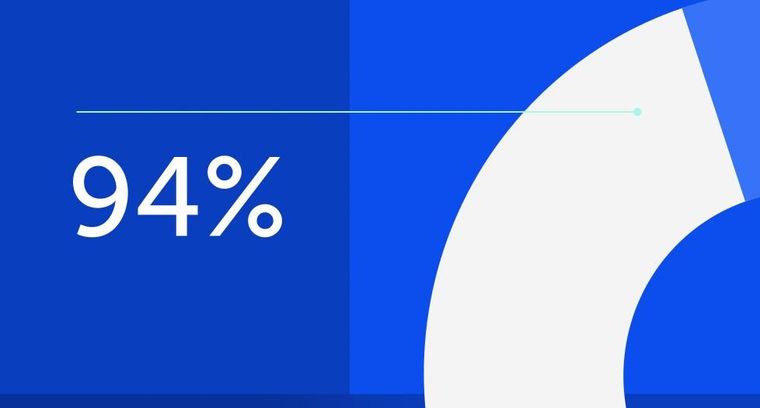
94% of researchers rate our articles as excellent or good
Learn more about the work of our research integrity team to safeguard the quality of each article we publish.
Find out more
ORIGINAL RESEARCH article
Front. Genet., 14 June 2021
Sec. Cancer Genetics and Oncogenomics
Volume 12 - 2021 | https://doi.org/10.3389/fgene.2021.676464
This article is part of the Research TopicPrecision Medicine in Cancer Treatment Using Multi-omics DataView all 7 articles
Objective: Recurrence remains the main cause of the poor prognosis in stage I-IIIA lung squamous cell carcinoma (LUSC) after surgical resection. In the present study, we aimed to identify the long non-coding RNAs (lncRNAs), microRNAs (miRNAs), and messenger RNAs (mRNAs) related to the recurrence of stage I-IIIA LUSC. Moreover, we constructed a risk assessment model to predict the recurrence of LUSC patients.
Methods: RNA sequencing data (including miRNAs, lncRNAs, and mRNAs) and relevant clinical information were obtained from The Cancer Genome Atlas (TCGA) database. The differentially expressed lncRNAs, miRNAs, and mRNAs were identified using the “DESeq2” package of the R language. Univariate Cox proportional hazards regression analysis and Kaplan-Meier curve were used to identify recurrence-related genes. Stepwise multivariate Cox regression analysis was carried out to establish a risk model for predicting recurrence in the training cohort. Moreover, Kaplan-Meier curves and receiver operating characteristic (ROC) curves were adopted to examine the predictive performance of the signature in the training cohort, validation cohort, and entire cohort.
Results: Based on the TCGA database, we analyzed the differentially expressed genes (DEGs) among 27 patients with recurrent stage I-IIIA LUSC and 134 patients with non-recurrent stage I-IIIA LUSC, and identified 431 lncRNAs, 36 miRNAs, and 746 mRNAs with different expression levels. Out of these DEGs, the optimal combination of DEGs was finally determined, and a nine-joint RNA molecular signature was constructed for clinical prediction of recurrence, including LINC02683, AC244517.5, LINC02418, LINC01322, AC011468.3, hsa-mir-6825, AC020637.1, AC027117.2, and SERPINB12. The ROC curve proved that the model had good predictive performance in predicting recurrence. The area under the curve (AUC) of the prognostic model for recurrence-free survival (RFS) was 0.989 at 3 years and 0.958 at 5 years (in the training set). The combined RNA signature also revealed good predictive performance in predicting the recurrence in the validation cohort and entire cohort.
Conclusions: In the present study, we constructed a nine-joint RNA molecular signature for recurrence prediction of stage I-IIIA LUSC. Collectively, our findings provided new and valuable clinical evidence for predicting the recurrence and targeted treatment of stage I-IIIA LUSC.
