- 1Department of Laboratory Medicine, General Hospital of Southern Theatre Command of PLA, Guangzhou, China
- 2The First College of Clinical Medical, South Medical University, Guangzhou, China
- 3IT and Cloud Computing Center, Geneplus-Shenzhen, Shenzhen, China
Background: Translocase of inner mitochondrial membrane 17A (TIMM17A) is overexpressed in breast cancer (BRCA), and upregulation can increase the aggressiveness of BRCA cells. This study examined the influence of the TIMM17A gene network on BRCA outcome.
Methods: Expression levels of TIMM17A were compared between normal and tumor tissues from the OncomineTM database, and the association with patient survival was analyzed using Kaplan–Meier Plotter. Clinical factors influencing TIMM17A expression were studied by UALCAN. cBioPotal was then used to identify genes interacting with TIMM17A, and network relationships were assessed using the R clusterProfiler package. The association between TIMM17A mutation and mRNA expression in BRCA was examined using the LinkFinder application in LinkedOmics, and coexpressed genes were assessed for functional enrichment using the LinkInterpreter application. Furthermore, TIMM17A expression correlation with cell cycle phase distribution was performed by flow cytometry. Finally, the target networks of kinases, microRNAs (miRNAs), and transcription factors were identified using GeneMANIA. The expression and correlation of potential miRNAs and targets were further validated in BRCA cell lines by qRT-PCR.
Results: Expression of TIMM17A was significantly elevated in BRCA compared with normal tissue (p < 0.05), and overexpression was associated with both poor overall survival (OS) and shorter distant metastasis-free survival (DMFS) (p < 0.05). Expression of TIMM17A was not associated with age, sex, BRCA subclass, clinical stage, or patient ethnicity. The coexpressed TIMM17A network was enriched in genes targeted by cell cycle regulators such as CDK1, miR-331, and E2F family transcription factors (FDR < 0.001). Furthermore, flow cytometry revealed a strong association between higher TIMM17A expression and faster cell cycle progression in these BRCA cell lines. In addition, expression of TIMM17A protein was correlated with CDK1 protein expression in BRCA cell lines as measured by western blotting.
Conclusion: Elevated TIMM17A expression accelerates the progression of BRCA, thereby reducing OS and DMFS. The TIMM17A-associated networks identified here provide clues to the molecular pathogenesis of BRCA and potential targets for BRCA treatment.
Introduction
According to recent global estimates, breast cancer (BRCA) is now the most frequent cause of cancer-related mortality among adult females (Bray et al., 2018). However, BRCA is a highly heterogeneous clinical entity, with multiple subtypes according to distinct histological features and treatment sensitivity profiles (Harbeck and Gnant, 2017; Yeo and Guan, 2017; Liang et al., 2020). This heterogeneity has also been confirmed at the gene expression level by high-throughput molecular profiling (Ahn et al., 2020; Krug et al., 2020). More precise delineation of these BRCA subtypes may eventually yield more efficacious individualized treatments (Zardavas et al., 2015). Currently, however, therapeutic options for many cancers are limited by our relatively poor understanding of the underlying molecular and cellular mechanisms (Garrido-Castro and Winer, 2018). Malignancy results from the disruption of multiple coordinated gene networks (Garrido-Castro and Winer, 2018), so elucidation of the gene network changes characterizing various BRCA subtypes may facilitate the design of patient-specific interventions (Garrido-Castro and Winer, 2018; Radvanyi, 2018; Zacharakis et al., 2018).
Translocase of inner mitochondrial membrane 17A (TIMM17A) is an essential component of the highly conserved TIM23 translocase complex involved in mitochondrial protein import and metabolism (Bomer et al., 1996). Expression of TIMM17A is elevated in BRCA (Kannangai et al., 2007; Yang et al., 2016). Furthermore, TIMM17A upregulation can increase the aggressiveness of BRCA cells (Yang et al., 2016) and is associated with poor clinical outcome in human BRCA patients (Salhab et al., 2012). Indeed, Xu at el. identified TIMM17A as a biomarker for poor outcome from BRCA (Xu et al., 2010; Salhab et al., 2012).
