- 1College of Animal Science and National Engineering Research Center for Breeding Swine Industry, South China Agricultural University, Guangdong, China
- 2College of Animal Science and Technology, Zhongkai University of Agriculture and Engineering, Guangdong, China
The Duroc × (Landrace × Yorkshire) hybrid pigs (DLY) are the most popular commercial pigs, providing consumers with the largest source of pork. In order to gain more insights into the genetic architecture of economically important traits in pigs, we performed a genome-wide association study (GWAS) using the GeneSeek Porcine 50 K SNP Chip to map the genetic markers and genes associated with body conformation traits (BCT) in 311 DLY pigs. The quantitative traits analyzed included body weight (BW), carcass length (CL), body length (BL), body height (BH), and body mass index (BMI). BMI was defined as BMICL, BMIBL, and BMIBH, respectively, based on CL, BL, and BH phenotypic data. We identified 82 SNPs for the seven traits by GEMMA-based and FarmCPU-based GWASs. Both methods detected two quantitative trait loci (QTL) on SSC8 and SSC17 for body conformation traits. Several candidate genes (such as TNFAIP3, KDM4C, HSPG2, BMP2, PLCB4, and GRM5) were found to be associated with body weight and body conformation traits in pigs. Notably, the BMP2 gene had pleiotropic effects on CL, BL, BH, BMICL, and BMIBL and is proposed as a strong candidate gene for body size due to its involvement in growth and bone development. Furthermore, gene set enrichment analysis indicated that most of the pathway terms are associated with regulation of cell growth, negative regulation of cell population proliferation, and chondrocyte differentiation. We anticipate that these results further advance our understanding of the genetic architecture of body conformation traits in the popular commercial DLY pigs and provide new insights into the genetic architecture of BMI in pigs.
Introduction
In recent decades, pork has made up a large share of total worldwide meat production to accommodate growing human consumption. Growth and body conformation traits (such as body height and length) are economic traits which are moderately to highly important in pig production. It may be of interest to consider these traits in pig breeding schemes. Body height (BH) and body length (BL) are associated with meat production and were typical polygenic quantitative traits. Several studies have revealed significant single-nucleotide polymorphisms (SNPs) associated with BH or BL using the genome-wide association study (GWAS). For instance, Fan et al. (2009) (Fan et al., 2009) showed that COL9A1, APOE, CART, INSL3, and DKFZ were significantly associated with BL. Soma et al. (2011) (Soma et al., 2011) identified that four QTLs respectively on SSC4, SSC8, SSC13, and SSC14 were significantly associated with BL. Zhou et al. (2016) showed that ss131324074 on SSC7 and ss107849935 on SSC9 were significantly associated with BH, and ss131389597 on SSC9 and ss478942250 on SSC10 were significantly associated with BL in a Chinese Laiwu pig population (Zhou et al., 2016). An SNP (EU169095: g.40395T > G) within the PPARδ gene was found to be associated with the carcass length in a Large White × Meishan resource pig population (Xu et al., 2013). In addition, the number of thoracolumbar vertebrae can affect carcass length, which is an economically important trait in pig production. Rohrer and Keele (1998) (Rohrer and Keele, 1998) and Wada et al. (2000) (Wada et al., 2000) reported QTL for carcass length and vertebra number on the corresponding region of SSC 1. Mikawa et al. (2011) (Mikawa et al., 2011) proved that VRTN is the suspected cause of the heterogeneity of the number of vertebrae in commercial-breed pigs. Although previous findings have provided a certain number of molecular markers to help elucidate the genetic basis of swine body conformation traits, inadequacies and challenges remain when elucidating the biological mechanisms of the complex traits.
With the aid of high-density SNPs across the porcine genome, genome-wide association studies (GWASs) were utilized to dissect quantitative trait loci (QTLs) and genes associated with body conformation traits in pigs. The GWAS based on the mixed linear model (MLM) is the most popular method by taking account of population structure and genetic relatedness in deciphering the genetic architecture of complex traits in livestock (Sanchez et al., 2014). Multiple algorithms have been developed to boost both the computational efficiency and the statistical power of MLM methods (Kang et al., 2008; Zhou and Stephens, 2012). A recently developed GWAS model, named fixed and random model Circulating Probability Unification (FarmCPU) (Liu et al., 2016), has been widely used for detecting QTLs for economically important traits (Zhou J. et al., 2019; Tang et al., 2019; Wang et al., 2019). FarmCPU splits the MLM into separated fixed-effect and random-effect models and iteratively uses the two models to remove confounding, prevents model overfitting, and controls false positives simultaneously for an efficient computation (Liu et al., 2016). The major feature of FarmCPU is to correct for the effects of other markers by incorporating multiple markers simultaneously as covariates.
The Duroc × (Landrace × Yorkshire) hybrid pigs (DLY) are the most popular commercial pigs used in the Chinese pig industry. In this study, we performed GEMMA-based and FarmCPU-based GWASs for the body conformation traits including carcass length (CL), BL, and BH in 311 DLY pigs to identify the significant SNPs and then compare the SNP set respectively detected by the two methods. In addition, pigs provide a good animal model for studying the genetic basis of human disease due to their numerous physiological and phenotypic similarities with humans, including obesity. In human, the body mass index (BMI) is often used as an indicator to assess obesity (Locke et al., 2015), which is defined as a ratio of weight and height2 (Keys et al., 2014; Zhou et al., 2016). However, rare research has reported BMI differences in pigs (Zhou et al., 2016). Thus, we further analyzed the BMI in pigs and anticipated that this work will contribute to a better understanding of the genetic control of body conformation traits in agricultural animals and provide insights into the studies of human obesity and obesity-related diseases.
Materials and Methods
Experimental Animals and Phenotyping
Three-way crossbred DLY pigs intercrossed by Duroc boars and (Landrace × Yorkshire) sows were used in this study to conduct genetic analyses for body conformation traits. The experimental animals used in this study consisted of 311 DLY boars born in 2017 and were castrated at day 90 and raised in the same farm of Wen’s Foodstuffs Group Co., Ltd. (Guangdong, China). All pigs were fed with the same diets, raised under the same management conditions, and then slaughtered at 210 ± 3 days of age in a commercial abattoir in Shenzhen, Guangdong province. Three hundred eleven pigs were in the same batch. All the pigs were measured on the following traits: body weight (BW), body height (BH, from shoulder to ground), and body length (BL, from the midpoint of the ears to the tail head measured by a cloth tape). All the pigs were measured for BH and BL on the same flat ground before slaughter (Ma et al., 2009).