Globally, lung cancer contributes greatly to the high incidence and mortality of cancer. Lung squamous cell carcinoma (LUSC) accounts for 20–30% of non-small cell lung cancer (NSCLC) cases, and it is the second most common pulmonary malignancy worldwide (Chen et al., 2017; Bray et al., 2018; Gao et al., 2019). When patients are diagnosed with stage I-IIIA LUSC, the standard treatment for patients is radical resection. Besides, the treatment of stage IIIA LUSC consists of either concurrent chemoradiation or surgery with perioperative chemotherapy with or without thoracic radiotherapy resection (Hirsch et al., 2017; Kamigaichi et al., 2019; Leroy et al., 2019). Patients with LUSC beyond stage IIIA are in the advanced stage of the disease, and usually no longer undergo surgical treatment. Moreover, radiotherapy and chemotherapy for these pateints are more conservative (Ettinger et al., 2017). Most recently, despite progress has been made in the diagnosis and treatment, the recurrence rate is still high (Kataoka et al., 2019). Recurrence is the most common cause of treatment failure in LUSC patients and the main obstacle to long-term survival (Dziedzic et al., 2016; Zhang et al., 2018). Moreover, 10–60% of patients with stage IA–IIIA LUSC develop relapse within 5 years after surgical resection (Chen et al., 2019; Kataoka et al., 2019). Therefore, evaluating the risk of postoperative recurrence for patients with stage I-IIIA is of great significance for guiding clinical treatment.
Recently, a large number of studies have used abnormal molecular expression to predict the prognosis of cancer patients, including long non-coding RNAs (lncRNAs), microRNAs (miRNAs), and messenger RNA (mRNAs). LncRNAs are a class of RNA transcripts with more than 200 nucleotides in length. Generally speaking, lncRNAs exhibit a wide range of regulatory activities without the coding capacity of proteins or peptides (Chi et al., 2019). MiRNAs are very short (20–24 nucleotides) non-coding RNAs first discovered in 1993 (Lee et al., 1993). They play a role in tumorigenesis by regulating cell apoptosis, metabolism, metastasis, cycle, and angiogenesis (Iqbal et al., 2019). Moreover, mRNAs are a class of essential biological macromolecules, which carry genetic information from the nucleus to the cytoplasm for the expressions of functional proteins. Abnormal expression of proteins can cause many diseases, such as cancer and genetic diseases (Li et al., 2019). Increasing evidence has demonstrated that these molecules can not only act as oncogenes or tumor suppressor genes but also participate in the occurrence and development of tumors through a complex mutual regulatory network (Mirgayazova et al., 2019). Besides, their expressions are tumor-tissue specific and have the potential as diagnostic or prognostic markers (Sim et al., 2018; Hou et al., 2020; Peng et al., 2020). Therefore, it is urgently needed to investigate the integration of multiple RNA expression profiles to identify molecular signatures.
In the present study, the data of lncRNAs, miRNAs, and mRNAs of patients with stage I-IIIA LUSC were simultaneously analyzed. We screened differentially expressed genes (DEGs) and established a prognostic model based on a nine-joint RNA molecular signature to predict the recurrence of stage I-IIIA. Clinically, our newly identified signature could predict the possibility of postoperative recurrence for patients with stage I-IIIA LUSC.
On November 15, 2020, the RNA sequencing data (including lncRNAs, miRNAs, and mRNAs) and clinical data of LUSC patients were obtained from The Cancer Genome Atlas (TCGA) database.1 We searched for the lncRNA and mRNA profiles of LUSC in TCGA datasets. The search formula was set to: click “cases,” Primary Site is bronchus and lung, Program Name is TCGA, Project ID is TCGA-LUSC, Workflow Type is HTseq-Counts, Data Category is transcriptome profiling, Data Type is Gene Expression Quantification, and Experimental Strategy is RNA-Seq. We obtained lncRNA and mRNA datasets of LUSC. We searched for the miRNA profiles of LUSC in TCGA datasets. The search formula was set to: click “cases,” Primary Site is bronchus and lung, Program Name is TCGA, Project ID is TCGA-LUSC, Data Category is transcriptome profiling, Data Type is miRNA Expression Quantification, and Experimental Strategy is miRNA-Seq. We obtained miRNA datasets of LUSC. Download path of clinicopathological information of LUSC was as follows; click “cases,” Primary Site is bronchus and lung, Program Name is TCGA, Project ID is TCGA-LUSC, and Data Category is clinical. We obtained the clinicopathological data of LUSC. We conducted a careful screening and analysis of the downloaded data. There were 504 patients in the TCGA-LUSC cohort, 170 of which had clear recurrence information. Finally, 27 patients with recurrent stage I-IIIA LUSC and 134 patients with non-recurrent stage I-IIIA LUSC were selected in this study. The inclusion criteria were set as follows: 1. patients with stage I-IIIA LUSC; 2. patients whose database contained three sequences of lncRNAs, mRNAs, and miRNAs; and 3. patients with complete clinical-pathological parameters. Normalized RNASeq data and clinical data were matched according to patient barcodes. The Chi-square test was used to analyze the correlation of clinical-pathological parameters between the data cohorts.
