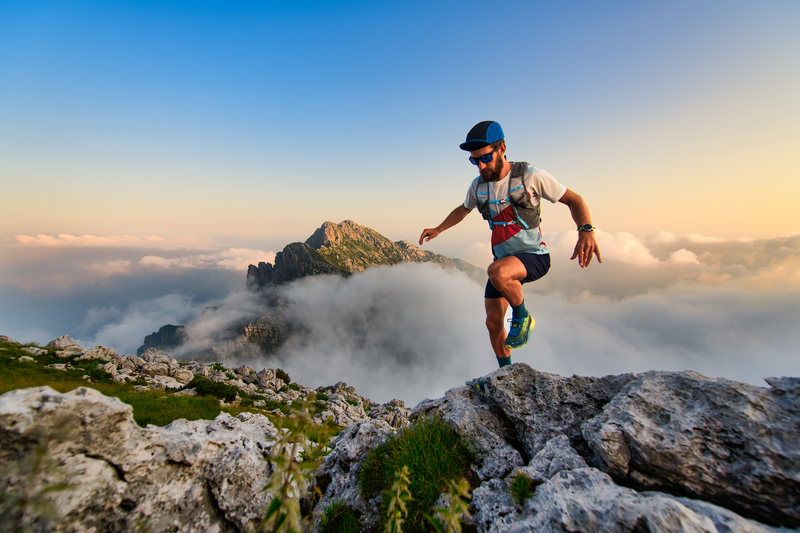
95% of researchers rate our articles as excellent or good
Learn more about the work of our research integrity team to safeguard the quality of each article we publish.
Find out more
BRIEF RESEARCH REPORT article
Front. Genet. , 12 July 2021
Sec. Evolutionary and Population Genetics
Volume 12 - 2021 | https://doi.org/10.3389/fgene.2021.678625
Sphingonotus Fieber, 1852 (Orthoptera: Acrididae), is a grasshopper genus comprising approximately 170 species, all of which prefer dry environments such as deserts, steppes, and stony benchlands. In this study, we aimed to examine the adaptation of grasshopper species to arid environments. The genome size of Sphingonotus tsinlingensis was estimated using flow cytometry, and the first high-quality full-length transcriptome of this species was produced. The genome size of S. tsinlingensis is approximately 12.8 Gb. Based on 146.98 Gb of PacBio sequencing data, 221.47 Mb full-length transcripts were assembled. Among these, 88,693 non-redundant isoforms were identified with an N50 value of 2,726 bp, which was markedly longer than previous grasshopper transcriptome assemblies. In total, 48,502 protein-coding sequences were identified, and 37,569 were annotated using public gene function databases. Moreover, 36,488 simple tandem repeats, 12,765 long non-coding RNAs, and 414 transcription factors were identified. According to gene functions, 61 cytochrome P450 (CYP450) and 66 heat shock protein (HSP) genes, which may be associated with drought adaptation of S. tsinlingensis, were identified. We compared the transcriptomes of S. tsinlingensis and two other grasshopper species which were less tolerant to drought, namely Mongolotettix japonicus and Gomphocerus licenti. We observed the expression of CYP450 and HSP genes in S. tsinlingensis were higher. We produced the first full-length transcriptome of a Sphingonotus species that has an ultra-large genome. The assembly characteristics were better than those of all known grasshopper transcriptomes. This full-length transcriptome may thus be used to understand the genetic background and evolution of grasshoppers.
Sphingonotus Fieber, 1852 (Orthoptera: Acrididae), is a species-rich genus of grasshoppers that comprises 192 valid species and subspecies (Cigliano et al., 2017). This genus shows extensive radiation in the Palearctic, and many species are endemic to islands (Husemann et al., 2015). These grasshoppers are distributed in arid zones of the Northern Hemisphere, with diverse hotspots in the Mediterranean and Central and Eastern Asia (Dey et al., 2018). Single species have been recorded in the Galapagos Islands, Mexico, the Caribbean, Brazil, and northwestern Australia (Rentz, 1996; Cigliano et al., 2017). Their wide distribution, regional high incidence of endemism, and polymorphic stridulation organs make Sphingonotus an attractive study system for biogeographic and evolutionary questions (Johnsen, 1985; Benediktov, 2009; Husemann et al., 2011). The most intriguing characteristic of these species is that they prefer dry environments such as deserts, steppes, and dry stony benchlands (Husemann et al., 2014). Recently, several studies examined the phylogeography and evolution of this genus. However, it remains unclear how its species have adapted to drought environments and radiated to such richness. Sphingonotus tsinlingensis (Orthoptera: Acridoidea) is a grasshopper endemic to China, where it occurs on sandy and stony benchlands along the northern Qinling Mountains (Zheng et al., 1963). This species belongs to the oriental lineage of Sphingonotus, represents the adaptive status of Sphingonotus spp. in the East, and may serve as an ideal model for studying the evolution and ecology of grasshoppers in arid environments (Husemann et al., 2014; Moussi et al., 2018). Nonetheless, only few studies and sequences of respective functional genes of this species are available (Cui and Huang, 2012; Shah et al., 2019). Thus, further reference sequences and molecular genetic studies are required to elucidate its evolutionary and ecological characteristics.
