- 1Science for Life Laboratory, Department of Medical Biochemistry and Microbiology, Uppsala University, Uppsala, Sweden
- 2Science for Life Laboratory, Department of Immunology, Genetics and Pathology, Uppsala University, Uppsala, Sweden
- 3Science for Life Laboratory, Department of Medical Sciences, Uppsala University, Uppsala, Sweden
- 4Department of Rheumatology, University Hospital Linköping, Linköping, Sweden
- 5Department of Biomedical and Clinical Sciences, Linköping University, Linköping, Sweden
- 6Broad Institute of MIT and Harvard, Cambridge, MA, United States
Objectives: To further elucidate the role of the MHC in ankylosing spondylitis by typing 17 genes, searching for HLA-B∗27 independent associations and assessing the impact of sex on this male biased disease.
Methods: High-confidence two-field resolution genotyping was performed on 310 cases and 2196 controls using an n-1 concordance method. Protein-coding variants were called from next-generation sequencing reads using up to four software programs and the consensus result recorded. Logistic regression tests were applied to the dataset as a whole, and also in stratified sets based on sex or HLA-B∗27 status. The amino acids driving association were also examined.
Results: Twenty-five HLA protein-coding variants were significantly associated to disease in the population. Three novel protective associations were found in a HLA-B∗27 positive population, HLA-A∗24:02 (OR = 0.4, CI = 0.2–0.7), and HLA-A amino acids Leu95 and Gln156. We identified a key set of seven loci that were common to both sexes, and robust to change in sample size. Stratifying by sex uncovered three novel risk variants restricted to the female population (HLA-DQA1∗04.01, -DQB1∗04:02, -DRB1∗08:01; OR = 2.4–3.1). We also uncovered a set of neutral variants in the female population, which in turn conferred strong effects in the male set, highlighting how population composition can lead to the masking of true associations.
Conclusion: Population stratification allowed for a nuanced investigation into the tightly linked MHC region, revealing novel HLA-B∗27 signals as well as replicating previous HLA-B∗27 dependent results. This dissection of signals may help to elucidate sex biased disease predisposition and clinical progression.
Introduction
Ankylosing spondylitis (AS) is a chronic inflammatory disease defined by the inflammation of the spine and sacroiliac joints, which if left untreated, leads to vertebrae fusion (Cortes et al., 2013). The disease prevalence in Sweden is 0.18% (Exarchou et al., 2015) and Europe in 0.24% (Dean et al., 2014), and contrary to most immunological diseases, AS affects males more often than females [Sweden 1.6:1 (Exarchou et al., 2015), Europe 2–3:1 (Lee et al., 2008)]. Not only is the prevalence of disease different between sexes, but so are the manifestations, e.g., males have greater radiographic changes compared with female patients (Lee et al., 2008; Ward et al., 2009).
Approximately 80% of AS cases are HLA-B∗27 positive, while only a small fraction (<5%) of carriers develop disease (Cortes et al., 2013). This clearly indicates that there are other genetic factors involved in disease predisposition. Together with HLA-B, more than 45 genes have been suggested to contribute to disease risk, e.g., ERAP1, IL23R, and RUNX3 (Burton et al., 2007; Evans et al., 2011; Cortes et al., 2013; Ranganathan et al., 2017), but combined, these explain less than 30% of the genetic heritability (Burton et al., 2007; Evans et al., 2011; Cortes et al., 2013) of this highly heritable disease (h2 > 90%, Cortes et al., 2013).
The strongest AS association signal comes from the MHC (Reveille, 2014), a region where the genetic contribution is hard to dissect due to high linkage disequilibrium (LD). Previous studies have indicated that variants in addition to HLA-B∗27 are driving the signal, for example, HLA-A∗02:01 has been associated with HLA-B∗27 positive [odds ratio (OR) = 1.2] (Reveille, 2014; Cortes et al., 2015), as well as HLA-B∗27 negative disease (OR = 1.4) (Reveille, 2014; Cortes et al., 2015). Whereas HLA-B∗07:02 (OR = 0.8), -B∗40:01 (OR = 1.2), and -DRB1∗01:03 (OR = 1.2) were shown to be significantly associated with AS in a mixed HLA-B∗27 study, i.e., one containing both HLA-B∗27 positive and negative samples (Cortes et al., 2015). These studies highlight the challenges in assessing disease HLA associations, with inconsistencies in replication partly driven by differing sample ancestry, sample sizes, gene loci considered, genotyping methods and levels of phenotypic information (e.g., sex ratio). For example, the small risk conferred by HLA-A∗02:01 is seldom replicated, likely due to the low odds ratio requiring larger sample sizes (Reveille, 2014). In other examples, associations may be a reflection of HLA-B∗27 carrier status and enriched haplotypic pairs within a population. Several variants within the HLA-B locus have reported disease association, but only three have been associated in distinct HLA-B∗27 positive (HLA-B∗40:01) (Cortes et al., 2015), or negative (HLA-B∗44 and -B∗49) (Reveille et al., 2019), populations. The same is true for MICA, where MICA∗007:01 has been shown to contribute strong susceptibility to both HLA-B∗27 mixed (OR = 60.7) and negative disease (OR = 9.1) (Zhou et al., 2014). A separate study failed to replicate the latter and claimed that the mixed result may be due to linkage to HLA-B∗27 (Cortes et al., 2018). The first study used lab typing at a two-field resolution (Zhou et al., 2014) while the latter imputed MICA with SNP2HLA and reported at one-field resolution (Cortes et al., 2018). Although both examined Caucasian populations of European ancestry, these differed in size and composition (sex distribution was not reported in one study), confounding the comparison (Zhou et al., 2014; Cortes et al., 2018).
While 37 separate genes are referenced in the IPD-IMGT/HLA database (release 3.37.0), to date the maximum number of genes studied in any single study was six, HLA-A, -B, -C, -DRB1, -DQB1, and -DPB1 (Reveille et al., 2019). That analysis of a PCR lab-typed Caucasian population (1948 cases/990 controls) performed analyses on their total dataset, followed by an nested test on only those samples which were HLA-B∗27 negative (Reveille et al., 2019). At least one variant from each gene examined was shown to be significantly associated to disease in the HLA-B∗27 mixed population, with almost two thirds conferring protection (28 variants; OR = 0.3–0.8, risk OR = 1.3–21.4) (Reveille et al., 2019). Fewer associations were detected in the HLA-B∗27 negative population; however five genes (11 variants) were shown to be linked to AS, with the protective contribution dropping to around 50% (Reveille et al., 2019). No similar efforts have been performed in a HLA-B∗27 positive population.