By comparing the patients with recurrent stage I-IIIA LUSC to the patients with non-recurrent stage I-IIIA LUSC, the screening criteria of | log2 Fold Change | > 1, and P value <0.05 were used to identify significant lncRNAs, mRNAs, and miRNAs. All data in this study were obtained from the TCGA database, and ethical consent was not required.
Using the “survival” and “survminer” packages of the R software, the recurrence-related DEGs were initially screened through univariate Cox regression analysis. The Kaplan-Meier survival curve was further drawn for the above-mentioned recurrence-related DEGs, and log-rank test P value <0.05 was adopted to clarify their relationship with the recurrence-free survival (RFS) of stage I-IIIA LUSC.
Univariate Cox regression analysis and log-rank test P value were combined to further screen out recurrence-related DEGs. Patients with stage I-IIIA LUSC were randomly divided into the training cohort and validation cohort through the random serial number generated by the computer. Subsequently, a stepwise multivariate Cox’s proportional hazards regression model was used to evaluate the relative contribution of DEGs in the training cohort to recurrence prediction and identify gene-based prognostic signature with independent prognostic value. Based on the expressions of genes included in the constructed signature and the corresponding coefficients, the risk score of each sample was calculated using the formula as follows:
Using the median risk score as the cutoff point, LUSC patients were separated into high- (> median risk score) and low-risk (< median risk score) subgroups.
Kaplan-Meier analysis was used to evaluate the RFS difference between low- and high-risk groups. A P-value <0.05 was considered statistically significant. The receiver operating characteristic (ROC) curve was performed to assess the effectiveness of the risk scoring model. The area under the curve (AUC) value was calculated based on the ROC curve. Additionally, the performance of this model was evaluated in the validation cohort as well as the entire cohort.
A total of 161 I-IIIA LUSC samples with RNA expression profiles (including lncRNAs, miRNAs, and mRNAs) and their clinical-pathological data were obtained from the TCGA database, including 27 recurrent samples and 134 non-recurrent samples. Figure 1 presents the flow chart of the study procedure. Through the “DESeq2” package, we identified 431 lncRNAs, 36 miRNAs, and 746 mRNAs with different expression levels. Supplementary Tables 1–3 show the identified DEGs.
Figure 1. Overall design of the present study. LUSC, lung squamous cell carcinoma; RFS, Recurrence-free survival.
Univariate Cox regression analysis was performed to identify the association of the DEGs with recurrence in LUSC patients. Using the cut-off threshold of Cox P < 0.05, a set of 46 lncRNAs, four miRNAs, and 48 mRNAs were selected (Supplementary Tables 4–6). Furthermore, the Kaplan-Meier survival curve was drawn for the above-mentioned recurrence-related DEGs to determine the relationship with the RFS of patients with stage I-IIIA LUSC, and 91 RNA molecules were identified as candidates, including 46 lncRNAs (up-regulated genes: n = 23; down-regulated genes: n = 23), four miRNAs (up-regulated genes: n = 3; down-regulated genes: n = 1), and 41 mRNAs (up-regulated genes: n = 11; down-regulated genes: n = 30) (Supplementary Table 7).
A total of 161 patients (recurrence = 27; non-recurrence = 134) of stage I-IIIA LUSC with and without recurrence were randomly assigned to the training cohort (n = 96; recurrence = 18; non-recurrence = 78) and validation cohort (n = 65; recurrence = 9; non-recurrence = 56). There was no statistical difference in age and gender between the two groups. Table 1 summarizes their clinical parameters.