The large genomes of Acrididae and widespread occurrence of repetitive elements entail challenges regarding genome assembly, and the sequencing costs are considerably high (Wang et al., 2014; Verlinden et al., 2020). Transcriptomics represent an alternative to genomic approaches for non-model organisms (Yuan et al., 2019). Published Acridid transcriptomes include those of Gomphocerus sibiricus (Shah et al., 2019), Locusta migratoria (Zhang et al., 2018), Ceracris nigricornis (Yuan et al., 2019), Chorthippus biguttulus (Berdan et al., 2017), Shirakiacris shirakii (Qiu et al., 2016), and Xenocatantops brachycerus (Zhao et al., 2018). Many available transcripts are associated with growth, development, environmental adaptability, and metabolism of nutrients and bioactive components, including candidate olfactory-related genes, pigmentation, and green pigment metabolism pathways.
Full-length transcriptome analysis can help identify coding and non-coding RNA and quantify differential gene expression. Moreover, it plays an important role in deciphering genomic functions with respect to physiological mechanisms and responses to environmental challenges (Jiang et al., 2015). Full-length transcriptomes of the two grasshoppers Gomphocerus licenti and Mongolotettix japonicus have been published previously, which yielded 590,112 and 566,165 circular consensus sequences (CCSs) as well as 458,131 and 428,979 full-length non-chimeric (FLNC) reads, respectively. In total, 17,970 and 16,766 unigenes were identified, with 17,495 and 16,373 coding sequences (CDSs), 1,082 and 813 transcription factors (TFs), 11,840 and 10,814 simple sequence repeats (SSRs), and 905 and 706 long non-coding RNAs (lncRNAs) by analyzing the transcriptomes of G. licenti and M. japonicus, respectively; 15,803 and 14,846 respective unigenes were annotated in public databases (Yuan et al., 2020).
In this study, the genome size (GS) and transcriptome of S. tsinlingensis were produced and examined for further functional and ecological studies using the deep-coverage PacBio isoform sequencing technique (Camacho et al., 2015; Yuan et al., 2019). In addition to protein-coding genes, different types of genetic elements such as TFs, SSRs, and lncRNAs were identified and classified. The produced full-length transcriptome of S. tsinlingensis was compared to published transcriptomes of other grasshoppers for quality evaluation, and heat shock protein (HSP) and cytochrome P450 (CYP450) genes were analyzed to investigate drought adaptation in this species.
Six adult S. tsinlingensis males were collected from a natural population at a pebble beach in Xi’an (34°02′05.2″ N, 108°33′04.3″ E) on September 14, 2020. Some grasshoppers were kept alive in insect mesh cages until flow cytometry (FCM) was performed in the laboratory, while others were dissected, immediately immersed in liquid nitrogen, and stored at −80°C.
Flow cytometry was used to investigate the GS of S. tsinlingensis (Dolezel and Bartos, 2005). DNA content is directly proportional to FCM fluorescence intensity. Thus, the GS of an organism can be calculated by comparison of a sample’s fluorescence intensity with that of an internal standard of known GS. L. migratoria (1C = 6.5 G) (Wang et al., 2014) was chosen as an internal standard. FCM was performed as previously described (Gregory and Johnston, 2008; Hare and Johnston, 2011). To prepare single cell suspensions, head tissues of L. migratoria and S. tsinlingensis were removed, placed in a tissue grinder with 1 mL Galbraith buffer (Galbraith et al., 1983), and ground 20 times. Then, the solution was filtered through a 38-μm nylon mesh to remove cellular debris and stained using 50 μg/mL propidium iodide. The above steps were performed on ice. The solution was then stored in the dark at 4°C for 30 min. The GS was assessed using a flow cytometer (Cytoflex S; Beckman Coulter, Krefeld, Germany) with three technical replicates, which were activated with a 488-nm laser and low flow rates.
Total RNA was isolated from muscle tissues of the intact body of all individuals after removing the guts. Extractions were conducted using TRIzol reagent (Invitrogen, Carlsbad, CA, United States), following the manufacturer’s instructions. RNA degradation and contamination were screened using 1% agarose gel electrophoresis, and RNA integrity and purity were determined using an Agilent 2100 Bioanalyzer (Agilent Technologies, CA, United States) and a NanoDrop 2000 device (Thermo Scientific, Wilmington, DE, United States), respectively. Only total RNA samples with an RNA integrity index of ≥8 were used for producing high-throughput sequencing libraries.
The protocol of SMARTer PCR cDNA Synthesis Kit (TaKaRa, Dalian, China) was used to synthesize full-length cDNA and cDNA fractions. The BluePippin Size Selection system (Sage Science, Massachusetts, United States) was used to select the PCR products. SMRTbell (Pacific Biosciences, Menlo Park, CA, United States) template libraries were produced using the SMRTbell Template Prep Kit (Pacific Biosciences) according to the manufacturer’s instructions. An Agilent 2100 Bioanalyzer and a Qubit 2.0 device (Life Technologies, Carlsbad, CA, United States) were used to assess library quality and concentration, respectively. SMRT sequencing was performed using a PacBio Sequel platform (PacBio) at Novogene Technology Co. (Novogene, Beijing, China). An Illumina (San Diego, CA, United States) sequencing library was constructed using a Gene Expression Sample Prep Kit (Illumina), according to the manufacturer’s instructions. The qualified library was paired-end sequenced (2 × 150 bp) on an Illumina HiSeq X Ten (Illumina) platform by a commercial provider (Novogene).
