A., Li, Z., Boulanger, M. C., Arsenault, B. J., Bossé, Y., et al. (2019). A Mendelian randomization study of IL6 signaling in cardiovascular diseases, immune-related disorders and longevity. NPJ Genom. Med. 4:23. doi: 10.1038/s41525-019-0097-4
Rothman, K. J., Gallacher, J. E., and Hatch, E. E. (2013). Why representativeness should be avoided. Int. J. Epidemiol. 42, 1012–1014. doi: 10.1093/ije/dys223
Ruan, Q., Yang, K., Wang, W., Jiang, L., and Song, J. (2020). Clinical predictors of mortality due to COVID-19 based on an analysis of data of 150 patients from Wuhan, China. Intensive Care Med. 46, 846–848. doi: 10.1007/s00134-020-05991-x
Salvarani, C., Dolci, G., Massari, M., Merlo, D. F., Cavuto, S., Savoldi, L., et al. (2021). Effect of Tocilizumab vs Standard Care on Clinical Worsening in Patients Hospitalized With COVID-19 Pneumonia: a Randomized Clinical Trial. JAMA Intern. Med. 181, 24–31. doi: 10.1001/jamainternmed.2020.6615
Schall, T. J., Bacon, K., Camp, R. D., Kaspari, J. W., and Goeddel, D. V. (1993). Human macrophage inflammatory protein alpha (MIP-1 alpha) and MIP-1 beta chemokines attract distinct populations of lymphocytes. J. Exp. Med. 177, 1821–1826. doi: 10.1084/jem.177.6.1821
Schooling, C. M., Lopez, P. M., Yang, Z., Zhao, J. V., Au Yeung, S. L., and Huang, J. V. (2020). Use of multivariable Mendelian randomization to address biases due to competing risk before recruitment. bioRxiv [Preprint]. doi: 10.1101/716621
Stone, J. H., Frigault, M. J., Serling-Boyd, N. J., Fernandes, A. D., Harvey, L., Foulkes, A. S., et al. (2020). Efficacy of Tocilizumab in Patients Hospitalized with Covid-19. New Eng. J. Med. 383, 2333–2344. doi: 10.1056/NEJMoa2028836
Sun, D., Li, H., Lu, X. X., Xiao, H., Ren, J., Zhang, F. R., et al. (2020). Clinical features of severe pediatric patients with coronavirus disease 2019 in Wuhan: a single center’s observational study. World J. Pediatr. 16, 251–259. doi: 10.1007/s12519-020-00354-4
Sun, Y.-Q., Burgess, S., Staley, J. R., Wood, A. M., Bell, S., Kaptoge, S. K., et al. (2019). Body mass index and all cause mortality in HUNT and UK Biobank studies: linear and non-linear mendelian randomisation analyses. BMJ 364:l1042. doi: 10.1136/bmj.l1042
Sung, P. S., Hong, S. H., Lee, J., Park, S. H., Yoon, S. K., Chung, W. J., et al. (2017). CXCL10 is produced in hepatitis A virus-infected cells in an IRF3-dependent but IFN-independent manner. Sci. Rep. 7:6387. doi: 10.1038/s41598-017-06784-x
Tedla, N., Wang, H. W., McNeil, H. P., Di Girolamo, N., Hampartzoumian, T., Wakefield, D., et al. (1998). Regulation of T lymphocyte trafficking into lymph nodes during an immune response by the chemokines macrophage inflammatory protein (MIP)-1 alpha and MIP-1 beta. J. Immunol. 161, 5663–5672.
Yetley, E. A., MacFarlane, A. J., Greene-Finestone, L. S., Garza, C., Ard, J. D., Atkinson, S. A., et al. (2017). Options for basing Dietary Reference Intakes (DRIs) on chronic disease endpoints: report from a joint US-/Canadian-sponsored working group. Am. J. Clin. Nutr. 105, 249S–285S. doi: 10.3945/ajcn.116.139097
Keywords: cytokine, coronavirus disease, Mendelian randomization, genetics, immune system
Citation: Li M, Yeung CHC and Schooling CM (2021) Circulating Cytokines and Coronavirus Disease: A Bi-Directional Mendelian Randomization Study. Front. Genet. 12:680646. doi: 10.3389/fgene.2021.680646
Received: 16 March 2021; Accepted: 10 May 2021;
Published: 07 June 2021.
Edited by:
Chaeyoung Lee, Soongsil University, South KoreaReviewed by:
Kaixiong Ye, University of Georgia, United StatesBor-Sen Chen, National Tsing Hua University, Taiwan
Copyright © 2021 Li, Yeung and Schooling. This is an open-access article distributed under the terms of the Creative Commons Attribution License (CC BY). The use, distribution or reproduction in other forums is permitted, provided the original author(s) and the copyright owner(s) are credited and that the original publication in this journal is cited, in accordance with accepted academic practice. No use, distribution or reproduction is permitted which does not comply with these terms.
*Correspondence: C. Mary Schooling, Y21zMUBoa3UuaGs=