All genes operate within multiple networks of coregulated and functionally related genes. However, TIMM17A networks are not well described. In this study, we examined the associations between clinical outcome measures and TIMM17A expression levels among BRCA cases from The Cancer Genome Atlas (TCGA). Furthermore, we constructed a network of genes coregulated with TIMM17A in BRCA patients to reveal new potential molecular targets for diagnosis and treatment.
Materials and Methods
Statistical Analysis
Statistical analyses were performed using SPSS Software version 26.0 (IBM SPSS Statistics, Chicago, IL, United States) and GraphPad Prism 7 (GraphPad Software, San Diego, CA, United States). Overall survival (OS) was compared between BRCA patients with high or low TIMM17A expression by the Kaplan–Meier method and log-rank test (Mishra et al., 2019; Okano et al., 2019). The associations between TIMM17A expression levels and various clinicodemographic features were analyzed by Spearman’s correlations. Group means were compared by independent samples t-test (Chandrashekar et al., 2017). The association between TIMM17A mutation and mRNA expression in the TCGA BRCA cohort was assessed by t-test with correction for multiple comparisons (Vasaikar et al., 2018). p-Value less than 0.05 (two-tailed) was considered significant for all tests.
Oncomine Analysis
Differences in TIMM17A expression and copy number between normal tissue and BRCA were evaluated for TCGA and Curtis datasets in the Oncomine database1.
Kaplan–Meier Plotter Analysis
The association of tumor TIMM17A expression level with BRCA outcome was examined in total BRCA datasets, GSE45255, and GSE7390 using Kaplan–Meier Plotter (publicly available at https://kmplot.com/). For this analysis, we chose TIMM17A gene chip results (Affymetrix ID 215171_s_at) for estimation of mRNA expression level, both OS and distant metastasis-free survival (DMFS) as clinical outcome metrics, and a follow-up duration of 120 months.
UALCAN Analysis
Clinical factors influencing TIMM17A expression were examined in the TCGA dataset using the ‘‘breast invasive carcinoma’’ section of UALCAN2. The influencing factors assessed were age, sex, tumor stage, BRCA subclass, and metastasis occurrence based on a previous factor analysis (Chandrashekar et al., 2017).
c-BioPortal Analysis
Genes interacting with TIMM17A were identified in the “breast invasive carcinoma” dataset of Firehose Legacy using c-BioPortal3. Neighboring genes with alteration frequencies greater than 5% were selected for network analysis.
clusterProfiler Analysis
The R 3.6.0 package cluster Profiler was used to visualize networks of genes related to functional changes identified by Disease Ontology (DO), Gene Ontology (GO), Kyoto Encyclopedia of Genes and Genomes (KEGG), and Reactome (Hayes, 2010). DO was used to identify genes associated with BRCA, GO to describe gene functions using standardized terminology, and KEGG to place genes into the following molecular interaction and reaction networks: metabolism, genetic information processing, environmental information processing, cellular processes, biological system, human diseases, and drug development. Reactome was used to supplement the results of KEGG analysis by visualizing various human reactions and biological channels. The Benjamini–Hochberg (BH) adjusted p method was used to determine significant enrichment at a cutoff of 0.05.
LinkedOmics Analysis
The RNAseq dataset of the ‘‘breast invasive carcinoma’’ cohort was obtained from LinkedOmics4 (Vasaikar et al., 2018). The dataset including TIMM17A expression comprised 1,093 patients. LinkFinder was used to identify the association between TIMM17A mutation and mRNA expression in the TCGA BRCA cohort and Pearson’s correlation analysis to assess the strengths of the associations (positive and negative). Kinase and KEGG pathway analyses were then performed using the gene set enrichment analysis (GSEA) tool in LinkInterpreter.