PacBio data were processed using the SMRT Link 5.1 software pipeline (Pacific Biosciences). First, subreads were identified, and CCSs were produced using corrections between subreads. The CCSs were divided into FLNC and NFL sequences according to whether they contained 5’-primers, 3’-primers, and poly-A tails. FLNC reads were clustered using the ICE algorithm to obtain consensus sequences; Arrow software1 was used to refine consensus isoforms using the NFL to produce refined consensus sequences. Illumina RNA-seq short reads were filtered to remove adaptor sequences, ambiguous reads with “N” bases, and low-quality reads. Filtered Illumina data were then used to refine consensus sequences using Proovread (Hackl et al., 2014). Redundant isoforms (identity < 0.9; coverage age < 0.85) were eliminated using the CD-HIT program without considering the 5’-difference. To process raw Illumina sequencing data, Trimmomatic and FastQC (Poluri et al., 2019) software programs were used. HISAT (Pertea et al., 2016) was then used to map the filtered reads to the genome. Fragments per kilobase of exon per million fragments mapped (FPKM) values were calculated using StringTie (Pertea et al., 2015), and 25,761 genes had FPKM values.
Gene functions were annotated using the following databases: non-redundant protein sequences (NR) (Li et al., 2002), non-redundant nucleotide sequences (NT), Protein Family (Pfam), clusters of orthologous groups of proteins (KOG) (Tatusov et al., 2003), Swiss-Prot (Bairoch and Apweiler, 2000), Kyoto Encyclopedia of Genes and Genomes (KEGG) (Kanehisa et al., 2004), and Gene Ontology (GO) (Ashburner et al., 2000). The Basic Local Alignment Search Tool (BLAST) was used with an e-value of 1–10 in the NT database analysis, and the Diamond BLASTX software was used with an e-value 1–10 in the NR, KOG, Swiss-Prot, and KEGG database analyses. Hmmscan software was used for Pfam database analysis.
ANGEL software (Shimizu et al., 2006) was used to determine CDSs among cDNA sequences. ANGEL calculates the coding potential of all codons using information from a short region around the target codon. Short regions are generated using a sliding window. All codons were labeled as CDS or ELSE, according to their coding potential. The most probable path was then traced using a Markov chain model with dynamic programming. Positions where a frame was changed were detected as the rough positions of frameshift errors in the path. Finally, each rough position was modified by selecting the most probable position from the candidate positions located near the rough positions.
Transcription factors were predicted using the AnimalTFDB 2.0 database2. Hmmscan software was used for comparison with the AnimalTFDB database to screen third-generation sequences (e-value < 0.0001) for TF identification and assign transcripts to different families. The Coding–Non-Coding Index (Sun et al., 2013), coding potential calculator (Kong et al., 2007), Pfam-scan (Finn et al., 2016), and predictors of long non-coding RNAs and messenger RNAs based on an improved k-mer scheme (Li et al., 2014) tools were used to predict the coding potential of transcripts. Transcripts with predicted coding potential according to all four tools were filtered out, and those without coding potential constituted the candidate set of lncRNAs.
Simple sequence repeats of the transcriptome were identified using MIcroSAtellite3, which allows the identification and localization of perfect microsatellites as well as compound microsatellites that are interrupted by a certain number of bases.
The GS of S. tsinlingensis was estimated using FCM. Two peaks were identified, with P1 and P2 representing the counted cell intensities of L. migratoria and S. tsinlingensis, respectively. The mean fluorescent intensities of peaks P1 and P2 were 8.11 × 105 and 15.98 × 105 (low coefficient of variation, less than 5%), respectively. The ratio of GS of S. tsinlingensis to L. migratoria was equal to that of P2 to P1. The GS of S. tsinlingensis was calculated to be approximately 12.81 Gb (Supplementary Figure 1). This GS was considerably larger than that of L. migratoria and was one of the largest among Acrididae.
In total, 146.98 Gb raw polymerase reads were obtained using the PacBio isoform sequencing platform. After filtering and self-correcting the raw data, 28.6 million subreads were processed into 901,383 CCS reads. These CCSs were then clustered and polished into 88,693 non-redundant full-length, non-chimeric isoforms, which was the final molecular sequence pool for screening gene components (Supplementary Table 1; Supplementary Figure 2). The full-length transcriptome of S. tsinlingensis showed better quality characteristics than other reported grasshopper genomes and transcriptomes. The transcript number was 88,693, and the percentage of long non-redundant isoforms (over 1,000 bp) was 99.28%. The most outstanding parameter was an average length of 2,497 bp and N50 value of 2,726 bp, which was longer than other transcriptomes (Table 1).
Table 1. Comparison of transcriptome assemblies and gene numbers with the eight published Acrididae transcriptomes.
Among full-length, non-chimeric isoforms of S. tsinlingensis, 48,502 transcripts with CDSs were identified, accounting for approximately 54.68% of the total isoforms. The N50 length of CDSs was 1,230 bp, and each CDS encoded 229.3 amino acids on average (Supplementary Figure 3). In total, 37,569 CDSs were retrieved after integrated annotations using seven gene databases, accounting for 78.18% of the total CDSs (Figure 1A; Supplementary Table 2). A Venn diagram was generated to visualize the respective contribution of different databases to the annotations, and 3,575 genes were annotated by the five most commonly employed databases (Figure 1B).