After skinning, scalding, scrapping, and eviscerating, carcass weight and carcass length (CL; measured from the first cervical vertebra to pubis) were measured within 30 min postmortem by the same person to minimize measurement errors. The ratio of weight and height2 shows relative independence from height or body length and high correlation with weight and fatness or subcutaneous fat (Keys et al., 2014; Zhou et al., 2016), as well as the abovementioned other weight/height ratios, such as weight/height, weight/height3, and height/weight1/3. The definition of human height seems to be different from the definition of pig height. In pigs, the measure of height should include the body length and hind leg length. Due to the differences in body structure between humans and pigs, a simple summation of body length and hind leg length seems inappropriate to be the “height” for BMI analysis in pigs. As described in previous studies, body length (Zhou et al., 2016) and body height (Gong et al., 2019) of pigs were treated as “height” to calculate the BMI (Supplementary Figure S1). In this study, in addition to BL and BH, we also added CL as the “height” of DLY to get the BMI value (respectively marked as BMICL, BMIBL, and BMIBH) for subsequent analyses for a comprehensive understanding of the similarities and differences of different “height.”
Genotyping and Quality Control
Genomic DNA was extracted from ear tissues using an animal tissue DNA extraction kit (Generay Biotech Co., Ltd., Shanghai, China) following the manufacturer’s protocol. DNA quality was detected using a NanoDrop ND-1000 (Peqlab Biotechnology) and agarose gel electrophoresis. The DNA concentration of the samples was adjusted to 50 ng/μl. Samples were genotyped with the GeneSeek Porcine 50 K SNP Chip (Neogen, Lincoln, NE, United States) (Ding et al., 2018). Quality control (QC) was carried out using PLINK v1.07 (Purcell et al., 2007) software. SNPs with call rates lower than 95%, ambiguous locations, and minor allele frequencies less than 0.01 were discarded. SNPs that failed the Hardy–Weinberg equilibrium test (p < 10–6) and unmapped or located on the sex chromosomes were also removed.
Population Structure and Linkage Disequilibrium
Principal component analysis (PCA) was performed using the SNP dataset to assess the potential population stratification prior to conducting the GWAS. PCA was performed with the Genome-wide Complex Trait Analysis (GCTA) software (Yang et al., 2011) and Genome Association and Prediction Integrated Tool (GAPIT) (Lipka et al., 2012), respectively. Moreover, PLINK v1.07 (Purcell et al., 2007) was used to calculate the average linkage disequilibrium (LD) decay distance across the genome of the DLY pig population.
Association Analyses
GEMMA-Based GWAS
The seven traits were analyzed using the same linear mixed model fitted in genome-wide efficient mixed-model analysis (GEMMA) software (Zhou and Stephens, 2012), one trait at a time. The statistical linear mixed model is described as follows:
where y is an n×1 vector of phenotypes in the DLY pig population; α is a vector of the corresponding parameters including the intercept, sex, body weight (not included for BW), and the top five eigenvectors obtained prior to this analysis using the GCTA software (Yang et al., 2011); W is the incidence matrix of the appropriate dimension for the fixed effects; β is the effect of the marker; x is an n×1 vector of marker genotypes; u ∼
FarmCPU-Based GWAS
The GAPIT (version 3.0) R package (Lipka et al., 2012) was used to conduct FarmCPU-based GWAS. All parameters were set as default. Briefly, the FarmCPU model consists of two parts: the fixed-effect model (FEM) and the random-effect model (REM), which is evaluated iteratively. The effects in the FEM include the top five principal components, sex, and pseudo quantitative trait nucleotides as (Liu et al., 2016; Tang et al., 2019), as follows:
where y is a vector of phenotypes of the analyzed trait;
The REM model updates the pseudo QTNs using the SUPER algorithm as follows (Wang et al., 2014):
where y is a vector of phenotypes,
Identification of Significant Single-Nucleotide Polymorphisms Associated With body Conformations
Significant SNPs were identified for each trait as those that surpassed the threshold with a false discovery rate (FDR) controlled at 0.01 (Benjamini and Hochberg, 1995; Wang et al., 2017). Briefly, the threshold p-value was defined as follows:
where n represents the number of SNPs with p < 0.01 in the GEMMA-based GWAS results, ordered ascendingly by their effects, and m is the number of qualified SNPs. The phenotypic variance explained by each significant SNP was estimated by the GCTA software (Yang et al., 2011). The Haploview v4.2 software (Barrett et al., 2005) was used to evaluate the LD pattern in specific genomic regions and conducted haplotype block analysis. The quantile–quantile (Q-Q) plots were generated to assess the influence of potential population stratification on GWAS using the GenABEL package (Aulchenko et al., 2007) after the top five principal components were added in the GWAS model.
Identification of Candidate Genes and Functional Enrichment Analysis
Candidate genes were retrieved within 0.5 Mb on either side of the significant SNPs for the seven traits from the Ensembl genome database version 99 of the Sus scrofa genome (Sscrofa11.1, http://jan2020.archive.ensembl.org, as of Jun 30, 2021) using the “biomaRt” package. Gene set enrichment analyses were conducted with these genes in the Metascape database (Zhou Y. et al., 2019). The terms with p < 0.01 were highlighted to further explore pathways and biological processes in which the genes are involved.
Results
Single-Nucleotide Polymorphism Genotyping and Phenotypic Variation
After QC, 38,398 SNPs with genotypes on 311 DLY pigs were retained for subsequent analyses. The descriptive statistics of BW, CL, BH, BL, and BMI for the 311 pigs are listed in Table 1. The phenotypic correlation coefficient between BW and BL (r = 0.55) was higher than that between BW and BH (r = 0.36) or CL (r = 0.31). The index shows a high correlation between BL and BH or CL (r ≥ 0.53), but lower between BH and CL (r = 0.35) (Supplementary Table S1). The phenotypic correlation coefficient between any two of these three BMI traits was high (r ≥ 0.69) (Supplementary Table S2).
Population Structure and Linkage Disequilibrium
As shown in Supplementary Figure S2A, a slight genetic differentiation among the DLY pigs was observed, and the first five principal components were retrieved from gapit to reduce the influence of population stratification on the GWAS (Supplementary Figure S2B). All filtered SNPs were used to determine LD decay. At r2 = 0.1, the LD decay distance decreases to 700 kb in the DLY population (Supplementary Figure S2C).
Summary of GWASs Results for Body Conformation Traits
In total, 82 SNPs surpassing the threshold with an FDR controlled at 0.01 were identified by the two GWAS methods (Tables 2, 3, 4). Among them, there were seven SNPs significantly associated with BW, 15 SNPs with CL, 26 SNPs with BL, 14 SNPs with BH, 17 SNPs with BMICL, 22 SNPs with BMIBL, and 13 SNPs with BMIBH. There were 53 significant SNPs found by GEMMA-based GWAS, 76 significant SNPs by FarmCPU-based GWAS, and 15 significant SNPs identified by both methods. Moreover, genes within the 1-Mb region of these significant SNPs were functionally annotated (Supplementary Table S3). The QQ plots of GWAS results are shown in Supplementary Figure S3.
