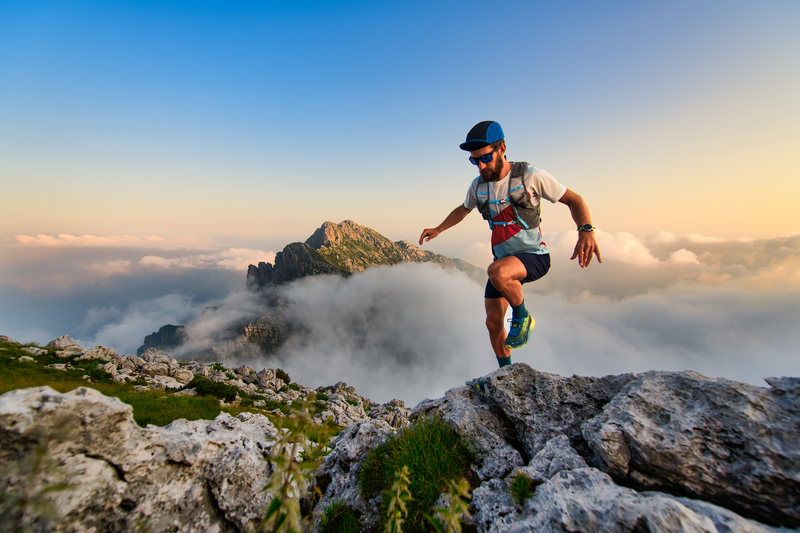
94% of researchers rate our articles as excellent or good
Learn more about the work of our research integrity team to safeguard the quality of each article we publish.
Find out more
ORIGINAL RESEARCH article
Front. Genet. , 31 August 2021
Sec. Statistical Genetics and Methodology
Volume 12 - 2021 | https://doi.org/10.3389/fgene.2021.673687
Background: Although several observational studies have attempted to investigate the association between type 2 diabetes mellitus (T2DM) and lung cancer risk, the results are controversial. Here, we intend to examine whether there is a causal association between T2DM and lung cancer risk.
Materials and Methods: We conducted a Mendelian randomization (MR) study to systematically investigate the effect of T2DM on lung cancer among 13,327 cases and 13,328 controls. A weighted genetic risk score (wGRS) was constructed as a proxy instrument by using 82 previously reported T2DM-related single nucleotide polymorphisms (SNPs). The logistic regression model was utilized to estimate associations of T2DM-related SNPs and wGRS with lung cancer risk. Sensitivity analyses were also performed to assess the robustness of the observed associations.
Results: We found no evidence for a causal relationship between T2DM and lung cancer risk (odds ratio, OR = 0.96, 95% confidence interval: 0.91–1.01, p = 0.96), and the association did not vary among populations of different age, sex, smoking status, and histological type. Sensitivity analyses (e.g., MR-Egger test) suggest that pleiotropic effects did not bias the result.
Conclusion: In this MR study with a large number of lung cancer cases, we found no evidence to support the causal role of T2DM in lung cancer risk. Further large-scale prospective studies are warranted to replicate our findings.
Lung cancer is one of the most commonly diagnosed cancers and the leading cause of cancer-related death globally (Sung et al., 2021). It is estimated that 2.24 million new lung cancer cases and 1.8 million deaths occurred worldwide in 2020 (Sung et al., 2021). Tobacco consumption is recognized as the most critical risk factor for lung cancer, and approximately 90% of the cases can be attributed to tobacco exposure (Doll and Hill, 1950). Genetic factors also play an important role in the carcinogenesis of the lung. In the past decade, genome-wide association studies (GWASs) report 51 lung cancer susceptibility loci in different ethnic populations and highlight suspected causal genes at each locus (Bosse and Amos, 2018; Dai et al., 2019). However, the reported variants contribute to only 18% of the heritability of lung cancer (Mucci et al., 2016). The risk factors of lung cancer remain short of explored.
