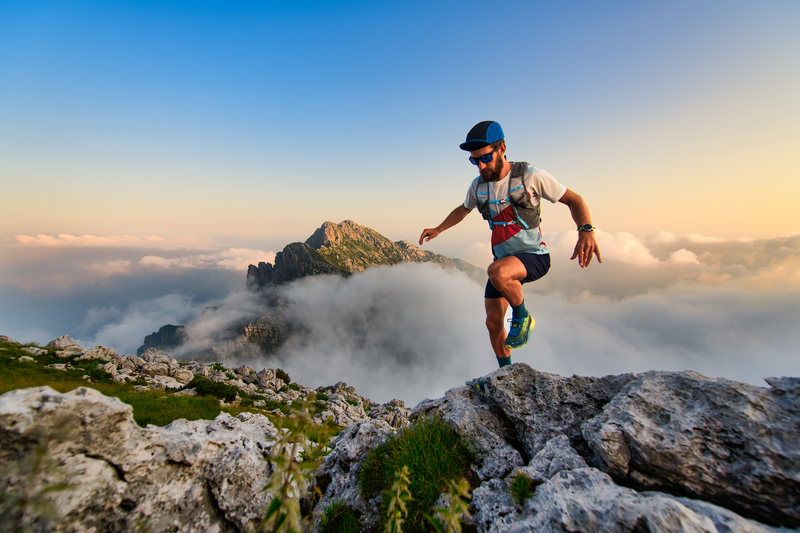
94% of researchers rate our articles as excellent or good
Learn more about the work of our research integrity team to safeguard the quality of each article we publish.
Find out more
ORIGINAL RESEARCH article
Front. Genet. , 08 July 2021
Sec. Computational Genomics
Volume 12 - 2021 | https://doi.org/10.3389/fgene.2021.668702
This article is part of the Research Topic Network Based Multi-Omics Analyses to Reveal Mechanisms and Biomarkers of Complex Diseases View all 8 articles
Heart failure with preserved ejection fraction (HFpEF) is a complex disease characterized by dysfunctions in the heart, adipose tissue, and cerebral arteries. The elucidation of the interactions between these three tissues in HFpEF will improve our understanding of the mechanism of HFpEF. In this study, we propose a multilevel comparative framework based on differentially expressed genes (DEGs) and differentially correlated gene pairs (DCGs) to investigate the shared and unique pathological features among the three tissues in HFpEF. At the network level, functional enrichment analysis revealed that the networks of the heart, adipose tissue, and cerebral arteries were enriched in the cell cycle and immune response. The networks of the heart and adipose tissues were enriched in hemostasis, G-protein coupled receptor (GPCR) ligand, and cancer-related pathway. The heart-specific networks were enriched in the inflammatory response and cardiac hypertrophy, while the adipose-tissue-specific networks were enriched in the response to peptides and regulation of cell adhesion. The cerebral-artery-specific networks were enriched in gene expression (transcription). At the module and gene levels, 5 housekeeping DEGs, 2 housekeeping DCGs, 6 modules of merged protein–protein interaction network, 5 tissue-specific hub genes, and 20 shared hub genes were identified through comparative analysis of tissue pairs. Furthermore, the therapeutic drugs for HFpEF-targeting these genes were examined using molecular docking. The combination of multitissue and multilevel comparative frameworks is a potential strategy for the discovery of effective therapy and personalized medicine for HFpEF.
Heart failure with preserved ejection fraction (HFpEF) is a common coronary disease characterized by left ventricular diastolic dysfunction, unimpaired left ventricular ejection fraction, and cardiac remodeling (Cuijpers et al., 2020). The mechanisms underlying HFpEF have not been completely elucidated, which has limited the development of effective drugs for HFpEF (Roh et al., 2017). HFpEF is a complex disease involving multiple genes and interactions between the adipose tissue, heart, and cerebral arteries (Borlaug, 2014; Altara et al., 2017; Cogswell et al., 2017; Kitzman and Nicklas, 2018). The interaction between tissues, which are involved in various biological functions, is involved in the pathogenesis of HFpEF. Thus, analyzing the shared and unique pathological features of these three tissues in HFpEF at the molecular level can aid in elucidating the underlying mechanisms and therapeutic targets.
The methods used in systems biology and high-throughput techniques have successfully reconstructed various disease-related networks for pathological conditions, such as cancer, type 2 diabetes mellitus (T2DM), and influenza. These methods enable the integration and interpretation of functional genomic datasets and the identification of novel biomarkers or modules, which can aid in the elucidation of the molecular mechanisms of diseases (Tang et al., 2018; Sun et al., 2019b; Wu Y. et al., 2020). In particular, multilevel analysis based on tissue-related networks can systemically reveal the pathophysiology of the disease through the integration of several target tissues and the identification of key pathways or biomarkers (Knaack et al., 2014; Sun et al., 2019a). Molecular docking can identify therapeutic drugs based on the results of network analysis (Gao et al., 2020). In particular, molecular docking can potentially identify therapies and enable the development of personalized medicine. Furthermore, molecular docking can aid in the discovery of the binding sites of molecular compounds in key genes and the elucidation of the molecular effects of therapeutic agents on key genes.
Here, we propose a multitissue and multilevel comparative framework to identify effective therapies and personalized medicine for HFpEF. A detailed flowchart (drawn using Pathway Builder Toll 2.0) is shown in Figure 1. In addition to the differentially expressed genes (DEGs), we propose differentially correlated gene pairs (DCGs) to obtain multigene information. A wild-type disease-related network (WDRN) was generated by integrating DEGs and protein–protein interaction (PPI) networks. Next, an intensive-type disease-related network was generated based on the identified DCGs. The network structure was explored by extracting densely connected network components using the molecular complex detection (MCODE) algorithm in the merged network of the three tissue-specific disease-related networks. Thus, we performed a comprehensive analysis at the gene, network, and module levels and presented the shared and unique pathophysiological features of the cerebral artery, adipose tissue, and heart in HFpEF. The Comparative Toxicogenomics Database (CTD) was used to verify the results of this study. The hub genes characterized in this study were closely related to HFpEF in humans. This indicated that these genes are effective personalized therapeutic targets that can indicate the efficacy of HFpEF treatment. Finally, molecular docking was performed to identify personalized therapeutic agents for HFpEF.
Figure 1. The flowchart of this research. We proposed a multiple-tissue and multilevel comparative framework based on differentially expressed genes and differentially correlated gene pairs for heart failure with preserved ejection fraction (HFpEF).
Twelve specific pathogen-free male Dahl salt-sensitive rats (aged 6-week-old and weighing 160–180 g) (Certificate No. 2016-0006) were obtained from the Charles River Animal Laboratory (Beijing, China). The rats were housed under the following controlled conditions: circadian conditions, 12-h dark/light cycle; temperature, 20–24°C; relative humidity, 40–60%; noise levels, ≤ 60 dB. The rats were randomly divided into the following two groups (six rats/group): HFpEF group, fed on chow supplemented with 8% NaCl for 11 weeks, and control group, fed on chow supplemented with 0.3% NaCl for 11 weeks. The adipose tissue was collected from the HFpEF and control groups after 11 weeks. All experiments were approved by the Animal Ethics Committee of the Shandong University of Traditional Chinese Medicine (Ethics No. SDUTCM2018071501). The total RNA was extracted from the samples and quantified. Oligo (dT) magnetic beads were used to enrich the messenger RNA (mRNA). The complementary DNA (cDNA) library was quantified using a Qubit Fluorometer, hermo Fisher Scientific, United States. The insert size of the cDNA library was analyzed using an Agilent 2100 bioanalyzer (Agilent Technologies, Sta. Clara, CA, United States). Finally, the cDNA was sequenced using the synthesis method. The gene expression profiles of the heart (accession ID: GSE126062) and cerebral artery (accession ID: GSE5488) were obtained from the Gene Expression Omnibus database1. The GSE126062 and GSE5488 datasets contained six samples (three healthy samples and three HFpEF samples).
RNA degradation and contamination was monitored on 1% agarose gels. A NanoPhotometer® spectrophotometer (IMPLEN, Westlake Village, CA, United States) was used to check the purity of RNA. RNA integrity was checked by RNA Nano 6000 Assay Kit of the Bioanalyzer 2100 system (Agilent Technologies). A total amount of 1 μg RNA per sample was regarded as RNA sample for mRNA sequencing. Poly-T oligo-attached magnetic beads was used to obtain total mRNA from the RNA sample. M-MuLV Reverse Transcriptase (RNase H-) and random hexamer primer were used to obtain the first-strand cDNA. DNA Polymerase I and RNase H were used for second-strand cDNA synthesis. cDNAs of 250–300 bp were selected, and purified by AMPure XP system (Beckman Coulter, Beverly, MA, United States). USER Enzyme (NEB, Ipswich, MA, United States) was used to the adaptor-ligated cDNA. Adaptor-ligated cDNA, Phusion High-Fidelity DNA polymerase, and Universal PCR primers were used for PCR. In addition, the AMPure XP system, Beckman Coulter, USA was used to purify the PCR products, and library quality was assessed by Agilent Bioanalyzer 2100 system. The clustering of the index-coded samples was performed on a cBot Cluster Generation System using TruSeq PE Cluster Kit v3-cBot-HS (Illumina, San Diego, CA, United States) according to the manufacturer’s instructions. After cluster generation, the library preparations were sequenced on an Illumina Hiseq platform, and 125 bp/150 bp paired-end reads were generated.