Body Weight
We identified seven SNPs that were significantly associated with body weight, located on chromosomes 1, 3, 6, and 10, respectively (Table 2). The GEMMA-based GWAS detected three of these SNPs, and the FarmCPU-based GWAS detected five of them (Figure 1A,B). Of them, four SNPs were located within the previously reported QTLs for BW (https://www.animalgenome.org/cgi-bin/QTLdb/SS/index/). ASGA0091894 is the only SNP detected by both methods, which is significantly associated with BW. There were three SNPs, ASGA0001774, ASGA0005703, and WU_10.2_6_135404715, which showed significant effects on body weight, which otherwise was not reported previously. The most significant SNP detected by MLM and FarmCPU was WU_10.2_1_306708221 (p = 3.40 × 10−5) on SSC1, and ASGA0091894 (p = 3.90 × 10−5) explained 5.32 and 8.5% of the phenotypic variance of BW, respectively.
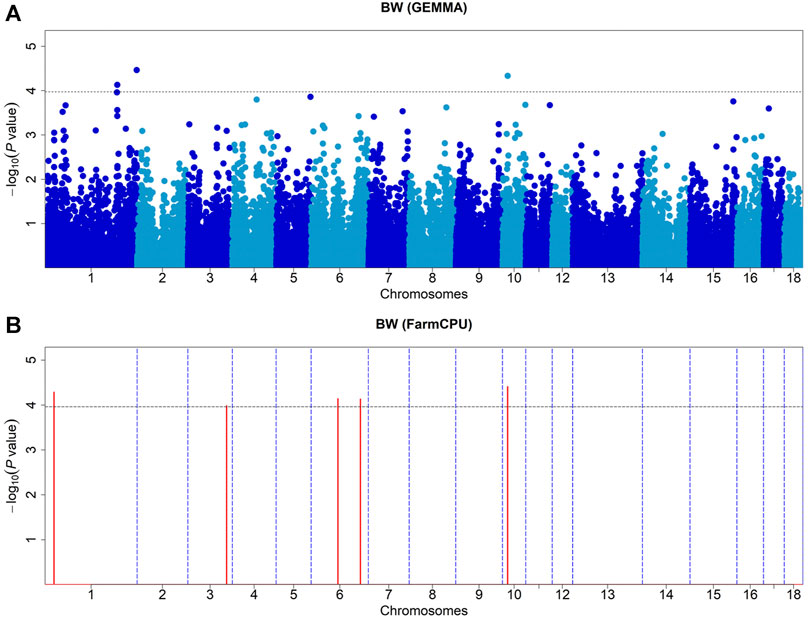
FIGURE 1. Manhattan plots of GEMMA-based GWAS (A) and FarmCPU-based GWAS (B) for BW in DLY pigs. The x-axis represents the chromosomes, and the y-axis represents the −log10 (p-value). The dashed lines indicate the thresholds for BW in DLY of GEMMA-based GWAS (p = 1.07E-04) and FarmCPU-based GWAS (p = 1.10E-04).
Carcass Length, Body Length, and Body Height
Fifteen significant SNPs were found for CL. MLM found seven significant SNPs, and FarmCPU detected nine SNPs. The WU_10.2_17_17981232 on SSC17 was the most significant SNP identified by GEMMA-based GWAS (p = 1.60 × 10−7) (Figure 2A) and also identified by FarmCPU GWAS which explained 9.74% of the phenotypic variance (Table 3). The WU_10.2_7_49907567 on SSC7 was the most significant SNP identified by FarmCPU GWAS (p = 1.71 × 10−8) (Figure 2B). For BL, the lead SNP was ALGA0123867 on SSC17, detected by the GEMMA-based GWAS (p = 7.91 × 10−8). Meanwhile, ALGA0123867 was the significant SNP detected by both methods. ALGA0056836 (p = 1.07 × 10−8) on SSC10 was found by FarmCPU (Figures 2C,D). For BH, there is a significant SNP, WU_10.2_17_17479009, on SSC17, which was detected by the two methods. It was also the most significant SNP detected by GEMMA-based GWAS (p = 9.23 × 10−8) and FarmCPU (p = 3.71 × 10−8) (Figures 2E,F). The SNPs for BH detected by GEMMA-based GWAS were mainly concentrated on chromosome 17. In contrast, except for one significant SNP, all other SNPs identified by FarmCPU were concentrated on other chromosomes outside SSC17. Among these SNPs, the most significant SNP for BH, namely, WU_10.2_17_17479009, was also significantly associated with CL and BL. Meanwhile, WU_10.2_17_17479009 had a similar effect on these three traits, including CL, BL, and BH. In addition, DBMA0000205 was significantly associated with CL and BL. WU_10.2_17_15712448 and ALGA0123867 were significantly associated with BL and BH.
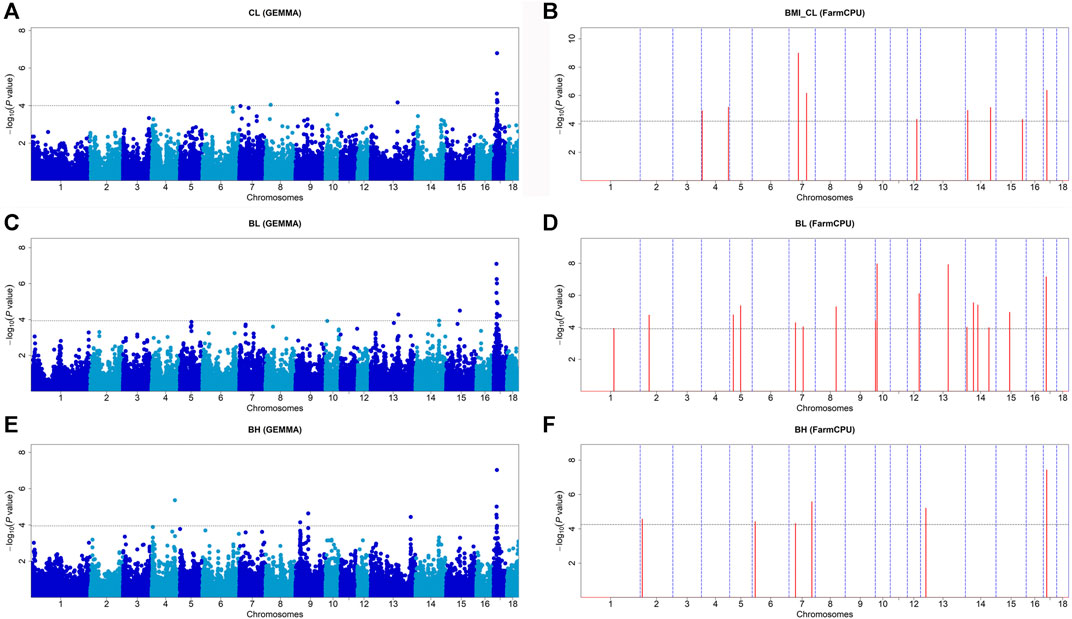
FIGURE 2. Manhattan plots of GEMMA-based GWAS and FarmCPU-based GWAS for CL, BL, and BH in DLY pigs. (A,B) represent the GWAS results conducted by GEMMA-based GWAS (threshold: p = 1.00E-04) and FarmCPU-based GWAS (threshold: p = 5.05E-05) for CL, respectively. (C,D) represent the GWAS results conducted by GEMMA-based GWAS (threshold: p = 1.17E-04) and FarmCPU-based GWAS (threshold: p = 1.22E-04) for BL, respectively. (E,F) represent the GWAS results conducted by GEMMA-based GWAS (threshold: p = 1.13E-04) and FarmCPU-based GWAS (threshold: p = 5.55E-05) for BH, respectively. The x-axis represents the chromosomes, and the y-axis represents the -log10 (p-value).
