- 1Hainan Key Laboratory for Computational Science and Application, Hainan Normal University, Haikou, China
- 2Yangtze Delta Region Institute (Quzhou), University of Electronic Science and Technology of China, Quzhou, China
- 3Director of Preventive Treatment of Disease Centre, Qinhuangdao Hospital of Traditional Chinese Medicine, Qinhuangdao, China
- 4Department of Neurology, The Second Affiliated Hospital, Harbin Medical University, Harbin, China
- 5Department of Internal Medicine-Oncology, Heilongjiang Province Land Reclamation Headquarters General Hospital, Harbin, China
- 6Department of Radiology, The Second Affiliated Hospital, Harbin Medical University, Harbin, China
Circular RNAs (circRNAs) are a class of endogenous non-coding RNAs (ncRNAs) with a closed-loop structure that are mainly produced by variable processing of precursor mRNAs (pre-mRNAs). They are widely present in all eukaryotes and are very stable. Currently, circRNA studies have become a hotspot in RNA research. It has been reported that circRNAs constitute a significant proportion of transcript expression, and some are significantly more abundantly expressed than other transcripts. CircRNAs have regulatory roles in gene expression and critical biological functions in the development of organisms, such as acting as microRNA sponges or as endogenous RNAs and biomarkers. As such, they may have useful functions in the diagnosis and treatment of diseases. CircRNAs have been found to play an important role in the development of several diseases, including atherosclerosis, neurological disorders, diabetes, and cancer. In this paper, we review the status of circRNA research, describe circRNA-related databases and the identification of circRNAs, discuss the role of circRNAs in human diseases such as colon cancer, atherosclerosis, and gastric cancer, and identify remaining research questions related to circRNAs.
Introduction
Circular RNAs (circRNAs) are endogenous non-coding RNAs (ncRNAs) that have gained increasing attention in recent years. circRNAs are formed by exon or intron cyclization that ligates the 5′ terminal cap and 3′ terminal poly(A) tail to form a circular structure. They are mainly located in the cytoplasm or stored in exosomes, are unaffected by RNA exonucleases, are more stably expressed and less susceptible to degradation, and have been shown to exist in a wide variety of eukaryotic organisms (Li Y. et al., 2015; Pradeep et al., 2020). The widespread existence of circRNAs suggests that they have certain biological functions as lncRNAs and microRNAs (miRNAs) play (Jiang et al., 2009, 2014, 2015; Wang et al., 2014; Cheng L. et al., 2019; Liang et al., 2019; Wei and Liu, 2020; Yang et al., 2020). In recent years, studies have shown a diversity of formation mechanisms and biological functions of circRNAs. circRNAs are formed by various mechanisms; for example, spliceosomes (intracellular protein–RNA complexes) catalyze splicing as follows (Salgia et al., 2003): first, the spliceosome recognizes introns, which are flanked by the splice donor (or 5′ splice site) and the splice acceptor (or 3′ splice site) with specific sequences at the 5′ and 3′ ends; then, the 2′ hydroxyl group of the downstream sequence attacks the splice donor, resulting in a circular intron lariat structure; finally, the 3′ hydroxyl group of the upstream exon splice donor attacks the splice acceptor, the upstream and downstream exons are sequentially spliced to form a linear structure, and the intron lariat structure is usually degraded rapidly by debranching enzyme. Variable splicing is the process by which a precursor mRNA (pre-mRNA) can be transcribed from different RNA splicing methods; that is, different combinations of splice sites, to produce mutually exclusive mRNA splice isoforms, which in turn are translated to produce different protein products (Pan et al., 2008). This is the main function of RNA cyclization. Cyclization of circRNAs can be divided into intron and exon cyclization (Sanger et al., 1976), and the current mainstream cyclization mechanisms are categorized as follows: (1) exon skipping, (2) direct back-splicing of intron, (3) circRNA formation by RNA-binding proteins (RBPs; Chen, 2016; Zhang et al., 2018), and (4) circular intron RNA cyclization (Stoddard, 2014); the detailed mechanisms are shown in Figure 1. The diversity of circRNAs, and thus their diverse biological functions, is a direct result of these multiple formation mechanisms. For example, circRNAs can act as miRNA sponges (Hansen et al., 2013; Memczak et al., 2013; Zhao et al., 2020a), be translated into proteins (Yang et al., 2017), bind functional proteins (Li Z. et al., 2015), regulate RNA splicing (Conn et al., 2017), and regulate transcription (Chao et al., 1998; Memczak et al., 2013). Therefore, the identification of circRNAs contributes to our understanding of the formation and biological functions of circRNAs.
