FI-Net: Identification of Cancer Driver Genes by Using Functional Impact Prediction Neural Network
- 1Faculty of Electronic Information and Electrical Engineering, Dalian University of Technology, Dalian, China
- 2Department of Breast Surgery, Institute of Breast Disease, Second Hospital of Dalian Medical University, Dalian, China
A Corrigendum on
FI-Net: Identification of Cancer Driver Genes by Using Functional Impact Prediction Neural Network
by Gu, H., Xu, X., Qin, P., and Wang, J. (2020). Front. Genet. 11:564839. doi: 10.3389/fgene.2020.564839
In the original article, there was an error. The “ExInAtor” code was incorrectly used for a comparison with our method. ExInAtor is designed to only be used with mutations from Whole Genome Sequences, not with Whole Exome Sequences as was done in this article. As such, the ExInAtor results present in the Supplementary Material and those referenced in the text and Figures should be discounted.
A correction has been made to Figure 4. The corrected Figure 4 appears below.
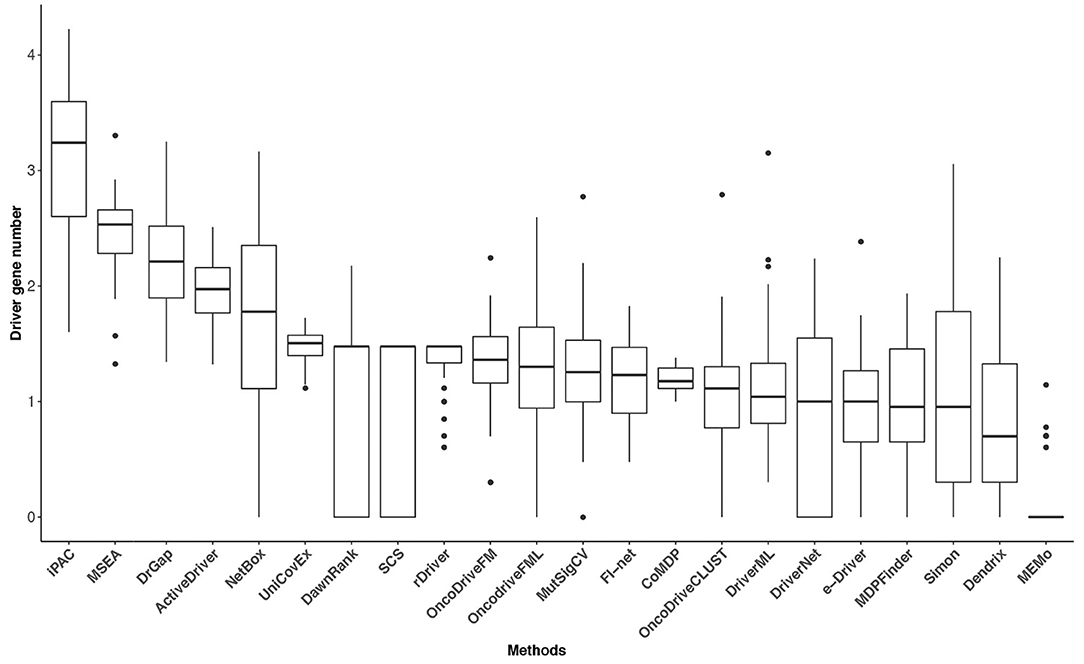
Figure 4. The driver gene number (on a log-10 scale) of the 22 methods in the 31 TCGA datasets. The numbers of driver genes predicted by different methods differ significantly.
Further, a correction has been made to Figure 5. The corrected Figure 5 appears below.