Figure 1. Statistics on the functional annotation of all Sphingonotus tsinlingensis protein-coding sequences (CDSs) in seven common databases (A), and a Venn diagram showing the functional annotation of S. tsinlingensis transcripts in the five most commonly used databases (B). NR, non-redundant protein sequence; KEGG, Kyoto Encyclopedia of Genes and Genomes; KOG, EuKaryotic Orthologous Groups; GO, gene ontology; NT, non-redundant nucleotide sequences; Pfam, protein family.
The main annotations were from the NR database (34,731 genes). Among these, S. tsinlingensis produced the most hits with Zootermopsis nevadensis (12.86%, 4,459 genes; Supplementary Figure 4A). The pattern of hits suggested that these species shared close phylogenetic positions, and the percentages corresponded to the number of their indexed sequences in the database, which also revealed that the proportion of Acrididae was not as high as that of other species. GO and KEGG annotations were used to describe the genome composition of S. tsinlingensis. Cells and cell parts (2,865 genes) were the most represented terms in the cell component categories, and binding (7,457 genes) was the most represented molecular function (Supplementary Figure 4B). In the KEGG annotations, 30,637 annotations were assigned to 355 signaling pathways. The most enriched KEGG pathways were associated with basic metabolism processes, including signal transduction (1,008 genes), transport and catabolism (608 genes), amino acid metabolism (606 genes), cancer overview (578 genes), and endocrine system (571 genes; Supplementary Figure 4C). Several highly conserved signaling pathways that play a critical role in insect growth and body development were markedly enriched, including the Wnt, Notch, transforming growth factor-β, Janus kinase/signal transducer and activator of transcription, mitogen-activated protein kinase, and Hedgehog pathways.
Drought adaption-related genes and their annotations were identified from the isoform sequences of S. tsinlingensis, including 61 xenobiotic CYP450 and 66 HSP genes. The lengths of CYP450 and HSP sequences varied from 1,019 to 5,346 bp and from 1,438 to 4,428 bp, respectively. Most BLAST identities of these target-focused sequences were below 95%, which suggested that they were novel to the current understanding of genetic mechanisms in S. tsinlingensis (Supplementary Figure 5).
To confirm that these sequences were associated with adaptation to drought in S. tsinlingensis, we compared the expression of CYP450 and HSP genes among the transcriptomes of S. tsinlingensis and two other grasshopper species that were less tolerant to dry areas (M. japonicus and G. licenti). The box plot illustrates that the distribution of expressions had a wider range with respect to CYP450 and HSP genes in S. tsinlingensis than in M. japonicus and G. licenti. In particular, the distribution ranges Q1 to Q3 of the FPKM values of CYP450 and HSP genes were 6.38 to 48.10 and 34 to 65.36, respectively, in S. tsinlingensis. However, these values were only 2.12 to 11.74 (CYP450) and 11.89 to 64.53 (HSP) in M. japonicus and 2.32 to 8.30 (CYP450) and 1.67 to 8.05 (HSP) in G. licenti, respectively (Figure 2). These results suggested that more members of CYP450 and HSP genes in S. tsinlingensis participated and contributed to its adaptation to drought. In addition, the overall expression levels of CYP450 and HSP genes were higher than those in other grasshoppers such as M. japonicus and G. licenti.
Figure 2. Expression levels of CYP450 and HSP genes in Sphingonotus tsinlingensis, Mongolotettix japonicus, and Gomphocerus licenti. The y-axis indicates FPKM values.
LncRNAs were identified using a combination of the Coding Potential Calculator, Coding–Non-Coding Index, Coding Potential Assessment Tool, and Pfam methods. In total, 12,765 (22.94%) valid lncRNAs (>200 bp long and with more than two exons) were identified (Figure 3). A length distribution analysis of lncRNAs revealed that their lengths ranged from 212 to 8,365 bp, with a mean length of 2,090 bp. The N50 length of these identified lncRNAs was 2,185 bp.
Figure 3. Venn diagram showing the distribution of long non-coding RNAs (lncRNAs) identified in Sphingonotus tsinlingensis. CNCI, Coding–Non-Coding Index; Pfam, protein family; PLEK, predictor of long non-coding RNAs and messenger RNAs based on an improved k-mer scheme; CPC, coding potential calculator.
The number of lncRNAs was significantly lower than that of mRNAs. To verify the two possible conditions, i.e., each type of lncRNA regulated multiple mRNAs or the valid lncRNA was not fully determined, the linkages between the 12,765 lncRNAs and 48,502 CDSs were checked. The lncRNAs were only related to 9,347 CDSs, suggesting that lncRNAs were not fully sequenced. Four HSP and no CYP450 gene-associated lncRNAs were identified, suggesting that the regulatory mechanisms of these HSP and CYP450 genes were not well characterized.
Transcription factors participate in gene expression regulation by linking lncRNAs and mRNAs. In this study, 414 putative TFs belonging to 38 TF gene families were predicted (Supplementary Table 3). zf-C2H2 (20.29%, 84/414) was the most abundant TF family, followed by ZBTB (16.18%, 67/414) and THAP (15.22%, 63/414; Supplementary Figure 6). The relationships among lncRNAs, TFs, and mRNAs are not discussed here because of the limited number of TFs. Instead, a GO enrichment analysis was conducted using genes in which TFs have been determined. Although lower in numbers, these genes did not present functional bias and covered almost all basic metabolic functions. Survival-dependent mechanisms such as multicellular organismal processes, developmental processes, and immune system processes were the most enriched mechanisms (Figure 4).