Here we aimed to build the largest typed MHC gene set (17 genes; HLA-A, -B, -C, -DOA, -DOB, -DPA1, -DPB1, -DQA1, -DQB1, -DRA, -DRB1, -E, -F, -G, MICA MICB, and TAP2) for a single AS population, and with this data address the following; can we identify novel signals of AS association, are these different between the sexes, and are they independent of HLA-B∗27.
Materials and Methods
Sample Data
Samples were drawn from existing targeted (SweAS and Uppsala Bioresource, Eriksson et al., 2016) or whole genome sequencing (SweGen, Ameur et al., 2017) experiments and used to build the case and control populations (Table 1). Cases were from SweAS (n = 310, 26.8% female and 73.2% males) and controls from SweAS (n = 381, age and region matched to cases from South East Sweden), SweGen (n = 1000, obtained from across Sweden), and the Uppsala Bioresource (n = 815, from South East Sweden). In total, 2196 controls were collated, 40.2% were males and 59.8% females (ages for cases and controls summarized in Supplementary Figure 1). Cases were diagnosed according to the modified New York criteria (van der Linden et al., 1984) and four comorbidities (psoriasis, uveitis, peripheral joint involvement and gut involvement) were recorded (Supplementary Figure 2). The SweAS population was enrolled under the ethical approval granted from the Regional Committee of Linköping, Dnrs 2010/182-3 and 98110, whereas ethical approvals for SweGen and the Uppsala Bioresource are as per their cited publications.
HLA Variant Typing
An n-1 concordance method (Nordin et al., 2020) was used to ensure high quality genotyping across the MHC. The inputs for this were raw HLA genotype calls generated from four separate software programs, with consensus variant calls reported with 2-field resolution. In brief, this meant that for a variant to be called in the final set, the result had to be identical across three out of four programs (Supplementary Figure 3). As noted previously (Nordin et al., 2020), this procedure can account for software biases, such as reference version and algorithm choice. Called chromosome 6 SNPs were the base data for imputation (SNP2HLA, Jia et al., 2013), whereas reads mapped to chromosome 6, plus unmapped reads, were used as inputs for inference tools [HLA-VBSeq (Nariai et al., 2015), HLAscan (Ka et al., 2017), and HLA-HD (Kawaguchi et al., 2017)]. SNP2HLA, HLA-VBSeq and HLAscan were previously used to genotype SweGen at eight HLA genes (Nordin et al., 2020), however this was expanded to 17 genes (HLA-A, -B, -C, -DOA, -DOB, -DPA1, -DPB1, -DQA1, -DQB1, -DRA, -DRB1, -E, -F, -G, MICA, MICB, and TAP2) with the inclusion of HLA-HD. The impact of biases on genotyping this set of genes was assessed with average read depth across each for the three sample populations [10 bp bins in BEDtools (Quinlan and Hall, 2010) v2.26.0], shared variant availability across software references, and concordance rate between the high confidence set and each software. See Supplementary Methods for information on software algorithms and running conditions.
Association Tests and Statistical Methods
In order to address the question of sex bias, the dataset was partitioned into three sets; ALL, all samples; F, female samples; M, male samples (Table 1). To test for independence to HLA-B∗27, each analysis was repeated using only those samples carrying at least one copy of any HLA-B∗27 variant (HLA-B∗27 positive): ALL.B27, F.B27, and M.B27 (Table 1).
Genes were excluded from all six analyses if their genotyping rate was below 80% in ALL. Before the association tests were performed, the potential impact of data missingness was investigated with Fisher’s exact test, and sequencing batch effects were assessed with logistic regression association tests on targeted versus whole genome sequenced controls. Disease association employed logistic regression with an additive model. Sex was shown to be significantly associated with disease status in both the ALL and ALL.B27 populations and was included as a covariate in those tests (z-score for proportions, p-value < 1.0 × 10–5). The association between AS and HLA amino acids in the HLA-B∗27 positive population, or MICA’s transmembrane region (TM) for all six sets, were also explored (Fisher’s exact test, without covariates). This last phase of the association study was conducted per gene and did not consider individuals with missing data. Disease association tests were performed with PyHLA (Fan and Song, 2017). Phenotype permutations (n = 1000) were used to determine cohort specific significance thresholds (5% false discovery rate, FDR) for gene, amino acid and TM tests (Table 1). A second threshold (p-value < 0.05) was used to identify suggestive gene results, this time compared to Bonferroni adjusted p-values (number of variants for that gene∗unadjusted p-value).
Gene level pair-wise LD was measured using the multi-marker statistic, x2’ (Zhao et al., 2005). Only samples with a 100% genotyping rate for the genes of interest were taken forward for phasing as described previously (PHASE v2.1.1, Stephens et al., 2001; Nordin et al., 2020). Variant level pair-wise LD was calculated with phased inputs (r2). For significant amino acids, Students t-test was used to assess phenotype enrichment (age and C-reactive protein, CRP). The potential consequence of significant amino acids on protein structure were explored with SNPeffect 4.0 (De Baets et al., 2012), MHC motif viewer (Rapin et al., 2010), and visualized with Chimera (Pettersen et al., 2004).
Results
Seventeen genes were genotyped at twofield resolution with a success rate of between 77 and 100% (Supplementary Table 1). This rate was driven by a combination of data input and software biases, where some genes were genotyped at a higher rate with targeted data than WGS (e.g., TAP2) and some gene call rates were affected by variant availability and software choice (Supplementary Table 3 and Supplementary Figures 4–6). The majority of cases were HLA-B∗27 positive (cases/controls 96.8/13.9%, Table 1), and as a group, cases were less polymorphic than controls in terms of variant count, but more heterozygous overall (Supplementary Table 1). The presence of HLA-B∗27 was skewed across the sets, with a slightly higher fraction observed in males compared with females (e.g., cases M/F = 98.3/92.9%, Table 1).