As a metabolic disease, the prevalence of type 2 diabetes mellitus (T2DM) is increasing worldwide, especially in Asia (Zheng et al., 2018). The International Diabetes Federation estimates that the age-adjusted comparative prevalence of T2DM among 20- to 79-year-olds in China was 9.7% in 2017 although the European region has a lower prevalence of about 6.8% (International Diabetes Federation, 2017). Because inflammation, insulin resistance, hyperinsulinemia, and hyperglycemia, which are biological alterations frequently observed in T2DM patients, may promote the initiation and progression of tumors, multiple studies have attempted to investigate the association of T2DM with cancer risk (Coussens and Werb, 2002; Arcidiacono et al., 2012; Ryu et al., 2014; Vigneri et al., 2016). Recently, several studies have also explored the association between T2DM and lung cancer risk, but no consistent conclusions have been drawn possibly due to the small sample sizes and confounding factors (Hall et al., 2005; Kuriki et al., 2007; Ogunleye et al., 2009; Ehrlich et al., 2010; Luo et al., 2012; Lo et al., 2013). In addition, for the existence of reverse causation, previous observational studies may not evaluate the causality accurately.
Mendelian randomization (MR) provides a novel approach to unbiasedly infer the causal relationship between exposure and outcome by using genetic variants as instrumental variables (IVs; Smith and Ebrahim, 2003), which can be quickly and accurately detected in large-scale epidemiological studies. This approach has been successfully applied to estimate the causal effect of polyunsaturated fatty acids and mosaic loss of chromosome Y on lung cancer (Wang et al., 2017; Qin et al., 2019). Recently, a large-scale meta-analysis (36,614 cases and 155,150 controls of Japanese ancestry) was conducted to evaluate the genetic influence on T2DM (Suzuki et al., 2019), providing us an opportunity to investigate the association between T2DM and lung cancer using the MR approach.
In this study, by using the genotype data of 26,655 participants (13,327 cases and 13,328 controls) with Asian ancestry, we derived a weighted genetic risk score (wGRS) with 82 T2DM-associated variants reported in Suzuki et al. (2019) as the IVs and applied the MR approach to investigate the causal relationship between T2DM and lung cancer.
In this study, a total of 13,327 cases and 13,328 controls from previously published lung cancer GWASs were included (Hu et al., 2011; Dai et al., 2019): (i) the Global Screening Array (GSA) Project of Nanjing Medical University (NJMU GSA Project with 10,248 cases and 9,298 controls) (Dai et al., 2019); (ii) the NJMU GWAS with 2,126 cases and 3,077 controls (Hu et al., 2011); and (iii) the NJMU OncoArray GWAS with 953 cases and 953 controls (Dai et al., 2019). Informed consent was obtained from all the participants included in this study, and each study was approved by the Ethics and Human Subject Committee of Nanjing Medical University. The basic characteristics of the included participants are summarized in Supplementary Table 1.
Standard quality control processes were performed for each of these data sets to exclude unqualified samples and variants (Hu et al., 2011; Dai et al., 2019). Briefly, one single nucleotide polymorphism (SNP) was filtered out if it met one of the following criteria: (1) maps onto autosomal chromosomes, (2) had a call rate <95%, (3) had a minor allele frequency (MAF) in controls <0.005, or (4) showed a departure from Hardy–Weinberg equilibrium (HWE) in all samples (p ≤ 1 × 10–5) or deviated from HWE in the controls (p-value < 1.00 × 10–7) or cases (p-value < 1.00 × 10–12). We further excluded ineligible individuals if they (1) have overall genotype call rates less than 95%, (2) have gender discrepancies, (3) were duplicates or probable relatives (PI_HAT > 0.25), (4) have extreme heterozygosity rates (≥6 SD), or (5) were defined as outliers according to a principal component analysis (PCA) computed by EIGENSTRAT 3.0.
Imputation was performed for all data sets and has been described in our previous papers (Hu et al., 2011; Dai et al., 2019). Briefly, SHAPEIT V2 (Delaneau et al., 2011, 2013) was used to pre-phase the haplotypes. Then, IMPUTE2 (Howie et al., 2009) was used to impute ungenotyped SNPs to hg19 with the 1000 Genomes Project (Phase III integrated variant set across 2,504 samples1) as the reference. Poorly imputed variants with imputation quality score less than 0.40 were excluded from our analysis.