KEGG Pathway Analysis
KEGG is composed of 15 comprehensive database resources, a manually planned database, and four system information category databases generated by calculation. The KEGG pathway system can link gene expression to multiple “cells and biological functions” domains, including “metabolism,” “cellular processes,” “biological functions,” and “human diseases” (Kanehisa et al., 2017). We identified the pathways for 26 enriched neighboring genes by KEGG5.
GeneMANIA Analysis
Protein--protein interaction (PPI) networks were constructed using GeneMANIA6 (Warde-Farley et al., 2010). For each network, we examined “physical interactions,” “coexpression,” predicted, “colocalization,” “pathway,” “genetic interactions,” and “shared protein domains.” The classified function were “protein targeting,” “mitochondrial transport,” “establishment of protein localization to mitochondrion,” “protein localization to mitochondrion,” “protein targeting to mitochondrion,” and (or) “mitochondrion organization.”
Cell Culture
The human BRCA cell lines BT-549 and SK-BR-3 were obtained from the Shanghai Cell Bank Type Culture Collection Committee (Shanghai, China). Cells were cultured in complete growth medium as recommended by the distributor in a humidified incubator at 37°C under a 5% CO2 atmosphere.
Cell Transfection
Expression of TIMM17A in cancer cell lines was manipulated by transfection with small interfering RNAs (siRNAs). A negative control siRNA (si-NC) and TIMM17A-targeting siRNA (si-TIMM17A) were designed and synthesized by LAIDEMENG (Guangzhou, China). Cell lines in logarithmic growth phase were seeded at 6 × 105 cells/well in six-well plates, allowed to adhere overnight, and then incubated in transfection medium containing the indicated siRNA and Lipofectamine 3000 (Invitrogen, Waltham, MA, United States) according to the standard protocol of the manufacturer. After 6 h, the transfection medium was exchanged for complete medium and cells incubated for another 48 h to allow expression changes before subsequent experiments.
Quantitative RT-PCR
Total RNA was extracted from cells using Trizol reagent (Invitrogen, CA, United States) according to the instructions of the manufacturer, and cDNAs generated using EvoM-MLV RT Premix. Gene expression levels were then measured by quantitative PCR on a Bio-Rad CFX RT-qPCR detection system using SYBR Premix Ex TaqII (Takara, Dalian, China). The 18S rRNA gene was used as an internal control. All samples were run in triplicate. Fold changes in gene expression were calculated using the ΔΔCt method. Details of the primer sequences used for qPCR are listed in Supplementary Table 8.
Western Blotting
Whole cell extracts were prepared by incubation of harvested cells in ice-cold lysis buffer containing 1:100 PMSF and 1:100 protease inhibitor cocktail for 30 min. Lysates were then centrifuged at 14,000 rpm for 5 min at 4°C, and the supernatant was collected and stored at −80°C. Lysates were mixed with 5 × THE buffer at 4:1 (vol/vol), boiled for 10 min, cooled slowly to room temperature, centrifuged briefly, and stored at 20°C. Proteins were separated by sodium dodecyl sulfate polyacrylamide gel electrophoresis and electro-transferred (100 V at low temperature, 1 min/kDa) to polyvinylidene fluoride (PVDF) membranes pretreated with methanol for 5∼30 s, and transmembrane buffer for 20 min. Blotted membranes were then rinsed with Tris-buffered saline containing Triton-X (TBST) for 5 min, incubated in TBST with 5% skimmed milk powder rinsed in TBST for 8 min, and incubated in anti-TIMM17A, anti-CDK1, or anti-GAPDH (gel-loading control) at 4°C overnight. Immunoblotted membranes were then incubated with the indicated secondary antibody at 37°C for 50 min to 3 h, and washed three times in TBST (8 min/wash). Protein bands were visualized with chemiluminescence substrate (1∼5 min) and captured using MiniChemi imaging system (Bio-OI, Beijing, China).