The optimal combination of nine recurrence-related DEGs was determined in the training cohort using the stepwise multivariate Cox regression analysis, including LINC02683, AC244517.5, LINC02418, LINC01322, AC011468.3, hsa-mir-6825, AC020637.1, AC027117.2, and SERPINB12. Table 2 lists the general information of these nine DEGs. Figure 2 shows that all nine DEGs were independent influencing factors for the recurrence of patients with I-IIIA LUSC. Kaplan-Meier survival analysis further revealed that the RFS of patients in the high expression group of LINC02683, AC244517.5, LINC02418, LINC01322, AC011468.3, and hsa-mir-6825 was lower compared with the low expression group. The RFS of patients in the low expression group of AC020637.1, AC027117.2, and SERPINB12 was significantly lower compared with the high expression group (Figure 3). These nine joint RNA molecules constituted a model, and the formula for prognostic risk score was as follows:
Figure 2. Expression profiles of nine genes for prediction of RFS in stage I-IIIA LUSC by multivariate Cox regression.
Figure 3. Kaplan-Meier analysis of RFS with nine genes (including LINC02683, AC244517.5, LINC02418, LINC01322, AC011468.3, hsa-mir-6825, AC020637.1, AC027117.2, and SERPINB12) in stage I-IIIA LUSC.
Based on the results of the multivariate Cox stepwise regression model and median risk score point, the patients in the training cohort could be divided into “high risk” and “low risk,” respectively.
In the training cohort, the risk score and survival status of all patients are shown in Figure 4A. Patients in the high-risk group had higher risk scores and tended to have shorter RFS, and significantly more patients died in this group. The heatmap represented the expression profiles of the RFS model based on the risk scoring system (Figure 4B). The high-risk patients showed worse RFS compared with the low-risk group in the training cohort (χ2 = 8.842, p < 0.0001) (Figure 4C). There was only one patient with recurrence in the low-risk group (1.0%), while there were 17 patients with recurrence in the high-risk group (17.7%). Additionally, ROC curve analysis depicted the discriminative value of our established risk scoring system. We found that the AUC of the prognostic model for RFS was 0.989 at 3 years and 0.958 at 5 years (Figure 4D), indicating that the model had a good predictive performance. Taken together, these data demonstrated that the impressive RFS model based on the stepwise multivariate Cox regression analysis could be an effective method for predicting recurrence.
Figure 4. (A) Scatter diagram of the risk score and survival status of patients with stage I-IIIA LUSC in the training cohort. (B) The heatmap of nine-gene expression profiles for predicting recurrence risk model. (C) Kaplan-Meier plot showed significance between high-risk and low-risk patients in RFS by the prognostic model (P < 0.05). (D) The ROC curve analysis for the recurrence risk model. ROC, receiver operating characteristic; AUC, area under the ROC curve.
In the validation cohort (n = 65) and the entire sample cohort (n = 161), the RFS of patients in the high-risk group was generally lower compared with the low-risk group, and more patients in the high-risk group were likely to die (Figures 5A,B). Figure 5C shows that in the validation cohort, the median RFS in the high-risk group was significantly shorter compared with the lower-risk group (χ2 = 5.575, P = 0.034). In the entire sample concentration, the RFS of the high-risk group was lower compared with the low-risk group (χ2 = 8.642, P < 0.0001) (Figure 5D). The time-dependent ROC curve suggested that the model showed an equal predictive performance in the validation cohort (3-year AUC = 0.802, 5-year AUC = 0.931) and the entire cohort (3-year AUC = 0.944, 5-year AUC = 0.943) (Figures 6A,B). The above-mentioned results suggested that the nine-gene recurrence model could effectively distinguish the possibility of postoperative recurrence between high- and low-risk groups of patients with stage I-IIIA LUSC.
Figure 5. The distribution of risk score and survival status of the nine-gene signature in the validation cohort (A) and entire cohort. (B) Kaplan-Meier curves of overall survival in the validation cohort (C) and entire cohort (D).
Figure 6. ROC analysis of the nine-gene risk model in the validation cohort (A) and the entire sample cohort (B).