Of the 88 T2DM-associated loci reported in Suzuki et al. (2019), six variants were excluded from further analysis: (1) five variants located on chromosome X and (2) rs77792157 with a MAF < 0.01 in all data sets. In addition, because indels were excluded in our imputation process, eight indels reported in Suzuki et al. (2019) were replaced by SNPs in high linkage disequilibrium (LD) (r2> 0.40) (Supplementary Table 2). The remaining 82 SNPs were independent and not in LD with each other (r2 < 0.10). The LD was estimated in the East Asian (EAS) population from the 1000 Genomes Project Phase 3 data set.
A wGRS was constructed to predict T2DM for MR by multiplying the genotype dosage of 82 independent T2DM-increasing alleles with the following formula: , where βi is the beta coefficient of the ith SNP for T2DM from previous study (Suzuki et al., 2019) and SNPi is the dosage of the effect allele. We evaluated the association of genetically predicted T2DM and lung cancer risk in five data sets separately and then did a meta-analysis of these results. Associations of T2DM-related SNPs and wGRS with lung cancer risk were estimated by using a logistic regression model adjusted for age, sex, smoking status, and the first 10 principal components (PCs). Stratification analyses were performed based on age group (<60 and ≥60 years), gender, smoking status, and histology. In stratification analyses, a logistic regression model was also performed to evaluate the association with age, sex, smoking status, and the first 10 PCs as covariables when these were not the stratified factor. Cochran’s Q statistic was calculated to evaluate the heterogeneity between different subgroups.
In addition to the wGRS approach, the inverse-variance weighted (IVW) method with summary statistics of each genetic variant was also performed to assess the robustness of the association between genetically predicted T2DM and lung cancer risk (Burgess et al., 2013). The IVW regression function from the MR R package (Yavorska and Burgess, 2017) (0.4.1) was applied to evaluate the potential causal association between T2DM and lung cancer risk with T2DM-related SNPs as the IVs. Meanwhile, MR-Egger regression analysis was performed to evaluate the possible pleiotropic effect of this study’s genetic instruments (Bowden et al., 2015).
All statistical analyses were performed using PLINK (version 1.90) and R software (version 3.5.0). Two-sided p-values less than 0.05 were considered statistically significant.
A total of 13,327 cases and 13,328 cancer-free controls of Asian ancestry were included in this study. Of the included participants, 51.60% (6,876/13,325) of the lung cancer cases and 51.67% (6,886/13,327) of the controls were over 60 years old. The majority (65.75%; 8,761/13,325) histological type of the included lung cancer cases was adenocarcinoma. Detailed demographics of subjects included in this study are shown in Supplementary Table 1.
A total of 82 previously reported T2DM-related SNPs that achieved genome-wide significance (p ≤ 5.0 × 10–8) were included in the analysis. None of these SNPs had a significant association with lung cancer risk (p < 0.05/82), and the association between each variant with T2DM and risk of lung cancer is displayed in Figure 1. To evaluate the relationship between T2DM and lung cancer risk, we constructed a wGRS by using the genotype dosage of the abovementioned 82 SNPs. No statistically significant association was observed between genetically predicted T2DM and lung cancer [odds ratio (OR) = 0.96; 95% confidence interval (CI) = 0.91–1.01; p = 0.13; Pheterogeneity = 0.61; Figure 2] after adjusting for age, sex, smoking status, and the first 10 PCs.
Figure 1. Plots of the effect size of each single nucleotide polymorphism (SNP) on T2DM and lung cancer risk. The x-axis plots the previously published β-estimate for the association of each SNP with T2DM. The y-axis plots the β-estimate from the multivariate logistic regression model for the association of each SNP with lung cancer risk in our study population. Lines represent causal estimates from the different methods.
Stratification analyses were also performed based on age, sex, smoking status, and histological type to evaluate whether the association between genetically predicted T2DM and the risk of developing lung cancer varies among different subgroups. As shown in Figure 3, the associations between T2DM and lung cancer risk were similar among subgroups divided by age, sex, smoking status, and histological type (p-values for heterogeneity were 0.79, 0.77, 0.13, and 0.77, respectively). However, for those who were ever smokers, we identified a marginally significant association between genetically predicted T2DM risk and lung cancer risk (OR = 0.92; 95%CI = 0.85–0.99; p = 0.03) although no significant association was observed in never smokers (OR = 1.00; 95%CI = 0.93–1.07; p = 0.96; Pheterogeneity = 0.13).