Body Mass Index
Seventeen SNPs were significantly associated with BMICL, 22 SNPs with BMIBL, and 13 SNPs with BMIBH by these two GWAS methods (Figure 3 and Table 4). GEMMA found 10 significant SNPs for BMICL, 8 significant SNPs for BMIBL, and 4 significant SNPs for BMIBH. Based on FarmCPU, there were 9 SNPs significantly associated with BMICL, 17 SNPs significantly associated with BMIBL, and 12 SNPs significantly associated with BMIBH. WU_10.2_17_17981232 (p = 1.42 × 10−8) on SSC17 and WU_10.2_7_49907567 (p = 1.02 × 10−9) on SSC7 were the most significant SNP identified by GEMMA-based GWAS and FarmCPU GWAS for BMICL (Figure 3A,B and Table 4), respectively. WU_10.2_17_17981232 was the only SNP detected by both GWAS methods for BMICL and explained 10.13% of the phenotypic variance (Table 4). For BMIBL, the most significant SNP detected by GEMMA-based GWAS was WU_10.2_17_15712448 (p = 1.30 × 10−6) and detected by FarmCPU GWAS was WU_10.2_13_138014916 (p = 2.45 × 10−9) (Figure 3C,D and Table 4). Among all significant SNPs for BMIBL, the explained phenotypic variation of WU_10.2_17_15712448 was the largest and up to 12.06%. The most significant SNP for BMIBH detected by two GWAS methods was ASGA0022193 (p = 2.00 × 10−6 from GEMMA-based GWAS; p = 5.34 × 10−7 from FarmCPU) (Figure 3E,F and Table 4). This site explained 7.49% of the phenotypic variance (Table 4).
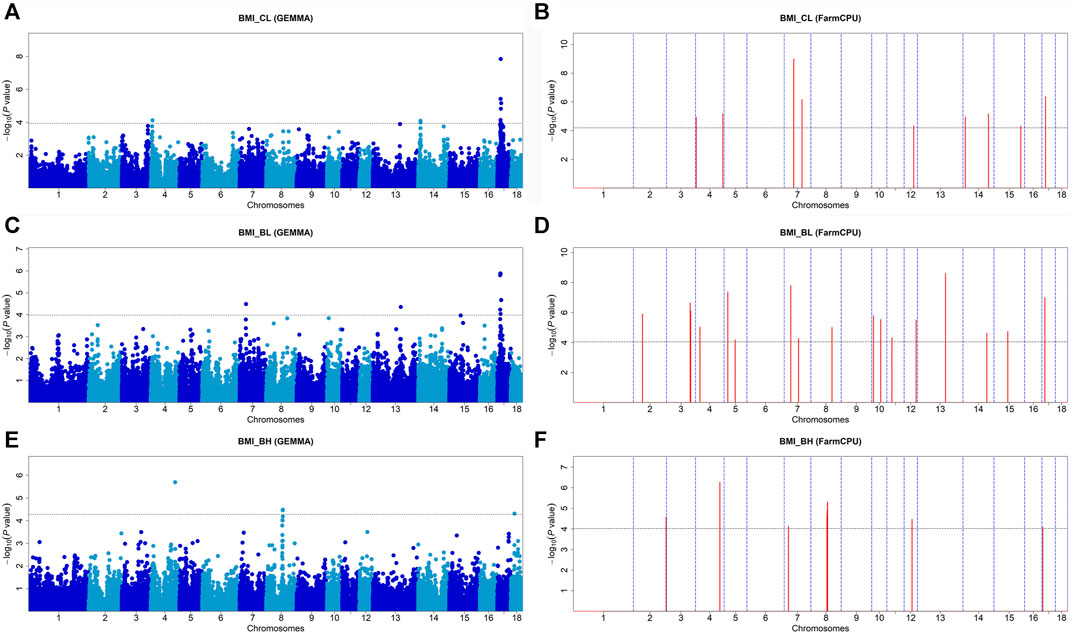
FIGURE 3. Manhattan plots of GEMMA-based GWAS and FarmCPU-based GWAS for BMICL, BMIBL, and BMIBH in DLY pigs. (A,B) represent the GWAS results conducted by GEMMA-based GWAS (threshold: p = 1.16E-04) and FarmCPU-based GWAS (threshold: p = 6.30E-05) for BMICL, respectively. (C,D) represent the GWAS results conducted by EMMA-based GWAS (threshold: p = 1.04E-04) and FarmCPU-based GWAS (threshold: p = 8.85E-05) for BMIBL, respectively. (E,F) represent the GWAS results conducted by EMMA-based GWAS (threshold: p = 5.31E-05) and FarmCPU-based GWAS (threshold: p = 9.58E-05) for BMIBH, respectively. The x-axis represents the chromosomes, and the y-axis represents the -log10 (p-value).
Linkage Disequilibrium Between Significant Single-Nucleotide Polymorphisms
In this study, the GWAS results showed that some QTL exhibited effects on more than one trait. For instance, WU_10.2_17_17479009 on SSC17 was significantly associated with CL, BL, BH, BMICL, and BMIBL, and WU_10.2_17_15712448 was significantly associated with BL, BH, and BMIBL. We further examined the LD pattern between SNPs in these QTL regions. Two LD block with r2 > 0.8 respectively distributed on SSC8 and 17 were found. The LD block on SSC8 was 15 kb, including two significant SNPs for BL and BMIBH, respectively (Figure 4A). On SSC17, the LD block was 263 kb, which contained one significant SNP for BMICL (Figure 4B). Interestingly, there are some SNPs on chromosome 17 that are significantly related to CL, BL, BH, and BMICL and on chromosome 8 for BMIBH, such as WU_10.2_8_80223477 in the LD block on SSC 8 and WU_10.2_17_17479009 adjacent to the LD block on SSC 17, whether having corrected or uncorrected the BW (Supplementary Table S4), which directly proves that these SNPs are less affected by body weight and have a greater impact on the inquired traits. It is worth noting that the most significant SNP for BH, WU_10.2_17_17479009, was adjacent to this block, which also significantly affected CL, BL, BH, BMICL, and BMIBL. Interestingly, the BMP2 gene, the nearest gene of WU_10.2_17_17479009, was also located in this 263-kb block. BMP2, which has a crucial role in chondrocyte proliferation and maturation, can be considered as one of the candidate genes for body length traits.