Association Tests Revealed Female Specific Results
Of the 15 genes passing quality control (HLA-DPA1 and TAP2 excluded), 9 genes (25 protein-coding variants) conferred a significant effect in ALL (Figure 1 and Supplementary Table 3). HLA-B∗27:05 demonstrated the most associated risk (OR = 54.9, p-value = 7.1 × 10–68), followed by MICA∗007:01 (OR = 89.4, p-value = 3.0 × 10–63) and -C∗02:02 (OR = 10.6, p-value = 6.3 × 10–61). Interestingly, HLA-A (∗02:01 and ∗31:01) showed a purely protective profile (Figure 1). Eight variants from HLA-A, -B, -C, -DQA1, -DQB1 and –F were suggestively associated with disease (Supplementary Figure 6).
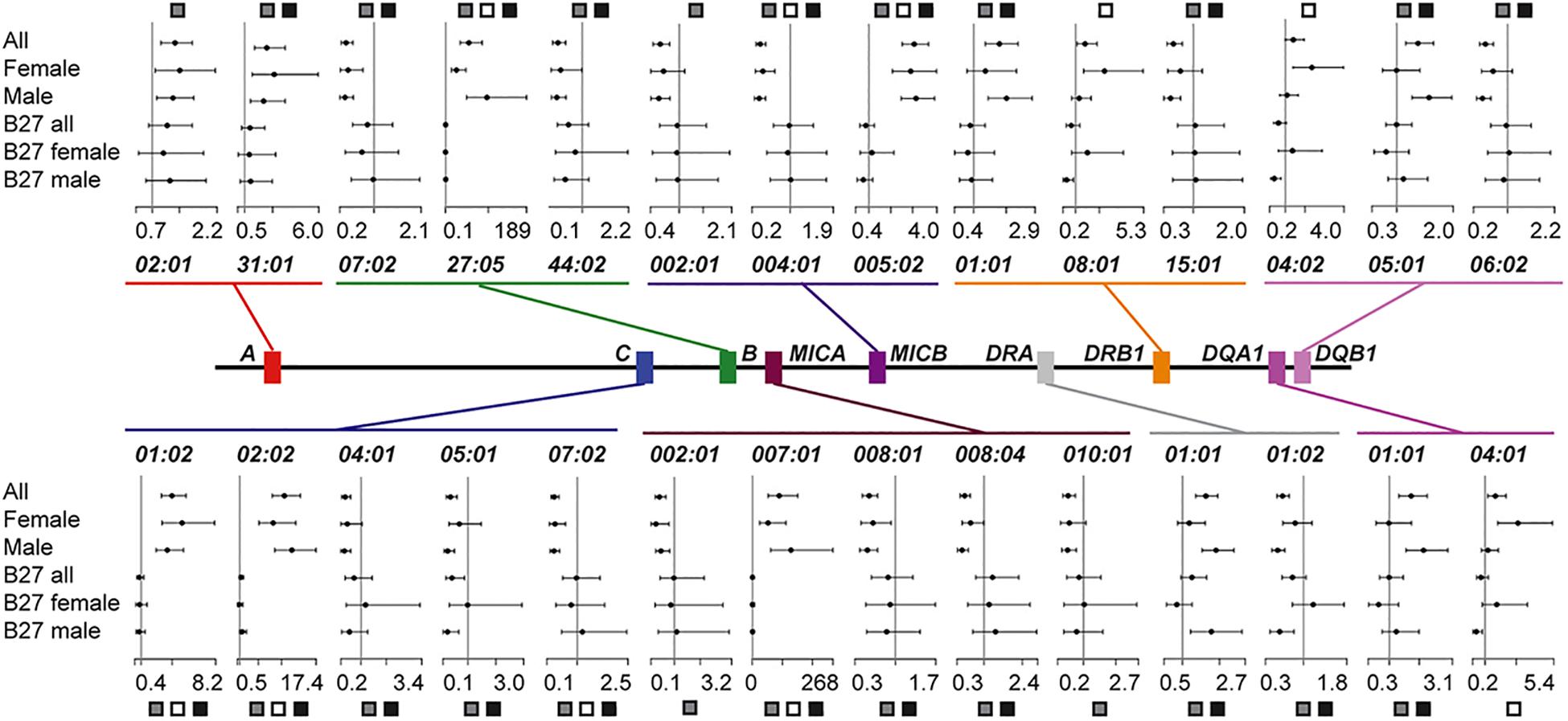
Figure 1. Summary of significant HLA protein-coding variant associations and their effect sizes. Plots illustrate the odds ratio (OR) and 95% confidence interval for each of the six datasets considered. An OR of 1 is indicated with a vertical line. The dataset for which the protein-coding variant was significant is indicated with boxes: All (ALL), gray; Female (F), white; Male (M), black.
We next stratified our ALL population by sex to assess if this covariate was masking signals of association. We found that seven protein-coding variants across HLA-B, -C, MICA, and MICB were significant, irrespective of sex or population size (Figure 1, Datasets ALL, F, and M). Perhaps reflecting ALL dataset composition (73% males), 14 significant variants were common to the ALL and M sets, but none were shared by only the ALL and F populations. For most variants shared between ALL and M (12/14), the OR for F had the same direction of effect, even though it was not significant. However, for some protein-coding variants, e.g., HLA-DQA1∗01:01 and -DQB1∗05:01, the modest effect conferred in ALL and M (OR = 1.6–1.7) was neutral in F (OR = 1). Intriguingly, three variants were significant only in F, HLA-DQA1∗04:01, -DQB1∗04:02 and -DRB1∗08:01 (F p-value = 3.4 × 10–5–1.3 × 10–3, M p-value > 0.39, Figure 1 and Supplementary Table 3). Each of these, class II variants conferred additional risk (OR = 2.4–3.1), but with broad confidence intervals (Supplementary Table 3). This result was not only driven by LD with HLA-B∗27 (r2 < 0.01), as the HLA-DQA1∗04:01 -DQB1∗04:02 -DRB1∗08:01 haplotype was also observed to segregate more frequently with HLA-B∗35:01 in cases than controls (Supplementary Table 4).