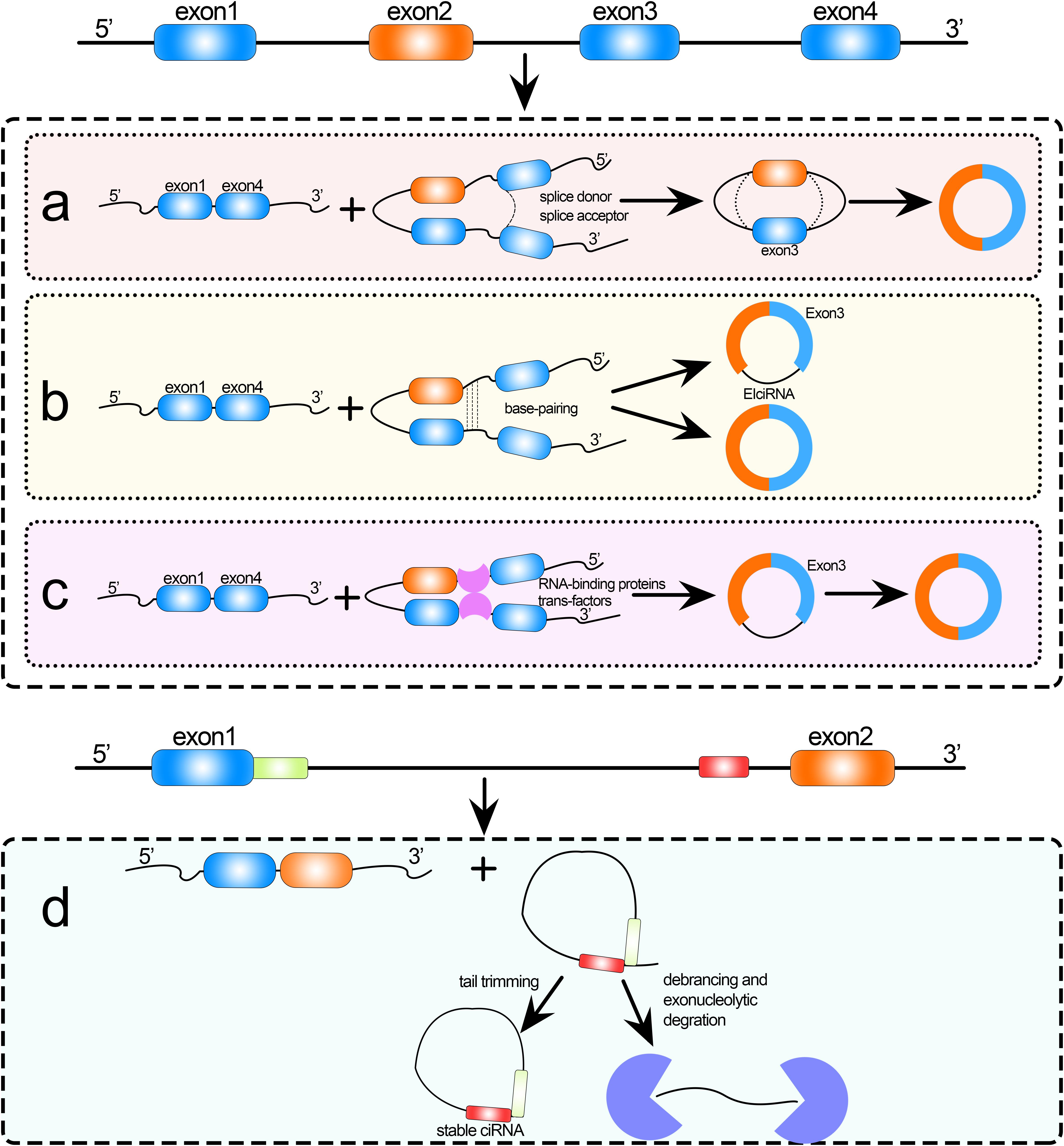
Figure 1. Formation of circRNAs by (a) exon skipping, (b) direct back-splicing, (c) formation by RNA-binding proteins (RBPs), and (d) circular intron RNA cyclization.
In 1976, Kolakofsky (1976) observed, for the first time, defective interfering RNAs in parainfluenza virus particles using electron microscopy. Sanger et al. (1976) discovered that plant-infecting viroids are a class of single-stranded, circular RNA molecules that have characteristics such as high thermal stability and a natural circular structure by self-complementary. In 1979, similar circular transcripts were found in HeLa cells and yeast mitochondria by electron microscopy (Hsu and Coca-Prados, 1979). In 1981, a ribosomal RNA (rRNA) gene was discovered in Tetrahymena that contained an intron sequence that formed a circular RNA after splicing. In 1988, the intron of 23S rRNA in archaea was found to be spliced at a specific site to form a stable circular RNA and to function as a transposon. In 1991, researchers identified several circular transcripts formed by different splicing patterns in the human oncogene DCC (Nigro et al., 1991), and these circular RNAs were then found in human ETS1 gene, mouse Sry (sex-determining region Y) gene, rat cytochrome P450 2C24 gene and human P450 2C18 gene.
Despite their early discovery, research on circRNAs has been slow in recent decades. Although circRNAs were discovered decades ago, they could not be detected by molecular techniques that relied on poly(A) enrichment because they did not have free 3′ and 5′ ends. Instead, cyclizable exons were spliced by reverse splicing, which was different from regular linear splicing. Moreover, the mapping algorithm of early transcriptome analysis could not directly map the sequenced fragments to the genome, leading to the idea that circRNAs were byproducts of missplicing. With the development of high-throughput sequencing and bioinformatics technologies, it was first proposed in 2012 that circRNAs are circular transcripts generated by reverse splicing of mRNA precursors, which are found to exist in large quantities in different types of human cells. In 2013, it was found that circRNAs can act as a sponge for miRNAs (Hansen et al., 2013; Memczak et al., 2013), which regulate the growth and development of organisms. Since then, circRNAs have rapidly become a research hotspot. To identify circRNAs, in addition to high-throughput techniques (RNA-seq), common analytical and computational methods are used, such as CIRI (Gao et al., 2015), segemehl (Hoffmann et al., 2014), Mapsplice (Wang et al., 2010), and CircSeq (Guo et al., 2014). In recent years, researchers have developed machine learning methods to identify circRNAs based on the above methods (Yin et al., 2021). Feature selection is an important part of these machine learning models. Feature selection, aiming to select a subset of features by eliminating redundant and noise features, is an important preprocessing step in bioinformatics. Recently, Su et al. (2018) proposed a binomial distribution based method to perform feature selection in computational genomics. The effectiveness of their method has been proved by predicting lncRNA subcellular localizations (Su et al., 2018). Since both nucleotide and amino acid composition obey binomial distribution, this method is suggested to be used for genomic and proteomic analysis. We provide here an overview of the research progress of circRNAs, including the development of circRNA databases, identification of circRNAs, and the role of circRNAs in human diseases such as colon cancer, atherosclerosis, and gastric cancer.
circRNA-Related Databases
In recent years, as circRNA research has progressed, an increasing number of circRNAs have been discovered in different species, and circRNA-related databases have been created. Some of the main circRNA databases published so far are listed below.
(1) circBase collects and merges public circRNA datasets and provides evidence of the genomic catalog of their expression, as well as scripts to identify circRNAs in sequencing data1 (Glazar et al., 2014).
(2) Circ2Trait is a comprehensive database that includes potential associations of circRNAs with diseases and traits by studying the interaction network of circRNAs with miRNAs and calculating their internal SNPs and Argonaute (Ago) interaction sites2 (Ghosal et al., 2013).
(3) deepBase contains about 150,000 circRNA genes from organisms, including human, mouse, Drosophila, and nematode. This database also constructs the most comprehensive expression map of circRNAs3 (Yang et al., 2010).