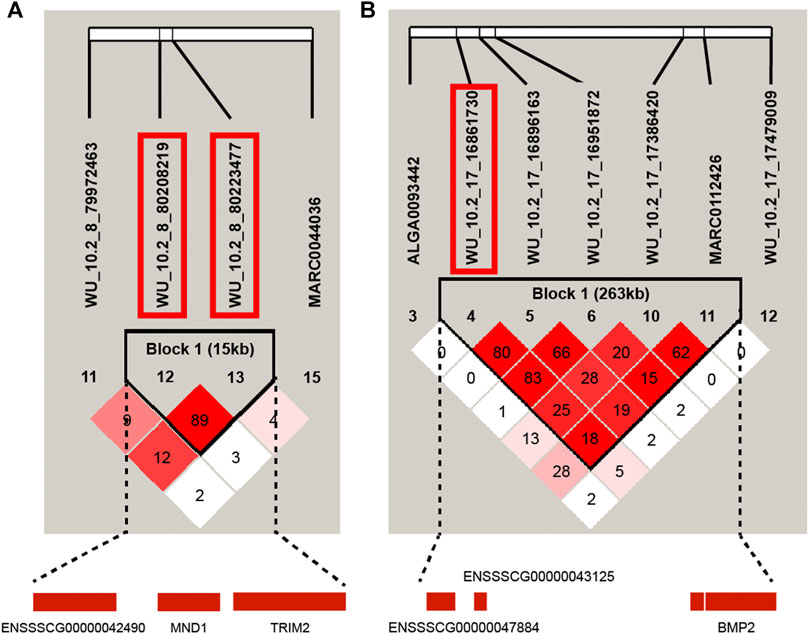
FIGURE 4. Haplotype blocks for significant SNPs indicate a haplotype block composed of significant SNPs located on SSC8 and SSC17. (A) represents the 15-kb LD block in the significant region on SSC8 with two significant SNPs (WU_10.2_8_80,208,219 and WU_10.2_8_80223477) for BMIBH. (B) is the haplotype block on SSC17 with two significant SNPs (WU_10.2_17_1,6,861,730 and WU_10.2_17_1,6,951,872) for BH.
Discussion
Genome-wide association studies provide an opportunity to dissect the genetic architecture of complex traits by leveraging LD between the causative mutations and common SNP markers in pigs (Zhuang et al., 2020). We performed two model-based GWASs on body conformation traits in a DLY pig population, detecting a set of trait-related SNPs, and then based on these SNPs and QTLs, candidate genes were annotated.
Genetic Loci and Genes for Body Weight
Body weight is a complex quantitative trait in domestic pigs and genomics, and molecular techniques can rapidly advance genetic improvement and increase production levels (Johnson and Nugent, 2003). In this study, four of the seven significant SNPs for BW corresponded to previously discovered QTL after assessment using PigQTLdb (https://www.animalgenome.org). The other three SNPs were newly found in the present study and two of them were identified by FarmCPU. According to two main criteria for listing the lead SNPs (lead signals) and candidate genes (secondary signals), the gene was the nearest to the index SNP, and the gene was found in the vicinity of the lead SNP and was biologically related to the trait (Berndt et al., 2013). We listed the candidate genes in the proximity of these significant SNPs for BW and checked their functions and involved pathways (Supplementary Table S3), all related to BW-relevant phenotypes such as growth, body size, digestive/alimentary system, and skeleton. For example, the CEL (Gilham et al., 2007) and MAP1LC3C (Patel et al., 2013) genes affecting the digestive/alimentary system of mice may affect the BW. The HSPG2 on SSC6 was involved in abnormal vertebral segmentation in human and animal models (Turnpenny et al., 2007) and presented different expressions between different genotype individuals at the VRTN QTNs, which are causal mutations for thoracic number variation of pig and wild-type pig embryos (Duan et al., 2018). Interestingly, the additional thoracic number will increase the body length and then improve pork production. This suggests that the HSPG2 gene may influence BW by affecting vertebral development.
Genetics Loci and Genes for Body Mass Index
Obesity, a growing worldwide health problem, is a complex trait. BMI was commonly employed to assess obesity in human disease study. In humans, BMI was determined by weight and height. In previous studies, BL and BH were used to represent “height” respectively in fat-type pigs, Chinese indigenous Laiwu and Bamaxiang pigs (Zhou et al., 2016; Gong et al., 2019). Nevertheless, limited to the physical structure determined by the pig’s walking style, simply adding the body length and limb height may cause a large error. Hence, we used CL, BL, and BH, respectively, as the “height” to calculate the pig’s BMI value. We found that all the QTLs identified in lean-type pigs, DLY, in this study were not reported previously in fat-type pigs. This suggests that the major effect loci of BMI trait of lean-type pigs and fat-type pigs are different. After comparing pig BMI loci and genes that are nearest to the index SNP of BMIs to these in human (https://www.ncbi.nlm.nih.gov/omim), there was no overlap between pig and human. Among all SNPs associated with these three BMI traits, only WU_10.2_17_17479009 and DBMA0000205 were significantly correlated with two or more traits simultaneously, and they had significant effects on both BMICL and BMIBL. The gene nearby WU_10.2_17_17479009 was bone morphogenetic protein 2 (BMP2), and the nearby gene of DBMA0000205 was phospholipase C Beta 4 (PLCB4). These two genes (BMP2 and PLCB4) were related to growth, body size, and skeleton. Moreover, PLCB4 also affected adipose tissue. Although the other SNPs are different, the function of genes nearest to the significant SNPs of three kinds of BMIs was similar. TGFBR3, BNIP3L, and PLCB4 were closest to the significant SNPs of BMICL. OTOG, ITPR3, ITPR3, and RASSF2 were nearest to significant SNPs of BMIBL. FRAS1, and FBXW7 were closest to significant SNPs of BMIBH. These genes all affect the adipose tissue, growth, body size, skeleton or nervous system (Ignatieva et al., 2016), and digestive/alimentary system of mice.
The BMI of a pig can be considered as meat yield ability in specific body length. The higher the BMI value, the stronger the pig’s potential meat yield for the pigs of the same age and under the same feeding environment. However, the BMIs of pigs at different ages are significantly different, and the corresponding significant SNPs were also different (Zhou et al., 2016). Considering that major genes affecting this trait may vary with the age of pigs, more genetic analyses of pig BMI are needed to discover BMI-related loci and their underlying mechanisms.