Figure 4. Gene ontology (GO) enrichment reveals the functions of genes that have been determined to be transcription factors (TFs). The rich factor refers to the ratio of the number of differentially expressed transcripts to the total number of annotated transcripts located in the GO term, and the Q-value is the P-value corrected by multiple hypothesis tests, with a value range of 0 to 1. The closer the Q-value is to zero, the more significant is the enrichment.
Simple sequence repeats are important molecular markers that indicate genetic polymorphisms. In total, 36,488 SSRs were identified among all isoforms of S. tsinlingensis; 3,719 genes contained more than one SSR, and 328 SSRs were present in the compound form. Among the six SSR types, mono-nucleotide repeats (18,078, 49.55%) were most abundant, followed by dinucleotide repeats (9,859, 27.02%), trinucleotide repeats (7,612, 20.86%), tetranucleotide repeats (791, 2.17%), pentanucleotide repeats (107, 0.29%), and hexanucleotide repeats (41, 0.11%; Supplementary Figure 7). This full-length transcriptome of S. tsinlingensis contained more SSRs than the other published full-length transcriptomes of grasshoppers, including that of G. licenti, M. japonicus, and S. shirakii, possibly because of higher heterozygosity of S. tsinlingensis or a more thorough SSR search.
To the best of our knowledge, this is the first genomic study on Sphingonotus spp. Although S. tsinlingensis is an oriental species endemic to China, it represents the most popular morphological type of the grasshopper genus Sphingonotus and the subgenus Spingonotus. These taxa show hind wings with a curved black bind and blue color at the base (Zheng et al., 1963). A comprehensive transcriptome comprises all expressed gene sequences of an organism. These sequences can act as gene pools to provide and complement species-specific sequences. In addition, full-length transcriptome has better connectivity and integrity compared to Illumina short reads, and play an important role in gene annotation. Therefore, the transcriptome of S. tsinlingensis may provide a reference for the study of Sphingonotus species. Data, including lncRNAs, TFs, and SSRs, may be used as a genetic background for gene identification, homologous gene screening, phylogenetics, and adaptive evolution analysis. Moreover, through well-connected and accurate sequences and short-read quantitative analyses, these elements were found to be involved in the unique physiological processes of this species. These results suggest that our data are reliable and may be of use for future studies.
Based on the ultra-large GS, we suggest using full-length transcriptomes instead of whole genomes when conducting genomic studies on grasshoppers of this genus. In general, PacBio sequences are 85% accurate at 30-fold coverage of the transcriptome (Midha et al., 2019). In this study, the full-length transcriptome was successfully produced at 60-fold coverage. The success of this strategy will help optimize the experimental design for similar future studies. Because grasshoppers have very large genomes and genome assemblies are difficult to construct owing to the highly repetitive regions (Schatz et al., 2010; Shah et al., 2019), grasshopper genome assembly requires a considerable amount of sequencing and computation and is thus time-consuming and expensive. Even with respect to mRNA, sequencing frequently fails to enrich effective sequences owing to the large GS, and rare genes are missed because of sequencing bias (Gao et al., 2016). To address this problem, sequencing depth was increased in the current study to produce a sufficient amount of effective sequences. The number of protein-expressing genes in the functional gene dataset observed in the current study was the same as that found in previous studies (Yuan et al., 2020). The proportion of annotated functional genes was increased, and the average length of genes was markedly improved. From the composition of gene functions, gene detection was complete, and many basic metabolism-related genes were detected. Among grasshopper species for which both transcriptome and genome was reported, the GS of S. tsinlingensis was the largest. Its GS of approximately 12.81 Gb was almost twice that of L. migratoria (Wang et al., 2014). A comprehensive exploration of the effective sequences in the genome was conducted when the ratio of transcript size to GS was less than 2%.
These results suggest that the full-length transcriptome is an effective method for studying the genomics of grasshopper species with ultra-large and complex genomes. However, there are some limitations to this study. For example, lncRNAs, miRNAs, TFs, and mRNAs obtained from the full-length transcriptome typically play important roles in regulating gene expression at epigenetic, transcriptional, and post-transcriptional levels and constructing miRNA-lncRNAs-mRNAs-TF networks (Ye et al., 2018). Nonetheless, no association between these four regulatory elements was identified in the present study. The regulatory elements detected using functional enrichment reflected only the basic metabolic processes. To obtain better data in future studies and better enrich the results of the full-length transcriptome, a combination of multi-omics methods should be used, such as competing endogenous RNA for identification (Jiang et al., 2020) supplemented with mRNA next-generation sequencing for expression profile analysis.
This is the first GS and full-length transcriptome study on a species of the genus Sphingonotus. S. tsinlingensis has an ultra-large genome (12.81 Gb). Deep PacBio sequencing is currently the best method for retrieving nucleic acid sequences from species with ultra-large and complex genomes. The full-length transcriptome produced in the present study provides a reference resource for future studies on gene identification and comparison and will help improve our understanding of the mechanisms by which grasshoppers adapt to arid environments.