(4) CirNet mainly includes RNA-seq data of more than 400 samples from 26 tissues collected from the sequence read archive database. This database not only includes basic information on circRNAs but also provides expression profile data of circRNAs in different tissues and the competing endogenous (ce)RNA regulatory network of circRNAs–miRNA–gene4 (Liu et al., 2016).
(5) starBase v2.0 integrates published circRNA data and constructs interaction networks of miRNAs with circRNAs and circRNAs with RBPs. In addition, the database looks for potential miRNA–ncRNA, miRNA–mRNA, ncRNA–RNA, RBP–ncRNA, and RBP–mRNA interactions through high-throughput data. starBase also predicts the function of ncRNAs from miRNA-mediated (ceRNA) regulatory networks (miRNAs, lncRNAs, and pseudogenes) and protein-coding genes using the online tools miRFunction and ceRNAFunction5 (Li et al., 2014).
Tools for Recognition of circRNAs
Because of the low expression level of circRNAs and limitations of previous computational methods, these RNA molecules were only found in small numbers in individual genes and therefore initially thought to be products of missplicing, byproducts of RNA splicing, incidental in animals, or precursors of linear RNAs. In recent years, with improved experimental and computational methods for circRNAs and the use of next-generation high-throughput sequencing technologies (Wang et al., 2009; Zeng et al., 2017, 2019), a large number of stable circRNAs have now been found in a variety of cells, and 85% of circRNAs can be mapped to known genes, of which 84% overlap with coding exons (Memczak et al., 2013). Because of the special structure of circRNAs—they lack a 5′ terminal cap and a 3′ terminal poly(A) tail and have a closed-loop structure with covalent bonds—and their maturation mechanism, early sequencing methods could not easily detect such molecules. Improvements in sequencing analysis techniques and computational methods have made detection more efficient (Malysiak-Mrozek et al., 2019; Mrozek, 2020). Therefore, studies on the identification of circRNAs are reviewed from two aspects: (1) identification based on sequencing data and (2) identification based on sequence features and machine learning methods.
Identification of circRNAs Based on Sequencing
Many algorithms exist for circRNA identification, including CIRI (Gao et al., 2015), segemehl (Hoffmann et al., 2014), Mapsplice (Wang et al., 2010), CircSeq (Guo et al., 2014), and find_circ (Memczak et al., 2013). Using these algorithms, researchers have identified a large number of circRNAs in human, mouse, nematode, archaea, and other organisms (Yang et al., 2011; Jeck and Sharpless, 2014). We describe here several of these commonly used sequencing-based tools for identification of circRNAs.
CIRI (Stoddard, 2014) was developed by Gao et al. (2015) to comprehensively identify circRNAs, and it is based on the novel chiastic clipping signal algorithm. CIRI can accurately detect circRNAs from transcriptomic data without bias through multiple filtering strategies. This tool is mainly used to identify and annotate circRNAs from RNA-seq data. Unlike other methods for annotating circRNAs, CIRI eliminates false positives by using a new algorithm based on paired cross-clip signal detection in the BWA-MEM sequence alignment/map and combining it with systematic filtering.
CIRCexplorer, a tool for identifying circRNAs developed by Zhang et al. (2014), was the first to elucidate the regulatory mechanism of complementary sequences on production of exon-derived circRNAs. This tool revealed that regulation of variable cyclization was mediated by competitive pairing of complementary sequences, providing a new theoretical perspective on the complexity and diversity of gene expression at the transcriptional and posttranscriptional levels. Nearly 10,000 circRNAs were identified in human embryonic stem cell line H9 using a special nuclease to enrich circRNAs in combination with computational analysis software, demonstrating exon cyclization mediated by the complementary sequence of intron RNA. Competitive pairing of complementary sequences between different regions can selectively generate either linear RNAs or circRNAs.
CircSeq, a tool developed by Guo et al. (2014) to identify and characterize mammalian circRNAs, is a computational pipeline to identify and quantify the relative abundance of circRNAs from RNA-seq databases. Compared with other identification tools, CircSeq does not require available gene annotation to identify circRNAs. The application of the identification tool to non-polyA-selected RNA sequencing data in the ENCODE project proved its ability to classify and globally characterize more than 7000 human circRNAs.
The above sequencing methods all identify back-splicing sites from high-throughput sequencing data to detect circRNAs. In comparing some of the above identification tools, Hansen et al. (2016) and Sekar et al. (2019) found that only a small percentage of circRNAs could be predicted simultaneously by these tools, indicating significant differences and species variability. Therefore, the above tools developed around high-throughput sequencing technology have poor identification performance and low consistency. Moreover, these tools generally have high false-positive rates and low sensitivity (Hansen et al., 2016). To address these shortcomings, researchers have developed tools to identify circRNAs on the basis of sequence features and machine learning.
Identification of circRNAs Based on Sequence Features and Machine Learning
Identifying circRNAs using sequence features that distinguish circRNAs from linear RNAs (especially mRNAs that encode proteins) is an urgent problem to be solved in bioinformatics. In recent years, the combination of sequence features and machine learning has been successfully used to solve biological problems such as the prediction of gene regulatory sites and splice sites (Wang et al., 2008; Xiong et al., 2015), and protein function (Cao et al., 2017; Gbenro et al., 2020; Hippe, 2020; Zhai et al., 2020), etc (Mrozek et al., 2007, 2009; Wei et al., 2017b,c, 2018; Jin et al., 2019; Stephenson et al., 2019; Su et al., 2019a,b; Liu B. et al., 2020; Liu Y. et al., 2020; Smith et al., 2020; Zhao et al., 2020b,c). Some tools have been developed to identify circRNAs using sequence features and machine learning methods. The basic framework of using machine learning methods to predict circRNAs is shown in Figure 2.
One study selected 100 RNA circularization-related sequence features, including length, adenosine-to-inosine (A-to-I) density, and Alu sequences of introns upstream and downstream of the splice site, and established a machine learning model to identify circRNAs in the human genome. The classification abilities of two machine learning methods, random forest (RF; Cheng et al., 2019b; Liu et al., 2019) and support vector machine (SVM; Jiang et al., 2013; Wei et al., 2014, 2017a, 2019; Zhao et al., 2015; Cheng, 2019; Hong et al., 2020; Li and Liu, 2020; Shao and Liu, 2020), were also compared. The results showed that the selected sequence features could effectively identify RNA circularization and that different sequence features contribute differently to the classification and prediction ability of the model. The RF method showed better classification than the SVM method.