The raw data in the GSE126062 and GSE5488 datasets were subjected to probe summarization and logarithmic transformation. The expression matrix was constructed using the robust multiarray average, and the probe ID was converted to a gene symbol. The DEGs in the heart, adipose tissue, and cerebral arteries were identified using the R package “limma” with the following parameters: p < 0.05 and | log2 fold change (FC)| > 0.5. The correlation between co-DEPs and cardiovascular diseases was examined using CTD2.
The PPI network was downloaded from STRING (version 11.03). We first downloaded the PPI network scored links between proteins from STRING (version 11.0, see text footnote 3) and reserved the interactions with scores above 900 at a confidence level (Sharma and Colonna, 2021). The edges were removed such that the vertices did not comprise DEGs. The DEGs and associated genes were identified, and a network was constructed with vertices comprising either DEGs or genes that directly interacted with a DEG. This network contained the molecular and functional factors perturbed in the disease condition as a wild-type control and was named WDRN.
The disease information was extracted from the constructed WDRN based on the disruption of PPI in the disease dataset. The interaction between genes or gene products (such as proteins) can be measured using the correlation coefficient. Thus, the disruption of one interaction in the disease dataset results in a change in its correlated interaction. If two gene expression vectors from a gene pair were highly correlated under a disease condition but were uncorrelated or exhibited low correlation under healthy conditions, they were defined as DCGs. The DCGs were considered disordered interactions caused by the disease.
To effectively measure the correlation of a gene pair in the datasets of three to five samples in each tissue, the correlation coefficient was considered, and the hypothesis was tested. Heterogeneity among expression profiles cannot be ruled out, as they were derived from multiple tissues and different platforms. Spearman’s correlation coefficient was used to measure the strength of the correlation between paired data. For the two random variables X = x1,x2,…,xn and Y = y1,y2,…,yn, Spearman’s correlation calculates Pearson’s correlation based on the ranked values of the data. If u1,u2,…,un denote the ranks of the n observed values of X and v1,v2,…,vn denote the ranks of the n observed values of Y, Spearman’s correlation coefficient is defined by the following equation:
where rs is the Spearman’s correlation coefficient, Suv is the sample covariance between u′s and v′s, and are the sample variances of v’s and u’s, respectively (Looney and Hagan, 2011).
Based on Spearman’s correlation coefficient, a significance test was performed to determine the linear correlation in X and Y. The correlation of a gene pair was considered significant at p < 0.05.
The Drugbank database4 was used to identify small-molecule compounds that target the genes observed in the identified MCODE components. The Protein Data Bank5 was used to determine the protein structures of these gene products. Water molecules and eutectic ligands were deleted from protein, and side chain fixation, residue repair, and hydrogenation also contribute to protein preparation. Molecular docking was performed in Surflex-Dock module of SYBYL 2.1. Energy optimization was carried out in AMBR7, and automatic mode was used to obtain the active pockets. In addition, the parameters for SYBYL 2.1 were set as default. The scores of molecular docking reflect the strength of the interactions between proteins and molecules.
In total, 427, 2,336, and 1,199 DEGs were identified in the cerebral artery, heart, and adipose tissue of the HFpEF group, respectively. Among these three tissues, only the following five housekeeping genes were identified: MLYCD (malonyl-CoA decarboxylase), FKBP5 (FKBP prolyl isomerase 5), PRKCD (protein kinase C, delta), SERPINE1 (serpin family E member 1), and GDA (guanine deaminase) (Figure 2A). The Venn diagram of DEGs among the three tissues and the biological process annotation for the five housekeeping genes provided a general and simple illustration of the pathophysiology of HFpEF (Figure 2B). For example, the biological processes of MLYCD, FKBP5, PRKCD, and GDA were associated with energy metabolism and immune response, while those of SERPINE1 were associated with the inflammatory response. This was consistent with the pathological phenotypes, including immune dysregulation, metabolic dysfunction, and activation of chronic inflammation, of various tissues in patients with HFpEF (Hage et al., 2020; Sava et al., 2020; Schiattarella et al., 2021).
Figure 2. The comparison of differentially expressed genes (DEGs) in the cerebral artery, heart, and adipose and biological processes related to housekeeping genes. (A) The comparison of DEGs in the heart, adipose, and cerebral artery. (B) The biological processes related to housekeeping genes.
In addition to individual genes, gene interactions are highly specific to the disease, as the functions of genes and gene products are dependent on biochemical or physical interactions. We assumed that molecular interactions are perturbed by genetic or epigenetic factors that lead to molecular dysfunctions associated with human diseases. To identify these interactions, a WDRN was constructed for each target tissue by integrating the DEGs and PPI network from STRING. For every edge, at least one vertex comprising DEGs between healthy and disease conditions was incorporated in the WDRN.
The tissue-specific WDRN can indicate global changes in the disease. The WDRN of the cerebral artery comprised 14,372 edges and 2,612 nodes encompassing 267 DEGs (10.22%). Meanwhile, the WDRN of the heart comprised 46,972 edges and 5,195 nodes comprising 859 DEGs (16.53%). Furthermore, the WDRN of the adipose tissue comprised 25,030 edges and 4,631 nodes encompassing 560 DEGs (12.09%).
For each tissue, the pathological interactions were captured based on the WDRN. Specifically, if two gene expression vectors from a gene pair were highly correlated under one condition (healthy or diseased condition) but uncorrelated or weakly correlated under the other condition (diseased or healthy condition), this gene pair was described as a DCG. The DCG interaction was considered a pathological interaction. In total, 783, 2,706, and 1,945 DCGs were identified for the cerebral artery, heart, and adipose tissue, respectively. Only PRKCD-LYZ2 and MLYCD-HSD17B4 (Figure 3A) were housekeeping DCGs for the three target tissues. The interaction between MLYCD and HSD17B4 influences fatty acid oxidation. Additionally, MLYCD and HSD17B4, which play an important role in mitochondrial function, lipid metabolism, and endothelial structure, are expressed in the cerebral artery and adipose tissue (Gautier et al., 2015; Chaanine et al., 2019; Jo et al., 2020). The PRKCD–LYZ2 pair is reported to be strongly correlated with the inflammation response (Xu et al., 2014) and may promote the activation of chronic systemic inflammation. These two housekeeping DCGs are closely correlated with HFpEF. However, further studies are needed to elucidate the specific correlation. An elementary functional analysis was performed for these two DCGs; the results are shown in Figure 3B. In Potential drug candidates by molecular docking for housekeeping DEGs and DCGs, we will further discuss drug discovery and molecular docking.
Figure 3. Two housekeeping differentially correlated gene pairs (DCGs). (A) List of housekeeping DCGs. (B) Function enrichment results of housekeeping DCGs.
For each tissue, the identified DCGs constitute an intensive-type disease-related network, which was termed differential network (DN). DN can characterize the phenotypic difference between the healthy and disease states at both molecular and interaction levels. In DN, each edge indicates the differential correlation of the molecular interaction between healthy and disease conditions. Each node or its linked node corresponds to the DEG or its product between healthy and diseased conditions. Thus, the DN could provide comprehensive and detailed information on HFpEF.
The cerebral artery DN comprised 783 edges and 779 nodes encompassing 163 DEGs (20.92%). The heart DN comprised 2,706 edges and 2,075 nodes encompassing 520 DEGs (25.06%), while the adipose tissue DN comprised 1,945 edges and 1,627 nodes encompassing 379 DEGs (23.29%). The coverage rate of DEGs in DN was higher than that in WDRNs. These results demonstrate that WDRN is a wild-type disease network, whereas DN is an intensive-type network.
The relevance of the three targeted tissues of HFpEF can be further investigated based on DNs. Figure 4 shows the Venn diagrams of the three tissue-specific DNs with edges, nodes, and covered DEGs. The edges, which indicate the differential interactions, were significantly different between the three tissues. Additionally, the DNs of the heart and adipose tissues exhibited high similarity. In addition, the characteristics of three tissue-specific differential networks are shown in Supplementary Table 1.
Figure 4. (A) The Venn diagram for edges among three tissue-dependent differential networks (DNs). (B) The Venn for nodes among three tissue-dependent DNs. (C) The Venn for differentially expressed genes (DEGs) covered by three tissue-dependent DNs.
Functional enrichment analysis of nonspecific genes in DN can indicate their relevance in the tissues. Consistent with the number of overlapping genes and interactions, the aberrant biological processes in the heart were similar to those in the adipose tissue. Shared biological processes among the cerebral artery, heart, and adipose tissue were closely related to cell cycle, mitosis, positive regulation of locomotion, adaptive immune system, signaling receptor tyrosine kinases, innate immune system, metabolism of RNA, and chemokine signaling pathway. The most common biological processes, including hemostasis, G-protein coupled receptor (GPCR) ligand binding, cancer-related pathway, mitotic G2–G2M phases, cytokine signaling in the immune system, and membrane trafficking, between the adipose tissue and heart were considered to be associated with HFpEF. For example, the cancer-related pathways were enriched. Cancer is a common comorbidity of HFpEF. Additionally, the treatment strategies for cancer increase the risk of HFpEF (Lund et al., 2014; Saiki et al., 2017). Gene expression (transcription) process was markedly enriched in the cerebral artery. The inflammatory response, regulation of vesicle-mediated transport, and regulation of cytosolic calcium ion concentration biological processes were specifically enriched in the heart. The response to peptides and regulation of cell adhesion were enriched in the adipose tissue (Figure 5A).