In the past 30 years, surgery alone has been the standard treatment for patients with stage I-IIIA NSCLC. Even with complete resection, the 5-year survival rates remain disappointing. Meanwhile, little progress has been made in reducing the recurrence and subsequent mortality of lung cancer, especially LUSC (Li et al., 2018; Nasim et al., 2019). According to previous reports, LUSC has a higher local recurrence rate compared with lung adenocarcinoma (Woody et al., 2017; Mcaleese et al., 2019). The recurrence risk of LUSC patients of stage IA, IB, IIA, IIB, and IIIA is 33.9, 37.8, 61.2, 57.9, and 62.8%, respectively (Consonni et al., 2015). Although IB patients have been considered for adjuvant therapy, the benefits for stage I patients are still controversial (Tsuboi et al., 2007). Meanwhile, patients with stage II-IIIA may also have a good prognosis since they neither need adjuvant therapy nor benefit from adjuvant therapy (Subramanian and Simon, 2010; Wu et al., 2017). Effective stratification and prediction of patient prognosis will help guide the treatment strategies. Recent studies have proved that molecular approaches are effective in predicting the prognosis of cancer patients, which can help optimize individualized treatment, such as breast cancer, and liver cancer (Wan et al., 2010; Gu et al., 2019). In the present study, patients with stage I-IIIA LUSC in the TCGA database were included as the research object. After a series of analyses, the nine-gene signature could effectively predict the recurrence risk of stage I-IIIA LUSC, which was beneficial to the clinical judgment of adjuvant treatment for stage I LUSC patients after surgery, avoiding excessive radiotherapy and chemotherapy for stage II and III patients with lower risk.
The combined analysis of a panel of multiple factors, rather than a single biomarker, will have more power to provide useful information for clinical practice. For example, by analyzing differential mRNAs, Sun et al. have found that S100A16, IGKV4, S100P, ANGPTL4, SEMA4B, and LGR4 are high-risk immune genes in NSCLC that affect the prognosis of the disease (Sun et al., 2020). Sim et al. have found that three miRNAs (let-7g-5p, miR-143-3p, and miR-374a-5p) are associated with postoperative recurrence of lung adenocarcinoma in TCGA datasets (Sim et al., 2018). We believed that an ideal prognostic classifier for LUSC recurrence risk should be robust and potentially feasible in clinical samples. Combined analysis of a variety of molecules to find the optimal combination of DEGs would overcome barriers to sample collection and storage. In our present study, lncRNAs, miRNAs, and mRNAs were included in the gene screening, and finally, a nine-gene signature related to tumor recurrence in LUSC patients was determined. Time-independent ROC analysis showed that our nine-gene signature was effective for prognostic evaluation, suggesting that the model could be used in the training cohort (3-year AUC = 0.989, 5-year AUC = 0.958), the validation cohort (3-year AUC = 0.802, 5-year AUC = 0.931), and the entire data cohort (3-year AUC = 0.944, 5-year AUC = 0.943). The Kaplan-Meier survival charts of the three groups also showed that the RFS of the high-risk group was significantly lower compared with the low-risk group.
Among the identified genes in the present study, we found that LINC02683, AC244517.5, LINC02418, LINC01322, AC011468.3, and hsa-mir-6825 were risk factors. In contrast, AC020637.1, AC027117.2, and SERPINB12 were protective factors. Multivariate analysis showed that these high-level risk factors and low-level protective factors were independent prognostic factors. These results were consistent with previous research showing that LINC02418 functions as an oncogene through regulating the miR-4677-3p/SEC61G axis to accelerate the progression of NSCLC (Han, 2019). There is also a literature reporting that high expression of LINC02418 in lung adenocarcinoma promotes the growth of cancer cells (Wang et al., 2020). Considering the protective factors, we noted that SERPINB12 is abnormally expressed in the lung in previous reports, while there is no further explanation for its role (Niehaus et al., 2015). SERPINB12, like some other intracellular serine protease inhibitors, may play an important role in the barrier function by providing epithelial cell protection (Askew et al., 2001). Another article has reported that SERPINB12 maybe related to the abnormal growth and dysfunction of ovarian cancer. It is a potential biomarker and can be used for the early detection of ovarian cancer in women (Jo et al., 2014). Other LINC02683, AC244517.5, LINC01322, AC011468.3, AC020637.1, AC027117.2, and hsa-mir-6825 were reported for the first time in LUSC in the present study.
However, this study also had certain limitations. First, the sample size of the TCGA database was limited, and the effectiveness of the nine-gene model established in this study still needs to be further confirmed by large-sample clinical studies. Second, the TCGA database is a human genome project jointly launched by the National Cancer Institute and the National Human Genome Institute. We need to further expand the subjects of different races and perform multi-center research.
Collectively, our study successfully created a nine-gene signature that could efficiently screen outpatients with a high risk of recurrence after radical surgery of stage I-IIIA LUSC. This signature was a powerful complement to the prognostic factors of LUSC. However, a series of clinical samples are still needed to further validate its prognostic value in LUSC patients.