In 2021, Yin et al. (2021) constructed a tool, named PCirc, to identify circRNAs using multiple sequence features and RF classification. This tool specifically targets the identification of circRNAs in plants, mainly from RNA sequence data. The tool encodes the sequence information of rice circRNAs by using three feature-encoding methods: k-mers, open reading frames, and splicing junction sequence coding (SJSC). The accuracy of the encoded information is greater than 80% when using the RF method for identification. The identification model can be used not only for the identification of rice circRNAs, but also for the recognition of circRNAs in plants such as Arabidopsis thaliana.
circRNAs and Human Diseases
In terms of disease diagnosis, studies have found that the exosomes released by cancer cells contain abundant circRNAs, suggesting that circRNAs might be used as biological markers for clinical diagnosis. The key when using circRNAs for disease prediction is to identify the interaction site between the circRNA and miRNA or RBP, and then indirectly determine the association between the circRNA and disease by analyzing the relationship between the miRNA or RBP and disease (Jiang et al., 2010; Cheng et al., 2018; Liu, 2020; Zeng et al., 2020; Zuo et al., 2020).
In 2015, Li Y. et al. (2015) reported that exosomes are enriched with circRNAs, so it is possible that diseases such as colon cancer could be diagnosed by detecting circRNAs in serum. Aberrant expression of circRNAs in colorectal cancer and pancreatic ductal adenocarcinoma has been used as a diagnostic or predictive biomarker. By studying their expression profile, it was found that circRNAs may be associated with the molecular pathogenesis of cutaneous basal cell carcinoma (Sand et al., 2016).
The first validated circRNA, cANRIL, is closely related to a single nucleotide polymorphism (SNP) that is thought to alter the splicing of cANRIL, leading to expression of the INK4A/ARF loci, resulting in an increased incidence of atherosclerosis (Burd et al., 2010). Hypoxia is one of the key factors contributing to the development of atherosclerosis, and is therefore also regulated by circRNA (Boeckel et al., 2015).
Xu et al. (2015) showed that mice of a transgenic line overexpressing the miR-7 gene in β-cells developed diabetes mellitus. The same study showed that overexpression of the circRNA ciRS-7 inhibited miR-7 function and thus improved insulin secretion. Potential target genes of miR-7 have been identified by bioinformatics analysis and include Myrip (a gene regulating insulin secretory granules) and Pax6 (a gene enhancing insulin transcription).
A study by Li P. et al. (2015) identified the circRNA hsa-circ002059 as being associated with gastric cancer. In that study, expression of this circRNA was downregulated in gastric tissues of patients compared with healthy controls. In addition, hsa-circ002059 was found at significantly lower levels in plasma of patients with gastric cancer than in healthy controls.
In bladder cancer, circRNAs have been identified using high-throughput microarray technology. Using this approach, Zhong et al. (2016) found two downregulated circRNAs (circFAM169A and circTRIM24) and 4 upregulated circRNAs (circTCF25, circZFR, circPTK2, and circBC048201) in bladder cancer tissue compared with adjacent non-tumor tissues. In addition, in the cancer tissues, circTCF25 could increase expression of the CDK6 gene by modulating miR-103a-3p and miR-107. This is closely related to the development of cancer.
Qin et al. (2016) identified hsa-cir0001649 in hepatocellular carcinoma (HCC) and found that its expression was significantly decreased compared with that in adjacent normal liver tissue. In contrast, Shang et al. (2016) found that another circRNA, hsa-cir0005075, was significantly downregulated in HCC compared with adjacent normal tissue.
Exosomes are highly enriched with circRNAs. Exosomes are extracellular vesicles, 40 to 160 nm in diameter, that function as important intercellular signaling pathways (Li Y. et al., 2015; Kalluri and LeBleu, 2020). The exosome database exoRBase included 92 sequenced samples of serum exosomes, including samples from healthy volunteers and patients with coronary heart disease and colon cancer. The exosome samples contained 58,330 circRNAs and 18,333 mRNAs (Li et al., 2018). Zhang et al. (2019) demonstrated that circNRIP1, when secreted via exosome, can be taken up by gastric cancer cells and promote their proliferation, migration, and invasion. Therefore, exosomes can be regarded as in vivo carriers of circRNAs that can amplify their biological functions.
Challenges and Prospects
Compared with long non-coding RNAs and miRNAs, research on circRNAs is still in its infancy and many questions remain to be answered, primarily in four areas:
(1) Transport and degradation: because circRNAs can resist RNase digestion and are stable in cells, the process of their degradation is unclear.
(2) Formation: it is unknown whether circRNAs are produced during or after transcription.
(3) Expression, translation, and function of circRNAs: circRNAs have stable structures and are highly conserved, underpinning their ability to play important roles in different organisms. Their unconfirmed roles, including acting as miRNA sponges, regulating gene expression, and targeting RBPs, require comprehensive and extensive elucidation.
(4) Research methodology: the experimental methodologies and bioinformatics used to identify circRNAs are challenging. For example, in experimental methods, general RNA-seq procedures such as reverse transcription may cause technical mis-ligation and generate a large number of artificial circRNAs. These pseudo circRNAs can account for 34–55% of the sequencing quantity, seriously affecting the accuracy of the data. As for methods that use machine learning and sequence features, only a few identification tools exist and their accuracy needs to be improved. These tools are not stable across different species. Therefore, in the future, stable identification models and deep learning methods are needed to establish identification tools for circRNAs and improve the robustness of the models.
Accurate identification will help determine additional biological functions of circRNAs. The unique features of circRNAs such as ceRNA may provide new ideas for drug discovery and development. The tissue specificity and stability of circRNAs make them potentially useful biomarkers. In the near future, it is likely that circRNAs will play important roles in the prevention, diagnosis, and treatment of various diseases.
Author Contributions
ML and BG: conceptualization, writing—review and editing, and supervision. SJ, SH, and SW: investigation and writing—original draft preparation. All authors have read and agreed to the published version of the manuscript.
Funding
The work was supported by National Natural Science Foundation of China (No. 62002087).
Conflict of Interest
The authors declare that the research was conducted in the absence of any commercial or financial relationships that could be construed as a potential conflict of interest.