Genetic Loci and Genes for Carcass Length, Body Length, and Body Height
Among 53 significant SNPs that are associated with CL, BL, and BH traits based on two GWAS models, five SNPs (ALGA0123867, DBMA0000205, SIRI0000046, WU_10.2_17_15712448, and WU_10.2_17_17479009) were identified to be significantly associated with more than one trait. The candidate genes closest to these five SNPs were PRNP, PLCB4, ITPR3, SHLD1, and BMP2. These genes all influence skeletal growth and body size in mice. We further aggregated evidence from our data, human (https://www.ncbi.nlm.nih.gov/omim/) and mouse genetic databases (http://www.informatics.jax.org/), and proposed the candidate genes based on their functional relevance to traits. A number of genes, such as DAGLA, RASSF2, MMUT, PEBP4, NR4A2, FERMT1, SOCS6, TBX3, TGFBR3, TRAPPC9, UCHL5, VAC14, WNT2B, GRM5, ULK4, and SCN8A affect growth, body size, and skeleton in mice. Interestingly, among these genes, EGLN3, KSR1, and GRM5 were involved in the growth of adipose tissue. This hints that these genes may affect the two traits simultaneously or influence body length traits by changing the backfat thickness or conversely.
The most strongly associated variant was often located near the causal genes (Lango Allen et al., 2010). Nearly half of the significant SNPs for CL, BL, and BH traits were on SSC17. Among these SNPs, only WU_10.2_17_17479009 showed significant effects on multiple carcass traits, including CL, BL, and BH, even BMICL and BMIBL. Considering these facts, we postulate that one or more causative genes exist there that regulate the pathway involving the growth and carcass traits of pigs. The most likely candidate gene is BMP2, which was nearest to WU_10.2_17_17479009. Thus, gene encodes a secreted ligand of the TGF-beta superfamily of proteins, which involves transforming the growth factor-beta (TGF-beta) signaling pathway, playing a role in bone and cartilage development (Blaj et al., 2018). The BMP2 conditional knockout (cKO) mice showed smaller calvaria, thoracic cavities, and shorter spines and hind limbs than Cre-negative littermates (Shu et al., 2011). In humans, the heterozygous mutation in the BMP2 gene resulted in short stature, facial dysmorphism, and skeletal anomalies (Tan et al., 2017). Although there is no direct evidence in pigs suggesting BMP2 as a causal gene of pig body size traits, some studies have shown that some mutation sites in or near this gene were associated with body length, body depth, and body width (Fan et al., 2011). Then, we conducted a gene enrichment analysis using candidate genes including the closest genes and those within the 1-Mb region of significant SNPs for body conformation traits to have a comprehensive understanding of their signaling pathways involved in regulating body size (Supplementary Table S5). The top 20 GO-enriched terms included cell growth, negative regulation of cell population proliferation, and chondrocyte differentiation, which are closely related to body conformation. Of note, BMP2 was in two of these three pathways. This result provided further evidence that BMP2 was the most probable candidate gene for body conformation traits. Although further studies are needed to dissect the genetic architecture of body conformation traits, our findings have identified a number of novel loci pinpointing biologically relevant genes and pathways for body weights and conformations.
Conclusion
In this study, we identified 82 SNPs associated with seven body conformation traits in DLY pigs using GEMMA-based GWAS and FarmCPU-based GWAS. We then identified three genomic regions and several genes related to body conformation traits in pigs. WU_10.2_17_17479009 was the only SNP that affected more than three traits and showed pleiotropic effects on CL, BL, BH, BMICL, and BMIBL in pigs. Specifically, the BMP2 gene is proposed as a strong candidate gene for body size due to the effect on CL, BL, BH, BMICL, and BMIBL and is involved in growth and bone development. In addition, we expect that our results provide a comprehensive understanding of the BMI trait, which has not been studied adequately in pigs. Altogether, this study not only benefits the molecular breeding for body conformation-related traits of the DLY pig but also advances our knowledge of the poorly understood of genetic loci or genes controlling BMI in pigs.
Data Availability Statement
All genotypic data were deposited in Figshare https://doi.org/10.6084/m9.figshare.16692094.v1.
Ethics Statement
All samples were collected, and experimental procedures were used in this study according to the guidelines of the Animal Care and Use Committee of the South China Agricultural University (SCAU) (Guangzhou, People’s Republic of China). All animal experiments described in this study were approved by the Animal Care and Use Committee of the SCAU.
Author Contributions
ZW and JY conceived and designed the experiments. HZ, ZZ, MY, RD, JQ, SZ, TG, ZX, and EZ collected the samples and recorded the phenotypes. ZZ, RD, JQ, and EZ extracted the DNA for genotyping. HZ, ZZ, RD, SZ, TG, and ZX contributed to the visualization of data. ZZ, DR, and JQ analyzed the data. ZW and GC contributed the materials. HZ, ZZ, and JY wrote and revised the manuscript. All authors reviewed and approved the manuscript.
Funding
This study was supported by the Key-Area Research and Development Program of Guangdong Province (2018B020203002), the Local Innovative and Research Teams Project of Guangdong Province (2019BT02N630), the Natural Science Foundation of Guangdong Province (2018B030315007), the Pearl River S and T Nova Program of Guangzhou (201906010011), and The Basic and Applied Basic Research Foundation of Guangdong Province (2020A1515111103). The funders had no role in the study design, data collection and analysis, the decision to publish, and manuscript preparation.
Conflict of Interest
The authors declare that the research was conducted in the absence of any commercial or financial relationships that could be construed as a potential conflict of interest.
Publisher’s Note
All claims expressed in this article are solely those of the authors and do not necessarily represent those of their affiliated organizations, or those of the publisher, the editors and the reviewers. Any product that may be evaluated in this article, or claim that may be made by its manufacturer, is not guaranteed or endorsed by the publisher.
Supplementary Material
The Supplementary Material for this article can be found online at: https://www.frontiersin.org/articles/10.3389/fgene.2021.664343/full#supplementary-material
Abbreviations
BCT, body conformation traits; BMI, body mass index; BW, body weight; BL, body length; BH, body height; BMICL, body mass index (weight/height2, CL be height); BMIBL, body mass index (weight/height2, BL be height); BMIBH, body mass index (weight/height2, BH be height); CL, carcass length; GWAS, genome-wide association studies; SNP, single-nucleotide polymorphism.
