- 1Beijing Advanced Innovation Center for Tree Breeding by Molecular Design, Beijing Forestry University, Beijing, China
- 2Peony International Institute, School of Landscape Architecture, Beijing Forestry University, Beijing, China
- 3Beijing Key Laboratory of Ornamental Plants Germplasm Innovation & Molecular Breeding, Beijing Forestry University, Beijing, China
- 4Key Laboratory of Genetics and Breeding in Forest Trees and Ornamental Plants of Ministry of Education, Beijing Forestry University, Beijing, China
- 5National Engineering Research Center for Floriculture, Beijing Forestry University, Beijing, China
- 6Beijing Guose Peony Technology Co. Ltd., Beijing, China
Allelic variation in floral quantitative traits, including the elements of flowers and fruits, is caused by extremely complex regulatory processes. In the genetic improvement of flare tree peony (Paeonia rockii), a unique ornamental and edible oil woody species in the genus Paeonia, a better understanding of the genetic composition of these complex traits related to flowers and fruits is needed. Therefore, we investigated the genetic diversity and population structure of 160 P. rockii accessions and conducted single-marker association analysis for 19 quantitative flower and fruit traits using 81 EST-SSR markers. The results showed that the population had a high phenotypic diversity (coefficients of variation, 11.87–110.64%) and a high level of genetic diversity (mean number of alleles, NA = 6.09). These accessions were divided into three subgroups by STRUCTURE analysis and a neighbor-joining tree. Furthermore, we also found a low level of linkage disequilibrium between these EST-SSRs and, by single-marker association analysis, identified 134 significant associations, including four flower traits with 11 EST-SSRs and 10 fruit traits with 32 EST-SSRs. Finally, based on the sequence alignment of the associated markers, P280, PS2, PS12, PS27, PS118, PS131, and PS145 may be considered potential loci to increase the yield of flare tree peony. These results laid the foundation for further analysis of the genetic structure of some key traits in P. rockii and had an obvious potential application value in marker-assisted selection breeding.
Introduction
Flare tree peony (Paeonia rockii; FTP) is one of the most representative species in the Paeonia section Moutan that is native to China (Hong and Pan, 1999). Approximately 300 cultivars have been derived from this species, mainly distributed in northwest China, consisting of a large cultivar group that is distinct from the common tree peony (Paeonia suffruticosa) in China (Cheng et al., 2005; Cheng and Yu, 2008; Yuan et al., 2012). In addition to being cultivated as an ornamental species since the Tang Dynasty (618–906 AD), FTP has rapidly developed into a new woody edible oil plant in recent years as its seeds contain a high unsaturated fatty acid content (Li et al., 2015a,b; Zhang et al., 2017a,b; Wang et al., 2020). Therefore, improving ornamental quality and increasing the potential yield have become important objectives for FTP breeding. Among them, ornamental quality mainly includes floral traits, while yield is a complex character affected by multiple factors, in which the most important contributing factor is the fruit trait (Liu and Cheng, 2020b). Quantitative flower and fruit traits are controlled by multiple genes and have moderate to high heritability. As conventional breeding methods take more than 10 years to develop new FTP cultivars with stable comprehensive characteristics (Cheng, 2012), molecular breeding approaches have become inevitable selection methods to breed new tree peony cultivars (Gao et al., 2013; Zhou et al., 2015).
The development of DNA markers has provided broad prospects for accelerating the selection of intricate quantitative traits in trees, especially in tree peonies (Du et al., 2013; Yu et al., 2013; Wang et al., 2014; Resende et al., 2017). Quantitative trait locus (QTL) mapping studies have been carried out on parental populations and attempts have been made to use QTLs for breeding (Cai et al., 2015; Guo et al., 2017; Zhang et al., 2019). When used in selective breeding, substantial deficiencies in QTL mapping have been widely discussed (Bernardo, 2008; Grattapaglia and Resende, 2011). Due to the method’s low mapping ability, only a few QTLs hidden under target traits were found, and the variances explained by these QTLs were overestimated (Beavis, 1998). Association mapping based on linkage disequilibrium (LD), proposed as a way to deconvolute QTL mapping, can identify natural alleles for specific phenotypes, providing a valuable opportunity to shorten breeding years and improve breeding efficiency (Neale and Savolainen, 2004; Thumma et al., 2005). In molecular marker-assisted selection (MAS) breeding, expressed sequence tag (EST)-simple repeat sequence (SSR) markers are ideal choices because they are highly variable, codominant, and highly informative (Varshney et al., 2005). In FTP, a few associations between polymorphisms in EST-SSR and certain traits have been reported (Wu et al., 2017; Cui et al., 2018; Liu and Cheng, 2020a). Wu et al. (2017) employed single-marker association analysis on a sample of 462 individuals to identify 46 significant associations, including 37 EST-SSRs involving 11 flower traits, which explained 2.68–23.97% (mean 5.50%) of the phenotypic variation. Cui et al. (2018) found that 15 EST-SSRs were significantly associated with five oil-related traits based on association analysis of 205 individuals with phenotypic traits over three consecutive years. In an association analysis of 420 individuals, Liu et al. identified 141 significant associations involving 17 yield-related traits and 41 EST-SSRs, and the phenotypic variation was relatively small (mean 11.34%; Liu and Cheng, 2020a). To reduce environmental disturbances and measurement errors, asexual reproduction is often necessary to increase the accuracy of phenotypic measurements (Du et al., 2013). Although there have been previous studies on the association analysis of important traits in FTP, the samples used were all individuals without asexual reproduction; the core germplasm resources of FTP were not used.
In this study, 160 accessions (each containing three clones) from core germplasm resources of FTP and 81 EST-SSRs were used for single-marker association analysis to explore the allelic effects of floral and fruit trait variation. The results of this study laid a foundation for further identifying the key trait linkage loci of FTP, which is of great value to the genetic improvement of related traits.
Materials and Methods
Plant Materials
A total of 160 accessions (each containing three clones), representing the core germplasm resources of FTP that had been demonstrated and described by Guo et al. (2020), were used in this study. All the samples were originally introduced from Gansu Province in Northwest China, which was the main cultivation area, and had been randomly cultivated in the same nursery field of Beijing Guose Peony Garden in Beijing, China (40°45'N, 115°97'E) for more than 10 years. All the measured plants, approximately 15 years old, can produce stable flowers and fruits annually. The complete list of accessions used in this study is provided in Supplementary Table S1.
Phenotyping
Seven flower quantitative traits, namely, flower diameter (FD), petal length (PL), petal width (PW), flare length (FL), flare width (FW), petal number (PN), and carpel number (CN), in the 160 accessions were measured at full bloom using digital calipers (YB5001B, Kraftwelle Industrial Co. Ltd., China) in May 2019. In addition, a total of 12 fruit quantitative traits, namely, the number of carpels with seeds (NCWS), multiple fruit fresh weight (MFFW), single fruit length (SFL), single fruit width (SFW), single fruit height (SFH), single fruit pericarp thickness (SFPT), multiple fruit seed number (MFSN), multiple fruit seed fresh weight (MFSFW), individual fruit number (IFN), individual seed number (ISN), individual fruit fresh weight (IFFW), and individual seed fresh weight (ISFW), were measured using digital calipers and electronic balances in September 2019. All measurements were carried out, as described in Supplementary Table S2. A total of nine flowers and nine fruits of each accession were collected, with three flowers and three fruits from one plant per replicate. The average value for each trait from three replicates for each accession was used for statistical and association analysis.
DNA Extraction and SSR Marker Genotyping
The total genomic DNA of 160 accessions was extracted from fresh young leaves using a DNAsecure plant kit (Tiangen Biotech, Beijing, China), following the manufacturer’s instructions. The quality of the extracted DNA was determined by electrophoresis on 2% agarose gels and visualization using a UnicoUV-visible Spectrophotometer (Agilent, Palo Alto, CA, United States). Then, the DNA were diluted with deionized water to 20–30 ng/μl and stored in a freezer at –20°C.
The polymorphism of 140 previously developed SSR markers (Homolka et al., 2010; Hou et al., 2011; Gai et al., 2012; Zhang et al., 2012; Yu et al., 2013; Wu et al., 2014; Liu and Cheng, 2020a) was evaluated using a random sample of 12 accessions. After screening, a total of 81 EST-SSRs (Supplementary Table S3) were used to reveal the polymorphism of these 160 accessions. The SSR-PCR amplification reaction was conducted in a 10-μl solution, including 5 μl of 2×Power Taq PCR Master MIX (Aidlab Biotechnologies, Beijing, China), 3 μl of ddH2O, 1 μl of 20–25 ng/μl genomic DNA, and 0.5 μl of 10 μmol/L each of forward and reverse primers, and the procedure was performed as described by Wu et al. (2014). The PCR products were differentiated by capillary electrophoresis using an ABI3730xl DNA Analyzer (Applied Biosystems, Carlsbad, CA, United States). Micro-Checker 2.2.3 (Van Oosterhout et al., 2004) was applied to identify and correct genotyping errors.