Acknowledgments
We thank Louise Adam, ELS(D), from Liwen Bianji, Edanz Editing China (www.liwenbianji.cn/ac), for editing the English text of a draft of this manuscript.
Footnotes
- ^ http://www.circbase.org/
- ^ http://gyanxet-beta.com/circdb/
- ^ http://deepbase.sysu.edu
- ^ http://syslab5.nchu.edu.tw/CircNet
- ^ http://starbase.sysu.edu.cn/
References
Boeckel, J. N., Jae, N., Heumueller, A. W., Chen, W., Boon, R. A., Stellos, K., et al. (2015). Identification and. characterization of hypoxia-regulated endothelial circular RNA. Circ. Res. 117, 884–890.
Burd, C. E., Jeck, W. R., Liu, Y., Sanoff, H. K., Wang, Z., and Sharpless, N. E. (2010). Expression of linear and novel circular forms of an INK4/ARF-associated non-coding RNA correlates with atherosclerosis risk. PLoS Genet. 6:e1001233. doi: 10.1371/journal.pgen.1001233
Cao, R., Freitas, C., Chan, L., Sun, M., Jiang, H., and Chen, Z. (2017). ProLanGO: protein function prediction using neural machine translation based on a recurrent neural network. Molecules 22:1732. doi: 10.3390/molecules22101732
Chao, C. W., Chan, D. C., Kuo, A., and Leder, P. (1998). The mouse formin (Fmn) gene: abundant circular RNA transcripts and gene-targeted deletion analysis. Mol. Med. 4, 614–628. doi: 10.1007/bf03401761
Chen, L. L. (2016). The biogenesis and emerging roles of circular RNAs. Nat. Rev. Mol. Cell Biol. 17, 205–211. doi: 10.1038/nrm.2015.32
Cheng, L. (2019). Computational and biological methods for gene therapy. Curr. Gene Ther. 19, 210–210. doi: 10.2174/156652321904191022113307
Cheng, L., Hu, Y., Sun, J., Zhou, M., and Jiang, Q. (2018). DincRNA: a comprehensive web-based bioinformatics toolkit for exploring disease associations and ncRNA function. Bioinformatics 34, 1953–1956. doi: 10.1093/bioinformatics/bty002
Cheng, L., Wang, P., Tian, R., Wang, S., Guo, Q., Luo, M., et al. (2019). LncRNA2Target v2.0: a comprehensive database for target genes of lncRNAs in human and mouse. Nucl. Acids Res. 47, D140–D144.
Cheng, L., Zhao, H., Wang, P., Zhou, W., Luo, M., Li, T., et al. (2019b). Computational methods for identifying similar diseases. Mol. Ther. Nucl. Acids. 18, 590–604. doi: 10.1016/j.omtn.2019.09.019
Conn, V. M., Hugouvieux, V., Nayak, A., Conos, S. A., Capovilla, G., Cildir, G., et al. (2017). A circRNA from SEPALLATA3 regulates splicing of its cognate mRNA through R-loop formation. Nat. Plants 3:17053.
Gao, Y., Wang, J., and Zhao, F. (2015). CIRI: an efficient and unbiased algorithm for de novo circular RNA identification. Genome Biol. 16:4.
Gbenro, S., Hippe, K., and Cao, R. (2020). “HMMeta: Protein function prediction using hidden markov models,” in Proceedings of the BCB ’20: 11th ACM International Conference on Bioinformatics, Computational Biology and Health Informatics (New York, NY: Association for Computing Machinery).
Ghosal, S., Das, S., Sen, R., Basak, P., and Chakrabarti, J. (2013). Circ2Traits: a comprehensive database for circular RNA potentially associated with disease and traits. Front. Genet. 4:283. doi: 10.3389/fgene.2013.00283
Glazar, P., Papavasileiou, P., and Rajewsky, N. (2014). circBase: a database for circular RNAs. RNA 20, 1666–1670. doi: 10.1261/rna.043687.113
Guo, J. U., Agarwal, V., Guo, H., and Bartel, D. P. (2014). Expanded identification and characterization of mammalian circular RNAs. Genome Biol. 15:409.
Hansen, T. B., Jensen, T. I., Clausen, B. H., Bramsen, J. B., Finsen, B., Damgaard, C. K., et al. (2013). Natural RNA circles function as efficient microRNA sponges. Nature 495, 384–388. doi: 10.1038/nature11993
Hansen, T. B., Veno, M. T., Damgaard, C. K., and Kjems, J. (2016). Comparison of circular RNA prediction tools. Nucl. Acids Res. 44:e58. doi: 10.1093/nar/gkv1458
Hippe, K. (2020). “Sola gbenro; renzhi cao in prolango2: protein function prediction with ensemble of encoder-decoder networks,” in Proceedings of the BCB ’20: 11th ACM International Conference on Bioinformatics, Computational Biology and Health Informatics (New York, NY: Association for Computing Machinery).
Hoffmann, S., Otto, C., Doose, G., Tanzer, A., Langenberger, D., Christ, S., et al. (2014). A multi-split mapping algorithm for circular RNA, splicing, trans-splicing and fusion detection. Genome Biol. 15:R34.
Hong, Z., Zeng, X., Wei, L., and Liu, X. (2020). Identifying enhancer-promoter interactions with neural network based on pre-trained DNA vectors and attention mechanism. Bioinformatics 36, 1037–1043.
Hsu, M. T., and Coca-Prados, M. (1979). Electron microscopic evidence for the circular form of RNA in the cytoplasm of eukaryotic cells. Nature 280, 339–340. doi: 10.1038/280339a0
Jeck, W. R., and Sharpless, N. E. (2014). Detecting and characterizing circular RNAs. Nat. Biotechnol. 32, 453–461. doi: 10.1038/nbt.2890
Jiang, Q., Hao, Y., Wang, G., Juan, L., Zhang, T., Teng, M., et al. (2010). Prioritization of disease microRNAs through a human phenome-microRNAome network. BMC Syst. Biol. 4(Suppl. 1):S2. doi: 10.1186/1752-0509-4-S1-S2
Jiang, Q., Ma, R., Wang, J., Wu, X., Jin, S., Peng, J., et al. (2015). LncRNA2Function: a comprehensive resource for functional investigation of human lncRNAs based on RNA-seq data. BMC Genomics. 16(Suppl. 3):S2. doi: 10.1186/1471-2164-16-S3-S2
Jiang, Q., Wang, G., Jin, S., Li, Y., and Wang, Y. (2013). Predicting human microRNA-disease associations based on support vector machine. Int. J. Data Min. Bioinform. 8, 282–293. doi: 10.1504/ijdmb.2013.056078
Jiang, Q., Wang, J., Wang, Y., Ma, R., Wu, X., and Li, Y. (2014). TF2LncRNA: identifying common transcription factors for a list of lncRNA genes from ChIP-Seq data. Biomed Res. Int. 2014:317642.