References
Aulchenko, Y. S., Ripke, S., Isaacs, A., and van Duijn, C. M. (2007). GenABEL: an R Library for Genome-wide Association Analysis. Bioinformatics 23 (10), 1294–1296. doi:10.1093/bioinformatics/btm108
Barrett, J. C., Fry, B., Maller, J., and Daly, M. J. (2005). Haploview: Analysis and Visualization of LD and Haplotype Maps. Bioinformatics 21 (2), 263–265. doi:10.1093/bioinformatics/bth457
Benjamini, Y., and Hochberg, Y. (1995). Controlling the False Discovery Rate: A Practical and Powerful Approach to Multiple Testing. J. R. Stat. Soc. Ser. B (Methodological) 57 (1), 289–300. doi:10.1111/j.2517-6161.1995.tb02031.x
Berndt, S. I., Gustafsson, S., Mägi, R., Ganna, A., Wheeler, E., Feitosa, M. F., et al. (2013). Genome-wide Meta-Analysis Identifies 11 New Loci for Anthropometric Traits and Provides Insights into Genetic Architecture. Nat. Genet. 45 (5), 501–512. doi:10.1038/ng.2606
Blaj, I., Tetens, J., Preuß, S., Bennewitz, J., and Thaller, G. (2018). Genome-wide Association Studies and Meta-Analysis Uncovers New Candidate Genes for Growth and Carcass Traits in Pigs. PLoS One 13 (10), e0205576. doi:10.1371/journal.pone.0205576
Ding, R., Yang, M., Wang, X., Quan, J., Zhuang, Z., Zhou, S., et al. (2018). Genetic Architecture of Feeding Behavior and Feed Efficiency in a Duroc Pig Population. Front. Genet. 9, 220. doi:10.3389/fgene.2018.00220
Duan, Y., Zhang, H., Zhang, Z., Gao, J., Yang, J., Wu, Z., et al. (2018). VRTN Is Required for the Development of Thoracic Vertebrae in Mammals. Int. J. Biol. Sci. 14 (6), 667–681. doi:10.7150/ijbs.23815
Fan, B., Onteru, S. K., Du, Z. Q., Garrick, D. J., Stalder, K. J., and Rothschild, M. F. (2011). Genome-wide Association Study Identifies Loci for Body Composition and Structural Soundness Traits in Pigs. PLoS One 6 (2), e14726. doi:10.1371/journal.pone.0014726
Fan, B., Onteru, S. K., Mote, B. E., Serenius, T., Stalder, K. J., and Rothschild, M. F. (2009). Large-scale Association Study for Structural Soundness and Leg Locomotion Traits in the Pig. Genet. Sel. Evol. 41 (1), 14. doi:10.1186/1297-9686-41-14
Gilham, D., Labonté, E. D., Rojas, J. C., Jandacek, R. J., Howles, P. N., and Hui, D. Y. (2007). Carboxyl Ester Lipase Deficiency Exacerbates Dietary Lipid Absorption Abnormalities and Resistance to Diet-Induced Obesity in Pancreatic Triglyceride Lipase Knockout Mice. J. Biol. Chem. 282 (34), 24642–24649. doi:10.1074/jbc.M702530200
Gong, H., Xiao, S., Li, W., Huang, T., Huang, X., Yan, G., et al. (2019). Unravelling the Genetic Loci for Growth and Carcass Traits in Chinese Bamaxiang Pigs Based on a 1.4 Million SNP Array. J. Anim. Breed. Genet. 136 (1), 3–14. doi:10.1111/jbg.12365
Ignatieva, E. V., Afonnikov, D. A., Saik, O. V., Rogaev, E. I., and Kolchanov, N. A. (2016). A Compendium of Human Genes Regulating Feeding Behavior and Body Weight, its Functional Characterization and Identification of GWAS Genes Involved in Brain-specific PPI Network. BMC Genet. 17 (Suppl. 3), 158. doi:10.1186/s12863-016-0466-2
Johnson, Z. B., and Nugent, R. A. (2003). Heritability of Body Length and Measures of Body Density and Their Relationship to Backfat Thickness and Loin Muscle Area in Swine. J. Anim. Sci. 81 (8), 1943–1949. doi:10.2527/2003.8181943x
Kang, H. M., Zaitlen, N. A., Wade, C. M., Kirby, A., Heckerman, D., Daly, M. J., et al. (2008). Efficient Control of Population Structure in Model Organism Association Mapping. Genetics 178 (3), 1709–1723. doi:10.1534/genetics.107.080101
Keys, A., Fidanza, F., Karvonen, M. J., Kimura, N., and Taylor, H. L. (2014). Indices of Relative Weight and Obesity. Int. J. Epidemiol. 43 (3), 655–665. doi:10.1093/ije/dyu058
Lango Allen, H., Estrada, K., Lettre, G., Berndt, S. I., Weedon, M. N., Rivadeneira, F., et al. (2010). Hundreds of Variants Clustered in Genomic Loci and Biological Pathways Affect Human Height. Nature 467 (7317), 832–838. doi:10.1038/nature09410
Lipka, A. E., Tian, F., Wang, Q., Peiffer, J., Li, M., Bradbury, P. J., et al. (2012). GAPIT: Genome Association and Prediction Integrated Tool. Bioinformatics 28 (18), 2397–2399. doi:10.1093/bioinformatics/bts444
Liu, X., Huang, M., Fan, B., Buckler, E. S., and Zhang, Z. (2016). Iterative Usage of Fixed and Random Effect Models for Powerful and Efficient Genome-wide Association Studies. Plos Genet. 12 (2), e1005767. doi:10.1371/journal.pgen.1005767
Locke, A. E., Kahali, B., Berndt, S. I., Justice, A. E., Pers, T. H., Day, F. R., et al. (2015). Genetic Studies of Body Mass index Yield New Insights for Obesity Biology. Nature 518 (7538), 197–206. doi:10.1038/nature14177
Mikawa, S., Sato, S., Nii, M., Morozumi, T., Yoshioka, T., Imaeda, N., et al. (2011). Identification of a Second Gene Associated with Variation in Vertebral Number in Domestic Pigs. BMC Genet. 12, 5. doi:10.1186/1471-2156-12-5
Patel, K. K., Miyoshi, H., Beatty, W. L., Head, R. D., Malvin, N. P., Cadwell, K., et al. (2013). Autophagy Proteins Control Goblet Cell Function by Potentiating Reactive Oxygen Species Production. Embo j 32 (24), 3130–3144. doi:10.1038/emboj.2013.233
Purcell, S., Neale, B., Todd-Brown, K., Thomas, L., Ferreira, M. A., Bender, D., et al. (2007). PLINK: a Tool Set for Whole-Genome Association and Population-Based Linkage Analyses. Am. J. Hum. Genet. 81 (3), 559–575. doi:10.1086/519795