The protein-coding variant frequency differences driving these sex specific signals were explored further. For the three F specific significant variants, the delta variant frequency (ΔVF) between cases and controls was greater than 6% (HLA-DQA1∗04:01 = 9.3%, -DQB1∗04:02 = 6.6%, -DRB1∗08:01 = 6.5%). For the same protein-coding variants in M, the ΔVF was < 0.02%, explaining why these signals were not significant in ALL or M. The largest ΔVFs calculated between M and F datasets were for both DRA variants (>10%, only considering variants significant in ALL).
HLA-B∗27 Positive Cohort Reveals Novel HLA-A Association
While the pair-wise LD between the significant MHC genes was low (x2′ < 0.01, Supplementary Table 5), this did not indicate that the individual protein-coding variants across MHC genes were independent. For that question, the HLA-B∗27 positive datasets were tested. The result was a single significant variant from ALL.B27, HLA-A∗24:02 (OR = 0.4, p-value = 1.7 × 10–3, Figure 2A and Supplementary Table 3). Phasing revealed the HLA-A∗24:02-HLA-B∗27:05 combination to be one of the most common haplotypes in either cases or controls for this dataset (haplotype case/control = 3.1/8.1%; Supplementary Table 6). However, HLA-A∗24:02 was observed to be segregating with nine additional HLA-B protein-coding variants, none of which were HLA-B∗27 variants (Supplementary Table 7) and the LD between the HLA-B∗27 and –A∗24:02 was negligible (r2 < 0.01). Within the divided sex sets, HLA-A∗24:02 showed suggestive significance within M.B27, along with the risk variant HLA-DRA∗01:01 and protective variant HLA-DRA∗01:02 (p-value < 3.0 × 10–3, Supplementary Table 3). No significant or suggestive associated variants were detected in F.B27.
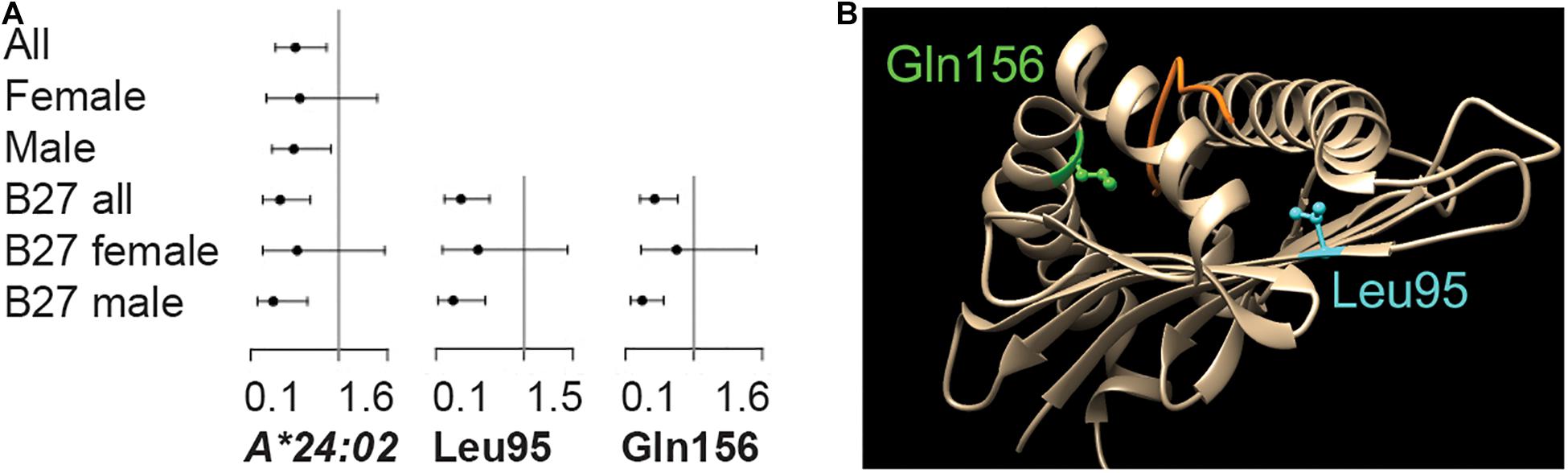
Figure 2. Variants within the HLA-A locus are significantly associated with HLA-B*27 positive disease. (A) Significant association was observed for protein-coding variant HLA-A*24:02 and amino acid position Leu95 in the ALL cohort and Gln156 in the Male dataset. Odds ratios and 95% confidence intervals are indicated. (B) The physical position of each amino acid is indicated.
Amino Acids in HLA-A Are Significantly Associated With Disease
While no significant associations were observed between disease and MICA TM repeats, tests of association using amino acids across the HLA-B∗27 positive population did resolve divided or underpowered signals from the gene tests. The result was two protective HLA-A amino acids, Ile/Val95Leu (ALL.B27 p-value = 3.2 × 10–4) and Arg/Leu/Trp156Gln (M.B27 p-value = 1.8 × 10–4; F.B27 p-value > 0.05) (Figure 2A and Supplementary Table 3). For Leu95, the overall ΔVF between cases and controls (13.7%) was reflected in both M.27 and F.27 (17.4% and 8.8% respectively), however, the skew was more pronounced for Gln156 (ALL.B27 = 18.0%, M.B27 = 22.6% and F.B27 = 4.6%). The protein-coding variant driving the result for both amino acids was HLA-A∗24:02 (significant in ALL.B27 and suggestive in M.B27, Figure 2A), with additional amino acid frequency contributed via, HLA-A∗02:05 and -A∗23:01 for Leu95, and HLA-A∗03:02, -A∗11:01, and -A∗26:08 for Gln156. Figure 2B illustrates both amino acids relative to protein structure 3UTQ (HLA-A∗02:01). Leu95 is located on the β-sheet of the binding groove in the peptide-binding pocket F and interacts with the C-terminus of the peptide, while Gln156 is located on an α-chain which is a part of peptide binding pockets D and E (Guan et al., 2005) and interacts with three peptide positions. Due to the HLA-A protein-coding variant composition in our dataset, the potential amino acid combinations for each position are Ile/Val95Leu and Arg/Leu/Trp156Gln. For position 95, all options are hydrophobic, with Val having the smallest side chain. Arg156 is positively charged, while the other three amino acids available at this position are uncharged. However Leu (smallest side chain) and Trp are hydrophobic, compared to hydrophilic Gln. Both the change of charge and the size of side chain are factors that can affect peptide binding. We searched for an enrichment of the protein-coding variant containing these amino acids with respect to comorbidities, CRP levels and age at sampling, but none was significant.