Figure 5. Functional enrichment analysis. (A) Functional enrichment analysis of nonspecific genes. (B) Functional enrichment analysis of tissue-specific genes.
The functional enrichment of tissue-specific genes was explored to identify the biological processes specific to each tissue. Consistent with the functional enrichment analysis results of nonspecific genes, the metabolism of RNA and processing of capped intron-containing pre-mRNA were highly enriched in the cerebral artery. Furthermore, rho GTPase signaling, response to peptides, and nonsense-mediated decay were significantly enriched in the adipose tissue, while other biological processes were similar to those obtained in the nonspecific gene enrichment analysis.
The innate immune system, response to extracellular stimuli, cytokine signaling in the immune system, adaptive immune system, detection of chemical stimuli involved in sensory perception of bitter taste, GPCR ligand binding, and G alpha signaling were highly enriched in the heart (Figure 5B).
Next, the tissue-specific hub genes were captured in the networks. Tissue-specific MCODE components were first divided based on the tissue-specific edges, and the components score higher than 3 were reserved in our study. MCODE algorithm could detect densely connected regions in large protein–protein interaction networks that may represent molecular complexes; it owns the advantage over other graph clustering methods of having a directed mode that allows fine tuning of clusters of interest without considering the rest of the network and allows examination of cluster interconnectivity, which is relevant for protein networks (Bader and Hogue, 2003). Three MCODE components were obtained for each tissue sample. The specific genes in these nine MCODE components were analyzed, and the genes with a score higher than 10 were selected as tissue-specific hub genes. In the cerebral artery, none of the genes exhibited a score higher than 5. Hence, POLR1C with a score of 5 was selected as the artery-specific hub gene. One, one, and three hub genes were identified for the cerebral artery, heart, and adipose tissue, respectively.
Figure 6 shows the hub genes and their first neighbors in the network. The hub genes exhibited tissue-specific expression. The tissue-specific hub genes are associated with specific biological functions of each tissue. IGFBP7 (insulin-like growth factor binding protein 7), KNG2 (kininogen 2), and CCL27 (C–C motif chemokine ligand 27) were the heart-specific hub genes. Previous studies have reported that the expression level of IGFBP7 is associated with diastolic function and exercise capacity in patients with HFpEF (Gandhi et al., 2016). The interaction between IGFBP7 and KNG2 was associated with the biological processes of the extracellular space and the severity of myocardial fibrosis in patients with HFpEF (Rommel et al., 2017). Additionally, the enhanced interaction between KNG2 and C5AR1 (C5a anaphylatoxin chemotactic receptor 1) promoted the expression of C5AR1 and activated chronic inflammation in the heart. Most edges of the adipose-tissue-specific network were linked to the hub gene CPD (carboxypeptidase D), which is involved in the posttranslational processes and influences the activation of peptides (Xin et al., 1997). Changes in the strength of the interactions between CPD and ARRB 1 (Arrestin Beta 1) may inhibit the activation of ARRB 1 and consequently affect the posttranslational processes (Xu et al., 2020). POLR1C (RNA polymerase I and III subunit C), which was the cerebral artery-specific hub gene, plays an important role in ribosome biogenesis (Noack Watt et al., 2016). Consistent with the biological function of POLR1C, genes linked to POLR1C exerted similar effects (Fujii et al., 2011; Ribeiro-Silva et al., 2018).
Figure 6. Tissue-specific hub genes and their corresponding modules. (A) IGFBP 7, KNG 2, and CCL 27. (B) CPD. (C) POLR1C. Gene colored in green means differential expression between disease and health conditions and was significantly downregulated under disease, while red means upregulated. One edge colored with green means the interaction between its two linked genes was perturbed and was inactivated under disease condition, while red means activated. The width of edges reflects the magnitude of the perturbation. Tissue-specific hub genes were shaped with round rectangle and labeled with blue.
Next, the shared and unique hub genes between the tissue pairs were examined. To achieve this, the common hub genes between tissue pairs were defined as those in Hub genes of tissue-specific genes. Based on the common hub genes, their neighbors in the corresponding tissue-specific DN and modules were selected. Thus, we observed the differences and similarities between the same hub genes in different tissues (Figure 7).
Figure 7. Hub genes of common genes between tissue pair and their corresponding modules. (A1) Cerebral artery and adipose in adipose. (A2) Cerebral artery and adipose in cerebral artery. (B1) Cerebral artery and heart in cerebral artery. (B2) Cerebral artery and heart in heart. (C1) Adipose and heart in adipose. (C2) Adipose and heart in heart. Gene colored in green was differentially expressed between disease and health conditions and was significantly downregulated under disease, while red means upregulated. Edge colored in green means the interaction between its two linked genes was perturbed and was inactivated under disease condition, while red means activated. The width of edges reflect the magnitude of the perturbation. Tissue-specific hub genes were shaped with round rectangle and labeled with blue.
Complement factor D (CFD), coagulation factor II thrombin receptor (F2R), mitogen-activated protein kinase 1 (MAPK1), mitochondrial ribosomal protein S35 (MRPS35), and PRKCD were important in both the adipose tissue and cerebral artery. Consistent with the common biological functions in the heart and adipose tissue, the interaction between PRKCD and F2R activates the biological process of positive regulation of the MAPK cascade. MAPK1 is closely related to the increased extracellular volume in the cerebral artery and adipose tissue, which is a characteristic in patients with HFpEF (Omori et al., 2020). In the cerebral artery, the interaction between MAPK1, FABP5 (recombinant human fatty acid-binding protein-5), and FN1 (fibronectin 1) was involved in the biological processes of response to wounding, which may accelerate the process of atherosclerosis (Pintucci et al., 2002). Additionally, IL6ST (interleukin 6 signal transducer) uniquely interacted with MAPK1 in the adipose tissue, which could influence the activation of the JAK-STAT3 pathway and regulate lipid metabolism (Odermatt et al., 2020).
Three common hub genes were identified between the cerebral artery and the heart. The function of these three hub genes is shown in Figure 7A. The hub gene ANAPC4 (anaphase-promoting complex subunit 4) was significantly upregulated in the artery but downregulated in the heart. Most interactions linked with ANAPC4 in the heart were inactivated in HFpEF. The expression of ANAPC4 was closely related to cell cycle in the heart and cerebral artery. The interaction between ANAPC4 and FBXL22 (F-box and leucine-rich protein 22) was weak in the heart, which may result in the upregulation of FBXL22 and impaired contractile function of the heart (Spaich et al., 2012). In the cerebral artery, ANAPC4 may increase the expression of PSMB1 (proteasome 20S subunit beta 1), which is closely related to vascular remodeling (Wang et al., 2013). The hub gene PRKCD was upregulated in both tissues. Several interactions linked with PRKCD were perturbed in the heart of patients with HFpEF but not in the artery. In this study, PRKCD was correlated with shared biological processes of the heart and cerebral artery. For example, PRKCD suppresses macro-/microautophagy (Zhang et al., 2017). PRKCD regulates the expression of VEGFR 2 (VEGF receptor 2) and PDGFR-β in arteries and is closely related to collateral vessel formation (Lizotte et al., 2013). However, the interaction between PRKCD and TTR (transthyretin) was enhanced in the heart and may promote the progression of cardiac amyloidosis in patients with HFpEF (Chen et al., 2018). GRN (Granulin) was activated in the cerebral artery and heart, and the interaction between GRN and related genes was enhanced in the heart. The interaction between LYZ2 (Lysozyme C-2) and GRN in the heart is associated with the activation of chronic inflammation (Wang C. et al., 2018). Additionally, GRN may influence the remodeling of the cerebral artery by positively regulating N2 (Niemann–Pick type C2) and inhibiting the activation of the ERK 1/2 MAPK signaling pathway (Csepeggi et al., 2011).
The following 12 hub genes were shared between the adipose tissue and the heart: ADRA1A (adrenoceptor alpha 1A), CCNF (cyclin F), CCR1 (C–C motif chemokine receptor 1), EGFR (epidermal growth factor receptor), MLYCD (malonyl-CoA decarboxylase), NRAS (NRAS proto-oncogene, GTPase), NSTR1 (neurotensin receptor 1), PRKCD, PTGFR (prostaglandin F receptor), SKP1 (S-phase kinase-associated protein 1), TTBK2 (tau tubulin kinase 2), and YKT6 (Ykt6 v-Snare homolog). These hub genes can activate the calcium signaling pathway and promote the dysfunction of the heart and adipose tissue (Huang and Chen, 2008; Li et al., 2008; Ye et al., 2014; Kao et al., 2015; Kuchay et al., 2017; Wang M. et al., 2018; Abe et al., 2020; da Silva Rosa et al., 2020; Esteves et al., 2020; Wu L. et al., 2020). CCR1, which was downregulated in the adipose tissue but significantly upregulated in the heart, is directly associated with more than 10 other genes in the two tissues. The major interactions of CCR1 were significantly activated in the heart but not in the adipose tissue. The interaction between CCR1 and MCHR 1 (melanin-concentrating hormone receptor 1) was upregulated in the adipose tissue, which promoted fat accumulation (Kring et al., 2008). However, the activation of CCR1 inhibited the expression of CXCL 11 (C–X–C motif chemokine 11) and consequently alleviated heart inflammation (Ramirez-Carracedo et al., 2020).