The datasets presented in this study can be found in online repositories. The names of the repository/repositories and accession number(s) can be found below: https://www.ncbi.nlm.nih.gov/, PRJNA707366 and https://zenodo.org/record/4588269#.YEdESKSwM3s, 4588269.
LZ, D-LG, and S-QX conceived the study and designed the experiments. D-LG analyzed the data. LZ wrote the manuscript. LZ, HW, PL, and KS performed the sequencing experiments. D-LG and S-QX revised the manuscript. All authors read and approved the final manuscript.
This work was supported by the Excellent Doctor Innovation Project of Shaanxi Normal University (S2015YB03) and Fundamental Research Funds for the Central Universities (GK201903063 and GK202105003). This work was also partly supported by the National Natural Science Foundation of China (No. 31872273).
The authors declare that the research was conducted in the absence of any commercial or financial relationships that could be construed as a potential conflict of interest.
We thank Hua-Teng Huang and Hao Yuan from Shaanxi Normal University, Xi’an, China, for providing data support of Mongolotettix japonicus and Gomphocerus licenti.
The Supplementary Material for this article can be found online at: https://www.frontiersin.org/articles/10.3389/fgene.2021.678625/full#supplementary-material
Ashburner, M., Ball, C. A., Blake, J. A., Botstein, D., Butler, H., Cherry, J. M., et al. (2000). Gene ontology: tool for the unification of biology. The Gene Ontology Consortium. Nat. Genet. 25, 25–29. doi: 10.1038/75556
Bairoch, A., and Apweiler, R. (2000). The SWISS-PROT protein sequence database and its supplement TrEMBL in 2000. Nucleic Acids Res. 28, 45–48. doi: 10.1093/nar/28.1.45
Benediktov, A. A. (2009). To the taxonomy and bioacoustics of grasshoppers of the genus Sphingonotus Fieber, 1852 (Orthoptera, Acrididae, Oedipodinae). Proc. Russ. Entomol. Soc. 80, 21–33. doi: 10.3897/jor.26.14550
Berdan, E. L., Finck, J., Johnston, P. R., Waurick, I., Mazzoni, C. J., and Mayer, F. (2017). Transcriptome profiling of ontogeny in the acridid grasshopper Chorthippus biguttulus. PLoS One 12:e0177367. doi: 10.1371/journal.pone.0177367
Bier, K., and Muller, W. J. B. Z. (1969). DNS-Messungen bei Insekten und eine Hypothese uber retardierte Evolution and besonderen DNS-Reichtum im Tierreich. Biol. Zent. Bl. 88, 425–449. doi: 10.1007/978-3-642-49227-3_29
Camacho, J. P., Ruiz-Ruano, F. J., Martín-Blázquez, R., López-León, M. D., Cabrero, J., Lorite, P., et al. (2015). A step to the gigantic genome of the desert locust: chromosome sizes and repeated DNAs. Chromosoma 124, 263–275. doi: 10.1007/s00412-014-0499-0
Cigliano, M. M., Braun, H., Eades, D. C., and Otte, D. (2017). Orthoptera Species File. Version 5.0/5.0. Available Online at: http://Orthoptera.SpeciesFile.org [accessed Dec 11, 2017].
Cui, A. M., and Huang, Y. (2012). [Phylogenetic relationships among Orthoptera insect groups based on complete sequences of 16S ribosomal RNA]. Yi Chuan 34, 597–608. doi: 10.3724/sp.j.1005.2012.00597
Dey, L. S., Saboori, A., Hodjat, S. H., Tork, M., Pahlow, F., and Husemann, M. (2018). A faunistic review of the Iranian species of Sphingonotus (Orthoptera, Oedipodinae) with an online key to species. Zootaxa 4379, 151–176. doi: 10.11646/zootaxa.4379.2.1
Dolezel, J., and Bartos, J. (2005). Plant DNA flow cytometry and estimation of nuclear genome size. Ann. Bot. 95, 99–110. doi: 10.1093/aob/mci005
Finn, R. D., Coggill, P., Eberhardt, R. Y., Eddy, S. R., Mistry, J., Mitchell, A. L., et al. (2016). The Pfam protein families database: towards a more sustainable future. Nucleic Acids Res. 44, D279–85. doi: 10.1093/nar/gkv1344
Fox, D. P. J. C. (1970). A non-doubling DNA series in somatic tissues of the locusts Schistocerca gregaria (Forskål) and Locusta migratoria (Linn.). Chromosoma 29, 446–461.