Data Analysis
The statistics software SPSS 18.0 (IBM Inc., Chicago, IL, United States; Davis, 2008) was used to analyze the average values of the investigated traits per accession. The coefficient of variation (CV) of each trait was calculated as follows: (standard deviation/mean) × 100%. The variation in 19 traits was estimated by one-way analysis of variance (ANOVA), and Pearson’s correlations between traits were calculated. Prior to ANOVA and Pearson’s correlation analysis, all SM data were tested for normality with the Shapiro-Wilk W test and for homogeneity of variance with Levene’s test, and the nonnormal data were logarithmically transformed. In addition, Benjamini-Hochberg (BH) FDR correction was used to correct the values of p for Pearson’s correlation analysis.
The capillary electrophoresis data were analyzed using GeneMarker 2.2.0. GenAIEx 6.5 (Peakall and Smouse, 2012) was used to calculate the following statistics: number of different alleles (NA), number of effective alleles (NE), Shannon’s information index (I), observed heterozygosity (Ho), expected heterozygosity (He), inbreeding coefficients (FIS), and Nei’s genetic diversity (GD). The polymorphism information content (PIC) of each locus was calculated using the Microsatellite Toolkit. GENEPOP 4.2 (Rousset, 2008) was used to detect microsatellite loci deviating from the Hardy-Weinberg equilibrium (HWE), and the results were applied to multiple tests with Bonferroni correction. Additionally, MEGA-X was used to construct a neighbor-joining (NJ) phylogenetic tree based on Nei’s unbiased genetic distance (Kumar et al., 2018).
The number of subpopulations (K) was detected by STRUCTURE 2.3.4 through an admixture model (Pritchard et al., 2000). First, the K value ranges from 1 to 10, and 10 independent operations were carried out for each K value, with a burn-in period of 100,000 times and 200,000 replications. Then, the results were uploaded to Structure Harvester (Earl and VonHoldt, 2012) to determine the final optimal K value. The optimum K value was inferred from LnP(D) and ΔK (Evanno et al., 2005). For 10 repetitions of each K value, CLUMPP 1.1 was used to analyze the results from replicate analyses (Jakobsson and Rosenberg, 2007). Then, the outputs of each K value were visualized using CLUMPP and DISTRUCT (Rosenberg, 2004). The matrix corresponding to the K value of the optimal population structure was used for association analysis to correct false positives.
The degree of LD between loci was evaluated by the square of the allelic frequency (r2), which was calculated by using TASSEL 2.0.1. r2 = 0.1 was taken as the critical value to determine whether two loci had LD (r2 > 0.1: LD). Then, a mixed linear model (MLM) of TASSEL 2.0.1 was used to incorporate SSR data, phenotypic traits, Q matrix, and kinship matrix for association analysis. Q was the matrix of the optimal K value obtained through Structure Harvester. The kinship matrix was calculated by SPAGeDi 1.2 (Hardy and Vekemans, 2002). An adjusted value of p was employed for association analysis using false discovery rate (FDR) correction under QVALUE in R (Storey and Tibshirani, 2003). The ratio of dominance (d) to additive (a) effects was used to assess the gene effects of the significant loci obtained by association analysis. The boundaries of additive effect, partial to full dominance and overdominance, were |d/a| ≤ 0.50, 0.50 < |d/a| < 1.25, and |d/a| > 1.25, respectively. The calculation formulas of additive (a) effects and dominance (d) were as follows: 2a = |GBB − Gbb|; d = GBb − 0.5 (GBB + Gbb; G represents the average of phenotypes corresponding to genotype, BB and bb: homozygous genotypes, and Bb: heterozygous genotypes; Eckert et al., 2009).
Open Reading Frame Finder (ORF Finder) was used to find the complete ORFs of the associated marker sequences and translate the ORF sequences into protein sequences (Rombel et al., 2002). Then, the results were compared in the Arabidopsis Information Resource (TAIR) to find protein sequences with higher homology (Berardini et al., 2015). Finally, DNAMAN was used to construct a phylogenetic tree and protein sequence alignment map.
Results
Statistical and Correlation Analyses of Phenotypic Traits
As the tested samples can represent the current situation of FTP germplasm resources in China, ANOVA showed that the phenotypic variation range of all measured traits were wide (Table 1). The CVs ranged from 11.87% (FD) to 110.64% (ISFW), with an average of 48.01%. The CVs of fruit traits (mean 58.44%) were higher than those of flower traits (mean 30.13%), among them the traits ISFW (110.64%), PN (109.70%), and ISN (102.77%) were relatively higher. Correlation analysis of different traits showed 81 significant correlations (p < 0.05), of which 65 were very significant correlations (p < 0.01; Table 2). Considering the flower traits, excluding FL and PN, FW and CN, and PN and CN, all other traits were significantly correlated. Among the 66 correlation factors in fruit traits, 39 were significantly correlated. Especially when we considered ISFW as the yield index, MFFW, SFL, SFW, MFSN, MFSFW, IFN, ISN, and IFFW were very significantly positively correlated with ISFW, and the correlation coefficients were 0.726, 0.474, 0.599, 0.730, 0.820, 0.515, 0.960, and 0.814, respectively.
Genetic Diversity
In total, 81 polymorphic EST-SSRs were used to evaluate the diversity of 160 accessions (Table 3). Then, 493 alleles were identified, ranging from 2 to 20 (NA). The NE ranged from 1.006 to 8.516, with an average of 2.603. The I varied from 0.021 to 2.357 (mean 1.026). The mean values of HO and HE were 0.501 and 0.524, respectively. The mean FIS of 81 SSRs was −0.439, among which 63 pairs were negative. In addition, the PIC values ranged from 0.006 to 0.871, with an average of 0.476. After Bonferroni multiple comparisons, 43 SSR sites deviated significantly from HWE. Therefore, these deviated sites were removed in the subsequent analysis and 38 SSR sites were ultimately used for subsequent population structure analysis and association mapping.
Population Structure
Through STRUCTURE analysis of 160 accessions with 38 EST-SSRs, as the K value increased, LnP(D) progressively increased overall (Figure 1). When K reached 3, the rising trend of LnP(D) slowed down. Moreover, the ΔK value corresponding to the maximum K value was the population structure; therefore, the 160 accessions could be divided into three subgroups. The outputs of K = 3 were visualized through CLUMPP and DISTRUCT, and all samples showed a wide range of mixed lineages. Then, we visualized the outputs from K = 2–5 and found that each sample was highly heterozygous. The Q matrix output of the three subgroups can be used for structure-based association analysis. The phylogenetic tree divided these samples into three major clades (Figure 2), revealing similar clustering results to those obtained using STRUCTURE. In summary, the two methods classified these samples into three subgroups.