The modules effectively indicate the characteristics of the PPI network. The PPI networks of the cerebral artery, heart, and adipose tissue were merged. Six modules were identified in the merged PPI network (Figure 8A). Modules 1, 2, and 6 revealed the interactions between the heart and adipose tissue. Modules 3 and 5 indicate the interactions between the heart, adipose tissue, and cerebral arteries. Module 4 indicated the heart characteristics. The functional enrichment analysis of the six modules revealed the characteristics of HFpEF (Figure 8B). For example, ITGB1, PTK2, and ACTB in module 3 were involved in the biological processes of leukocyte transendothelial migration. This indicated that the migration of proinflammatory factors among these tissues promotes the activation of systemic chronic inflammation.
Figure 8. Modules in the merged protein–protein interaction (PPI) network. (A) The composition of the modules. (B) Function enrichment results of the modules.
The identified housekeeping DEGs and DCGs were closely related to the common biological functions of all three tissues. Thus, these genes may be effective therapeutic targets for common biological functions. The query of the CTD database indicated that these genes can be potential therapeutic targets for HFpEF in humans (Figure 9A). Fostamatinib may be the best choice for targeting the housekeeping DEGs and DCGs (Figures 9B–F), as it formed hydrogen bonds with GLN 87, HIS 84, ASP 330, HIS 279, SER 310, and ARG 213 of GDA, which suggested that the binding was strong and that fostamatinib may effectively regulate the activity of GDA. Further studies are needed to elucidate the structures of LYZ2 and HSD17B4. In addition, the docking scores are shown in Supplementary Table 2.
Figure 9. Housekeeping genes and housekeeping interactions. (A) The heatmap of score between heart failure with preserved ejection fraction (HFpEF) and genes of housekeeping genes and housekeeping interactions. (B) The binding site and interactions between fostamatinib and FKBP 5. (C) The binding site and interactions between fostamatinib and GDA. (D) The binding site and interactions between fostamatinib and MLYCD. (E) The binding site and interactions between fostamatinib and PRKCD. (F) The binding site and interactions between fostamatinib and SERPINE 1.
The modules of the merged PPI network were the representative biological functions in HFpEF, and the genes of all modules were the drug targets of the representative biological functions. The correlation between the genes of the modules and HFpEF is shown in Supplementary Figure 1A. The structure of some genes has not been elucidated and hence cannot be used to discover potential therapeutic agents through molecular docking. Benzalkonium and capsaicin may be potential drugs for targeting genes in module 1 (Supplementary Figure 1B). The docking scores of ginsenoside Rb1, vandetanib, ergocalciferol, and afatinib were the highest for the genes of module 2 (Supplementary Figure 1C). Dinoprostone and dinoprost tromethamine exhibited strong binding to the gene products of module 3 (Supplementary Figure 1D). Zinc oxide, baclofen, DL-dimyristoylphosphatidylglycerol, and dobutamine can be personalized medicine for targeting the genes of module 4 (Supplementary Figure 1E). Gentamicin and hydroxyethyl starch exhibited good binding to the gene products of module 5 (Supplementary Figure 1F). Adenosine may be an effective drug for targeting the genes in module 6 (Supplementary Figure 1G). Hydrogen bonds were formed between most compounds and genes of modules, which indicated that these compounds may effectively regulate the activity of these genes. In addition, the docking scores are shown in Supplementary Table 2.
The tissue-specific hub genes were involved in the specific biological functions of tissues. CPD, POLR1C, IGFBP7, KNG2, and CCL27 were closely related to HFpEF in humans (Supplementary Figure 2A). Guanidinoethylmercaptosuccinic acid may effectively target the tissue-specific genes (Supplementary Figures 2B,C), as it formed hydrogen bonds with the gene products. However, the structures of POLR1C, IGFBP7, and KNG2 have not been elucidated, and further studies are needed for the development of personalized medicine for HFpEF. In addition, the docking scores are shown in Supplementary Table 2.
The correlation between the common hub genes and HFpEF is shown in Supplementary Figure 3. Fostamatinib and 13-acetylphorbol exhibited the highest docking scores for the hub genes of adipose tissue and cerebral arteries [Supplementary Figures 3A(b–d)]. Erlotinib, lapatinib, nizatidine, and (2S)-8-[(tert-butoxycarbonyl) amino]-2-(1H-indol-3-yl) octanoic acid are potential drugs for targeting the hub genes of the heart and adipose tissue [Supplementary Figures 3B(b–j)]. Tamoxifen can be a potential drug for targeting the hub genes of the cerebral artery and heart [Supplementary Figures 3C(b–e)]. In addition, the docking scores are shown in Supplementary Table 2.
The progression of HFpEF, a systemic disease, is characterized by the dysfunction of the cerebral artery, adipose tissue, and heart. In this study, the shared and unique pathological features of the cerebral artery, adipose tissue, and heart in HFpEF were investigated at the network, gene, and module levels. Additionally, the correlation between key genes and HFpEF was investigated. Some small-molecule compounds showed beneficial effects on complex diseases (Zhao et al., 2019, 2021; He et al., 2021; Zheng et al., 2020). Furthermore, the Drugbank database and molecular docking were used to identify potential drug targets for HFpEF.
In total, 2,336, 427, and 1,199 DEGs were identified in the heart, cerebral artery, and adipose tissue. The following five housekeeping genes were identified in the three tissues: MLYCD, FKBP5, PRKCD, SERPINE1, and GDA. Additionally, housekeeping gene interactions, modules in the merged PPI networks, tissue-specific hub genes, and shared hub genes were identified using the multilevel comparative framework. These specific genes indicated the characteristics of HFpEF. Additionally, the results of the CTD database analysis revealed that these genes were closely related to HFpEF in humans, which indicated that these genes are potential therapeutic targets for the development of personalized therapy and precision medicine for HFpEF. A large part of treatment strategies for HFpEF were based on symptoms of HFpEF patients and expert consensus. Although mineralocorticoid antagonist, exercise therapy, and control hypervolemia showed beneficial effects on HFpEF, effective drugs are still lacking. The combination of molecular docking and multilevel comparative frameworks is a potential strategy for the discovery of effective therapy and personalized medicine for HFpEF. Fostamatinib, benzalkonium, capsaicin, baclofen, etc., maybe personalized medicine for HFpEF. Animal experiments will contribute to explore pharmacological functions of these drug on HFpEF, and some prospective clinical trials will help to identify the clinical effects of these drugs.
Functional enrichment analysis of nonspecific genes revealed that the aberrant biological processes in the heart were similar to those in the adipose tissue. Additionally, some biological processes were unique to the cerebral artery. Furthermore, the common biological processes among the three tissues were mainly related to cell cycle and immune response. These findings were consistent with those of previous studies, which demonstrated that cell cycle and immune response are closely related to diastolic dysfunction and impaired cardiac reserves (Roh et al., 2020; Sava et al., 2020). Heart function is related to the flow dynamics of the cerebral artery, which may influence the gene expression in the cerebral artery and increase the incidence of cerebrovascular events (Karahan et al., 2020). Additionally, the cell cycle and immune response are associated with the incidence of cerebrovascular events, which can lead to cardiac dysfunction (Min et al., 2009). Cell cycle and immune response are the main factors contributing to adipose tissue dysfunction, which could increase the level of proinflammatory adipocytokines and consequently contribute to cardiac dysfunction and cerebrovascular events (Packer, 2019). The housekeeping genes and housekeeping gene interactions are involved in these biological functions. This indicated that housekeeping genes may be effective targets for common biological processes. The results of molecular docking revealed that fostamatinib may be a potential drug for these housekeeping genes. Fostamatinib exerts beneficial effects on cell cycle and immune system in other diseases. Further clinical and basic studies are needed to examine the function of adenosine in HFpEF (Gomez-Puerta and Mócsai, 2013). Additionally, six modules were identified in the merged PPI network. The biological functions of these modules can indicate the differential characteristics of HFpEF. The modules were regarded as personalized treatment targets for HFpEF. Molecular docking of these modules will contribute to the discovery of precision medicine and personalized treatment strategies for HFpEF. The DEGs of modules 3 and 5 were identified in all three tissues. The biological processes of modules 3 and 5 were similar to the common biological processes among the three tissues. The binding of dinoprostone and dinoprost tromethamine was strong with the gene products of module 3. However, the effects of these drugs in HFpEF remain unclear. Gentamicin and hydroxyethyl exhibited strong binding with the gene products of module 5. However, further studies are needed to explore the function of these drugs in HFpEF. Modules 1, 2, and 6 comprised DEGs of the heart and adipose tissue. Thus, these modules may be effective targets for blocking the interaction between the heart and the adipose tissue. The major biological functions of module 1 are related to the dysfunction of adipose tissue and impaired heart function (Pachera et al., 2015). Adipose tissue dysfunction also leads to diastolic dysfunction and impaired cardiac reserve. Capsaicin was the most effective drug for targeting module 1. A previous study also indicated that capsaicin could improve muscle function and insulin resistance in an animal model of chronic heart failure (Okajima and Harada, 2008; Wang et al., 2010). However, further studies are needed to examine the beneficial effects of capsaicin on HFpEF. The biological function of module 2 is related to the inflammatory response (Ryzhov et al., 2017; Easter et al., 2020). The systemic proinflammatory state can exacerbate the symptoms of HFpEF (Sani et al., 2018). The docking scores of ginsenoside Rb1, vandetanib, ergocalciferol, and afatinib were high for the gene products of module 2. Ginsenoside Rb1, vandetanib, and ergocalciferol also exerted beneficial effects against inflammatory responses. Further studies are needed to explore the effects of afatinib on the inflammatory response. The genes of module 6 were enriched in steatosis and lipotoxicity (Yazıcı and Sezer, 2017; Mahmod et al., 2018), which are closely related to diastolic dysfunction. Adenosine may be an effective drug for targeting the genes in module 6. Previous studies have suggested that adenosine can improve tissue perfusion and heart function in an animal model of HFpEF (Davila et al., 2019). However, further clinical and experimental studies are needed to identify the function of adenosine. Module 4 comprised only heart-related DEGs. The biological function of module 4 was associated with the immune response. Zinc oxide, baclofen, DL-dimyristoylphosphatidylglycerol, and dobutamine were potential drugs for targeting the genes in module 4. Most of these drugs are reported to exert beneficial effects on the immune response (Zou et al., 2002; Crowley et al., 2015; Liu et al., 2020).