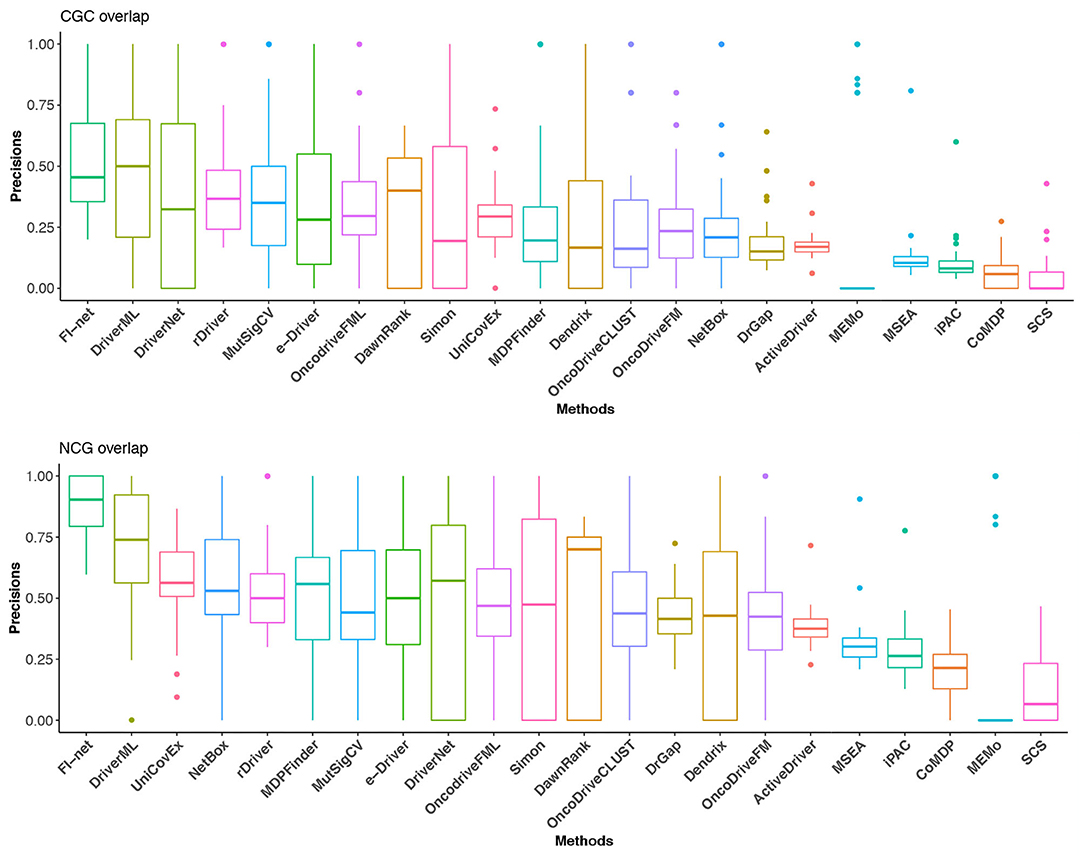
Figure 5. The precisions in CGC and NCG of the 22 methods in the 31 TCGA datasets. Methods are sorted with respect to the mean of precisions.
Lastly, a correction has been made to Table 1. The corrected Table 1 appears below.
The authors apologize for this error and state that this does not change the scientific conclusions of the article in any way. The original article has been updated.
Keywords: cancer research, driver genes, functional impact, artificial neural network, multi-omics features, hierarchical clustering algorithm
Citation: Gu H, Xu X, Qin P and Wang J (2021) Corrigendum: FI-Net: Identification of Cancer Driver Genes by Using Functional Impact Prediction Neural Network. Front. Genet. 12:675351. doi: 10.3389/fgene.2021.675351
Received: 03 March 2021; Accepted: 15 March 2021;
Published: 13 April 2021.
Edited and reviewed by: Yunyan Gu, Harbin Medical University, China
Copyright © 2021 Gu, Xu, Qin and Wang. This is an open-access article distributed under the terms of the Creative Commons Attribution License (CC BY). The use, distribution or reproduction in other forums is permitted, provided the original author(s) and the copyright owner(s) are credited and that the original publication in this journal is cited, in accordance with accepted academic practice. No use, distribution or reproduction is permitted which does not comply with these terms.
*Correspondence: Pan Qin, qp112cn@dlut.edu.cn; Jia Wang, wangjia77@hotmail.com