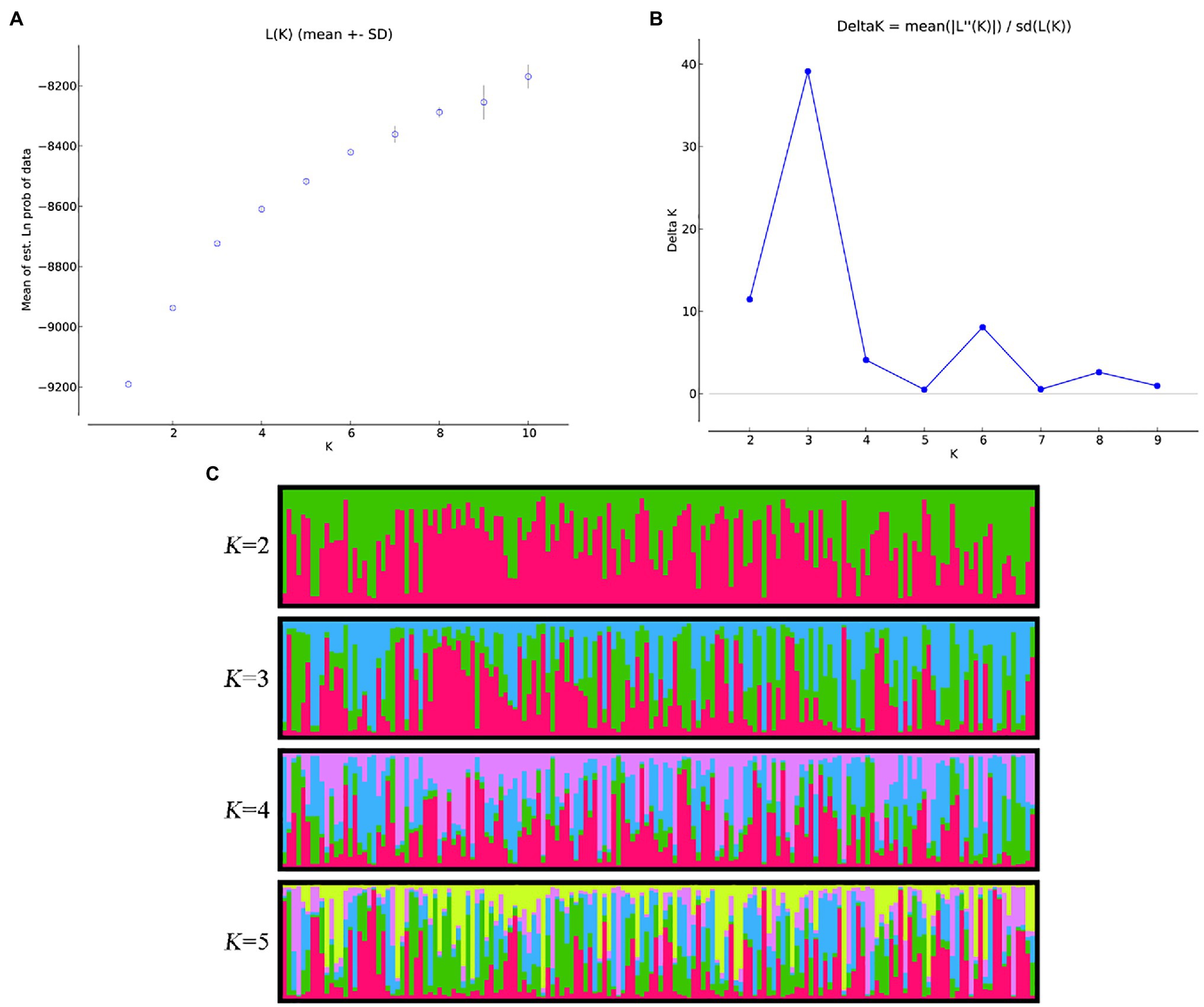
Figure 1. Estimation of genetic structure of 160 accessions using 38 simple repeat sequences (SSRs) based on the STRUCTURE. (A) Log probability data [LnP(D)] for each K value (10 replicates). (B) ΔK estimates of the posterior probability distribution of the data for a given K. (C) Estimated population structure and clustering of 160 accessions with K = 2–5. Accessions are shown by thin vertical lines.
LD Level
The LD levels of 38 EST-SSRs in the 160 accessions were evaluated (Figure 3). Based on the r2 estimates, only 0.71% (r2 ≥ 0.1) of the loci sites had significant LD. In all 698 locus pairs (r2 < 0.1), 17.62, 30.23, and 50.14% of the locus pairs displayed linkage equilibrium at p < 0.05, p < 0.01, and p < 0.001, respectively. Therefore, the overall LD level of 38 EST-SSRs in the 160 accessions was low, and most loci were in linkage equilibrium with each other. However, there were also several loci with significant LD levels between them, such as marker P235-PS50 (r2 > 0.3; p < 0.001).
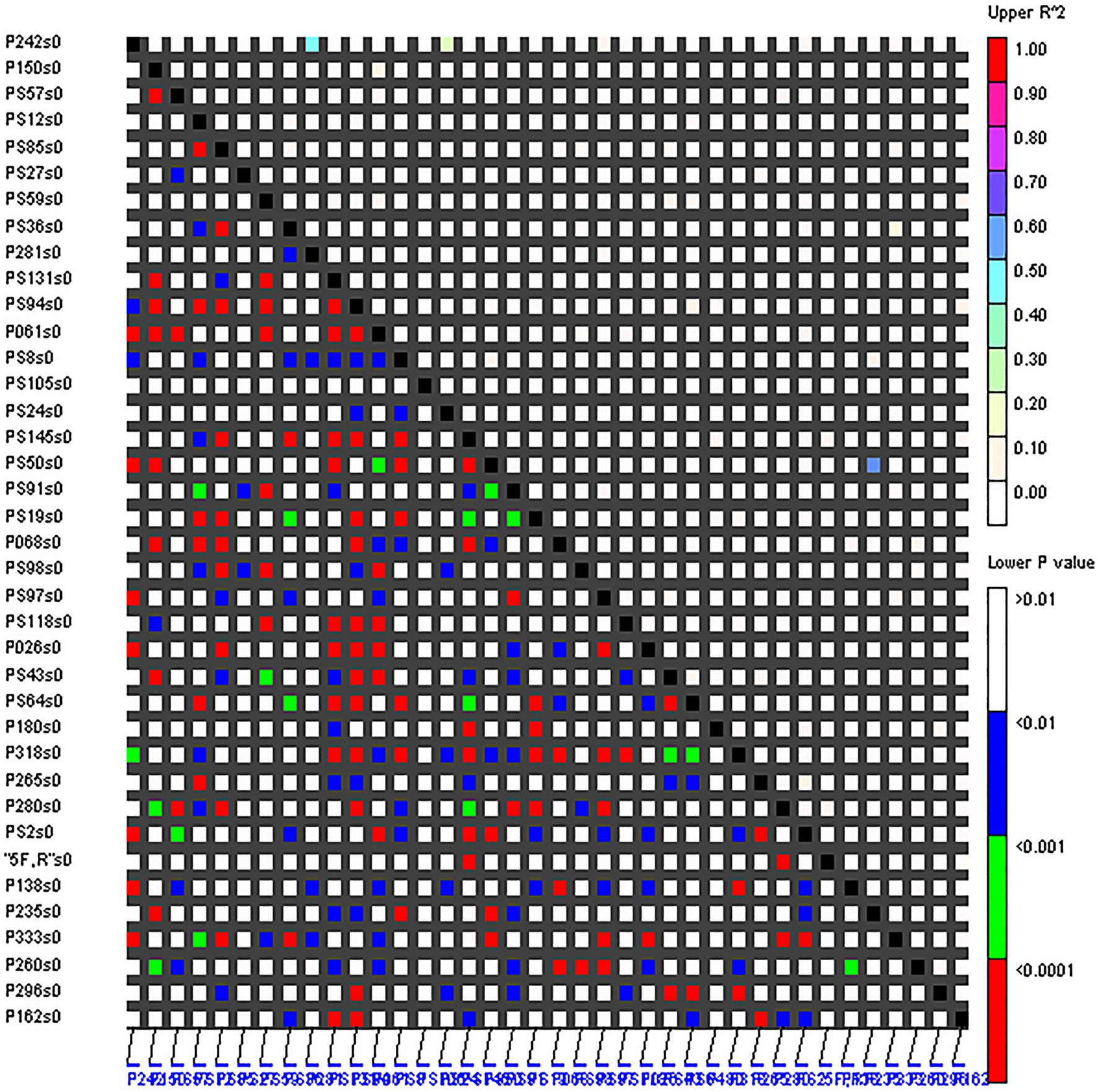
Figure 3. Pairwise linkage equilibrium (LD; r2) between 38 SSRs. The x- and y-axes represent the 38 SSRs, r2 < 0.1 represents linkage equilibrium, and the different colors correspond to the thresholds of r2 and p.
Single-Marker Associations of Floral Traits
For flower traits, 266 (38 EST-SSRs × 7 traits) single-marker associations were detected by the MLM model, and a total of 21 (7.89%) significant associations between four floral traits and 14 EST-SSRs were detected under the condition of p < 0.01. Then, FDR test was carried out on these 21 significantly associated combinations (Q < 0.05), and 17 significantly associated combinations of four flower traits and 11 EST-SSRs were ultimately identified, with an explainable rate of 2.23–26.34% (mean 11.80%; Table 4). The statistical results of the gene effects indicated that 2 (11.76%) associated combinations presented additive effects, 2 (11.76%) presented partial to full dominance, and 13 (76.47%) presented overdominance.
The number of significantly associated combinations for each trait ranged from two (FD) to eight (FL). There were two markers that were significantly related to FD and CN, and the highest interpretation rates were found for locus PS19, which were 26.34 and 25.38%, respectively. There were eight EST-SSRs that were significantly related to FL, with interpretation rates of 5.03–12.53% (mean 10.15%), and the highest explanatory rate was locus PS91. Among them, there were one and seven combinations with additive effects and overdominance, respectively. There were five EST-SSRs that were significantly related to FW, with explanatory rates ranging from 6.72 to 14.68% (mean 9.43%), and the highest interpretation rate was locus PS43. No significant associations with PL, PW, and PN were detected in this study. Additionally, five EST-SSRs were markedly associated with more than one trait. For instance, P26 was significantly related to FD, FL, and CN, while P235, P281, and PS24 were significantly associated with FL and FW.