Genes involved in hemostasis, GPCR ligand, cancer-related pathway, chemokine signaling pathway, and membrane trafficking were upregulated in the heart and adipose tissue. Some studies have demonstrated that hemostasis and chemokine signaling pathways play important roles in HFpEF (Glezeva et al., 2015; Pei et al., 2017). The chemokine signaling pathway increases the secretion of inflammatory factors in the adipose tissue, which may exacerbate systemic proinflammatory state and promote the biological process of hemostasis in the heart. The GPCR ligand, cancer-related pathway, and membrane trafficking biological processes can provide novel insights into the role of the heart and adipose tissue in HFpEF. The hub genes of heart and adipose tissue, including ADRA1A, CCNF, CCR1, EGFR, MLYCD, NRAS, NTSR1, PRKCD, PTGFR, SKP1, TTBK2, and YKT6, were also associated with these pathways. The results of molecular docking revealed that erlotinib, lapatinib, nizatidine, and (2S)-8-[(tert-butoxycarbonyl) amino]-2-(1H-indol-3-yl) octanoic acid may be effective drugs for targeting these genes. Most of these drugs are reported to regulate these pathways (Akimoto et al., 2005; Li et al., 2019; Shen et al., 2019). Cell cycle, mitosis, positive regulation of locomotion, adaptive immune system, signaling receptor tyrosine kinases, innate immune system, metabolism of RNA, and chemokine signaling pathways are the shared pathways of the heart and cerebral artery. Previous studies have demonstrated that these pathways are associated with diastolic function and cerebral arterial perfusion. Brain hypoperfusion may induce hypertension, and impaired diastolic function contributes to brain hypoperfusion (Adamski et al., 2018; Walas et al., 2019). The hub genes of the heart and cerebral artery, including ANAPC4, GRN, and PRKCD, play an important role in these pathways. Tamoxifen may be an effective drug for targeting these genes. However, the effects of tamoxifen in HFpEF remain unclear. The common pathways of the adipose tissue and cerebral arteries are associated with cell development, chemotaxis, and apoptosis. A previous study also indicated that fostamatinib regulates cell development, chemotaxis, and apoptosis (Kuiatse et al., 2015). Further studies are needed to examine the role of fostamatinib in HFpEF.
Furthermore, the functional enrichment of all DEGs of the heart, adipose tissue, and cerebral arteries revealed that they are enriched in inflammatory response and cardiac hypertrophy in the heart. These biological processes are important pathophysiological mechanisms in HFpEF (Wu et al., 2001; Xuan et al., 2020). The enrichment analysis of tissue-specific genes also highlighted that immune response was significantly enriched in heart tissues, which is closely related to the inflammatory response and cardiac hypertrophy (Adzika et al., 2019). Some studies have also demonstrated that the heart-specific hub genes, including IGFBP7, CCL27, and KNG2, are involved in the immune response. Guanidinoethylmercaptosuccinic acid was an effective drug for targeting these genes. A previous study also indicated that guanidinoethylmercaptosuccinic acid could regulate the immune response in vitro (Lynch et al., 1988). Further studies are needed to examine if guanidinoethylmercaptosuccinic acid can exert similar beneficial effects in HFpEF. These results indicated that the response to peptides and regulation of cell adhesion are specific biological processes in the adipose tissue. Previous studies have demonstrated that the response to peptides is related to lipid metabolism and influences the regulation of cell adhesion, which is associated with the prognosis of HFpEF. The function enrichment analysis of tissue-specific genes indicated that lipid metabolism is enriched in the adipose tissue. The effect of CPD and guanidinoethylmercaptosuccinic acid on lipid metabolism is not clear. Gene expression (transcription) was enriched in the cerebral arteries. Furthermore, the functional enrichment analysis of tissue-specific genes revealed similar biological processes. POLR1C also exhibited related biological functions in a previous study (Gauquelin et al., 2019). The structure of POLR1C requires further investigation.
The multitissue and multilevel comparative analyses provided novel insights into the pathogenesis of HFpEF. MLYCD, FKBP5, PRKCD, SERPINE1, and GDA are housekeeping genes in the adipose tissue, heart, and cerebral arteries. The aberrant biological processes in the heart were similar to those in the adipose tissue. Cell cycle and immune system were common biological processes of the adipose tissue, heart, and cerebral arteries. Hemostasis, GPCR ligand, cancer-related pathway, chemokine signaling pathway, and membrane trafficking were significantly enriched in the heart and adipose tissue. Cell cycle, mitosis, positive regulation of locomotion, adaptive immune system, signaling receptor tyrosine kinases, innate immune system, metabolism of RNA, and chemokine signaling pathway were the common pathways of the heart and cerebral artery. The common pathways of the adipose tissue and cerebral artery were associated with cell development, chemotaxis, and apoptosis. Inflammatory response, cardiac hypertrophy, and immune response were enriched in the heart. Lipid metabolism is a specific biological function of the adipose tissue. Furthermore, gene expression (transcription) was enriched in the cerebral artery. Potential therapeutic agents for HFpEF were investigated using molecular docking.
All data used or analyzed in this study are available upon request by contacting the corresponding authors.
The animal study was reviewed and approved by Animal Ethics Committee of Shandong University of Traditional Chinese Medicine (Ethics No. SDUTCM2018071501).
SS and XL designed the study. SS performed the computation. GZ, SS, and QY analyzed the results and wrote the manuscript. YW revised the manuscript. All authors read and approved the submitted version of the manuscript.
This work has been supported by the Chinese National Natural Science Foundations (Nos. 81673970 and 11901272) and the National Key Research and Development Program of China (No. 2020YFA0712402).
The authors declare that the research was conducted in the absence of any commercial or financial relationships that could be construed as a potential conflict of interest.