The datasets presented in this study can be found in online repositories. The names of the repository/repositories and accession number(s) can be found in the article/Supplementary Material.
LS and JL designed the study, performed the data analysis, and wrote the manuscript. XL, XY, and SZ acquired the data and carried out the bioinformatic analysis. XW, NW, and KX collected important background information and carried out a literature search. XJ and YZ drafted the manuscript. All authors read and approved the final manuscript.
This research was supported by grant from the Natural Science Foundation of Shandong Province, China (Grant No. ZR2020MH008) and Taishan Scholar Program of Shandong Province.
The authors declare that the research was conducted in the absence of any commercial or financial relationships that could be construed as a potential conflict of interest.
The Supplementary Material for this article can be found online at: https://www.frontiersin.org/articles/10.3389/fgene.2021.676464/full#supplementary-material
Askew, Y. S., Pak, S. C., Luke, C. J., Askew, D. J., Cataltepe, S., Mills, D. R., et al. (2001). SERPINB12 is a novel member of the human ov-serpin family that is widely expressed and inhibits trypsin-like serine proteinases. J. Biol. Chem. 276, 49320–49330. doi: 10.1074/jbc.m108879200
Bray, F., Ferlay, J., Soerjomataram, I., Siegel, R. L., Torre, L. A., and Jemal, A. (2018). Global cancer statistics 2018: GLOBOCAN estimates of incidence and mortality worldwide for 36 cancers in 185 countries. CA Cancer J. Clin. 68, 394–424. doi: 10.3322/caac.21492
Chen, R., Yang, X., Ding, Z., Zhu, L., Lu, S., and Yu, Y. (2019). Lung squamous cell carcinoma: a postoperative recurrence analysis of keratinizing and nonkeratinizing subtypes. Eur. J. Surg. Oncol. 45, 838–844. doi: 10.1016/j.ejso.2018.10.535
Chen, W. J., Tang, R. X., He, R. Q., Li, D. Y., Liang, L., Zeng, J. H., et al. (2017). Clinical roles of the aberrantly expressed lncRNAs in lung squamous cell carcinoma: a study based on RNA-sequencing and microarray data mining. Oncotarget 8, 61282–61304. doi: 10.18632/oncotarget.18058
Chi, Y., Wang, D., Wang, J., Yu, W., and Yang, J. (2019). Long non-coding RNA in the pathogenesis of cancers. Cells 8:1015. doi: 10.3390/cells8091015
Consonni, D., Pierobon, M., Gail, M. H., Rubagotti, M., Rotunno, M., Goldstein, A., et al. (2015). Lung cancer prognosis before and after recurrence in a population-based setting. J. Natl. Cancer Inst. 107:djv059.
Dziedzic, D. A., Rudzinski, P., Langfort, R., Orlowski, T., and Polish Lung Cancer Study Group (PLCSG) (2016). Risk factors for local and distant recurrence after surgical treatment in patients with non-small-cell lung cancer. Clin. Lung Cancer 17, e157–e167.
Ettinger, D. S., Wood, D. E., Aisner, D. L., Akerley, W., Bauman, J., Chirieac, L., et al. (2017). Non-Small cell lung cancer, Version 5.2017, NCCN clinical practice guidelines in oncology. J. Natl. Compr. Canc. Netw. 15, 504–535.
Gao, L., Guo, Y. N., Zeng, J. H., Ma, F., Luo, J., Zhu, H., et al. (2019). The expression, significance and function of cancer susceptibility candidate 9 in lung squamous cell carcinoma: a bioinformatics and in vitro investigation. Int. J. Oncol. 54, 1651–1664.
Gu, J. X., Zhang, X., Miao, R. C., Xiang, X., Fu, Y., Zhang, J., et al. (2019). Six-long non-coding RNA signature predicts recurrence-free survival in hepatocellular carcinoma. World J. Gastroenterol. 25, 220–232. doi: 10.3748/wjg.v25.i2.220
Han, B. (2019). LncRNA LINC02418 regulates proliferation and apoptosis of non-small cell lung cancer cells by regulating miR-4677-3p/SEC61G. Eur. Rev. Med. Pharmacol. Sci. 23, 10354–10362.