To evaluate the robustness of the observed association between genetically predicted T2DM and lung cancer risk, the IVW method was also conducted, and the association showed no difference with main results (OR = 0.99; 95%CI = 0.98–1.00, p = 0.13; Figure 1). Similar results were observed in simple median (OR = 0.99; 95%CI = 0.98–1.00, p = 0.13; Figure 1) and weighted median methods. The result of MR-Egger regression analysis suggests that no potential pleiotropic effect exists for variants used in constructing the genetic instruments (p = 0.85).
In this study, by using genotype data of 26,655 participants with Asian ancestry and 82 previously reported T2DM-related SNPs, we found no strong evidence to support the causal role of T2DM in lung cancer risk. This study is one of the largest MR analyses on T2DM and lung cancer risk to the best of our knowledge, and the findings are robust in sensitivity analyses with different methods.
Increasing evidence indicates the association between T2DM and cancer risk, and several plausible mechanisms underlying carcinogenesis are proposed (Giovannucci et al., 2010). Inflammation, insulin resistance, hyperinsulinemia, and hyperglycemia are the main pathophysiological characteristics of T2DM, which may be implicated in the pathogenesis of cancer among patients with T2DM (Kahn et al., 2014). Proinflammatory pathways can promote malignant transformation of cell carcinogenesis by inducing the production of inflammatory mediators, upregulating the expression of anti-apoptotic genes, and stimulating cell proliferation as well as angiogenesis (Landskron et al., 2014). Hyperglycemia can regulate cancer cell behavior, such as proliferation, migration, invasion, and recurrence, by causing DNA damage and activating various signaling pathways (Cencioni et al., 2014; Duan et al., 2014). Insulin resistance and hyperinsulinemia ultimately lead to elevated plasma insulin concentration, which may stimulate tumor growth by inducing the mitogenic effect and increasing bioavailable insulin-like growth factor 1 (Kazer, 1995; Chappell et al., 2001).
In the past decades, observational studies report somewhat inconsistent results regarding T2DM and lung cancer (Ehrlich et al., 2010; Rao Kondapally Seshasai et al., 2011; Hu et al., 2020). In the Nurses’ Health Study and the Health Professionals Follow-up Study with 3,814 lung cancer cases observed, incident T2DM was associated with an increased risk of lung cancer (Hu et al., 2020). In the retrospective cohort study with 1,811,228 participants, individuals with T2DM are at increased risk of several pulmonary conditions (asthma, COPD, fibrosis, and pneumonia) but not lung cancer (Ehrlich et al., 2010). However, these studies still cannot control the influence of potential biases because of the nature of the observational study. The present study provides no evidence to support a causal association between genetically predicted T2DM and lung cancer risk using an MR approach, which may control unmeasured confounders and reverse causation.
The strengths of our study include the large sample size with 13,327 lung cancer cases and the use of 82 independent T2DM-associated SNPs, which increases the statistical power of our study. By using individual genotype data of 26,655 participants, we were able to explore if there are any differences in the effect between different subgroups. Last, the consistency of findings across various MR methods, each based on different assumptions regarding pleiotropy, suggests that potential bias was unlikely to exist. Meanwhile, our study also has some limitations. Initially, it is difficult to avoid the influence of potential pleiotropy completely in any MR study, which may lead to biased causal effect estimates (Bowden et al., 2015). However, the pleiotropic effect was not observed in MR-Egger regression, and similar results were observed in sensitivity analyses using several other robust models. Furthermore, the findings were limited because T2DM-associated SNPs were derived from the Japanese population. For there is currently no large-scale T2DM GWAS study based on the Chinese population available.
In conclusion, we do not find clear evidence for a causal role of genetically predicted T2DM in the risk of lung cancer in a large, well-powered study, suggesting that previous associations between T2DM and lung cancer are possibly confounded by potential biases or due to reverse causation.
The datasets generated for this study are available on request to the corresponding author.
The studies involving human participants were reviewed and approved by the Ethics Commission of the Nanjing Medical University. Written informed consent to participate in this study was provided by the participants’ legal guardian/next of kin.