Galbraith, D. W., Harkins, K. R., Maddox, J. M., Ayres, N. M., Sharma, D. P., and Firoozabady, E. (1983). Rapid flow cytometric analysis of the cell cycle in intact plant tissues. Science 220, 1049–1051. doi: 10.1126/science.220.4601.1049
Gao, R., Yu, K., Nie, J., Lian, T., Jin, J., Liljas, A., et al. (2016). Deep sequencing reveals global patterns of mRNA recruitment during translation initiation. Sci. Rep. 6:30170. doi: 10.1038/srep30170
Gosalvez, J., López-Fernandez, C., and Esponda, P. J. C. (1980). Variability of the DNA Content in Five Orthopteran Species. Caryologia 33, 275–281. doi: 10.1080/00087114.1980.10796840
Gregory, T. R., and Johnston, J. S. (2008). Genome size diversity in the family Drosophilidae. Heredity (Edinb) 101, 228–238. doi: 10.1038/hdy.2008.49
Hackl, T., Hedrich, R., Schultz, J., and Förster, F. (2014). proovread: large-scale high-accuracy PacBio correction through iterative short read consensus. Bioinformatics 30, 3004–3011. doi: 10.1093/bioinformatics/btu392
Hare, E. E., and Johnston, J. S. (2011). Genome size determination using flow cytometry of propidium iodide-stained nuclei. Methods Mol. Biol. 772, 3–12. doi: 10.1007/978-1-61779-228-1_1
Husemann, M., Deppermann, J., and Hochkirch, A. (2014). Multiple independent colonization of the Canary Islands by the winged grasshopper genus Sphingonotus Fieber, 1852. Mol. Phylogenet. Evol. 81, 174–181. doi: 10.1016/j.ympev.2014.09.017
Husemann, M., Habel, J. C., Namkung, S., Hochkirch, A., Otte, D., and Danley, P. D. (2015). Molecular evidence for an old world origin of Galapagos and Caribbean band-winged grasshoppers (Acrididae: Oedipodinae: Sphingonotus). PLoS One 10:e0118208. doi: 10.1371/journal.pone.0118208
Husemann, M., Ray, J., and Hochkirch, A. J. Z. (2011). A revision of the subgenus Parasphingonotus Benediktov & Husemann, 2009 (Orthoptera: Oedipodinae: Sphingonotini). Zootaxa 2916, 51–61. doi: 10.11646/zootaxa.2916.1.4
Jiang, J., Bi, Y., Liu, X. P., Yu, D., Yan, X., Yao, J., et al. (2020). To construct a ceRNA regulatory network as prognostic biomarkers for bladder cancer. J. Cell Mol. Med. 24, 5375–5386. doi: 10.1111/jcmm.15193
Jiang, Z., Zhou, X., Li, R., Michal, J. J., Zhang, S., Dodson, M. V., et al. (2015). Whole transcriptome analysis with sequencing: methods, challenges and potential solutions. Cell. Mol. Life Sci. 72, 3425–3439. doi: 10.1007/s00018-015-1934-y
John, B., and Hewitt, G. M. (1966). Karyotype stability and DNA variability in the Acrididae. Chromosoma 20, 155–172. doi: 10.1007/bf00335205
Johnsen, P. (1985). Contributions to the knowledge of the genera Sphingonotus, Pseudosphingonotus and Wernerella in Africa, with description of four new species (Acrididae: Oedipodinae). Natura Jutlandica 21, 149–168.
Kanehisa, M., Goto, S., Kawashima, S., Okuno, Y., and Hattori, M. (2004). The KEGG resource for deciphering the genome. Nucleic Acids Res. 32, D277–D280. doi: 10.1093/nar/gkh063
Kong, L., Zhang, Y., Ye, Z. Q., Liu, X. Q., Zhao, S. Q., Wei, L., et al. (2007). CPC: assess the protein-coding potential of transcripts using sequence features and support vector machine. Nucleic Acids Res. 35, W345–W349. doi: 10.1093/nar/gkm391
Li, A., Zhang, J., and Zhou, Z. (2014). PLEK: a tool for predicting long non-coding RNAs and messenger RNAs based on an improved k-mer scheme. BMC Bioinformatics 15:311. doi: 10.1186/1471-2105-15-311
Li, W., Jaroszewski, L., and Godzik, A. (2002). Tolerating some redundancy significantly speeds up clustering of large protein databases. Bioinformatics 18, 77–82. doi: 10.1093/bioinformatics/18.1.77
Midha, M. K., Wu, M., and Chiu, K. P. (2019). Long-read sequencing in deciphering human genetics to a greater depth. Hum. Genet. 138, 1201–1215. doi: 10.1007/s00439-019-02064-y
Moussi, A., Dey, L. S., Petit, D., Abba, A., Klesser, R., and Husemann, M. J. A. Z. (2018). First genetic data for band-winged grasshoppers (Orthoptera: Acrididae: Oedipodinae) of the Biskra region of Algeria with new records for the country. Afr. Zool. 53, 31–40. doi: 10.1080/15627020.2018.1463172
Pertea, M., Kim, D., Pertea, G. M., Leek, J. T., and Salzberg, S. L. (2016). Transcript-level expression analysis of RNA-seq experiments with HISAT, StringTie and Ballgown. Nat. Protoc. 11, 1650–1667. doi: 10.1038/nprot.2016.095
Pertea, M., Pertea, G. M., Antonescu, C. M., Chang, T. C., Mendell, J. T., and Salzberg, S. L. (2015). StringTie enables improved reconstruction of a transcriptome from RNA-seq reads. Nat. Biotechnol. 33, 290–295. doi: 10.1038/nbt.3122
Poluri, R. T. K., Beauparlant, C. J., Droit, A., and Audet-Walsh, É (2019). RNA sequencing data of human prostate cancer cells treated with androgens. Data Brief 25:104372. doi: 10.1016/j.dib.2019.104372