Hirsch, F. R., Scagliotti, G. V., Mulshine, J. L., Kwon, R., Curran, W. J. Jr., Wu, Y. L., et al. (2017). Lung cancer: current therapies and new targeted treatments. Lancet 389, 299–311. doi: 10.1016/s0140-6736(16)30958-8
Hou, J., Wang, Y., Zhang, H., Hu, Y., Xin, X., and Li, X. (2020). Silencing of LINC00461 enhances radiosensitivity of lung adenocarcinoma cells by down-regulating HOXA10 via microRNA-195. J. Cell. Mol. Med. 24, 2879–2890. doi: 10.1111/jcmm.14859
Iqbal, M. A., Arora, S., Prakasam, G., Calin, G. A., and Syed, M. A. (2019). MicroRNA in lung cancer: role, mechanisms, pathways and therapeutic relevance. Mol. Aspects Med. 70, 3–20. doi: 10.1016/j.mam.2018.07.003
Jo, G., Lim, W., Bae, S. M., Bazer, F., and Song, G. (2014). Avian SERPINB12 expression in the avian oviduct is regulated by estrogen and up-regulated in epithelial cell-derived ovarian carcinomas of laying hens. PLoS One 9:e99792. doi: 10.1371/journal.pone.0099792
Kamigaichi, A., Tsutani, Y., Fujiwara, M., Mimae, T., Miyata, Y., and Okada, M. (2019). Postoperative recurrence and survival after segmentectomy for clinical stage 0 or IA lung cancer. Clin. Lung Cancer 20, 397–403.e1.
Kataoka, Y., Igarashi, T., Ohshio, Y., Fujita, T., and Hanaoka, J. (2019). Predictive importance of galectin-3 for recurrence of non-small cell lung cancer. Gen. Thorac. Cardiovasc. Surg. 67, 704–711. doi: 10.1007/s11748-019-01074-x
Lee, R. C., Feinbaum, R. L., and Ambros, V. (1993). The C. elegans heterochronic gene lin-4 encodes small RNAs with antisense complementarity to lin-14. Cell 75, 843–854. doi: 10.1016/0092-8674(93)90529-y
Leroy, T., Monnet, E., Guerzider, S., Jacoulet, P., Bari, B. D., Falcoz, P., et al. (2019). Let us not underestimate the long-term risk of SPLC after surgical resection of NSCLC. Lung Cancer 137, 23–30. doi: 10.1016/j.lungcan.2019.09.001
Li, B., Zhang, X., and Dong, Y. (2019). Nanoscale platforms for messenger RNA delivery. Wiley Interdiscip. Rev. Nanomed. Nanobiotechnol. 11:e1530. doi: 10.1002/wnan.1530
Li, Y., Gu, J., Xu, F., Zhu, Q., Ge, D., and Lu, C. (2018). Transcriptomic and functional network features of lung squamous cell carcinoma through integrative analysis of GEO and TCGA data. Sci. Rep. 8:15834.
Mcaleese, J., Taylor, A., Walls, G. M., and Hanna, G. G. (2019). Differential relapse patterns for non-small cell lung cancer subtypes adenocarcinoma and squamous cell carcinoma: implications for radiation oncology. Clin. Oncol. (R. Coll. Radiol.) 31, 711–719. doi: 10.1016/j.clon.2019.07.008
Mirgayazova, R., Khadiullina, R., Mingaleeva, R., Chasov, V., Gomzikova, M., Garanina, E., et al. (2019). Novel Isatin-based activator of p53 transcriptional functions in tumor cells. Mol. Biol. Res. Commun. 8, 119–128.