Discussion
With this study we have extended the number of MHC genes examined for a single AS population from 6 up to 15, and found nine of these significantly linked to disease. Through a nested analysis, we were also able to reveal patterns of association related to sex, and HLA-B∗27 status. As we used an n-1 genotyping methodology, the results are agnostic to individual software choice (Nordin et al., 2020). This is essential given the variability of HLA variant frequency observed when only one method is considered (e.g., HLA-B∗27:05 frequency ranged between 4.8–8.0% in a study of 1000 Swedes) (Nordin et al., 2020).
The Swedish AS population examined here was largely reflective of published European datasets (Nordin et al., 2020), with the most frequent HLA-B∗27 protein-coding variant being HLA-B∗27:05 (97.8% cases, 7% controls). The risk conferred by this variant (ALL OR = 54.9) was one of seven significant signals we identified that had robust effect sizes in each dataset (ALL, M, and F; Figure 1). Within class I, HLA-C∗01:02 and –C∗02:02 added risk (ALL OR = 4.0–10.6), whilst –C∗07:02 was protective (ALL OR = 0.3). These variants were in high LD with HLA-B∗27 and have never been reported as independently associated. However, HLA-C∗08 variants have previously been linked to HLA-B∗27 positive disease (Jiao et al., 2010), but in our HLA-B∗27 positive population these variants were rare (1%), and had equal frequency in cases and controls.
For class I like genes, MICA∗007:01 and MICB∗005:02 enhanced risk (OR = 89.5 and OR = 3.1), while MICB∗004:01 was protective (OR = 0.4). The role and independence of MICA∗007:01 is debated with regards to AS predisposition (Zhou et al., 2014; Cortes et al., 2018; Cortes and Brown, 2019; Zhou and Reveille, 2019). However, in our population MICA∗007:01 and HLA-B∗27:05 were tightly linked (ALL, r2 = 0.93), and so not independently associated with disease. Interestingly, even though HLA-B∗27:05 has the lowest p-value in our study, MICA∗007:01 has the strongest effect (OR = 89.5), stronger than that noted for the 2014 discovery population (OR = 60.7) (Zhou et al., 2014). While MICA has been investigated in several publications (Reveille, 2014; Zhou et al., 2014; Cortes et al., 2018), MICB has not been equally studied, and except for suggestions of linkage via LD (Brown et al., 2002), the gene has been largely overlooked. Three MICB protein-coding variant conferred risk in ALL and whilst not independent of HLA-B∗27, may have a disease modifying effect. For example, the MICB∗005:02 variant encodes soluble MICB, which in turn inhibits signaling through NKG2D and leads to hyporesponsive NK cells (Cox et al., 2018). It is not clear how this reduced reactiveness would increase disease risk, but the effect could be related to other immune cell interactions.
Due to genotype resolution, it was only possible to compare our results for HLA-DRB1 and -DQB1 with those from the large lab-type six gene MHC study (Reveille et al., 2019). In that experiment, ten class II variants were associated with disease (protective OR = 0.5–0.6 and risk OR = 1.3–2.7) (Reveille et al., 2019). We were able to replicate four variants, with similar effect sizes and same directionality, even though our population was smaller and we employed additional software programs for typing (HLA-DRB1∗01:01 OR = 1.8, -DQB1∗05:01 OR = 1.6, -DRB1∗15:01 and -DQB1∗06:02 both OR = 0.5) (Reveille et al., 2019). The inability to replicate all signals could be a reflection of study size, or population differences (Swedish versus a mixed European). For example, HLA-DRB1∗04:04 had a published case/control variant frequency of 6.5/2.5% (OR = 2.72) (Reveille et al., 2019), while in our dataset it was 4.9/4.1% (OR = 1.28). Our choice of concordance methodology assisted reproducibility, but our homogenous genetic background meant some loci were largely neutral.
Our cases were predominantly male (73%), and this was likely reflected in the sharing of significant results between the ALL and M datasets (14 alleles, Figure 1). However, our smaller F set was sufficiently powered to identify three variants that increased disease susceptibility in this sex alone (HLA-DQA1∗04:01, -DRB1∗08:01, -DQB1∗04:02; Figure 1). While HLA-DQB1∗04:02 and -DQA1∗04:01 have not been noted previously, the presence of DRB1∗08:01 has been shown to be negatively associated with AS radiographic severity (as BASRI-spine score normalized for AS duration; Ward et al., 2009). It could be that the increased frequency of this protein-coding variant in the F dataset reflects the reduced BASRI-spine score observed in female patients (Lee et al., 2007). Intriguingly, DRB1∗08:01 has also been shown to enhance the effect of additional HLA variants in multiple sclerosis, an autoimmune disease more prevalent in women (Dyment et al., 2005). Shared genetic risk factors outside of the MHC have been reported for these diseases previously (e.g., IL7R, PTGER4, Cortes et al., 2013), and these results suggest additional investigations are warranted.