Single-Marker Associations of Fruit Traits
For fruit traits, 456 (38 EST-SSRs × 12 traits) single-marker associations were detected by the MLM model, and a total of 118 (25.88%) significant associations between 10 fruit traits and 32 EST-SSRs were detected under the condition of p < 0.01. Then, an FDR test was carried out on these 118 significantly associated combinations (Q < 0.05), and 117 significantly associated combinations of 10 fruit traits and 32 EST-SSRs were ultimately identified, with explanatory rates of 0.18–43.40% (average 17.68%; Table 5). The statistical results of gene effects showed that 13 (11.11%) associated combinations appeared to have additive effects, 28 (23.93%) appeared to have partial to full dominance, and 76 (64.96%) appeared to have overdominance.
The number of significantly associated combinations for each trait ranged from 1 (IFN) to 25 (MFSN). Of these, IFN had one significant association; NCWS had two significant associations; SFW had three significant associations; ISN had eight significant associations; SFL had 12 significant associations; IFFW had 13 significant associations; MFFW and IFSW had 15 significant associations each; MFSFW had 23 significant associations; and MFSN had 25 significant associations. We also found that in ISN, ISFW, and MFSFW, the interpretation rates of P280 were the highest, while in MFFW and SFL, the interpretation rates of P318 were the highest. No locus significantly associated with SFPT and SFH was detected in this study. The phenomenon in which one marker was significantly associated with multiple traits was more common in this part of the study. In the correlation analysis of fruit traits, ISN, IFFW, and ISFW were all significantly correlated with each other. In the association analysis results, six markers were significantly associated with these three traits at the same time, namely, loci P150, P242, P280, PS12, PS59, and PS91. Similarly, loci P242, PS12, PS131, PS145, PS24, PS27, PS50, PS59, PS85, and PS91 were significantly associated with MFSN, MFFW, and MFSFW.
There were 10 marker-trait combinations with explanatory rates exceeding 30%, including the following: MFFW associated with PS145, PS85, P318, and PS12; SFL associated with P318; MFSN associated with PS145; MFSFW associated with P280, PS145, and PS12; and IFFW associated with PS85. Through sequence alignment via the National Center for Biotechnology Information (NCBI), the gene sequences of P280, PS12, and PS145 were related to fruit and seed development in other species. The explanatory rate of P280 with MFSFW was the highest, at 43.4%, which was predicted to be WRINKLED Like 1 (WRIL1) belonging to the basal ANT subgroup of the APETALA2/ETHYLENE-RESPONSIVE ELEMENT-BINDING FACTOR (AP2/ERF) family AINTEGUMENTA (ANT) group. This gene, which has a typical AP2 domain, has a similarity of 44.79% with AtWRIL1 in Arabidopsis, and its main function is now known to regulate lipid biosynthesis (Supplementary Figure S1; Kong et al., 2020; Zhai et al., 2021). After comparison, PS145 had no similarity with the genes studied in the database, but the protein sequences that were translated had WD40 domains, which can control seed weight and volume in Arabidopsis (You et al., 2011). The predicted results of PS12 showed that it has the highest similarity with MYB5 of the MYB gene family in Arabidopsis and is mainly involved in seed coat development and oil biosynthesis (Supplementary Figure S2; Li et al., 2009, 2020; Cheng et al., 2021). In addition, PS2 and PS131 were also significantly associated with fruit traits in Liu’s research (Liu and Cheng, 2020a). PS2 was associated with MFFW, SFL, SFW, and MFSFW, and the explanation rate of MFFW was the highest, at 26.8%. This site has a MADS-box functional domain, and the study of the similar AtSEP3 sequence in Arabidopsis showed that it controlled carpel and ovule development (Supplementary Figure S3; Liljegren et al., 2000; Favaro et al., 2003; Renard et al., 2020). The predicted result of PS131 was WRKY22 of the WRKY gene family, which can mediate dark-induced leaf senescence in Arabidopsis (Supplementary Figure S4; Zhou et al., 2011; Hsu et al., 2013; Gaudinier et al., 2018). Furthermore, WRKY22 (PS131), GATA8 (PS118; Supplementary Figure S5), and ERF3 (PS27; Supplementary Figure S6) jointly participate in nitrogen metabolism to regulate growth and development in Arabidopsis (Dubois et al., 2015; Taylor-Teeples et al., 2015; Gaudinier et al., 2018); whether these genes affect fruit and seed development in FTP through nitrogen metabolism needs further research. Therefore, the abovementioned markers provide important references for the molecular breeding of FTP.
Discussion
Genetic Diversity of the Associated Population
The selection of populations with high genetic diversity and molecular markers with high polymorphism is crucial for association analysis (Ingvarsson, 2008). Here, 19 quantitative traits and 81 SSRs were utilized to evaluate the diversity of 160 accessions. In previous studies of phenotypic variation of FTP, Pang et al. (2012) reported that the CVs of 32 traits in 150 individuals ranged from 10 to 30%, while Wu et al. (2017) found that the CVs of 29 quantitative traits in 462 individuals ranged from 9.52 to 112.1%. In addition, Liu et al. studied 24 quantitative traits of 420 individuals and found that the CVs ranged from 12.03 to 106.63% (Liu and Cheng, 2020a). This study revealed CVs ranging from 11.87 to 110.64% for 19 quantitative traits in 160 accessions, which was consistent with the results of Wu et al. and Liu et al. Because the sampling strategies of these three studies were essentially the same, all the associated populations were constructed through the screening of a large number of FTP genotypes, reflecting the genetic background and structure of cultivated FTP to a certain extent. Additionally, the phenotypic variation of the 160 accessions in this study was similar to the CVs of other studies, indicating that the associated population composed of 160 accessions has high phenotypic diversity.
Phenotypic variation analysis allowed us to obtain a basic understanding of the associated population, but phenotypic variation was easily affected by the environment, and some individuals exhibited small differences in phenotypic variation, which was difficult to distinguish only by phenotypic traits. Therefore, it was very important to use molecular markers to analyze genetic diversity and population structures. In this study, 81 SSRs detected a total of 493 alleles in 160 accessions, and the NA was 6.09, which was larger than 40 SSRs in 462 individuals (NA = 4.5; Wu et al., 2017) and 34 SSRs in 282 cultivars (NA = 5.441; Guo et al., 2020), but smaller than 12 SSRs in 335 individuals of wild P. rockii (NA = 9.15; Yuan et al., 2012). This suggested that, on the one hand, the SSR primers and plant materials used in this study had comparatively higher allele variation, and on the other hand, the diverse primers and materials used in the studies gave rise to discrepancies. Additionally, the mean FIS of 81 SSRs was −0.439, among which 63 pairs were negative, demonstrating the existence of a heterozygote surplus in the 160 accessions. We speculated that this was correlated with the hybridization origin and self-incompatibility of tree peony, which was in accordance with the reported results (Yuan et al., 2014; Zhou et al., 2014). Simultaneously, compared with other outcrossing woody plants, the genetic diversity of this species was at a moderate level (PIC = 0.476; Botstein et al., 1980), which was higher than that of Populus tomentosa (Du et al., 2012), but inferior to that of Prunus avium (Ganopoulos et al., 2011).
LD and Population Structure
The level of LD of the association population was a prerequisite for association analysis. There was a low level of LD between different SSR markers in this study. In general, the LD levels of outcrossing woody plants were low (Kulheim et al., 2009; Chhetri et al., 2019), and tree peony was also an outcrossing species so the LD levels of FTP in other studies were also low (Wu et al., 2017; Cui et al., 2018; Liu and Cheng, 2020a). Moreover, we speculated that many human interventions, such as selective breeding, were also important contributors to the low LD levels. However, the mechanism of the LD level was still not clear for this associated population because the distances of these loci on chromosomes were unclear. Additionally, low LD levels at a small number of SSR sites did not represent the level of the entire genome or the intergenomic region. Therefore, it is necessary to use multiple markers distributed throughout the whole genome to determine the LD level in one population, because different types of markers may provide different types of insight depending on their characteristics (Neale and Savolainen, 2004).