Jiang, Q., Wang, Y., Hao, Y., Juan, L., Teng, M., Zhang, X., et al. (2009). miR2Disease: a manually curated database for microRNA deregulation in human disease. Nucl. Acids Res. 37, D98–D104.
Jin, Q., Meng, Z., Tuan, D. P., Chen, Q., Wei, L., and Su, R. (2019). DUNet: a deformable. Knowl. Based Syst. 178, 149–162. doi: 10.1016/j.knosys.2019.04.025
Kalluri, R., and LeBleu, V. S. (2020). The biology, function, and biomedical applications of exosomes. Science 367:eaau6977. doi: 10.1126/science.aau6977
Kolakofsky, D. (1976). Isolation and characterization of Sendai virus DI-RNAs. Cell 8, 547–555. doi: 10.1016/0092-8674(76)90223-3
Li, C. C., and Liu, B. (2020). MotifCNN-fold: protein fold recognition based on fold-specific features extracted by motif-based convolutional neural networks. Brief. Bioinform. 21, 2133–2141. doi: 10.1093/bib/bbz133
Li, J. H., Liu, S., Zhou, H., Qu, L. H., and Yang, J. H. (2014). starBase v2.0: decoding miRNA-ceRNA, miRNA-ncRNA and protein-RNA interaction networks from large-scale CLIP-Seq data. Nucl. Acids Res. 42, D92–D97.
Li, P., Chen, S., Chen, H., Mo, X., Li, T., Shao, Y., et al. (2015). Using circular RNA as a novel type of biomarker in the screening of gastric cancer. Clin. Chim. Acta 444, 132–136. doi: 10.1016/j.cca.2015.02.018
Li, S., Li, Y., Chen, B., Zhao, J., Yu, S., Tang, Y., et al. (2018). exoRBase: a database of circRNA, lncRNA and mRNA in human blood exosomes. Nucl. Acids Res. 46, D106–D112.
Li, Y., Zheng, Q., Bao, C., Li, S., Guo, W., Zhao, J., et al. (2015). Circular RNA is enriched and stable in exosomes: a promising biomarker for cancer diagnosis. Cell Res. 25, 981–984. doi: 10.1038/cr.2015.82
Li, Z., Huang, C., Bao, C., Chen, L., Lin, M., Wang, X., et al. (2015). Exon-intron circular RNAs regulate transcription in the nucleus. Nat. Struct. Mol. Biol. 22, 256–264. doi: 10.1038/nsmb.2959
Liang, C., Changlu, Q., He, Z., Tongze, F., and Xue, Z. (2019). gutMDisorder: a comprehensive database for dysbiosis of the gut microbiota in disorders and interventions. Nucl. Acids Res. 48:7603.
Liu, B., Gao, X., and Zhang, H. (2019). BioSeq-analysis2.0: an updated platform for analyzing DNA, RNA, and protein sequences at sequence level and residue level based on machine learning approaches. Nucl. Acids Res. 47:e127. doi: 10.1093/nar/gkz740
Liu, B., Zhu, Y., and Yan, K. (2020). Fold-LTR-TCP: protein fold recognition based on triadic closure principle. Brief. Bioinform. 21, 2185–2193. doi: 10.1093/bib/bbz139
Liu, Y. C., Li, J. R., Sun, C. H., Andrews, E., Chao, R. F., Lin, F. M., et al. (2016). CircNet: a database of circular RNAs derived from transcriptome sequencing data. Nucl. Acids Res. 44, D209–D215.
Liu, Y., Huang, Y., Wang, G., and Wang, Y. (2020). A deep learning approach for filtering structural variants in short read sequencing data. Brief Bioinform. doi: 10.1093/bib/bbaa370
Liu, Z. P. (2020). Predicting lncRNA-protein interactions by machine learning methods: a review. Curr. Bioinform. 15, 831–840. doi: 10.2174/1574893615666200224095925
Malysiak-Mrozek, B., Baron, T., and Mrozek, D. (2019). Spark-IDPP: high-throughput and scalable prediction of intrinsically disordered protein regions with Spark clusters on the cloud. Cluster Comput. J. Net. Softw. Tools Appl. 22, 487–508. doi: 10.1007/s10586-018-2857-9
Memczak, S., Jens, M., Elefsinioti, A., Torti, F., Krueger, J., Rybak, A., et al. (2013). F le noble., N rajewsky, circular RNAs are a large class of animal RNAs with regulatory potency. Nature 495, 333–338. doi: 10.1038/nature11928
Mrozek, D. (2020). A review of cloud computing technologies for comprehensive microRNA analyses. Comput. Biol. Chem. 88:107365. doi: 10.1016/j.compbiolchem.2020.107365
Mrozek, D., Malysiak, B., and Kozielski, S. (2007). “An optimal alignment of proteins energy characteristics with crisp and fuzzy similarity awards,” in Proceedings of the 2007 Ieee International Conference on Fuzzy Systems, Vol. 1-4 (London: IEEE), 1513–1518.
Mrozek, D., Malysiak-Mrozek, B., and Kozielski, S. (2009). Alignment of Protein Structure Energy Patterns Represented as Sequences of Fuzzy Numbers. Cincinnati, OH: IEEE, 35–40.