We used HLA-B∗27 positive datasets in the attempt to identify signals independent of this key variant, and discovered novel associations between HLA-A and AS (HLA-A∗24:02 and amino acids Ile/Val95Leu and Arg/Leu/Trp156Gln, OR = 0.3–0.4). While HLA-A∗24:02 was shown to segregate with HLA-B∗27:05, the presence of nine additional HLA-A∗24:02 haplotypes indicated that this protective signal was not solely driven by hitchhiking with HLA–B∗27:05. In terms of HLA-A amino acids, both Leu95 and Gln156 have the potential to interact with the peptide within the binding groove domain (Figure 2B). The Ile/Val95Leu change lies within pocket F which is critical for the binding of the peptide, and may mediate the peptide’s PΩ anchor binding ability (Bade-Doeding et al., 2007). A protective association was revealed between this amino acid and psoriasis vulgaris (Okada et al., 2014), however the mode of action was unknown. Residue 156 is part of pockets D and E, and changes here can directly impact the protein’s ability to bind peptides, and changes at Gln156 can also result in shorter than average peptide–MHC class I complexes being presented to nucleated cells (Eichmann et al., 2014). Alterations at this position have been suggested to act as part of the mechanism behind graft rejection following hematopoietic stem cell transplantation (HSCT) (Balas et al., 2017). Intriguingly, changes at both residues 95 and 156 have been shown to negatively affect the 100 days survival after HSCT, highlighting the role of these positions in the immune response (Marino et al., 2012). A recent study of HLA-DRB1 class II molecules in multiple sclerosis, suggested that HLA variants might act in trans to compensate for the effect of risk variants (Mamedov et al., 2020). In those studies, it was suggested that the protective molecule possessed the kinetic ability to discriminate between endogenous and exogenous peptide, a characteristic not present in the risk variant. This process would serve to reduce the density of functional MHC clusters and so down regulate T cell response (Mamedov et al., 2020). In the case of AS, the compensatory mechanism would be between HLA-A∗24:02 and HLA-B∗27. While certain HLA-A and HLA-B variants can recognize the same epitopes (Marsh et al., 2021), it is not known if peptide kinetics could be the protective mechanism for these class I molecules. It is clear that HLA-A∗24:02, and amino acid residues 95 and 156, warrant further investigation as to their protective role in AS disease modification.
This is the largest study to search for correlations between the MHC and AS in Sweden. It is also to date, the largest MHC gene set considered for this disease. However, there are certain limitations to the current study. We used short read next generation sequencing data and the concordance results from four software programs to genotype the case and control samples considered here. As noted, our genotypes are robust, but we were unable to access a similar dataset for replication, or an imputed or lab typed AS dataset in which to replicate our findings. We also limited our investigation of AS association to the MHC protein-coding variants, and note that further clinical investigation of these results should be undertaken in the context of the nuclear genomes of the patients and controls considered.
With this work we revealed novel associations with likely clinical consequence, and confirmed the impact of several key class I and II protein-coding variants to disease. We clearly showed that clinical phenotype, sex-stratification of disease, is mirrored by the underlying genetics of AS, and suggest future studies consider the sexes separately in order to tease apart the signals that are being masked in heterogeneous populations.
Data Availability Statement
The case and control population protein-coding variant frequency data are freely available (doi: 10.17044/scilifelab.13386653). Flat files containing per individual HLA genotyping data generated from each software program, and for the final concordance data set, are available upon request with agreement to terms and conditions for data download.
Ethics Statement
The studies involving human participants were reviewed and approved by the Regional Committee of Linköping, Dnrs 2010/182-3 and 98110 (SweAS population), and as per cited publications (SweGen and the Uppsala Bioresource). The patients/participants provided their written informed consent to participate in this study.
Author Contributions
JN and JM designed the project and drafted the manuscript with input from all authors. AlK, JC, PE, and PS attained and characterized the SweAS population. KT and LR performed a similar task for the Uppsala Bioresource. ÅsK, EM, AM, and JM generated sequencing data while JN, LHR, AM, and JM performed the bioinformatics analyses with statistical support from MP and insight from KL-T. All the authors revised and approved the manuscript.
Funding
This research was supported by the Swedish Research Council, FORMAS (Dnr 2012-1531), Knut and Alice Wallenberg Foundation (Dnr 2012-0268), Swedish Research Council for Medicine and Health (D0283001 and Dnr 2018-02399), the Swedish Rheumatism Association, and King Gustaf V’s 80-year Foundation. The funders had no role in study design, data collection and analysis, decision to publish, or preparation of the manuscript.
Conflict of Interest
The authors declare that the research was conducted in the absence of any commercial or financial relationships that could be construed as a potential conflict of interest.
Acknowledgments
Thank you to Maija-Leena Eloranta for her role in helping to establish and recruit individuals to the Uppsala Bioresource, and Matteo Bianchi for facilitating bioinformatics access to this resource. Raw sequencing data was provided following data agreements, and with thanks, from the SweGen Research Team (Uppsala University, Department of Immunology, Genetics and Pathology). Computational resources were provided by the Swedish National Infrastructure for Computing (SNIC) at the Uppsala Multidisciplinary Center for Advanced Computational Science (UPPMAX) under projects sens2017579 and sens2016003. We also thank patient and control members for their participation.