Flow Cytometry
The cell cycle progression of cancer cell lines was evaluated using a PI cell cycle Kit. Cells were harvested by trypsin digestion, washed twice in PBS, and fixed in 75% precooled ethanol for more than 2 h. Fixed cells were then treated with kit reagents according to the manufacturer’s protocol and analyzed using FACSCantoll flow cytometer (BD FACSCanto II, China).
Results
TIMM17A Expression in BRCA
We obtained multiple TIMM17A expression datasets from Oncomine 4.5 databases (Rhodes et al., 2004). Both mRNA expression level and DNA copy number variation (CNV) were significantly higher in BRCA tissues than healthy breast tissues (p < 0.05) (Figures 1A–F). Although the fold changes were less than 2, TIMM17A ranked within the top 15% of differentially expressed genes based on mRNA abundance and within the top 1% based on DNA CNV (Figures 1A–F). Kaplan–Meier survival analysis using Kaplan–Meier Plotter revealed that higher TIMM17A expression was associated with poorer OS and shorter distant metastases-free survival (DMFS) among 1,402 BRCA cases from the Kaplan–Meier Plotter database (p < 0.05), and similar results were observed for GSE45255 and GSE7390 cohorts (Figures 2A–F). Among these patients, 1,402 died due to BRCA within 120 months, and patients with high expression of TIMM17A demonstrated worse clinical outcome. We also evaluated TIMM17A transcription levels in the Oncomine database, which again revealed that TIMM17A expression was higher in BRCA patients than healthy controls. In contrast to cancer status, TIMM17A expression was not associated with sex, age, ethnicity, tumor stage, tumor grade, or BRCA subtype in the UALCAN database (Figures 3A–F). Thus, TIMM17A expression level may serve as a prognostic indicator of BRCA.
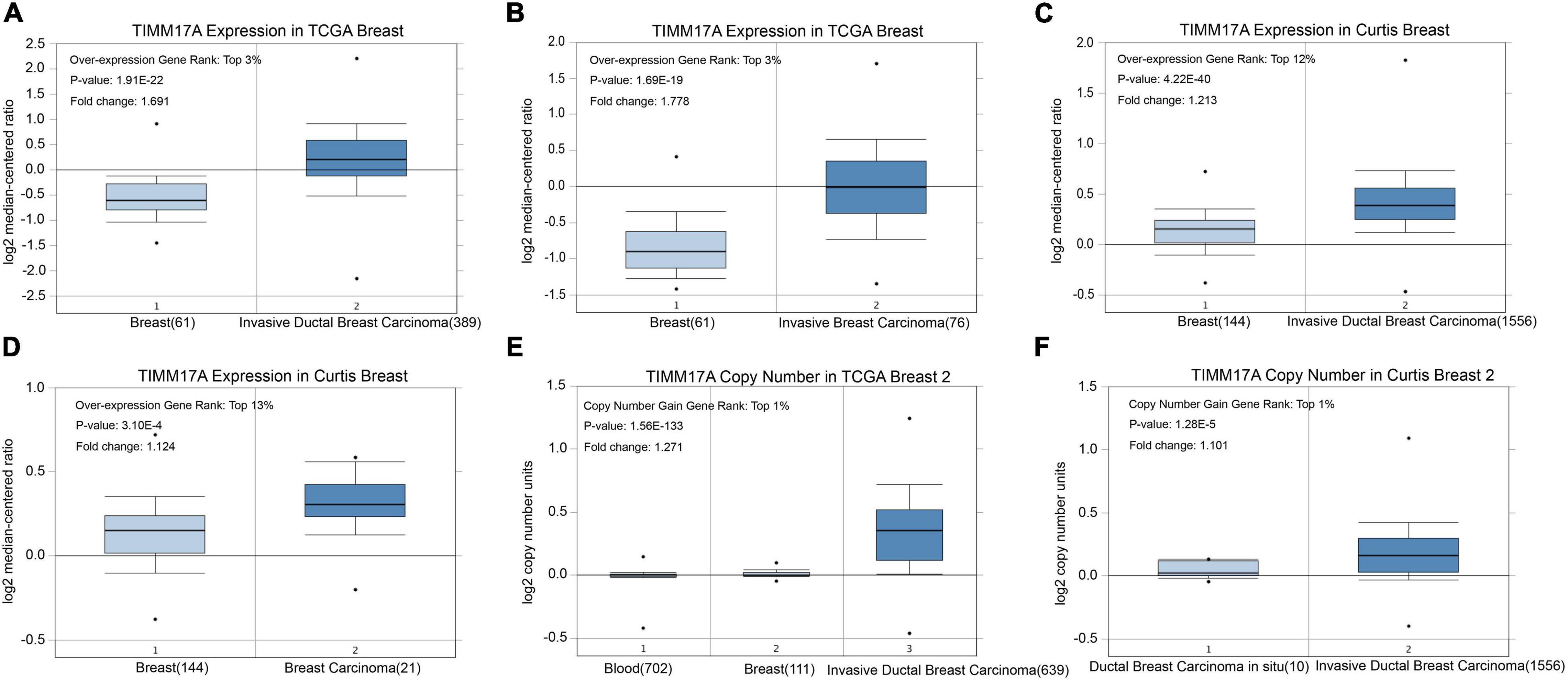
Figure 1. TIMM17A transcription in breast carcinoma (OncomineTM). Levels of TIMM17A mRNA and DNA copy number were significantly higher in breast carcinoma than in normal tissue. Shown are fold change, associated p values, and overexpression rank based on OncomineTM analysis. (A–D) Box plots showing TIMM17A mRNA levels for The Cancer Genome Atlas (TCGA) Invasive Ductal Breast Carcinoma, TCGA Invasive Breast Carcinoma, Curtis Invasive Ductal Breast Carcinoma, and Curtis Breast Carcinoma datasets, respectively. (E,F) Box plots showing TIMM17A copy number in TCGA Breast 2 and Curtis Breast 2 datasets, respectively.