The Supplementary Material for this article can be found online at: https://www.frontiersin.org/articles/10.3389/fgene.2021.668702/full#supplementary-material
Abe, S., Ueno, M., Nishitani, M., Akamatsu, T., Sato, T., Shimoda, M., et al. (2020). Citrus sudachi Peel Extract Suppresses Cell Proliferation and Promotes the Differentiation of Keratinocytes through Inhibition of the EGFR-ERK Signaling Pathway. Biomolecules 10:1468. doi: 10.3390/biom10101468
Adamski, M. G., Sternak, M., Mohaissen, T., Kaczor, D., Wierońska, J. M., Malinowska, M., et al. (2018). Vascular Cognitive Impairment Linked to Brain Endothelium Inflammation in Early Stages of Heart Failure in Mice. J. Am. Heart Assoc. 7:e007694. doi: 10.1161/jaha.117.007694
Adzika, G. K., Machuki, J. O., Shang, W., Hou, H., Ma, T., Wu, L., et al. (2019). Pathological cardiac hypertrophy: the synergy of adenylyl cyclases inhibition in cardiac and immune cells during chronic catecholamine stress. J. Mol. Med. 97, 897–907. doi: 10.1007/s00109-019-01790-0
Akimoto, M., Hashimoto, H., Shigemoto, M., Maeda, A., and Yamashita, K. (2005). Effects of antisecretory agents on angiogenesis during healing of gastric ulcers. J. Gastroenterol. 40, 685–689. doi: 10.1007/s00535-005-1611-2
Altara, R., Giordano, M., Nordén, E. S., Cataliotti, A., Kurdi, M., Bajestani, S. N., et al. (2017). Targeting Obesity and Diabetes to Treat Heart Failure with Preserved Ejection Fraction. Front. Endocrinol. 8:160. doi: 10.3389/fendo.2017.00160
Bader, G. D., and Hogue, C. W. (2003). An automated method for finding molecular complexes in large protein interaction networks. BMC Bioinformatics 4:2. doi: 10.1186/1471-2105-4-2
Borlaug, B. A. (2014). The pathophysiology of heart failure with preserved ejection fraction. Nat. Rev. Cardiol. 11, 507–515. doi: 10.1038/nrcardio.2014.83
Chaanine, A. H., Joyce, L. D., Stulak, J. M., Maltais, S., Joyce, D. L., Dearani, J. A., et al. (2019). Mitochondrial Morphology, Dynamics, and Function in Human Pressure Overload or Ischemic Heart Disease With Preserved or Reduced Ejection Fraction. Circ. Heart Fail. 12:e005131. doi: 10.1161/circheartfailure.118.005131
Chen, W., Ton, V. K., and Dilsizian, V. (2018). Clinical Phenotyping of Transthyretin Cardiac Amyloidosis with Bone-Seeking Radiotracers in Heart Failure with Preserved Ejection Fraction. Curr. Cardiol. Rep. 20:23. doi: 10.1007/s11886-018-0970-2
Cogswell, R. J., Norby, F. L., Gottesman, R. F., Chen, L. Y., Solomon, S., Shah, A., et al. (2017). High prevalence of subclinical cerebral infarction in patients with heart failure with preserved ejection fraction. Eur. J. Heart Fail. 19, 1303–1309. doi: 10.1002/ejhf.812
Crowley, T., Fitzpatrick, J. M., Kuijper, T., Cryan, J. F., O’Toole, O., O’Leary, O. F., et al. (2015). Modulation of TLR3/TLR4 inflammatory signaling by the GABAB receptor agonist baclofen in glia and immune cells: relevance to therapeutic effects in multiple sclerosis. Front. Cell Neurosci. 9:284. doi: 10.3389/fncel.2015.00284
Csepeggi, C., Jiang, M., Kojima, F., Crofford, L. J., and Frolov, A. (2011). Somatic cell plasticity and Niemann-Pick type C2 protein: fibroblast activation. J. Biol. Chem. 286, 2078–2087. doi: 10.1074/jbc.M110.135897
Cuijpers, I., Simmonds, S. J., van Bilsen, M., Czarnowska, E., González Miqueo, A., Heymans, S., et al. (2020). Microvascular and lymphatic dysfunction in HFpEF and its associated comorbidities. Basic Res. Cardiol. 115:39. doi: 10.1007/s00395-020-0798-y
da Silva Rosa, S. C., Martens, M. D., Field, J. T., Nguyen, L., Kereliuk, S. M., Hai, Y., et al. (2020). ∗BNIP3L/Nix-induced mitochondrial fission, mitophagy, and impaired myocyte glucose uptake are abrogated by PRKA/PKA phosphorylation. Autophagy 1–16. doi: 10.1080/15548627.2020.1821548 [Online ahead of print]
Davila, A., Tian, Y., Czikora, I., Li, J., Su, H., and Huo, Y. (2019). Adenosine Kinase Inhibition Augments Conducted Vasodilation and Prevents Left Ventricle Diastolic Dysfunction in Heart Failure With Preserved Ejection Fraction. Circ. Heart Fail. 12:e005762. doi: 10.1161/circheartfailure.118.005762
Easter, M., Garth, J., Harris, E. S., Shei, R. J., Helton, E. S., and Wei, Y. (2020). Fibroblast Growth Factor Receptor 4 Deficiency Mediates Airway Inflammation in the Adult Healthy Lung? Front. Med. 7:317. doi: 10.3389/fmed.2020.00317
Esteves, G. N. N., Ferraz, L. S., Alvarez, M. M. P., Costa, C. A. D., Lopes, R. M., Tersariol, I. L. D. S., et al. (2020). BRAF and NRAS mutated melanoma: different Ca(2+) responses, Na(+)/Ca(2+) exchanger expression, and sensitivity to inhibitors. Cell Calcium 90:102241. doi: 10.1016/j.ceca.2020.102241
Fujii, W., Nishimura, T., Kano, K., Sugiura, K., and Naito, K. (2011). CDK7 and CCNH are components of CDK-activating kinase and are required for meiotic progression of pig oocytes. Biol. Reprod. 85, 1124–1132. doi: 10.1095/biolreprod.111.091801
Gandhi, P. U., Gaggin, H. K., Redfield, M. M., Chen, H. H., Stevens, S. R., and Anstrom, K. J. (2016). Insulin-Like Growth Factor-Binding Protein-7 as a Biomarker of Diastolic Dysfunction and Functional Capacity in Heart Failure With Preserved Ejection Fraction: results From the RELAX Trial. JACC Heart Fail. 4, 860–869. doi: 10.1016/j.jchf.2016.08.002
Gao, J., Zhang, K., Wang, Y., Guo, R., Liu, H., and Jia, C. (2020). A machine learning-driven study indicates emodin improves cardiac hypertrophy by modulation of mitochondrial SIRT3 signaling. Pharmacol. Res. 155:104739. doi: 10.1016/j.phrs.2020.104739
Gauquelin, L., Cayami, F. K., Sztriha, L., Yoon, G., Tran, L. T., and Guerrero, K. (2019). Clinical spectrum of POLR3-related leukodystrophy caused by biallelic POLR1C pathogenic variants. Neurol. Genet. 5:e369. doi: 10.1212/nxg.0000000000000369
Gautier, S., Ouk, T., Pétrault, M., Pétrault, O., Bérézowski, V., and Bordet, R. (2015). PPAR-Alpha Agonist Used at the Acute Phase of Experimental Ischemic Stroke Reduces Occurrence of Thrombolysis-Induced Hemorrhage in Rats. PPAR Res. 2015:246329. doi: 10.1155/2015/246329
Glezeva, N., Voon, V., Watson, C., Horgan, S., McDonald, K., Ledwidge, M., et al. (2015). Exaggerated inflammation and monocytosis associate with diastolic dysfunction in heart failure with preserved ejection fraction: evidence of M2 macrophage activation in disease pathogenesis. J. Card. Fail. 21, 167–177. doi: 10.1016/j.cardfail.2014.11.004
Gomez-Puerta, J. A., and Mócsai, A. (2013). Tyrosine kinase inhibitors for the treatment of rheumatoid arthritis. Curr. Top. Med. Chem. 13, 760–773. doi: 10.2174/15680266113139990094
Hage, C., Löfgren, L., Michopoulos, F., Nilsson, R., Davidsson, P., and Kumar, C. (2020). Metabolomic Profile in HFpEF vs HFrEF Patients. J. Card Fail. 26, 1050–1059. doi: 10.1016/j.cardfail.2020.07.010
He, Y., Zhao, W., Dong, Z., Ji, Y., Li, M., Hao, Y., et al. (2021). A biodegradable antibacterial alginate/carboxymethyl chitosan/Kangfuxin sponges for promoting blood coagulation and full-thickness wound healing. Int. J. Biol. Macromol. 167, 182–192. doi: 10.1016/j.ijbiomac.2020.11.168
Huang, C. T., and Chen, C. H. (2008). Identification of gene transcripts in rat frontal cortex that are regulated by repeated electroconvulsive seizure treatment. Neuropsychobiology 58, 171–177. doi: 10.1159/000191123
Jo, D. S., Park, N. Y., and Cho, D. H. (2020). Peroxisome quality control and dysregulated lipid metabolism in neurodegenerative diseases. Exp. Mol. Med. 52, 1486–1495. doi: 10.1038/s12276-020-00503-9
Kao, D. P., Lowes, B. D., Gilbert, E. M., Minobe, W., Epperson, L. E., and Meyer, L. K. (2015). Therapeutic Molecular Phenotype of β-Blocker-Associated Reverse-Remodeling in Nonischemic Dilated Cardiomyopathy. Circ. Cardiovasc. Genet. 8, 270–283. doi: 10.1161/circgenetics.114.000767
Karahan, M., Kocabeyoglu, S. S., Kervan, U., Sert, D. E., Aygun, E., and Tola, M. (2020). Effects of continuous-flow left ventricular assist devices on cerebral hemodynamics. Artif. Organs 44, 457–464. doi: 10.1111/aor.13616
Kitzman, D. W., and Nicklas, B. J. (2018). Pivotal Role of Excess Intra-Abdominal Adipose in the Pathogenesis of Metabolic/Obese HFpEF. JACC Heart Fail. 6, 1008–1010. doi: 10.1016/j.jchf.2018.08.007
Knaack, S. A., Siahpirani, A. F., and Roy, S. (2014). A pan-cancer modular regulatory network analysis to identify common and cancer-specific network components. Canc. Informat. 13, 69–84. doi: 10.4137/cin.S14058
Kring, S. I., Larsen, L. H., Holst, C., Toubro, S., Hansen, T., and Astrup, A. (2008). Genotype-phenotype associations in obesity dependent on definition of the obesity phenotype. Obes. Facts 1, 138–145. doi: 10.1159/000137665
Kuchay, S., Giorgi, C., Simoneschi, D., Pagan, J., Missiroli, S., and Saraf, A. (2017). PTEN counteracts FBXL2 to promote IP3R3- and Ca(2+)-mediated apoptosis limiting tumour growth. Nature 546, 554–558. doi: 10.1038/nature22965
Kuiatse, I., Baladandayuthapani, V., Lin, H. Y., Thomas, S. K., Bjorklund, C. C., and Weber, D. M. (2015). Targeting the Spleen Tyrosine Kinase with Fostamatinib as a Strategy against Waldenström Macroglobulinemia. Clin. Cancer Res. 21, 2538–2545. doi: 10.1158/1078-0432.Ccr-14-1462
Li, G., Xie, Q., Yang, Z., Wang, L., Zhang, X., and Zuo, B. (2019). Sp1-mediated epigenetic dysregulation dictates HDAC inhibitor susceptibility of HER2-overexpressing breast cancer. Int. J. Cancer 145, 3285–3298. doi: 10.1002/ijc.32425
Li, H., Shao, J., Lu, X., Gao, Z., and Yu, Y. (2008). Identification of early responsive genes in human amnion epithelial FL cells induced by N-methyl-N’-nitro-N-nitrosoguanidine using oligonucleotide microarray and quantitative real-time RT-PCR approaches. Mutat. Res. 644, 1–10. doi: 10.1016/j.mrfmmm.2008.06.013
Liu, H., Hu, J., Mahfuz, S., and Piao, X. (2020). Effects of Hydrolysable Tannins as Zinc Oxide Substitutes on Antioxidant Status, Immune Function, Intestinal Morphology, and Digestive Enzyme Activities in Weaned Piglets. Animals 10:757. doi: 10.3390/ani10050757
Lizotte, F., Paré, M., Denhez, B., Leitges, M., Guay, A., and Geraldes, P. (2013). PKCδ impaired vessel formation and angiogenic factor expression in diabetic ischemic limbs. Diabetes 62, 2948–2957. doi: 10.2337/db12-1432
Looney, S. W., and Hagan, J. L. (2011). “Statistical Methods for Assessing Biomarkers and Analyzing Biomarker Data,” in Essential Statistical Methods for Medical Statistics, eds C. R. Rao, J. P. Miller, and D. C. Rao (Burlington: North Holland), 27–65.