TH, NQ, and JD contributed to the design and conception of the study. TH, NQ, and XZ did statistical analysis and wrote the manuscript. TH and XZ created the tables and figures. CW and YJ guided the manuscript writing. HM and JD corrected the manuscript draft and overall supervision of the project. All authors approved the final version of the manuscript.
This work was supported by National Key Research and Development Program of China (2019YFC1315700), the National Natural Science Foundation of China (81973123 and 81922061), and the Postgraduate Research & Practice Innovation Program of Jiangsu Province (KYCX19_1136).
The authors declare that the research was conducted in the absence of any commercial or financial relationships that could be construed as a potential conflict of interest.
All claims expressed in this article are solely those of the authors and do not necessarily represent those of their affiliated organizations, or those of the publisher, the editors and the reviewers. Any product that may be evaluated in this article, or claim that may be made by its manufacturer, is not guaranteed or endorsed by the publisher.
The Supplementary Material for this article can be found online at: https://www.frontiersin.org/articles/10.3389/fgene.2021.673687/full#supplementary-material
GWAS, genome-wide association study; T2DM, type 2 diabetes mellitus; MR, Mendelian randomization; IVs, instrumental variables; wGRS, weighted genetic risk score; GSA, Global Screening Array; SNP, single nucleotide polymorphism; MAF, minor allele frequency; HWE, Hardy–Weinberg equilibrium; PCA, principal component analysis; LD, linkage disequilibrium; PCs, principal components; IVW, inverse-variance weighted; OR, odds ratio; CI, confidence interval.
Arcidiacono, B., Iiritano, S., Nocera, A., Possidente, K., Nevolo, M. T., Ventura, V., et al. (2012). Insulin resistance and cancer risk: an overview of the pathogenetic mechanisms. Exp. Diabetes Res. 2012:789174. doi: 10.1155/2012/789174
Bosse, Y., and Amos, C. I. (2018). A decade of GWAS results in lung cancer. Cancer Epidemiol. Biomarkers Prev. 27, 363–379. doi: 10.1158/1055-9965.EPI-16-0794
Bowden, J., Davey Smith, G., and Burgess, S. (2015). Mendelian randomization with invalid instruments: effect estimation and bias detection through Egger regression. Int. J. Epidemiol. 44, 512–525. doi: 10.1093/ije/dyv080
Burgess, S., Butterworth, A., and Thompson, S. G. (2013). Mendelian randomization analysis with multiple genetic variants using summarized data. Genet. Epidemiol. 37, 658–665. doi: 10.1002/gepi.21758
Cencioni, C., Spallotta, F., Greco, S., Martelli, F., Zeiher, A. M., and Gaetano, C. (2014). Epigenetic mechanisms of hyperglycemic memory. Int. J. Biochem. Cell Biol. 51, 155–158. doi: 10.1016/j.biocel.2014.04.014
Chappell, J., Leitner, J. W., Solomon, S., Golovchenko, I., Goalstone, M. L., and Draznin, B. (2001). Effect of insulin on cell cycle progression in MCF-7 breast cancer cells. Direct and potentiating influence. J. Biol. Chem. 276, 38023–38028. doi: 10.1074/jbc.M104416200
Coussens, L. M., and Werb, Z. (2002). Inflammation and cancer. Nature 420, 860–867. doi: 10.1038/nature01322
Dai, J., Lv, J., Zhu, M., Wang, Y., Qin, N., Ma, H., et al. (2019). Identification of risk loci and a polygenic risk score for lung cancer: a large-scale prospective cohort study in Chinese populations. Lancet Respir. Med. 7, 881–891. doi: 10.1016/S2213-2600(19)30144-4
Delaneau, O., Marchini, J., and Zagury, J. F. (2011). A linear complexity phasing method for thousands of genomes. Nat. Methods 9, 179–181. doi: 10.1038/nmeth.1785