Niehaus, J. Z., Good, M., Jackson, L. E., Ozolek, J. A., Silverman, G. A., and Luke, C. J. (2015). Human SERPINB12 is an abundant intracellular serpin expressed in most surface and glandular epithelia. J. Histochem. Cytochem. 63, 854–865. doi: 10.1369/0022155415600498
Peng, K., Chen, E., Li, W., Cheng, X., Yu, Y., Cuiame>Yu, Y., Cui, Y., et al. (2020). A 16-mRNA signature optimizes recurrence-free survival prediction of Stages II and III gastric cancer. J. Cell. Physiol. 235, 5777–5786. doi: 10.1002/jcp.29511
Sim, J., Kim, Y., Kim, H., Shin, S., Kim, D., Paik, S., et al. (2018). Identification of recurrence-associated microRNAs in stage I lung adenocarcinoma. Medicine (Baltimore) 97:e10996. doi: 10.1097/md.0000000000010996
Subramanian, J., and Simon, R. (2010). Gene expression-based prognostic signatures in lung cancer: ready for clinical use? J. Natl. Cancer Inst. 102, 464–474. doi: 10.1093/jnci/djq025
Sun, L., Zhang, Z., Yao, Y., Li, W. Y., and Gu, J. (2020). Analysis of expression differences of immune genes in non-small cell lung cancer based on TCGA and ImmPort data sets and the application of a prognostic model. Ann. Transl. Med. 8:550. doi: 10.21037/atm.2020.04.38
Tsuboi, M., Ohira, T., Saji, H., Miyajima, K., Kajiwara, N., Uchida, O., et al. (2007). The present status of postoperative adjuvant chemotherapy for completely resected non-small cell lung cancer. Ann. Thorac. Cardiovasc. Surg. 13, 73–77.
Wan, Y. W., Qian, Y., Rathnagiriswaran, S., Castranova, V., and Guo, N. L. (2010). A breast cancer prognostic signature predicts clinical outcomes in multiple tumor types. Oncol. Rep. 24, 489–494.
Wang, T., Zhai, R., Lv, X., Wang, K., and Xu, J. (2020). LINC02418 promotes malignant behaviors in lung adenocarcinoma cells by sponging miR-4677-3p to upregulate KNL1 expression. BMC Pulm. Med. 20:217. doi: 10.1186/s12890-020-01229-0
Woody, N. M., Stephans, K. L., Andrews, M., Zhuang, T., Gopal, P., Xia, P., et al. (2017). A Histologic Basis for the Efficacy of SBRT to the lung. J. Thorac. Oncol. 12, 510–519. doi: 10.1016/j.jtho.2016.11.002
Wu, Y. C., Wei, N. C., Hung, J. J., Yeh, Y., Su, L., Hsu, W., et al. (2017). Generating a robust prediction model for stage I lung adenocarcinoma recurrence after surgical resection. Oncotarget 8, 79712–79721. doi: 10.18632/oncotarget.19161
Zhang, Y., Zheng, D., Xie, J., Li, Y., Wang, Y., Li, C., et al. (2018). Development and validation of web-based nomograms to precisely predict conditional risk of site-specific recurrence for patients with completely resected non-small cell lung cancer: a multiinstitutional study. Chest 154, 501–511. doi: 10.1016/j.chest.2018.04.040
Keywords: lung squamous cell carcinoma, recurrence, TCGA, RNA signature, biomarker
Citation: Sun L, Li J, Li X, Yang X, Zhang S, Wang X, Wang N, Xu K, Jiang X and Zhang Y (2021) A Combined RNA Signature Predicts Recurrence Risk of Stage I-IIIA Lung Squamous Cell Carcinoma. Front. Genet. 12:676464. doi: 10.3389/fgene.2021.676464
Received: 05 March 2021; Accepted: 20 May 2021;
Published: 14 June 2021.
Edited by:
Shuzhen Chen, Eastern Hepatobiliary Surgery Hospital, ChinaReviewed by:
Fazlur Rahman Talukdar, International Agency for Research on Cancer (IARC), FranceCopyright © 2021 Sun, Li, Li, Yang, Zhang, Wang, Wang, Xu, Jiang and Zhang. This is an open-access article distributed under the terms of the Creative Commons Attribution License (CC BY). The use, distribution or reproduction in other forums is permitted, provided the original author(s) and the copyright owner(s) are credited and that the original publication in this journal is cited, in accordance with accepted academic practice. No use, distribution or reproduction is permitted which does not comply with these terms.
*Correspondence: Xinquan Jiang, dGFpYW5qeHFAMTYzLmNvbQ==; Yi Zhang, Y256aGFuZzFAMTI2LmNvbQ==
†These authors have contributed equally to this work
Disclaimer: All claims expressed in this article are solely those of the authors and do not necessarily represent those of their affiliated organizations, or those of the publisher, the editors and the reviewers. Any product that may be evaluated in this article or claim that may be made by its manufacturer is not guaranteed or endorsed by the publisher.
Research integrity at Frontiers
Learn more about the work of our research integrity team to safeguard the quality of each article we publish.