Lund, L. H., Donal, E., Oger, E., Hage, C., Persson, H., and Haugen-Löfman, I. (2014). Association between cardiovascular vs. non-cardiovascular co-morbidities and outcomes in heart failure with preserved ejection fraction. Eur. J. Heart Fail. 16, 992–1001. doi: 10.1002/ejhf.137
Lynch, D. R., Venable, J. C., and Snyder, S. H. (1988). Enkephalin convertase in the heart: similar disposition to atrial natriuretic factor. Endocrinology 122, 2683–2691. doi: 10.1210/endo-122-6-2683
Mahmod, M., Pal, N., Rayner, J., Holloway, C., Raman, B., and Dass, S. (2018). The interplay between metabolic alterations, diastolic strain rate and exercise capacity in mild heart failure with preserved ejection fraction: a cardiovascular magnetic resonance study. J. Cardiovasc. Magn. Reson. 20:88. doi: 10.1186/s12968-018-0511-6
Min, J., Farooq, M. U., Greenberg, E., Aloka, F., Bhatt, A., and Kassab, M. (2009). Cardiac dysfunction after left permanent cerebral focal ischemia: the brain and heart connection. Stroke 40, 2560–2563. doi: 10.1161/strokeaha.108.536086
Noack Watt, K. E., Achilleos, A., Neben, C. L., Merrill, A. E., and Trainor, P. A. (2016). The Roles of RNA Polymerase I and III Subunits Polr1c and Polr1d in Craniofacial Development and in Zebrafish Models of Treacher Collins Syndrome. PLoS Genet. 12:e1006187. doi: 10.1371/journal.pgen.1006187
Odermatt, T. S., Dedual, M. A., Borsigova, M., Wueest, S., and Konrad, D. (2020). Adipocyte-specific gp130 signalling mediates exercise-induced weight reduction. Int. J. Obes. 44, 707–714. doi: 10.1038/s41366-019-0444-7
Okajima, K., and Harada, N. (2008). Promotion of insulin-like growth factor-I production by sensory neuron stimulation; molecular mechanism(s) and therapeutic implications. Curr. Med. Chem. 15, 3095–3112. doi: 10.2174/092986708786848604
Omori, T., Nakamori, S., Fujimoto, N., Ishida, M., Kitagawa, K., and Ichikawa, Y. (2020). Myocardial Native T(1) Predicts Load-Independent Left Ventricular Chamber Stiffness In Patients With HFpEF. JACC Cardiovasc. Imaging 13, 2117–2128. doi: 10.1016/j.jcmg.2020.05.030
Pachera, N., Papin, J., Zummo, F. P., Rahier, J., Mast, J., and Meyerovich, K. (2015). Heterozygous inactivation of plasma membrane Ca(2+)-ATPase in mice increases glucose-induced insulin release and beta cell proliferation, mass and viability. Diabetologia 58, 2843–2850. doi: 10.1007/s00125-015-3745-y
Packer, M. (2019). Disease-treatment interactions in the management of patients with obesity and diabetes who have atrial fibrillation: the potential mediating influence of epicardial adipose tissue. Cardiovasc. Diabetol. 18:121. doi: 10.1186/s12933-019-0927-9
Pei, J., Harakalova, M., den Ruijter, H., Pasterkamp, G., Duncker, D. J., and Verhaar, M. C. (2017). Cardiorenal disease connection during post-menopause: the protective role of estrogen in uremic toxins induced microvascular dysfunction. Int. J. Cardiol. 238, 22–30. doi: 10.1016/j.ijcard.2017.03.050
Pintucci, G., Moscatelli, D., Saponara, F., Biernacki, P. R., Baumann, F. G., and Bizekis, C. (2002). Lack of ERK activation and cell migration in FGF-2-deficient endothelial cells. FASEB J. 16, 598–600. doi: 10.1096/fj.01-0815fje
Ramirez-Carracedo, R., Tesoro, L., Hernandez, I., Diez-Mata, J., Piñeiro, D., and Hernandez-Jimenez, M. (2020). Targeting TLR4 with ApTOLL Improves Heart Function in Response to Coronary Ischemia Reperfusion in Pigs Undergoing Acute Myocardial Infarction. Biomolecules 10:1167. doi: 10.3390/biom10081167
Ribeiro-Silva, C., Aydin, ÖZ., Mesquita-Ribeiro, R., Slyskova, J., Helfricht, A., and Marteijn, J. A. (2018). DNA damage sensitivity of SWI/SNF-deficient cells depends on TFIIH subunit p62/GTF2H1. Nat. Commun. 9:4067. doi: 10.1038/s41467-018-06402-y
Roh, J., Houstis, N., and Rosenzweig, A. (2017). Why Don’t We Have Proven Treatments for HFpEF? Circ. Res. 120, 1243–1245. doi: 10.1161/circresaha.116.310119
Roh, J. D., Houstis, N., Yu, A., Chang, B., Yeri, A., and Li, H. (2020). Exercise training reverses cardiac aging phenotypes associated with heart failure with preserved ejection fraction in male mice. Aging Cell 19:e13159. doi: 10.1111/acel.13159
Rommel, K. P., Lücke, C., and Lurz, P. (2017). Diagnostic and Prognostic Value of CMR T(1)-Mapping in Patients With Heart Failure and Preserved Ejection Fraction. Rev. Esp. Cardiol. 70, 848–855. doi: 10.1016/j.rec.2017.02.018
Ryzhov, S., Matafonov, A., Galindo, C. L., Zhang, Q., Tran, T. L., and Lenihan, D. J. (2017). ERBB signaling attenuates proinflammatory activation of nonclassical monocytes. Am. J. Physiol. Heart Circ. Physiol. 312, H907–H918. doi: 10.1152/ajpheart.00486.2016
Saiki, H., Petersen, I. A., Scott, C. G., Bailey, K. R., Dunlay, S. M., and Finley, R. R. (2017). Risk of Heart Failure With Preserved Ejection Fraction in Older Women After Contemporary Radiotherapy for Breast Cancer. Circulation 135, 1388–1396. doi: 10.1161/circulationaha.116.025434
Sani, C. M., Pogue, E. P. L., Hrabia, J. B., Zayachkowski, A. G., Zawadka, M. M., and Poniatowski, A. G. (2018). Association between low-grade chronic inflammation and depressed left atrial compliance in heart failure with preserved ejection fraction: a retrospective analysis. Folia Med. Cracov. 58, 45–55. doi: 10.24425/fmc.2018.124657
Sava, R. I., Pepine, C. J., and March, K. L. (2020). Immune Dysregulation in HFpEF: a Target for Mesenchymal Stem/Stromal Cell Therapy. J. Clin. Med. 9:241. doi: 10.3390/jcm9010241
Schiattarella, G. G., Rodolico, D., and Hill, J. A. (2021). Metabolic inflammation in heart failure with preserved ejection fraction. Cardiovasc. Res. 117, 423–434. doi: 10.1093/cvr/cvaa217
Sharma, A., and Colonna, G. (2021). System-Wide Pollution of Biomedical Data: consequence of the Search for Hub Genes of Hepatocellular Carcinoma Without Spatiotemporal Consideration. Mol. Diagn. Ther. 25, 9–27. doi: 10.1007/s40291-020-00505-3
Shen, T., Cheng, X., Xia, C., Li, Q., Gao, Y., and Pan, D. (2019). ∗Erlotinib inhibits colon cancer metastasis through inactivation of TrkB-dependent ERK signaling pathway. J. Cell. Biochem. doi: 10.1002/jcb.28400 [Online ahead of print]
Spaich, S., Will, R. D., Just, S., Spaich, S., Kuhn, C., and Frank, D. (2012). F-box and leucine-rich repeat protein 22 is a cardiac-enriched F-box protein that regulates sarcomeric protein turnover and is essential for maintenance of contractile function in vivo. Circ. Res. 111, 1504–1516. doi: 10.1161/circresaha.112.271007
Sun, S., Sun, F., and Wang, Y. (2019a). Multi-Level Comparative Framework Based on Gene Pair-Wise Expression Across Three Insulin Target Tissues for Type 2 Diabetes. Front. Genet. 10:252. doi: 10.3389/fgene.2019.00252