Delaneau, O., Zagury, J. F., and Marchini, J. (2013). Improved whole-chromosome phasing for disease and population genetic studies. Nat. Methods 10, 5–6. doi: 10.1038/nmeth.2307
Doll, R., and Hill, A. B. (1950). Smoking and carcinoma of the lung; preliminary report. Br. Med. J. 2, 739–748. doi: 10.1136/bmj.2.4682.739
Duan, W., Shen, X., Lei, J., Xu, Q., Yu, Y., Li, R., et al. (2014). Hyperglycemia, a neglected factor during cancer progression. Biomed. Res. Int. 2014:461917. doi: 10.1155/2014/461917
Ehrlich, S. F., Quesenberry, C. P. Jr., Van Den Eeden, S. K., Shan, J., and Ferrara, A. (2010). Patients diagnosed with diabetes are at increased risk for asthma, chronic obstructive pulmonary disease, pulmonary fibrosis, and pneumonia but not lung cancer. Diabetes Care 33, 55–60. doi: 10.2337/dc09-0880
Giovannucci, E., Harlan, D. M., Archer, M. C., Bergenstal, R. M., Gapstur, S. M., Habel, L. A., et al. (2010). Diabetes and cancer: a consensus report. Diabetes Care 33, 1674–1685. doi: 10.2337/dc10-0666
Hall, G. C., Roberts, C. M., Boulis, M., Mo, J., and MacRae, K. D. (2005). Diabetes and the risk of lung cancer. Diabetes Care 28, 590–594. doi: 10.2337/diacare.28.3.590
Howie, B. N., Donnelly, P., and Marchini, J. (2009). A flexible and accurate genotype imputation method for the next generation of genome-wide association studies. PLoS Genet. 5:e1000529. doi: 10.1371/journal.pgen.1000529
Hu, Y., Zhang, X., Ma, Y., Yuan, C., Wang, M., Wu, K., et al. (2020). Incident type 2 diabetes duration and cancer risk: a prospective study in two US cohorts. J. Natl. Cancer Inst. 113, 381–389. doi: 10.1093/jnci/djaa141
Hu, Z., Wu, C., Shi, Y., Guo, H., Zhao, X., Yin, Z., et al. (2011). A genome-wide association study identifies two new lung cancer susceptibility loci at 13q12.12 and 22q12.2 in Han Chinese. Nat. Genet. 43, 792–796. doi: 10.1038/ng.875
International Diabetes Federation (2017). IDF Diabetes Atlas, 8th Edn. Brussels: International Diabetes Federation.
Kahn, S. E., Cooper, M. E., and Del Prato, S. (2014). Pathophysiology and treatment of type 2 diabetes: perspectives on the past, present, and future. Lancet 383, 1068–1083. doi: 10.1016/S0140-6736(13)62154-6
Kazer, R. R. (1995). Insulin resistance, insulin-like growth factor I and breast cancer: a hypothesis. Int. J. Cancer 62, 403–406. doi: 10.1002/ijc.2910620408
Kuriki, K., Hirose, K., and Tajima, K. (2007). Diabetes and cancer risk for all and specific sites among Japanese men and women. Eur. J. Cancer Prev. 16, 83–89. doi: 10.1097/01.cej.0000228404.37858.40
Landskron, G., De la Fuente, M., Thuwajit, P., Thuwajit, C., and Hermoso, M. A. (2014). Chronic inflammation and cytokines in the tumor microenvironment. J. Immunol. Res. 2014:149185. doi: 10.1155/2014/149185
Lo, S. F., Chang, S. N., Muo, C. H., Chen, S. Y., Liao, F. Y., Dee, S. W., et al. (2013). Modest increase in risk of specific types of cancer types in type 2 diabetes mellitus patients. Int. J. Cancer 132, 182–188. doi: 10.1002/ijc.27597
Luo, J., Chlebowski, R., Wactawski-Wende, J., Schlecht, N. F., Tinker, L., and Margolis, K. L. (2012). Diabetes and lung cancer among postmenopausal women. Diabetes Care 35, 1485–1491. doi: 10.2337/dc11-2108
Mucci, L. A., Hjelmborg, J. B., Harris, J. R., Czene, K., Havelick, D. J., Scheike, T., et al. (2016). Familial risk and heritability of cancer among twins in nordic countries. JAMA 315, 68–76. doi: 10.1001/jama.2015.17703
Ogunleye, A. A., Ogston, S. A., Morris, A. D., and Evans, J. M. (2009). A cohort study of the risk of cancer associated with type 2 diabetes. Br. J. Cancer 101, 1199–1201. doi: 10.1038/sj.bjc.6605240