Nigro, J. M., Cho, K. R., Fearon, E. R., Kern, S. E., Ruppert, J. M., Oliner, J. D., et al. (1991). Scrambled exons. Cell. 64, 607–613. doi: 10.1016/0092-8674(91)90244-s
Pan, Q., Shai, O., Lee, L. J., Frey, J., and Blencowe, B. J. (2008). Deep surveying of alternative splicing complexity in the human transcriptome by high-throughput sequencing. Nat. Genet. 40, 1413–1415. doi: 10.1038/ng.259
Pradeep, C., Nandan, D., Das, A. A., and Velayutham, D. (2020). Comparative transcriptome profiling of disruptive technology, single-molecule direct RNA sequencing. Curr. Bioinf. 15, 165–172. doi: 10.2174/1574893614666191017154427
Qin, M., Liu, G., Huo, X., Tao, X., Sun, X., Ge, Z., et al. (2016). Hsa_circ_0001649: a circular RNA and potential novel biomarker for hepatocellular carcinoma. Cancer Biomark. 16, 161–169.
Salgia, S. R., Singh, S. K., Gurha, P., and Gupta, R. (2003). Two reactions of Haloferax voicanii RNA splicing enzymes: joining of exons and circularization of introns. RNA 9, 319–330. doi: 10.1261/rna.2118203
Sand, M., Bechara, F. G., Sand, D., Gambichler, T., Hahn, S. A., Bromba, M., et al. (2016). Circular RNA expression in basal cell carcinoma. Epigenomics 8, 619–632. doi: 10.2217/epi-2015-0019
Sanger, H. L., Klotz, G., Riesner, D., Gross, H. J., and Kleinschmidt, A. K. (1976). Viroids are single-stranded covalently closed circular RNA molecules existing as highly base-paired rod-like structures. PNAS 73, 3852–3856. doi: 10.1073/pnas.73.11.3852
Sekar, S., Geiger, P., Cuyugan, L., Boyle, A., Serrano, G., Beach, T. G., et al. (2019). Identification of circular RNAs using RNA sequencing. J. Vis. Exp. 14:e59981. doi: 10.3791/59981
Shang, X., Li, G., Liu, H., Li, T., Liu, J., and Zhao, Q., et al. (2016). Comprehensive circular RNA profiling reveals that hsa_circ_0005075, a new circular RNA biomarker, is involved in hepatocellular crcinoma development. Medicine 95:e3811.
Shao, J., and Liu, B. (2020). ProtFold-DFG: protein fold recognition by combining directed fusion graph and pagerank algorithm. Brief. Bioinform. doi: 10.1093/bib/bbaa192
Smith, J., Conover, M., Stephenson, N., Eickholt, J., Si, D., Sun, M., et al. (2020). TopQA: a topological representation for single-model protein quality assessment with machine learning. J. Int. J. Comput. Biol. Drug Des. 13:144. doi: 10.1504/ijcbdd.2020.10026784
Stephenson, N., Shane, E., Chase, J., Rowland, J., Ries, D., Justice, N., et al. (2019). Survey of machine learning techniques in drug discovery. Curr. Drug Metab. 20, 185–193. doi: 10.2174/1389200219666180820112457
Stoddard, B. L. (2014). Homing endonucleases from mobile group I introns: discovery to genome engineering. Mobile DNA 5:7. doi: 10.1186/1759-8753-5-7
Su, R., Liu, X., Wei, L., and Zou, Q. (2019a). Deep-Resp-Forest: a deep forest model to predict anti-cancer drug response. Methods (San Diego, Calif.) 166, 91–102. doi: 10.1016/j.ymeth.2019.02.009
Su, R., Wu, H., Xu, B., Liu, X., and Wei, L. (2019b). Developing a multi-dose computational model for drug-induced hepatotoxicity prediction based on toxicogenomics data. IEEE-ACM Trans. Comput. Biol. Bioinform. 16, 1231–1239. doi: 10.1109/tcbb.2018.2858756
Su, Z. D., Huang, Y., Zhang, Z. Y., Zhao, Y. W., Wang, D., Chen, W., et al. (2018). iLoc-lncRNA: predict the subcellular location of lncRNAs by incorporating octamer composition into general PseKNC. Bioinformatics 34, 4196–4204.
Wang, G., Wang, Y., Feng, W., Wang, X., Yang, J. Y., Zhao, Y., et al. (2008). Transcription factor and microRNA regulation in androgen-dependent and -independent prostate cancer cells. BMC Genom. 9 (Suppl. 2):S22. doi: 10.1186/1471-2164-9-S2-S22
Wang, K., Singh, D., Zeng, Z., Coleman, S. J., Huang, Y., Savich, G. L., et al. (2010). MapSplice: accurate mapping of RNA-seq reads for splice junction discovery. Nucl. Acids Res. 38:e178. doi: 10.1093/nar/gkq622
Wang, P. L., Bao, Y., Yee, M. C., Barrett, S. P., Hogan, G. J., Olsen, M. N., et al. (2014). Circular RNA is expressed across the eukaryotic tree of life. PLoS One 9:e90859. doi: 10.1371/journal.pone.0090859
Wang, Z., Gerstein, M., and Snyder, M. (2009). RNA-Seq: a revolutionary. Nat. Rev. Genet. 10, 57–63.
Wei, H., and Liu, B. (2020). iCircDA-MF: identification of circRNA-disease associations based on matrix factorization. Brief. Bioinform. 21, 1356–1367. doi: 10.1093/bib/bbz057
Wei, L., Ding, Y., Su, R., Tang, J., and Zou, Q. (2018). Prediction of human protein subcellular localization using deep learning. J. Parallel Distrib. Comput. 117, 212–217.
Wei, L., Liao, M., Gao, Y., Ji, R., He, Z., and Zou, Q. (2014). Improved and promising identification of human MicroRNAs by incorporating a high-quality negative set. IEEE/ACM Trans. Comput. Biol. Bioinform. 11, 192–201. doi: 10.1109/tcbb.2013.146
Wei, L., Tang, J., and Zou, Q. (2017a). Local-DPP: an improved DNA-binding protein prediction method by exploring local evolutionary information. Inf. Sci. 384, 135–144. doi: 10.1016/j.ins.2016.06.026
Wei, L., Wan, S., Guo, J., and Wong, K. K. L. (2017c). A novel hierarchical selective ensemble classifier with bioinformatics application. Artif. Intell. Med. 83, 82–90. doi: 10.1016/j.artmed.2017.02.005
Wei, L., Xing, P., Shi, G., Ji, Z., and Zou, Q. (2019). Fast prediction of protein methylation sites using a sequence-based feature selection technique. IEEE-ACM Trans. Comput. Biol. Bioinform. 16, 1264–1273. doi: 10.1109/tcbb.2017.2670558
Wei, L., Xing, P., Zeng, J., Chen, J. X., Su, R., and Guo, F. (2017b). Improved prediction of protein-protein interactions using novel negative samples, features, and an ensemble classifier. Artif. Intell. Med. 83, 67–74. doi: 10.1016/j.artmed.2017.03.001
Xiong, H. Y., Alipanahi, B., Lee, L. J., Bretschneider, H., Merico, D., Yuen, R. K. C., et al. (2015). RNA splicing. the human splicing code reveals new insights into the genetic determinants of disease. Science 347, 1254806.