In addition to considering the LD level of the associated population, we should also pay attention to the genetic structure of the population, because in practical research, for various reasons, it is impossible to have a population without population structure. The effect of sample structure in populations used for genetic association studies has been well documented and identified as the cause of some false associations (Newman et al., 2001; Kang et al., 2010; Sul et al., 2018). Predicting the genetic structure of the population was the premise of association analysis, which can improve the accuracy and avoid the appearance of false positives as much as possible (King et al., 2010). SSR loci that deviate from HWE may indicate genotyping error, inbreeding, population subdivision, or selection (Balding, 2006). In this study, after the Bonferroni correction for multiple testing, 43 loci were found to deviate significantly from HWE, and they were excluded. Then, the two methods were utilized to evaluate population structure and produced coincident consequences. Through STRUCTURE analysis, the 160 accessions were divided into three subgroups, and to a large extent, this was supported by the NJ tree analysis, which was similar to previous reports of three subgroups in FTP (Wu et al., 2017; Guo et al., 2020). Furthermore, Yuan et al. (2012) revealed that 335 wild P. rockii individuals were mainly divided into three subpopulations, which were strongly linked to the geographical distribution pattern of wild P. rockii. We hypothesized that the three subgroups prescribed in this research correspond to the same three gene banks that were previously reported and also reflect their geographic origins.
Even if the subgroup structure of the associated population was considered, false positives could not be completely controlled by the general linear model (GLM; Sun et al., 2015). MLM has been demonstrated to be effective in controlling false positives and can effectively control the error rate due to its consideration of the population structure matrix (Q) and the kinship matrix (K; Yu et al., 2006). To further improve the accuracy of the association analysis results, FDR correction was carried out for all values of p of associations, greatly reducing the expansion of values of p. In this study, we found 139 significant associations, but this number dropped to 134 after FDR correction, akin to previous studies (Wu et al., 2017; Liu and Cheng, 2020a).
Associations With Floral and Fruit Traits in Flare Tree Peony
In this study, 11 SSRs were demonstrated to be significantly associated with four floral traits, four of which were derived from the transcriptome sequences of tree peony flower buds (Wu et al., 2014), and a total of 117 significant associations of 32 SSR markers related to 10 fruit traits were identified. More than two loci were significantly associated with each trait, indicating that quantitative traits were controlled by microeffect polygenes. Complex quantitative traits of plants for association analysis can be significantly associated with many sites (Sun et al., 2015); similar conclusions have been reported in other studies of trees (Dillon et al., 2012; Porth et al., 2013). Simultaneously, we also found that a marker was significantly associated with multiple traits, such as P26, which was associated with FD, FL, and CN, which may be due to the significant correlation between these phenotypic traits, and may reflect the characteristics of pleiotropism (Thudi et al., 2014).
Phenotyping is an important part of tree association analysis. Typical associated populations are usually composed of different unrelated individuals grown under the same ambient conditions and augment what is known about the measurement of phenotypes, which must usually be asexually reproduced to reduce environmental interference and measurement errors (Du et al., 2013). Although there have been previous studies on the association analysis of important traits in FTP, the samples used were all individuals and had no asexual reproduction (Wu et al., 2017; Cui et al., 2018; Liu and Cheng, 2020a). Hence, in this study, we used 480 phenotypes (160 genotypes × 3 clones) to compensate for the limited number of EST-SSR markers, and repeated data from each accession could be integrated to generate a phenotypic mean for analysis, which reduced the impact of measurement errors. In this study, the average explanatory rates of floral and fruit traits were 11.80 and 17.68%, respectively, higher than those of Wu et al. (2017; flower traits: 5.50%) and Liu and Cheng (2020a; fruit traits: 6.53%), which also reflected the improvement of the effectiveness of association mapping.
Additionally, replication of genotype-phenotype associations is crucial in association mapping to distinguish false-positive associations. Therefore, of the 38 SSRs used in this study, 17 markers were in conformity with Wu et al. (2017), but the association analysis results of flower traits in the two studies were inconsistent. This may be due to differences in sample size, gene-environment interactions, genetic background, gene-gene interactions, or other factors; therefore, some real associations may not be repeated in unrelated datasets (Greene et al., 2009; Beaulieu et al., 2011; Du et al., 2013). In addition, there were 21 markers identical to those described in Liu et al. Most of the associations were different, but associations between the same markers and traits were still found in the two studies. In both studies, PS2 was significantly associated with MFFW, PS12 was significantly associated with MFFW and MFSFW, and PS131 was significantly associated with SFL (Liu and Cheng, 2020a). We hypothesized that these repeated associations might help identify important genomic regions. These findings were also of great value in the use of marker-assisted selective breeding for trait improvement. Future studies will require multiple germplasm populations to combine multiyear and multiplot phenotypic data to validate the developed markers.
Flare tree peony has developed into an emerging woody oil crop in China, and its output mainly refers to ISFW. PS12, PS27, PS131, PS118, and ps280 were significantly associated with ISFW, and may be the key sites affecting the yield. Studies have shown that the MYB5 gene of PS12 is involved in seed coat development to control seed size and affect yield (Li et al., 2008; Su et al., 2011; Dong et al., 2017). The oil content in tree peony seeds can reach 27–33%, which can directly affect seed weight (Cui et al., 2016). The WRIL1 gene corresponding to P280 may be an important gene regulating oil synthesis in tree peony. Nitrogen metabolism is closely related to plant growth and development, thereby affecting yield (Tilman et al., 2002). In this study, GATA8 (PS118), ERF3 (PS27), and WRKY22 (PS131) may form a network to participate in nitrogen metabolism. In addition, WRKY22 was also involved in leaf senescence. Relevant studies have shown that the combination of related genes can delay the senescence process of plants and significantly increase yield (Distelfeld et al., 2014; Lira et al., 2017). This showed that these loci were potential genes to increase the yield of FTP, which is worthy of further study.
The analysis of the genetic regulatory relationships between different significant association sites of the same quantitative trait was helpful for breeding with significant association combinations. For example, in floral traits, FD was an important ornamental character that determined its ornamental value to a large extent. P26 was significantly associated with FD and showed an overdominant effect (d/a = 11.5136), indicating that individuals with P26 heterozygosity sites may produce flowers of larger diameter. For fruit traits, ISFW was considered a yield indicator, with 15 markers significantly associated with it. Among them, 11 markers, namely, P242, PS59, PS91, P280, PS24, PS131, PS64, PS145, P150, PS118, and PS27, showed the same pattern of gene action (overdominance), while the effects of P280 (d/a = 1.4674) and PS64 (d/a = 4.0449) were positive, showing that individuals with P280 and PS64 heterozygous loci might generate heavier seeds. In summary, marker combinations based on the gene effect value of associated loci can be used for the early selection of target traits.
Data Availability Statement
The datasets presented in this study can be found in online repositories. The names of the repository/repositories and accession number(s) can be found in the article/Supplementary Material.
Author Contributions
XG and FC: conceptualization and validation. XG: formal analysis, data curation, writing – original draft preparation, and visualization. XG, XC, and XT: investigation. XC and XT: resources. XG, CH, FC, YZ, XC, and XT: writing – review and editing. FC: supervision and project administration. All authors contributed to the article and approved the submitted version.
Funding
This research was mainly supported by the Special Foundation for National Science and Technology Basic Research Program of China (Grant No. 2019FY100802), the National Key Research and Development Program of China (Grant No. 2019YFD1001502), and the Science and Technology Project of Beijing (Grant No. Z181100002518001).
Conflict of Interest
XC and XT are employees of Beijing Guose Peony Technology Co. Ltd., Beijing, China.
The remaining authors declare that the research was conducted in the absence of any commercial or financial relationships that could be construed as a potential conflict of interest.
Publisher’s Note
All claims expressed in this article are solely those of the authors and do not necessarily represent those of their affiliated organizations, or those of the publisher, the editors and the reviewers. Any product that may be evaluated in this article, or claim that may be made by its manufacturer, is not guaranteed or endorsed by the publisher.
Acknowledgments
We would like to thank Lidan Sun (Beijing Forestry University) for her suggestions on this study.
Supplementary Material
The Supplementary Material for this article can be found online at: https://www.frontiersin.org/articles/10.3389/fgene.2021.664814/full#supplementary-material
References
Balding, D. J. (2006). A tutorial on statistical methods for population association studies. Nat. Rev. Genet. 7, 781–791. doi: 10.1038/nrg1916
Beaulieu, J., Doerksen, T., Boyle, B., Clément, S., Deslauriers, M., Beauseigle, S., et al. (2011). Association genetics of wood physical traits in the conifer white spruce and relationships with gene expression. Genetics 188, 197–214. doi: 10.1534/genetics.110.125781
Beavis, W. D. (1998). “QTL analyses: power, precision, and accuracy,” in Molecular Dissection of Complex Traits. ed. A. H. Patterson (Boca Raton, FL: CRC Press), 145–162.