Rohrer, G. A., and Keele, J. W. (1998). Identification of Quantitative Trait Loci Affecting Carcass Composition in Swine: I. Fat Deposition Traits. J. Anim. Sci. 76 (9), 2247–2254. doi:10.2527/1998.7692247x
Sanchez, M. P., Tribout, T., Iannuccelli, N., Bouffaud, M., Servin, B., Tenghe, A., et al. (2014). A Genome-wide Association Study of Production Traits in a Commercial Population of Large White Pigs: Evidence of Haplotypes Affecting Meat Quality. Genet. Sel. Evol. 46 (1), 12. doi:10.1186/1297-9686-46-12
Shu, B., Zhang, M., Xie, R., Wang, M., Jin, H., Hou, W., et al. (2011). BMP2, but Not BMP4, Is Crucial for Chondrocyte Proliferation and Maturation during Endochondral Bone Development. J. Cell Sci. 124 (Pt 20), 3428–3440. doi:10.1242/jcs.083659
Soma, Y., Uemoto, Y., Sato, S., Shibata, T., Kadowaki, H., Kobayashi, E., et al. (2011). Genome-wide Mapping and Identification of New Quantitative Trait Loci Affecting Meat Production, Meat Quality, and Carcass Traits within a Duroc Purebred Population. J. Anim. Sci. 89 (3), 601–608. doi:10.2527/jas.2010-3119
Tan, T. Y., Gonzaga-Jauregui, C., Bhoj, E. J., Strauss, K. A., Brigatti, K., Puffenberger, E., et al. (2017). Monoallelic BMP2 Variants Predicted to Result in Haploinsufficiency Cause Craniofacial, Skeletal, and Cardiac Features Overlapping Those of 20p12 Deletions. Am. J. Hum. Genet. 101 (6), 985–994. doi:10.1016/j.ajhg.2017.10.006
Tang, Z., Xu, J., Yin, L., Yin, D., Zhu, M., Yu, M., et al. (2019). Genome-Wide Association Study Reveals Candidate Genes for Growth Relevant Traits in Pigs. Front. Genet. 10, 1. doi:10.3389/fgene.2019.00302
Turnpenny, P. D., Alman, B., Cornier, A. S., Giampietro, P. F., Offiah, A., Tassy, O., et al. (2007). Abnormal Vertebral Segmentation and the Notch Signaling Pathway in Man. Dev. Dyn. 236 (6), 1456–1474. doi:10.1002/dvdy.21182
Wada, Y., Akita, T., Awata, T., Furukawa, T., Sugai, N., Inage, Y., et al. (2000). Quantitative Trait Loci (QTL) Analysis in a Meishan X Gottingen Cross Population [J]. Anim. Genet. 31 (6), 376–384. doi:10.1046/j.1365-2052.2000.00696.x
Wang, Q., Tian, F., Pan, Y., Buckler, E. S., and Zhang, Z. (2014). A SUPER Powerful Method for Genome Wide Association Study. PLoS One 9 (9), e107684. doi:10.1371/journal.pone.0107684
Wang, Y., Ding, X., Tan, Z., Ning, C., Xing, K., Yang, T., et al. (2017). Genome-Wide Association Study of Piglet Uniformity and Farrowing Interval. Front. Genet. 8, 194. doi:10.3389/fgene.2017.00194
Wang, Z., Zhu, B., Niu, H., Zhang, W., Xu, L., Xu, L., et al. (2019). Genome Wide Association Study Identifies SNPs Associated with Fatty Acid Composition in Chinese Wagyu Cattle. J. Anim. Sci. Biotechnol. 10, 27. doi:10.1186/s40104-019-0322-0
Xu, Y., Huang, W., Xiong, Y., and Zuo, B. (2013). Significant Association of One Intronic SNP within PPARδ Gene with Carcass Length Hi Pigs. J. Anim. Vet. Adv. 12, 439–441. doi:10.1016/b978-1-4160-3969-3.00198-0
Yang, J., Lee, S. H., Goddard, M. E., and Visscher, P. M. (2011). GCTA: a Tool for Genome-wide Complex Trait Analysis. Am. J. Hum. Genet. 88 (1), 76–82. doi:10.1016/j.ajhg.2010.11.011
Zhou, J., Liu, L., Chen, C. J., Zhang, M., Lu, X., Zhang, Z., et al. (2019a). Genome-wide Association Study of Milk and Reproductive Traits in Dual-Purpose Xinjiang Brown Cattle. BMC Genomics 20 (1). doi:10.1186/s12864-019-6224-x
Zhou, L., Ji, J., Peng, S., Zhang, Z., Fang, S., Li, L., et al. (2016). A GWA Study Reveals Genetic Loci for Body Conformation Traits in Chinese Laiwu Pigs and its Implications for Human BMI. Mamm. Genome 27 (11-12), 610–621. doi:10.1007/s00335-016-9657-4
Zhou, X., and Stephens, M. (2012). Genome-wide Efficient Mixed-Model Analysis for Association Studies. Nat. Genet. 44 (7), 821–824. doi:10.1038/ng.2310
Zhou, Y., Zhou, B., Pache, L., Chang, M., Khodabakhshi, A. H., Tanaseichuk, O., et al. (2019b). Metascape Provides a Biologist-Oriented Resource for the Analysis of Systems-Level Datasets. Nat. Commun. 10 (1), 1523. doi:10.1038/s41467-019-09234-6
Keywords: body conformation, carcass, crossbred pigs, GWAS, SNP
Citation: Zhang H, Zhuang Z, Yang M, Ding R, Quan J, Zhou S, Gu T, Xu Z, Zheng E, Cai G, Yang J and Wu Z (2021) Genome-Wide Detection of Genetic Loci and Candidate Genes for Body Conformation Traits in Duroc × Landrace × Yorkshire Crossbred Pigs. Front. Genet. 12:664343. doi: 10.3389/fgene.2021.664343
Received: 05 February 2021; Accepted: 16 September 2021;
Published: 11 October 2021.
Edited by:
Denis Milan, Institut National de recherche pour l’agriculture, l’alimentation et l’environnement (INRAE), FranceReviewed by:
Junwu Ma, State Key Laboratory for Pig Genetic Improvement and Production Technology, Jiangxi Agricultural University, ChinaJean-Pierre Bidanel, INRA UMR1313 Genetique Animale et Biologie Integrative, France
Copyright © 2021 Zhang, Zhuang, Yang, Ding, Quan, Zhou, Gu, Xu, Zheng, Cai, Yang and Wu. This is an open-access article distributed under the terms of the Creative Commons Attribution License (CC BY). The use, distribution or reproduction in other forums is permitted, provided the original author(s) and the copyright owner(s) are credited and that the original publication in this journal is cited, in accordance with accepted academic practice. No use, distribution or reproduction is permitted which does not comply with these terms.
*Correspondence: Jie Yang, amlleWFuZzIwMTJAaG90bWFpbC5jb20=; Zhenfang Wu, d3pmZW1haWxAMTYzLmNvbQ==
†These authors have contributed equally to this work