Sun, S., Yu, X., Sun, F., Tang, Y., Zhao, J., and Zeng, T. (2019b). Dynamically characterizing individual clinical change by the steady state of disease-associated pathway. BMC Bioinformatics 20:697. doi: 10.1186/s12859-019-3271-x
Tang, W., Wan, S., Yang, Z., Teschendorff, A. E., and Zou, Q. (2018). Tumor origin detection with tissue-specific miRNA and DNA methylation markers. Bioinformatics 34, 398–406. doi: 10.1093/bioinformatics/btx622
Walas, D., Nowicki-Osuch, K., Alibhai, D., von Linstow Roloff, E., Coghill, J., Waterfall, C., et al. (2019). Inflammatory pathways are central to posterior cerebrovascular artery remodelling prior to the onset of congenital hypertension. J. Cereb. Blood Flow Metab. 39, 1803–1817. doi: 10.1177/0271678x18769180
Wang, C., Wang, F., Cao, Q., Li, Z., Huang, L., and Chen, S. (2018). The Effect of Mecp2 on Heart Failure. Cell. Physiol. Biochem. 47, 2380–2387. doi: 10.1159/000491610
Wang, H. J., Li, Y. L., Gao, L., Zucker, I. H., and Wang, W. (2010). Alteration in skeletal muscle afferents in rats with chronic heart failure. J. Physiol. 588, 5033–5047. doi: 10.1113/jphysiol.2010.199562
Wang, J., Xu, L., Yun, X., Yang, K., Liao, D., and Tian, L. (2013). Proteomic analysis reveals that proteasome subunit beta 6 is involved in hypoxia-induced pulmonary vascular remodeling in rats. PLoS One 8:e67942. doi: 10.1371/journal.pone.0067942
Wang, M., Chen, F., Wang, J., Chen, X., Liang, J., and Yang, X. (2018). Calcium phosphate altered the cytokine secretion of macrophages and influenced the homing of mesenchymal stem cells. J. Mater. Chem. B 6, 4765–4774. doi: 10.1039/c8tb01201f
Wu, G., Yussman, M. G., Barrett, T. J., Hahn, H. S., Osinska, H., and Hilliard, G. M. (2001). Increased myocardial Rab GTPase expression: a consequence and cause of cardiomyopathy. Circ. Res. 89, 1130–1137. doi: 10.1161/hh2401.100427
Wu, L., Zhou, R., Diao, J., Chen, X., Huang, J., and Xu, K. (2020). Differentially expressed circular RNAs in orbital adipose/connective tissue from patients with thyroid-associated ophthalmopathy. Exp. Eye Res. 196:108036. doi: 10.1016/j.exer.2020.108036
Wu, Y., Li, T., Liu, X., and Chen, L. (2020). Differential network inference via the fused D-trace loss with cross variables. Electron. J. Statist. 14, 1269–1301. doi: 10.1214/20-EJS1691
Xin, X., Varlamov, O., Day, R., Dong, W., Bridgett, M. M., Leiter, E. H., et al. (1997). Cloning and sequence analysis of cDNA encoding rat carboxypeptidase D. DNA Cell Biol. 16, 897–909. doi: 10.1089/dna.1997.16.897
Xu, X., Hicks, C., Li, Y., Su, J., Shiloach, J., and Kaufman, J. B. (2014). Purified cell wall from the probiotic bacterium Lactobacillus gasseri activates systemic inflammation and, at higher doses, produces lethality in a rat model. Crit. Care 18:R140. doi: 10.1186/cc13966
Xu, X., Zhang, Z., Lu, Y., Sun, Q., Liu, Y., and Liu, Q. (2020). ARRB1 ameliorates liver ischaemia/reperfusion injury via antagonizing TRAF6-mediated Lysine 6-linked polyubiquitination of ASK1 in hepatocytes. J. Cell. Mol. Med. 24, 7814–7828. doi: 10.1111/jcmm.15412
Xuan, L., Zhu, Y., Liu, Y., Yang, H., Wang, S., and Li, Q. (2020). Up-regulation of miR-195 contributes to cardiac hypertrophy-induced arrhythmia by targeting calcium and potassium channels. J. Cell. Mol. Med. 24, 7991–8005. doi: 10.1111/jcmm.15431
Yazıcı, D., and Sezer, H. (2017). Insulin Resistance, Obesity and Lipotoxicity. Adv. Exp. Med. Biol. 960, 277–304. doi: 10.1007/978-3-319-48382-5_12
Ye, X., Zeng, H., Ning, G., Reiter, J. F., and Liu, A. (2014). C2cd3 is critical for centriolar distal appendage assembly and ciliary vesicle docking in mammals. Proc. Natl. Acad. Sci. U. S. A. 111, 2164–2169. doi: 10.1073/pnas.1318737111
Zhang, D., Xu, X., and Dong, Z. (2017). PRKCD/PKCδ contributes to nephrotoxicity during cisplatin chemotherapy by suppressing autophagy. Autophagy 13, 631–632. doi: 10.1080/15548627.2016.1269990
Zhao, W., Li, C., Zhang, H., Zhou, Q., Chen, X., Han, Y., et al. (2021). Dihydrotanshinone I Attenuates Plaque Vulnerability in Apolipoprotein E-Deficient Mice: role of Receptor-Interacting Protein 3. Antioxid. Redox Signal. 34, 351–363. doi: 10.1089/ars.2019.7796
Zhao, W., Yuan, Y., Zhao, H., Han, Y., and Chen, X. (2019). Aqueous extract of Salvia miltiorrhiza Bunge-Radix Puerariae herb pair ameliorates diabetic vascular injury by inhibiting oxidative stress in streptozotocin-induced diabetic rats. Food Chem. Toxicol. 129, 97–107. doi: 10.1016/j.fct.2019.04.018
Zheng, H., Feng, H., Zhang, W., Han, Y., and Zhao, W. (2020). Targeting autophagy by natural product Ursolic acid for prevention and treatment of osteoporosis. Toxicol. Appl. Pharmacol. 409:115271. doi: 10.1016/j.taap.2020.115271
Keywords: heart failure with preserved ejection fraction, differentially expressed genes, differentially correlated gene pairs, differential network, molecular docking
Citation: Zhou G, Sun S, Yuan Q, Zhang R, Jiang P, Li G, Wang Y and Li X (2021) Multiple-Tissue and Multilevel Analysis on Differentially Expressed Genes and Differentially Correlated Gene Pairs for HFpEF. Front. Genet. 12:668702. doi: 10.3389/fgene.2021.668702
Received: 17 February 2021; Accepted: 12 May 2021;
Published: 08 July 2021.
Edited by:
Xiaoping Liu, University of Chinese Academy of Sciences, ChinaReviewed by:
Jianxin Chen, Beijing University of Chinese Medicine, ChinaCopyright © 2021 Zhou, Sun, Yuan, Zhang, Jiang, Li, Wang and Li. This is an open-access article distributed under the terms of the Creative Commons Attribution License (CC BY). The use, distribution or reproduction in other forums is permitted, provided the original author(s) and the copyright owner(s) are credited and that the original publication in this journal is cited, in accordance with accepted academic practice. No use, distribution or reproduction is permitted which does not comply with these terms.
*Correspondence: Shaoyan Sun, c3Vuc3lfMjAxNEAxNjMuY29t; Xiao Li, bGl4aWFvNjE3QGhvdG1haWwuY29t
†These authors have contributed equally to this work
Disclaimer: All claims expressed in this article are solely those of the authors and do not necessarily represent those of their affiliated organizations, or those of the publisher, the editors and the reviewers. Any product that may be evaluated in this article or claim that may be made by its manufacturer is not guaranteed or endorsed by the publisher.
Research integrity at Frontiers
Learn more about the work of our research integrity team to safeguard the quality of each article we publish.