Berardini, T. Z., Reiser, L., Li, D., Mezheritsky, Y., Muller, R., Strait, E., et al. (2015). The arabidopsis information resource: making and mining the “gold standard” annotated reference plant genome. Genesis 53, 474–485. doi: 10.1002/dvg.22877
Bernardo, R. (2008). Molecular markers and selection for complex traits in plants: learning from the last 20 years. Crop Sci. 48, 1649–1664. doi: 10.2135/cropsci2008.03.0131
Botstein, D., White, R. L., Skolnick, M., and Davis, R. W. (1980). Construction of a genetic linkage map in man using restriction fragment length polymorphisms. Am. J. Hum. Genet. 32, 314–331. doi: 10.1016/0165-1161(81)90274-0
Cai, C. F., Cheng, F. Y., Wu, J., Zhong, Y., and Liu, G. X. (2015). The first high-density genetic map construction in tree peony (Paeonia sect. Moutan) using genotyping by specific-locus amplified fragment sequencing. PLoS One 10:e128584. doi: 10.1371/journal.pone.0128584
Cheng, F. Y. (2012). Advances in the breeding of tree peonies and a cultivar system for the cultivar group. Int. J. Plant Breed. 1, 89–104.
Cheng, F. Y., Li, J. J., Chen, D. Z., and Zhang, Z. S. (2005). Chinese Paeonia rockii. Beijing: Chinese Forestry Publishing House.
Cheng, F. Y., and Yu, X. N. (2008). Flare tree peonies (Paeonia rockii hybrids) and the origin of the cultivar group. Acta Hortic. 766, 375–382. doi: 10.17660/ActaHortic.2008.766.49
Cheng, T. H., Zhao, P., Ren, Y. R., Zou, J., and Sun, M. X. (2021). AtMIF1 increases seed oil content by attenuating GL2 inhibition. New Phytol. 229, 2152–2162. doi: 10.1111/nph.17016
Chhetri, H. B., Macaya-Sanz, D., Kainer, D., Biswal, A. K., Evans, L. M., Chen, J. G., et al. (2019). Multi-trait genome-wide association analysis of Populus trichocarpa identifies key polymorphisms controlling morphological and physiological traits. New Phytol. 223, 293–309. doi: 10.1111/nph.15777
Cui, H. L., Chen, C. R., Huang, N. Z., and Cheng, F. Y. (2018). Association analysis of yield, oil and fatty acid content, and main phenotypic traits in Paeonia rockii as an oil crop. J. Hortic. Sci. Biotechnol. 93, 425–432. doi: 10.1080/14620316.2017.1381045
Cui, H. L., Cheng, F. Y., and Peng, L. P. (2016). Determination of the fatty acid composition in tree peony seeds using near-infrared spectroscopy. J. Am. Oil Chem. Soc. 93, 943–952. doi: 10.1007/s11746-016-2839-0
Davis, J. W. (2008). Handbook of univariate and multivariate data analysis and interpretation with SPSS. Am. Stat. 62:268. doi: 10.1198/000313008X332287
Dillon, S. K., Brawner, J. T., Meder, R., Lee, D. J., and Southerton, S. G. (2012). Association genetics in Corymbia citriodora subsp. variegata identifies single nucleotide polymorphisms affecting wood growth and cellulosic pulp yield. New Phytol. 195, 596–608. doi: 10.1111/j.1469-8137.2012.04200.x
Distelfeld, A., Avni, R., and Fischer, A. M. (2014). Senescence, nutrient remobilization, and yield in wheat and barley. J. Exp. Bot. 65, 3783–3798. doi: 10.1093/jxb/ert477
Dong, H., Dumenil, J., Lu, F. H., Na, L., Vanhaeren, H., Naumann, C., et al. (2017). Ubiquitylation activates a peptidase that promotes cleavage and destabilization of its activating E3 ligases and diverse growth regulatory proteins to limit cell proliferation in Arabidopsis. Genes Dev. 31, 197–208. doi: 10.1101/gad.292235.116
Du, Q. Z., Pan, W., Xu, B. H., Li, B. L., and Zhang, D. Q. (2013). Polymorphic simple sequence repeat (SSR) loci within cellulose synthase (PtoCesA) genes are associated with growth and wood properties in Populus tomentosa. New Phytol. 197, 763–776. doi: 10.1111/nph.12072
Du, Q. Z., Wang, B. W., Wei, Z. Z., Zhang, D. Q., and Li, B. L. (2012). Genetic diversity and population structure of Chinese white poplar (Populus tomentosa) revealed by SSR markers. J. Hered. 103, 853–862. doi: 10.1093/jhered/ess061
Dubois, M., Van den Broeck, L., Claeys, H., Van Vlierberghe, K., Matsui, M., and Inzé, D. (2015). The ETHYLENE RESPONSE FACTORs ERF6 and ERF11 antagonistically regulate mannitol-induced growth inhibition in Arabidopsis. Plant Physiol. 169, 166–179. doi: 10.1104/pp.15.00335
Earl, D. A., and VonHoldt, B. M. (2012). STRUCTURE HARVESTER: a website and program for visualizing STRUCTURE output and implementing the Evanno method. Conserv. Genet. Resour. 4, 359–361. doi: 10.1007/s12686-011-9548-7
Eckert, A. J., Bower, A. D., Wegrzyn, J. L., Pande, B., Jermstad, K. D., Krutovsky, K. V., et al. (2009). Association genetics of coastal Douglas fir (Pseudotsuga menziesii var. menziesii, Pinaceae). I. Cold-hardiness related traits. Genetics 182, 1289–1302. doi: 10.1534/genetics.109.102350
Evanno, G., Regnaut, S., and Goudet, J. (2005). Detecting the number of clusters of individuals using the software structure: a simulation study. Mol. Ecol. 14, 2611–2620. doi: 10.1111/j.1365-294X.2005.02553.x
Favaro, R., Pinyopich, A., Battaglia, R., Kooiker, M., Borghi, L., Ditta, G., et al. (2003). MADS-Box protein complexes control carpel and ovule development in Arabidopsis. Plant Cell 15, 2603–2611. doi: 10.1105/tpc.015123
Gai, S. P., Zhang, Y. X., Mu, P., Liu, C. Y., Liu, S., Dong, L., et al. (2012). Transcriptome analysis of tree peony during chilling requirement fulfillment: assembling, annotation and markers discovering. Gene 497, 256–262. doi: 10.1016/j.gene.2011.12.013
Ganopoulos, I. V., Kazantzis, K., Chatzicharisis, I., Karayiannis, I., and Tsaftaris, A. S. (2011). Genetic diversity, structure and fruit trait associations in Greek sweet cherry cultivars using microsatellite based (SSR/ISSR) and morpho-physiological markers. Euphytica 181, 237–251. doi: 10.1007/s10681-011-0416-z
Gao, Z. M., Wu, J., Liu, Z. A., Wang, L. S., Ren, H. X., and Shu, Q. Y. (2013). Rapid microsatellite development for tree peony and its implications. BMC Genomics 14:886. doi: 10.1186/1471-2164-14-886
Gaudinier, A., Rodriguez-Medina, J., Zhang, L. F., Olson, A., Liseron-Monfils, C., Bagman, A., et al. (2018). Transcriptional regulation of nitrogen-associated metabolism and growth. Nature 563, 259–264. doi: 10.1038/s41586-018-0656-3
Grattapaglia, D., and Resende, M. D. V. (2011). Genomic selection in forest tree breeding. Tree Genet. Genomes 7, 241–255. doi: 10.1007/s11295-010-0328-4
Greene, C. S., Penrod, N. M., Williams, S. M., and Moore, J. H. (2009). Failure to replicate a genetic association may provide important clues about genetic architecture. PLoS One 4:e5639. doi: 10.1371/journal.pone.0005639
Guo, X., Cheng, F. Y., and Zhong, Y. (2020). Genetic diversity of Paeonia rockii (flare tree peony) germplasm accessions revealed by phenotypic traits, EST-SSR markers and chloroplast DNA sequences. Forests 11:672. doi: 10.3390/f11060672
Guo, Q., Guo, L. L., Zhang, L., Zhang, L. X., Ma, H. L., Guo, D. L., et al. (2017). Construction of a genetic linkage map in tree peony (Paeonia sect. Moutan) using simple sequence repeat (SSR) markers. Sci. Hortic. 219, 294–301. doi: 10.1016/j.scienta.2017.03.017
Hardy, O. J., and Vekemans, X. (2002). Spagedi: a versatile computer program to analyse spatial genetic structure at the individual or population levels. Mol. Ecol. Notes 2, 618–620. doi: 10.1046/j.1471-8286.2002.00305.x
Homolka, A., Berenyi, M., Burg, K., Kopecky, D., and Fluch, S. (2010). Microsatellite markers in the tree peony, Paeonia suffruticosa (Paeoniaceae). Am. J. Bot. 97, 42–44. doi: 10.3732/ajb.1000127
Hong, D. Y., and Pan, K. Y. (1999). Taxonomical history and revision of Paeonia sect. Moutan (Paeoniaceae). Acta Phytotaxon. Sin. 37, 48–65.