Qin, N., Li, N., Wang, C., Pu, Z., Ma, Z., Jin, G., et al. (2019). Association of mosaic loss of chromosome Y with lung cancer risk and prognosis in a chinese population. J. Thorac. Oncol. 14, 37–44. doi: 10.1016/j.jtho.2018.09.013
Rao Kondapally Seshasai, S., Kaptoge, S., Thompson, A., Di Angelantonio, E., Gao, P., Sarwar, N., et al. (2011). Diabetes mellitus, fasting glucose, and risk of cause-specific death. N. Engl. J. Med. 364, 829–841. doi: 10.1056/NEJMoa1008862
Ryu, T. Y., Park, J., and Scherer, P. E. (2014). Hyperglycemia as a risk factor for cancer progression. Diabetes Metab. J. 38, 330–336. doi: 10.4093/dmj.2014.38.5.330
Smith, G. D., and Ebrahim, S. (2003). ‘Mendelian randomization’: can genetic epidemiology contribute to understanding environmental determinants of disease? Int. J. Epidemiol. 32, 1–22. doi: 10.1093/ije/dyg070
Sung, H., Ferlay, J., Siegel, R. L., Laversanne, M., Soerjomataram, I., Jemal, A., et al. (2021). Global cancer statistics 2020: GLOBOCAN estimates of incidence and mortality worldwide for 36 cancers in 185 countries. CA Cancer J. Clin. 71, 209–249. doi: 10.3322/caac.21660
Suzuki, K., Akiyama, M., Ishigaki, K., Kanai, M., Hosoe, J., Shojima, N., et al. (2019). Identification of 28 new susceptibility loci for type 2 diabetes in the Japanese population. Nat. Genet. 51, 379–386. doi: 10.1038/s41588-018-0332-4
Vigneri, R., Goldfine, I. D., and Frittitta, L. (2016). Insulin, insulin receptors, and cancer. J. Endocrinol. Invest. 39, 1365–1376. doi: 10.1007/s40618-016-0508-7
Wang, C., Qin, N., Zhu, M., Chen, M., Xie, K., Cheng, Y., et al. (2017). Metabolome-wide association study identified the association between a circulating polyunsaturated fatty acids variant rs174548 and lung cancer. Carcinogenesis 38, 1147–1154. doi: 10.1093/carcin/bgx084
Yavorska, O. O., and Burgess, S. (2017). MendelianRandomization: an R package for performing Mendelian randomization analyses using summarized data. Int. J. Epidemiol. 46, 1734–1739. doi: 10.1093/ije/dyx034
Keywords: lung cancer, type 2 diabetes mellitus, Mendelian randomization, genome-wide association study, causation
Citation: Hong T, Qin N, Zhao X, Wang C, Jiang Y, Ma H and Dai J (2021) Investigation of Causal Effect of Type 2 Diabetes Mellitus on Lung Cancer: A Mendelian Randomization Study. Front. Genet. 12:673687. doi: 10.3389/fgene.2021.673687
Received: 06 March 2021; Accepted: 02 August 2021;
Published: 31 August 2021.
Edited by:
Hugues Aschard, Institut Pasteur, FranceReviewed by:
Shaoyu Li, University of North Carolina at Charlotte, United StatesCopyright © 2021 Hong, Qin, Zhao, Wang, Jiang, Ma and Dai. This is an open-access article distributed under the terms of the Creative Commons Attribution License (CC BY). The use, distribution or reproduction in other forums is permitted, provided the original author(s) and the copyright owner(s) are credited and that the original publication in this journal is cited, in accordance with accepted academic practice. No use, distribution or reproduction is permitted which does not comply with these terms.
*Correspondence: Juncheng Dai, ZGpjQG5qbXUuZWR1LmNu
†These authors have contributed equally to this work and share first authorship
Disclaimer: All claims expressed in this article are solely those of the authors and do not necessarily represent those of their affiliated organizations, or those of the publisher, the editors and the reviewers. Any product that may be evaluated in this article or claim that may be made by its manufacturer is not guaranteed or endorsed by the publisher.
Research integrity at Frontiers
Learn more about the work of our research integrity team to safeguard the quality of each article we publish.