Supplementary Material
The Supplementary Material for this article can be found online at: https://www.frontiersin.org/articles/10.3389/fgene.2021.659042/full#supplementary-material
References
Ameur, A., Dahlberg, J., Olason, P., Vezzi, F., Karlsson, R., Martin, M., et al. (2017). SweGen: a whole-genome data resource of genetic variability in a cross-section of the Swedish population. Eur. J. Hum. Genet. 25, 1253–1260. doi: 10.1038/ejhg.2017.130
Bade-Doeding, C., DeLuca, D. S., Seltsam, A., Blasczyk, R., and Eiz-Vesper, B. (2007). Amino acid 95 causes strong alteration of peptide position PΩ in HLA-B∗41 variants. Immunogenetics 59, 253–259. doi: 10.1007/s00251-007-0197-7
Balas, A., Sánchez-Gordo, F., Gomez-Zumaquero, J. M., and Vicario, J. L. (2017). HLA-A∗30:99 shows a new amino acid position 156 within HLA-A∗30 subtypes. HLA 89, 53–54. doi: 10.1111/tan.12934
Brown, M. A., Wordsworth, P. B., Reveille, J. D., Wordsworth, B. P., Reveille, J. D., and Brown, M. A. (2002). Genetics of ankylosing spondylitis. Clin. Exp. Rheumato 20, S43–S49. doi: 10.1016/j.molimm.2013.06.013
Burton, P. R., Clayton, D. G., Cardon, L. R., Craddock, N., Deloukas, P., Duncanson, A., et al. (2007). Association scan of 14,500 nonsynonymous SNPs in four diseases identifies autoimmunity variants. Nat. Genet. 39, 1329–1337. doi: 10.1038/ng.2007.17
Cortes, A., and Brown, M. A. (2019). Response to: ‘Letter in response to article “Imputation-based analysis of MICA alleles in the susceptibility to ankylosing spondylitis”’ by Zhou et al. Ann. Rheum. Dis. 79:e2. doi: 10.1136/annrheumdis-2018-214765
Cortes, A., Gladman, D., Raychaudhuri, S., Cui, J., Wheeler, L., and Brown, M. A. (2018). Imputation-based analysis of MICA alleles in the susceptibility to ankylosing spondylitis. Ann. Rheum. Dis. 77, 1691–1692. doi: 10.1136/annrheumdis-2018-213413
Cortes, A., Hadler, J., Pointon, J. P., Robinson, P. C., Karaderi, T., Leo, P., et al. (2013). Identification of multiple risk variants for ankylosing spondylitis through high-density genotyping of immune-related loci. Nat. Genet. 45, 730–738. doi: 10.1038/ng.2667
Cortes, A., Pulit, S. L., Leo, P. J., Pointon, J. J., Robinson, P. C., Weisman, M. H., et al. (2015). Major histocompatibility complex associations of ankylosing spondylitis are complex and involve further epistasis with ERAP1. Nat. Commun. 6:7146. doi: 10.1038/ncomms8146
Cox, S. T., Danby, R., Hernandez, D., Madrigal, J. A., and Saudemont, A. (2018). Functional characterisation and analysis of the soluble NKG2D ligand repertoire detected in umbilical cord blood plasma. Front. Immunol. 9:1282. doi: 10.3389/fimmu.2018.01282
De Baets, G., Van Durme, J., Reumers, J., Maurer-Stroh, S., Vanhee, P., Dopazo, J., et al. (2012). SNPeffect 4.0: on-line prediction of molecular and structural effects of protein-coding variants. Nucleic Acids Res. 40, 935–939. doi: 10.1093/nar/gkr996
Dean, L. E., Jones, G. T., MacDonald, A. G., Downham, C., Sturrock, R. D., and Macfarlane, G. J. (2014). Global prevalence of ankylosing spondylitis. Rheumatology 53, 650–657. doi: 10.1093/rheumatology/ket387
Dyment, D. A., Herrera, B. M., Cader, M. Z., Willer, C. J., Lincoln, M. R., Sadovnick, A. D., et al. (2005). Complex interactions among MHC haplotypes in multiple sclerosis: susceptibility and resistance. Hum. Mol. Genet. 14, 2019–2026. doi: 10.1093/hmg/ddi206
Eichmann, M., de Ru, A., van Veelen, P. A., Peakman, M., and Kronenberg-Versteeg, D. (2014). Identification and characterisation of peptide binding motifs of six autoimmune disease-associated human leukocyte antigen-class I molecules including HLA-B∗39:06. Tissue Antigens 84, 378–388. doi: 10.1111/tan.12413
Eriksson, D., Bianchi, M., Landegren, N., Nordin, J., Dalin, F., Mathioudaki, A., et al. (2016). Extended exome sequencing identifies BACH2 as a novel major risk locus for Addison’s disease. J. Intern. Med. 280, 595–608. doi: 10.1111/joim.12569
Evans, D. M., Spencer, C. C. A., Pointon, J. J., Su, Z., Harvey, D., Kochan, G., et al. (2011). Interaction between ERAP1 and HLA-B27 in ankylosing spondylitis implicates peptide handling in the mechanism for HLA-B27 in disease susceptibility. Nat. Genet. 43, 761–767. doi: 10.1038/ng.873
Exarchou, S., Lindström, U., Askling, J., Eriksson, J. K., Forsblad-d’Elia, H., Neovius, M., et al. (2015). The prevalence of clinically diagnosed ankylosing spondylitis and its clinical manifestations: a nationwide register study. Arthritis Res. Ther. 17:118. doi: 10.1186/s13075-015-0627-0
Fan, Y., and Song, Y.-Q. (2017). PyHLA: tests for the association between HLA alleles and diseases. BMC Bioinformatics 18:90. doi: 10.1186/s12859-017-1496-0
Guan, P., Doytchinova, I. A., Walshe, V. A., Borrow, P., and Flower, D. R. (2005). Analysis of Peptide–Protein binding using amino acid descriptors: prediction and experimental verification for human histocompatibility complex HLA-A∗0201. J. Med. Chem. 48, 7418–7425. doi: 10.1021/jm0505258
Jia, X., Han, B., Onengut-Gumuscu, S., Chen, W.-M. M., Concannon, P. J., Rich, S. S., et al. (2013). Imputing amino acid polymorphisms in human leukocyte antigens. PLoS One 8:e64683. doi: 10.1371/journal.pone.0064683
Jiao, Y.-L., Zhang, B.-C., You, L., Li, J.-F., Zhang, J., Ma, C.-Y., et al. (2010). Polymorphisms of KIR Gene and HLA-C alleles: possible association with susceptibility to HLA-B27-Positive patients with ankylosing spondylitis. J. Clin. Immunol. 30, 840–844. doi: 10.1007/s10875-010-9444-z
Ka, S., Lee, S., Hong, J., Cho, Y., Sung, J., Kim, H.-N., et al. (2017). HLAscan: genotyping of the HLA region using next-generation sequencing data. BMC Bioinformatics 18:258. doi: 10.1186/s12859-017-1671-3