Xu, H., Guo, S., Li, W., and Yu, P. (2015). The circular RNA Cdr1as, via miR-7 and its targets, regulates insulin transcription and secretion in islet cells. Sci. Rep. 5:12453.
Yang, J. H., Shao, P., Zhou, H., Chen, Y. Q., and Qu, L. H. (2010). deepBase: a database for deeply annotating and mining deep sequencing data. Nucl. Acids Res. 38, D123–D130.
Yang, L., Duff, M. O., Graveley, B. R., Carmichael, G. G., and Chen, L. L. (2011). Genomewide characterization of non-polyadenylated RNAs. Genome Biol. 12:R16.
Yang, Q., Wu, J., Zhao, J., Xu, T., Han, P., and Song, X. (2020). The expression profiles of lncrnas and their regulatory network during smek1/2 knockout mouse neural stem cells differentiation. Curr. Bioinform. 15, 77–88. doi: 10.2174/1574893614666190308160507
Yang, Y., Fan, X., Mao, M., Song, X., Wu, P., Zhang, Y., et al. (2017). Extensive translation of circular RNAs driven by N-6-methyladenosine. Cell Res. 27, 626–641. doi: 10.1038/cr.2017.31
Yin, S., Tian, X., Zhang, J., Sun, P., and Li, G. (2021). PCirc: random forest-based plant circRNA identification software. BMC Bioinf. 22:10. doi: 10.1186/s12859-020-03944-1
Zeng, X. X., Lin, W., Guo, M. Z., and Zou, Q. (2019). Details in the evaluation of circular RNA detection tools: reply to Chen and Chuang. PLoS Comput. Biol. 15:5. doi: 10.1371/journal.pcbi.1006916
Zeng, X., Lin, W., Guo, M., and Zou, Q. (2017). A comprehensive overview and evaluation of circular RNA detection tools. PLoS Comput. Biol. 13:e1005420. doi: 10.1371/journal.pcbi.1005420
Zeng, X., Zhong, Y., Lin, W., and Zou, Q. (2020). Predicting disease-associated circular rnas using deep forests combined with positive-unlabeled learning methods. Brief. Bioinform. 21, 1425–1436. doi: 10.1093/bib/bbz080
Zhai, Y., Chen, Y., Teng, Z., and Zhao, Y. (2020). Identifying antioxidant proteins by using amino acid composition and protein-protein interactions. Front. Cell Dev. Biol. 8:591487. doi: 10.3389/fcell.2020.591487
Zhang, X., Wang, S., Wang, H., Cao, J., Huang, X., Chen, Z., et al. (2019). Circular RNA circNRIP1 acts as a microRNA-149-5p sponge to promote gastric cancer progression via the AKT1/mTOR pathway. Mol. Cancer 18:20.
Zhang, X.-Q., Wang, H.-B., Zhang, Y., Lu, X., Chen, L.-L., and Yang, L. (2014). Complementary sequence-mediated exon circularization. Cell 159, 134–147. doi: 10.1016/j.cell.2014.09.001
Zhang, Z., Yang, T., and Xiao, J. (2018). Circular RNAs: promising biomarkers for human diseases. Ebiomedicine 34, 267–274. doi: 10.1016/j.ebiom.2018.07.036
Zhao, T., Hu, Y., and Cheng, L. (2020a). Deep-DRM: a computational method for identifying disease-related metabolites based on graph deep learning approaches. Brief. Bioinform. doi: 10.1093/bib/bbaa212
Zhao, T., Hu, Y., Peng, J., and Cheng, L. (2020b). DeepLGP: a novel deep learning method for prioritizing lncRNA target genes. Bioinformatics 36, 4466–4472. doi: 10.1093/bioinformatics/btaa428
Zhao, X., Jiao, Q., Li, H., Wu, Y., Wang, H., Huang, S., et al. (2020c). ECFS-DEA: an. ensemble classifier-based feature selection for differential expression analysis on expression profiles. BMC Bioinformatics 21:43. doi: 10.1186/s12859-020-3388-y
Zhao, Y., Wang, F., and Juan, L. (2015). MicroRNA promoter identification in arabidopsis using multiple histone markers. Biomed Res. Int. 2015:861402.
Zhong, Z., Lv, M., and Chen, J. (2016). Screening differential circular RNA expression profiles reveals the regulatory role of circTCF25-miR-103a-3p/miR-107-CDK6 pathway in bladder carcinoma. Sci. Rep. 6:30919.
Keywords: circRNAs, database, machine learning, circRNAs identification, diseases
Citation: Jiao S, Wu S, Huang S, Liu M and Gao B (2021) Advances in the Identification of Circular RNAs and Research Into circRNAs in Human Diseases. Front. Genet. 12:665233. doi: 10.3389/fgene.2021.665233
Received: 07 February 2021; Accepted: 01 March 2021;
Published: 19 March 2021.
Edited by:
Fa Zhang, Institute of Computing Technology, Chinese Academy of Sciences (CAS), ChinaCopyright © 2021 Jiao, Wu, Huang, Liu and Gao. This is an open-access article distributed under the terms of the Creative Commons Attribution License (CC BY). The use, distribution or reproduction in other forums is permitted, provided the original author(s) and the copyright owner(s) are credited and that the original publication in this journal is cited, in accordance with accepted academic practice. No use, distribution or reproduction is permitted which does not comply with these terms.
*Correspondence: Mingyang Liu, cmVkcnVtZmZAMTYzLmNvbQ==; Bo Gao, MTY3ODcyOTU4OEBxcS5jb20=
†These authors have contributed equally to this work