Hou, X. G., Guo, D. L., Cheng, S. P., and Zhang, J. Y. (2011). Development of thirty new polymorphic microsatellite primers for Paeonia suffruticosa. Biol. Plant. 55, 708–710. doi: 10.1007/s10535-011-0172-x
Hsu, F. C., Chou, M. Y., Chou, S. J., Li, Y. R., Peng, H. P., and Shih, M. C. (2013). Submergence confers immunity mediated by the WRKY22 transcription factor in Arabidopsis. Plant Cell 25, 2699–2713. doi: 10.1105/tpc.113.114447
Ingvarsson, P. K. (2008). Multilocus patterns of nucleotide polymorphism and the demographic history of Populus tremula. Genetics 180, 329–340. doi: 10.1534/genetics.108.090431
Jakobsson, M., and Rosenberg, N. A. (2007). CLUMPP: a cluster matching and permutation program for dealing with label switching and multimodality in analysis of population structure. Bioinformatics 23, 1801–1806. doi: 10.1093/bioinformatics/btm233
Kang, H. M., Sul, J. H., Service, S. K., Zaitlen, N. A., Kong, S., Freimer, N. B., et al. (2010). Variance component model to account for sample structure in genome-wide association studies. Nat. Genet. 42, 348–354. doi: 10.1038/ng.548
King, R. A., Harris, S. L., Karp, A., and Barker, J. H. A. (2010). Characterisation and inheritance of nuclear microsatellite loci for use in population studies of the allotetraploid Salix alba-Salix fragilis complex. Tree Genet. Genomes 6, 247–258. doi: 10.1007/s11295-009-0245-6
Kong, Q., Yang, Y. Z., Guo, L., Yuan, L., and Ma, W. (2020). Molecular basis of plant oil biosynthesis: insights gained from studying the WRINKLED1 transcription factor. Front. Plant Sci. 11:24. doi: 10.3389/fpls.2020.00024
Kulheim, C., Yeoh, S. H., Maintz, J., Foley, W. J., and Moran, G. F. (2009). Comparative SNP diversity among four eucalyptus species for genes from secondary metabolite biosynthetic pathways. BMC Genomics 10:452. doi: 10.1186/1471-2164-10-452
Kumar, S., Stecher, G., Li, M., Knyaz, C., and Tamura, K. (2018). MEGA X: molecular evolutionary genetics analysis across computing platforms. Mol. Biol. Evol. 35, 1547–1549. doi: 10.1093/molbev/msy096
Li, S. F., Allen, P. J., Napoli, R. S., Browne, R. G., Pham, H., and Parish, R. W. (2020). MYB-bHLH-TTG1 regulates Arabidopsis seed coat biosynthesis pathways directly and indirectly via multiple tiers of transcription factors. Plant Cell Physiol. 61, 1005–1018. doi: 10.1093/pcp/pcaa027
Li, S. F., Milliken, O. N., Pham, H., Seyit, R., Napoli, R., Preston, J., et al. (2009). The Arabidopsis MYB5 transcription factor regulates mucilage synthesis, seed coat development, and trichome morphogenesis. Plant Cell 21, 72–89. doi: 10.1105/tpc.108.063503
Li, S. S., Wang, L. S., Shu, Q. Y., Wu, J., Chen, L. G., Shao, S., et al. (2015a). Fatty acid composition of developing tree peony (Paeonia section Moutan DC.) seeds and transcriptome analysis during seed development. BMC Genomics 16:208. doi: 10.1186/s12864-015-1429-0
Li, S. S., Yuan, R. Y., Chen, L. G., Wang, L. S., Hao, X. H., Wang, L. J., et al. (2015b). Systematic qualitative and quantitative assessment of fatty acids in the seeds of 60 tree peony (Paeonia section Moutan DC.) cultivars by GC-MS. Food Chem. 173, 133–140. doi: 10.1016/j.foodchem.2014.10.017
Li, Y. H., Zheng, L. Y., Corke, F., Smith, C., and Bevan, M. W. (2008). Control of final seed and organ size by the DA1 gene family in Arabidopsis thaliana. Genes Dev. 22, 1331–1336. doi: 10.1101/gad.463608
Liljegren, S. J., Ditta, G. S., Eshed, Y., Savidge, B., Bowman, J. L., and Yanofsky, M. F. (2000). SHATTERPROOF MADS-box genes control seed dispersal in Arabidopsis. Nature 404, 766–770. doi: 10.1038/35008089
Lira, B. S., Gramegna, G., Trench, B. A., Alves, F. R. R., Silva, E. M., Silva, G. F. F., et al. (2017). Manipulation of a senescence-associated gene improves fleshy fruit yield. Plant Physiol. 175, 77–91. doi: 10.1104/pp.17.00452
Liu, N., and Cheng, F. Y. (2020a). Association mapping for yield traits in Paeonia rockii based on SSR markers within transcription factors of comparative transcriptome. BMC Plant Biol. 20:245. doi: 10.1186/s12870-020-02449-6
Liu, N., and Cheng, F. Y. (2020b). Variation and yield grading standards of fruit traits in Paeonia rockii (S. G. Haw et L. A. Lauener) T. Hong et J. J. Li. Plant Sci. J. 38, 378–389. doi: 10.11913/PSJ.2095-0837.2020.30378
Neale, D. B., and Savolainen, O. (2004). Association genetics of complex traits in conifers. Trends Plant Sci. 9, 325–330. doi: 10.1016/j.tplants.2004.05.006
Newman, D. L., Abney, M., McPeak, M. S., Ober, C., and Cox, N. J. (2001). The importance of genealogy in determining genetic associations with complex traits. Am. J. Hum. Genet. 69, 1146–1148. doi: 10.1086/323659
Pang, L. Z., Cheng, F. Y., Zhong, Y., Cai, C. F., and Cui, H. L. (2012). Phenotypic analysis of association population for flare tree peony. J. Beijing For. Univer. 34, 115–120.