Kawaguchi, S., Higasa, K., Shimizu, M., Yamada, R., and Matsuda, F. (2017). HLA-HD: an accurate HLA typing algorithm for next-generation sequencing data. Hum. Mutat. 38, 788–797. doi: 10.1002/humu.23230
Lee, W., Reveille, J. D., Davis, J. C., Learch, T. J., Ward, M. M., and Weisman, M. H. (2007). Are there gender differences in severity of ankylosing spondylitis? Results from the PSOAS cohort. Ann. Rheum. Dis. 66, 633–638. doi: 10.1136/ard.2006.060293
Lee, W., Reveille, J. D., and Weisman, M. H. (2008). Women with ankylosing spondylitis: a review. Arthritis Rheum. 59, 449–454. doi: 10.1002/art.23321
Mamedov, A., Vorobyeva, N., Filimonova, I., Zakharova, M., Kiselev, I., Bashinskaya, V., et al. (2020). Protective allele for multiple sclerosis HLA-DRB1∗01:01 provides kinetic discrimination of myelin and exogenous antigenic peptides. Front. Immunol. 10:3088. doi: 10.3389/fimmu.2019.03088
Marino, S. R., Lin, S., Maiers, M., Haagenson, M., Spellman, S., Klein, J. P., et al. (2012). Identification by random forest method of HLA class i amino acid substitutions associated with lower survival at day 100 in unrelated donor hematopoietic cell transplantation. Bone Marrow Transplant. 47, 217–226. doi: 10.1038/bmt.2011.56
Marsh, J. C., Stanworth, S. J., Pankhurst, L. A., Kallon, D., Gilbertson, A. Z., Pigden, C., et al. (2021). An epitope-based approach of HLA-matched platelets for transfusion: a noninferiority crossover randomized trial. Blood 137, 310–322. doi: 10.1182/blood.2020007199
Nariai, N., Kojima, K., Saito, S., Mimori, T., Sato, Y., Kawai, Y., et al. (2015). HLA-VBSeq: accurate HLA typing at full resolution from whole-genome sequencing data. BMC Genomics 16:S7. doi: 10.1186/1471-2164-16-S2-S7
Nordin, J., Ameur, A., Lindblad-Toh, K., Gyllensten, U., and Meadows, J. R. S. (2020). SweHLA: the high confidence HLA typing bio-resource drawn from 1000 Swedish genomes. Eur. J. Hum. Genet. 28, 1–9. doi: 10.1038/s41431-019-0559-2
Okada, Y., Han, B., Tsoi, L. C., Stuart, P. E., Ellinghaus, E., Tejasvi, T., et al. (2014). Fine mapping major histocompatibility complex associations in psoriasis and its clinical subtypes. Am. J. Hum. Genet. 95, 162–172. doi: 10.1016/j.ajhg.2014.07.002
Pettersen, E. F., Goddard, T. D., Huang, C. C., Couch, G. S., Greenblatt, D. M., Meng, E. C., et al. (2004). UCSF Chimera - A visualization system for exploratory research and analysis. J. Comput. Chem. 25, 1605–1612. doi: 10.1002/jcc.20084
Quinlan, A. R., and Hall, I. M. (2010). BEDTools: a flexible suite of utilities for comparing genomic features. Bioinformatics 26, 841–842. doi: 10.1093/bioinformatics/btq033
Ranganathan, V., Gracey, E., Brown, M. A., Inman, R. D., and Haroon, N. (2017). Pathogenesis of ankylosing spondylitis — recent advances and future directions. Nat. Rev. Rheumatol. 13, 359–367. doi: 10.1038/nrrheum.2017.56
Rapin, N., Hoof, I., Lund, O., and Nielsen, M. (2010). The MHC motif viewer: a visualization tool for MHC binding motifs. Curr. Protoc. Immunol. 18:17. doi: 10.1002/0471142735.im1817s88
Reveille, J. D. (2014). An update on the contribution of the MHC to as susceptibility. Clin. Rheumatol. 33, 749–757. doi: 10.1007/s10067-014-2662-7
Reveille, J. D., Zhou, X., Lee, M., Weisman, M. H., Yi, L., Gensler, L. S., et al. (2019). HLA class I and II alleles in susceptibility to ankylosing spondylitis. Ann. Rheum. Dis. 78, 66–73. doi: 10.1136/annrheumdis-2018-213779
Stephens, M., Smith, N. J., and Donnelly, P. (2001). A new statistical method for haplotype reconstruction from population data. Am. J. Hum. Genet. 68, 978–989. doi: 10.1086/319501
van der Linden, S., Valkenburg, H. A., and Cats, A. (1984). Evaluation of diagnostic criteria for ankylosing spondylitis. Arthritis Rheum 27, 361–368. doi: 10.1002/art.1780270401
Ward, M. M., Hendrey, M. R., Malley, J. D., Learch, T. J., Davis, J. C., Reveille, J. D., et al. (2009). Clinical and immunogenetic prognostic factors for radiographic severity in ankylosing spondylitis. Arthritis Care Res. 61, 859–866. doi: 10.1002/art.24585
Zhao, H., Nettleton, D., Soller, M., and Dekkers, J. C. M. (2005). Evaluation of linkage disequilibrium measures between multi-allelic markers as predictors of linkage disequilibrium between markers and QTL. Genet. Res. 86, 77–87. doi: 10.1017/S001667230500769X
Zhou, X., Wang, J., Zou, H., Ward, M. M., Weisman, M. H., Espitia, M. G., et al. (2014). MICA, a gene contributing strong susceptibility to ankylosing spondylitis. Ann. Rheum. Dis. 73, 1552–1557. doi: 10.1136/annrheumdis-2013-203352
Keywords: ankylosing spondylitis, HLA-B∗27 positive, HLA-A∗24:02, sex biased, major histocompatibility complex, HLA allele typing
Citation: Nordin J, Pettersson M, Rosenberg LH, Mathioudaki A, Karlsson Å, Murén E, Tandre K, Rönnblom L, Kastbom A, Cedergren J, Eriksson P, Söderkvist P, Lindblad-Toh K and Meadows JRS (2021) Association of Protective HLA-A With HLA-B∗27 Positive Ankylosing Spondylitis. Front. Genet. 12:659042. doi: 10.3389/fgene.2021.659042
Received: 26 January 2021; Accepted: 09 June 2021;
Published: 15 July 2021.
Edited by:
Nelson L. S. Tang, The Chinese University of Hong Kong, ChinaReviewed by:
Eric Spierings, Utrecht University, NetherlandsJamie Duke, Children’s Hospital of Philadelphia, United States
Copyright © 2021 Nordin, Pettersson, Rosenberg, Mathioudaki, Karlsson, Murén, Tandre, Rönnblom, Kastbom, Cedergren, Eriksson, Söderkvist, Lindblad-Toh and Meadows. This is an open-access article distributed under the terms of the Creative Commons Attribution License (CC BY). The use, distribution or reproduction in other forums is permitted, provided the original author(s) and the copyright owner(s) are credited and that the original publication in this journal is cited, in accordance with accepted academic practice. No use, distribution or reproduction is permitted which does not comply with these terms.
*Correspondence: Jennifer R. S. Meadows, Jennifer.Meadows@imbim.uu.se