Qiu, Z., Liu, F., Lu, H., Yuan, H., Zhang, Q., and Huang, Y. (2016). De Novo Assembly and Characterization of the Transcriptome of Grasshopper Shirakiacris shirakii. Int. J. Mol. Sci. 17:1110. doi: 10.3390/ijms17071110
Rentz, D. C. (1996). Grasshopper country: the abundant orthopteroid insects of Australia. Q. Rev. Biol. 72:337. doi: 10.1086/419901
Schatz, M. C., Delcher, A. L., and Salzberg, S. L. (2010). Assembly of large genomes using second-generation sequencing. Genome Res. 20, 1165–1173. doi: 10.1101/gr.101360.109
Shah, A., Hoffman, J. I., and Schielzeth, H. (2019). Transcriptome assembly for a colour-polymorphic grasshopper (Gomphocerus sibiricus) with a very large genome size. BMC Genomics 20:370. doi: 10.1186/s12864-019-5756-4
Shimizu, K., Adachi, J., and Muraoka, Y. (2006). ANGLE: a sequencing errors resistant program for predicting protein coding regions in unfinished cDNA. J. Bioinform. Comput. Biol. 4, 649–664. doi: 10.1142/s0219720006002260
Sun, L., Luo, H., Bu, D., Zhao, G., Yu, K., Zhang, C., et al. (2013). Utilizing sequence intrinsic composition to classify protein-coding and long non-coding transcripts. Nucleic Acids Res. 41:e166. doi: 10.1093/nar/gkt646
Tatusov, R. L., Fedorova, N. D., Jackson, J. D., Jacobs, A. R., Kiryutin, B., Koonin, E. V., et al. (2003). The COG database: an updated version includes eukaryotes. BMC Bioinformatics 4:41. doi: 10.1186/1471-2105-4-41
Verlinden, H., Sterck, L., Li, J., Li, Z., Yssel, A., Gansemans, Y., et al. (2020). First draft genome assembly of the desert locust, Schistocerca gregaria. F1000Res 9:775. doi: 10.12688/f1000research.25148.1
Wang, X., Fang, X., Yang, P., Jiang, X., Jiang, F., Zhao, D., et al. (2014). The locust genome provides insight into swarm formation and long-distance flight. Nat. Commun. 5:2957. doi: 10.1038/ncomms3957
Wilmore, P. J., and Brown, A. K. J. C. (1975). Molecular properties of orthopteran DNA. Chromosoma 51, 337–345. doi: 10.1007/bf00326320
Ye, Y., Li, S. L., and Wang, S. Y. (2018). Construction and analysis of mRNA, miRNA, lncRNA, and TF regulatory networks reveal the key genes associated with prostate cancer. PLoS One 13:e0198055. doi: 10.1371/journal.pone.0198055
Yuan, H., Chang, H., Zhao, L., Yang, C., and Huang, Y. (2019). Sex- and tissue-specific transcriptome analyses and expression profiling of olfactory-related genes in Ceracris nigricornis Walker (Orthoptera: Acrididae). BMC Genomics 20:808. doi: 10.1186/s12864-019-6208-x
Yuan, H., Zhang, X., Zhao, L., Chang, H., Yang, C., Qiu, Z., et al. (2020). Characterization and analysis of full-length transcriptomes from two grasshoppers, Gomphocerus licenti and Mongolotettix japonicus. Sci. Rep. 10:14228. doi: 10.1038/s41598-020-71178-5
Zhang, X., Kang, X., Wu, H., Silver, K., Zhang, J., Ma, E., et al. (2018). Transcriptome-wide survey, gene expression profiling and exogenous chemical-induced transcriptional responses of cytochrome P450 superfamily genes in migratory locust (Locusta migratoria). Insect Biochem. Mol. Biol. 100, 66–77. doi: 10.1016/j.ibmb.2018.06.006
Zheng, Z. M., Tu, Q., and Liang, L. Q. (1963). A New Species of the Genus Sphingonotus Fieb. from China (Orthoptera: Acrididae). Acta Zoologica Sinica, 15, 279–281.
Keywords: Sphingonotus Fieber, grasshoppers, PacBio isoform sequencing, gene functions, genetic background
Citation: Zhao L, Wang H, Li P, Sun K, Guan D-L and Xu S-Q (2021) Genome Size Estimation and Full-Length Transcriptome of Sphingonotus tsinlingensis: Genetic Background of a Drought-Adapted Grasshopper. Front. Genet. 12:678625. doi: 10.3389/fgene.2021.678625
Received: 12 March 2021; Accepted: 14 June 2021;
Published: 12 July 2021.
Edited by:
Alison G. Nazareno, Federal University of Minas Gerais, BrazilReviewed by:
Zhihang Zhuo, Hainan University, ChinaCopyright © 2021 Zhao, Wang, Li, Sun, Guan and Xu. This is an open-access article distributed under the terms of the Creative Commons Attribution License (CC BY). The use, distribution or reproduction in other forums is permitted, provided the original author(s) and the copyright owner(s) are credited and that the original publication in this journal is cited, in accordance with accepted academic practice. No use, distribution or reproduction is permitted which does not comply with these terms.
*Correspondence: De-Long Guan, Z3VhbmRlbG9uZ0Bzbm51LmVkdS5jbg==; Sheng-Quan Xu, eHVzaGVuZ3F1YW5Ac25udS5lZHUuY24=
Disclaimer: All claims expressed in this article are solely those of the authors and do not necessarily represent those of their affiliated organizations, or those of the publisher, the editors and the reviewers. Any product that may be evaluated in this article or claim that may be made by its manufacturer is not guaranteed or endorsed by the publisher.
Research integrity at Frontiers
Learn more about the work of our research integrity team to safeguard the quality of each article we publish.