Peakall, R., and Smouse, P. E. (2012). GenAlEx 6.5: genetic analysis in excel. Population genetic software for teaching and research-an update. Bioinformatics 28, 2537–2539. doi: 10.1093/bioinformatics/bts460
Porth, I., Klapste, J., Skyba, O., Hannemann, J., McKown, A. D., Guy, R. D., et al. (2013). Genome-wide association mapping for wood characteristics in Populus identifies an array of candidate single nucleotide polymorphisms. New Phytol. 200, 710–726. doi: 10.1111/nph.12422
Pritchard, J. K., Stephens, M., and Donnelly, P. (2000). Inference of population structure using multilocus genotype data. Genetics 155, 945–959. doi: 10.1093/genetics/155.2.945
Renard, J., Niñoles, R., Martínez Almonacid, I., Gayubas, B., Mateos Fernández, R., Bissoli, G., et al. (2020). Identification of novel seed longevity genes related to oxidative stress and seed coat by genome-wide association studies and reverse genetics. Plant Cell Environ. 43, 2523–2539. doi: 10.1111/pce.13822
Resende, R. T., Resende, M. D., Silva, F. F., Azevedo, C. F., Takahashi, E. K., Silva-Junior, O. B., et al. (2017). Regional heritability mapping and genome-wide association identify loci for complex growth, wood and disease resistance traits in eucalyptus. New Phytol. 213, 1287–1300. doi: 10.1111/nph.14266
Rombel, I. T., Sykes, K. F., Rayner, S., and Johnston, S. A. (2002). ORF-FINDER: a vector for high-throughput gene identification. Gene 282, 33–41. doi: 10.1016/S0378-1119(01)00819-8
Rosenberg, N. A. (2004). Distruct: a program for the graphical display of population structure. Mol. Ecol. Notes 4, 137–138. doi: 10.1046/j.1471-8286.2003.00566.x
Rousset, F. (2008). Genepop’ 007: a complete re-implementation of the Genepop software for windows and Linux. Mol. Ecol. Resour. 8, 103–106. doi: 10.1111/j.1471-8286.2007.01931.x
Storey, J. D., and Tibshirani, R. (2003). Statistical significance for genome-wide experiments. Proc. Natl. Acad. Sci. U. S. A. 100, 9440–9445. doi: 10.1073/pnas.1530509100
Su, Z. Q., Hao, C. Y., Wang, L. F., Dong, Y. C., and Zhang, X. Y. (2011). Identification and development of a functional marker of TaGW2 associated with grain weight in bread wheat (Triticum aestivum L.). Theor. Appl. Genet. 122, 211–223. doi: 10.1007/s00122-010-1437-z
Sul, J. H., Martin, L. S., and Eskin, E. (2018). Population structure in genetic studies: confounding factors and mixed models. PLoS Genet. 14:e1007309. doi: 10.1371/journal.pgen.1007309
Sun, X. Y., Du, Z. M., Ren, J., Amombo, E., Hu, T., and Fu, J. M. (2015). Association of SSR markers with functional traits from heat stress in diverse tall fescue accessions. BMC Plant Biol. 15:116. doi: 10.1186/s12870-015-0494-5
Taylor-Teeples, M., Lin, L., de Lucas, M., Turco, G., Toal, T. W., Gaudinier, A., et al. (2015). An Arabidopsis gene regulatory network for secondary cell wall synthesis. Nature 517, 571–575. doi: 10.1038/nature14099
Thudi, M., Upadhyaya, H. D., Rathore, A., Mal Gaur, P., Krishnamurthy, L., Roorkiwal, M., et al. (2014). Genetic dissection of drought and heat tolerance in chickpea through genome-wide and candidate gene-based association mapping approaches. PLoS One 9:e96758. doi: 10.1371/journal.pone.0096758
Thumma, B. R., Nolan, M. F., Evans, R., and Moran, G. F. (2005). Polymorphisms in Cinnamoyl CoA reductase (CCR) are associated with variation in microfibril angle in eucalyptus spp. Genetics 171, 1257–1265. doi: 10.1534/genetics.105.042028
Tilman, D., Cassman, K. G., Matson, P. A., Naylor, R., and Polasky, S. (2002). Agricultural sustainability and intensive production practices. Nature 418, 671–677. doi: 10.1038/nature01014
Van Oosterhout, C., Hutchinson, W. F., Wills, D. P. M., and Shipley, P. (2004). Micro-checker: software for identifying and correcting genotyping errors in microsatellite data. Mol. Ecol. Notes 4, 535–538. doi: 10.1111/j.1471-8286.2004.00684.x
Varshney, R. K., Graner, A., and Sorrells, M. E. (2005). Genic microsatellite markers in plants: features and applications. Trends Biotechnol. 23, 48–55. doi: 10.1016/j.tibtech.2004.11.005
Wang, X. W., Fan, H. M., Li, Y. Y., Sun, X., Sun, X. Z., Wang, W. L., et al. (2014). Analysis of genetic relationships in tree peony of different colors using conserved DNA-derived polymorphism markers. Sci. Hortic. 175, 68–73. doi: 10.1016/j.scienta.2014.05.026
Wang, X. Q., Li, C. H., Contreras, M. D. M., Verardo, V., Gómez-Caravaca, A. M., and Xing, C. (2020). Integrated profiling of fatty acids, sterols and phenolic compounds in tree and herbaceous peony seed oils: marker screening for new resources of vegetable oil. Foods 9:770. doi: 10.3390/foods9060770
Wu, J., Cai, C. F., Cheng, F. Y., Cui, H. L., and Zhou, H. (2014). Characterisation and development of EST-SSR markers in tree peony using transcriptome sequences. Mol. Breed. 34, 1853–1866. doi: 10.1007/s11032-014-0144-x
Wu, J., Cheng, F. Y., Cai, C. F., Zhong, Y., and Jie, X. (2017). Association mapping for floral traits in cultivated Paeonia rockii based on SSR markers. Mol. Gen. Genomics. 292, 187–200. doi: 10.1007/s00438-016-1266-0
You, X. H., Li, W., Tao, Q. C., Sun, X. F., and Tang, K. X. (2011). At1g65030, a WD40-repeat protein gene, regulates seed mass and size in Arabidopsis. Plant Physiol. J. 47, 715–725. doi: 10.13592/j.cnki.ppj.2011.07.013
Yu, H. P., Cheng, F. Y., Zhong, Y., Cai, C. F., Wu, J., and Cui, H. L. (2013). Development of simple sequence repeat (SSR) markers from Paeonia ostii to study the genetic relationships among tree peonies (Paeoniaceae). Sci. Hortic. 164, 58–64. doi: 10.1016/j.scienta.2013.06.043
Yu, J., Pressoir, G., Briggs, W. H., Vroh, B. I., Yamasaki, M., Doebley, J. F., et al. (2006). A unified mixed-model method for association mapping that accounts for multiple levels of relatedness. Nat. Genet. 38, 203–208. doi: 10.1038/ng1702
Yuan, J. H., Cheng, F. Y., and Zhou, S. L. (2012). Genetic structure of the tree peony (Paeonia rockii) and the Qinling Mountains as a geographic barrier driving the fragmentation of a large population. PLoS One 7:e34955. doi: 10.1371/journal.pone.0034955
Yuan, J. H., Cornille, A., Giraud, T., Cheng, F. Y., and Hu, Y. H. (2014). Independent domestications of cultivated tree peonies from different wild peony species. Mol. Ecol. 23, 82–95. doi: 10.1111/mec.12567
Zhai, Z. Y., Keereetaweep, J., Liu, H., Feil, R., Lunn, J. E., and Shanklin, J. (2021). Expression of a bacterial trehalose-6-phosphate synthase otsA increases oil accumulation in plant seeds and vegetative tissues. Front. Plant Sci. 12:656962. doi: 10.3389/fpls.2021.656962
Zhang, L., Guo, D. L., Guo, L. L., Guo, Q., Wang, H. F., and Hou, X. G. (2019). Construction of a high-density genetic map and QTLs mapping with GBS from the interspecific F1 population of P. ostii ‘Fengdan Bai’ and P. suffruticosa ‘Xin Riyuejin’. Sci. Hortic. 246, 190–200. doi: 10.1016/j.scienta.2018.10.039
Zhang, Q. Y., Niu, L. X., Yu, R., Zhang, X. X., Bai, Z. Z., Duan, K., et al. (2017a). Cloning, characterization, and expression analysis of a gene encoding a putative lysophosphatidic acid acyltransferase from seeds of Paeonia rockii. Appl. Biochem. Biotechnol. 182, 721–741. doi: 10.1007/s12010-016-2357-4
Zhang, J. J., Shu, Q. Y., Liu, Z. A., Ren, H. X., Wang, L. S., and De Keyser, E. (2012). Two EST-derived marker systems for cultivar identification in tree peony. Plant Cell Rep. 31, 299–310. doi: 10.1007/s00299-011-1164-1
Zhang, Q. Y., Yu, R., Sun, D. Y., Bai, Z. Z., Li, H., Xue, L., et al. (2017b). PrLPAAT4, a putative lysophosphatidic acid acyltransferase from Paeonia rockii, plays an important role in seed fatty acid biosynthesis. Molecules 22:1694. doi: 10.3390/molecules22101694
Zhou, H., Cheng, F. Y., Wu, J., and He, C. Y. (2015). Isolation and functional analysis of flowering locus T in tree peonies (PsFT). J. Am. Soc. Hortic. Sci. 140, 265–271. doi: 10.21273/JASHS.140.3.265
Zhou, X., Jiang, Y. J., and Yu, D. Q. (2011). WRKY22 transcription factor mediates dark-induced leaf senescence in Arabidopsis. Mol. Cells 31, 303–313. doi: 10.1007/s10059-011-0047-1
Keywords: flare tree peony (P. rockii), SSR markers, floral and fruit traits, association mapping, linkage disequilibrium
Citation: Guo X, He C, Cheng F, Zhong Y, Cheng X and Tao X (2021) Dissection of Allelic Variation Underlying Floral and Fruit Traits in Flare Tree Peony (Paeonia rockii) Using Association Mapping. Front. Genet. 12:664814. doi: 10.3389/fgene.2021.664814
Edited by:
Shang-Qian Xie, Hainan University, ChinaReviewed by:
Guoan Shi, Henan University of Science and Technology, ChinaRakesh Chahota, Chaudhary Sarwan Kumar Himachal Pradesh Krishi Vishvavidyalaya, India
Copyright © 2021 Guo, He, Cheng, Zhong, Cheng and Tao. This is an open-access article distributed under the terms of the Creative Commons Attribution License (CC BY). The use, distribution or reproduction in other forums is permitted, provided the original author(s) and the copyright owner(s) are credited and that the original publication in this journal is cited, in accordance with accepted academic practice. No use, distribution or reproduction is permitted which does not comply with these terms.
*Correspondence: Fangyun Cheng, Y2hlbmdmeThAMjYzLm5ldA==, orcid.org/0000-0002-3928-5731