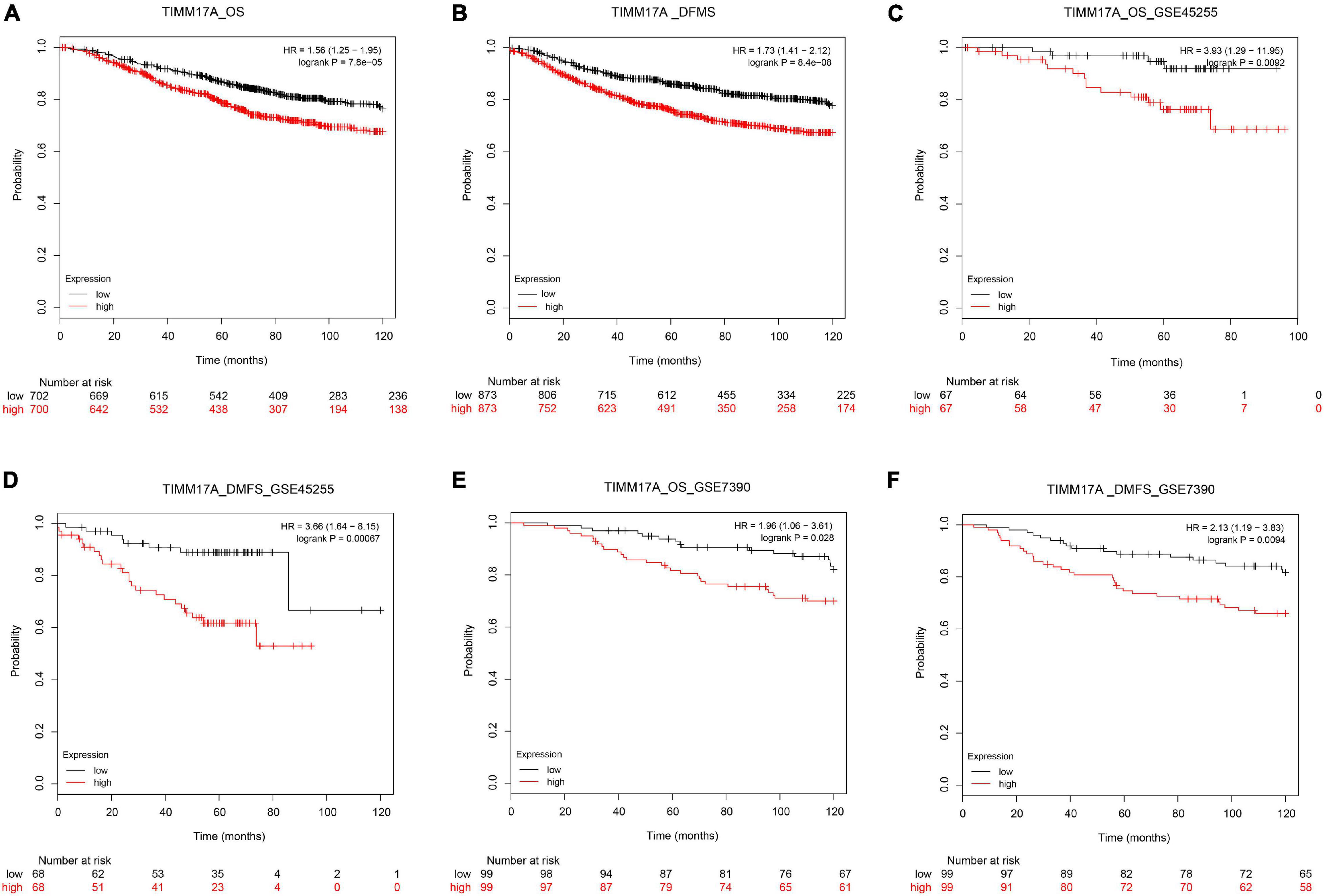
Figure 2. Kaplan–Meier survival curves showing significantly reduced overall survival (OS) and shorter distant metastases-free survival (DMFS) among breast cancer patients with high levels of TIMM17A expression. Clinical survival outcomes of breast cancer patients with high or low expression levels of TIMM17A. (A) OS in TCGA cohorts. (B) DMFS in TCGA cohorts. (C) OS in the GSE45255 cohort. (D) DMFS in the GSE45255 cohort. (E) OS in the GSE7390 cohort. (F) DMFS in the GSE7390 cohort. Plots are truncated at 10 years.
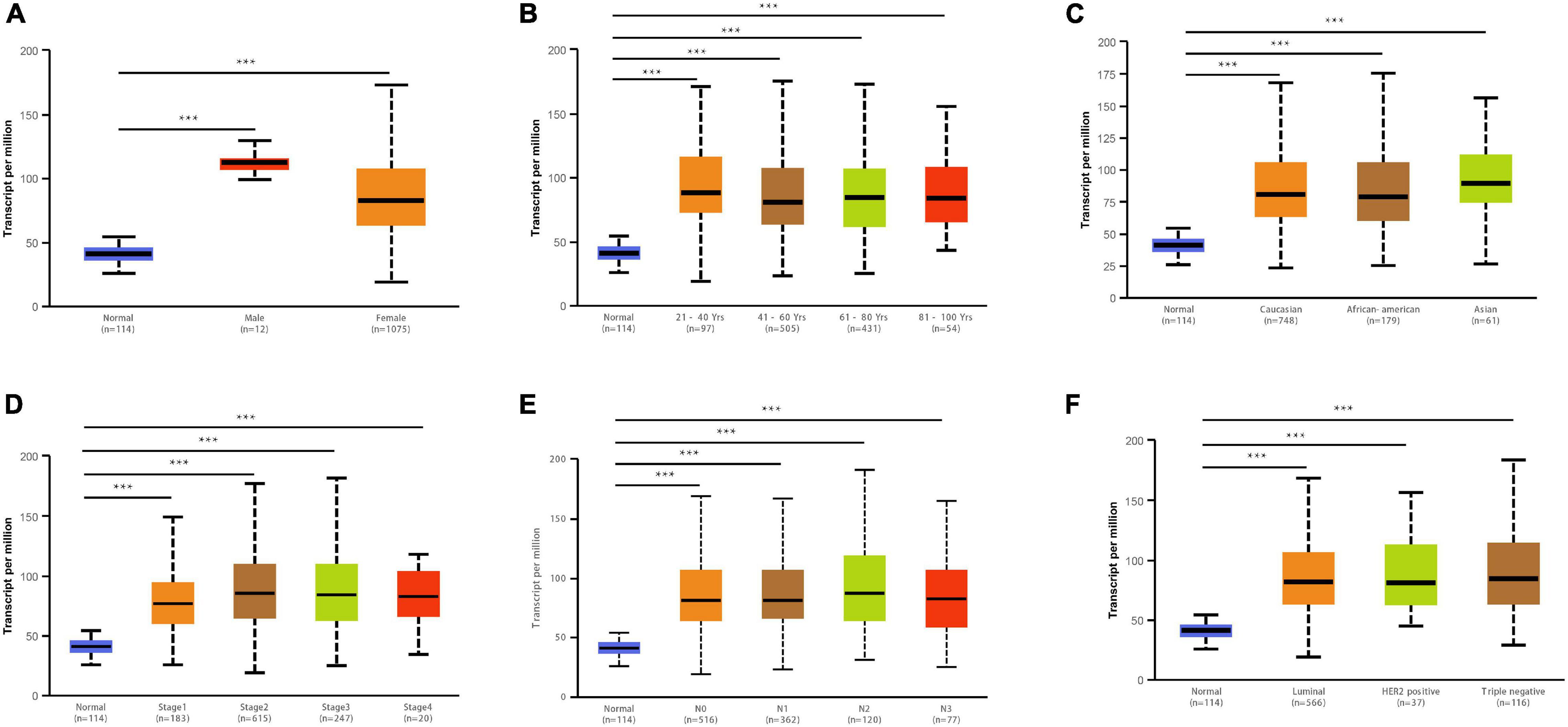
Figure 3. TIMM17A transcription in breast cancer (BRCA) patient subgroups stratified by sex, age, ethnicity, cancer stage, and metastasis (UALCAN). (A) Boxplot showing relative expression of TIMM17A in normal individuals of either sex and separately for male and female BRCA patients. (B) Boxplot showing relative expression of TIMM17A in normal individuals of any age and separately for BRCA patients aged 21–40, 41–60, 61–80, or 81–100 years. (C) Boxplot showing relative expression of TIMM17A in normal individuals of any ethnicity and separately for BRCA patients of Caucasian, African-American, or Asian ethnicity. (D) Boxplot showing relative expression of TIMM17A in normal individuals and separately for BRCA patients in stages 1, 2, 3, or 4. (E) Boxplot showing relative expression of TIMM17A in normal individuals and separately for BRCA patients with regional lymph node metastasis N0, N1, N2, or N3 tumors. (F) Boxplot showing relative expression of TIMM17A in normal individuals and separately for BRCA patients with regional lymph node metastasis N0, N1, N2 or N3 tumors. Data are mean ± SE. *P < 0.05; **P < 0.01; ***P < 0.001.
Genomic Alterations of TIMM17A in BRCA
Frequencies and Types of TIMM17A Alterations
The TCGA Firehose Legacy database from cBioPortal was used to assess the types and frequencies of TIMM17A alterations in BRCA (Gao et al., 2013). Among the 1,093 samples, TIMM17A was altered in 153 cases (14%) (Figure 4A). The most common alteration was amplification (111 cases, 10.16%), followed by multiple alterations (13 cases, 1.19%), and mutation only (two cases, 0.18%), while there were no samples showing mRNA upregulation (Table 1).
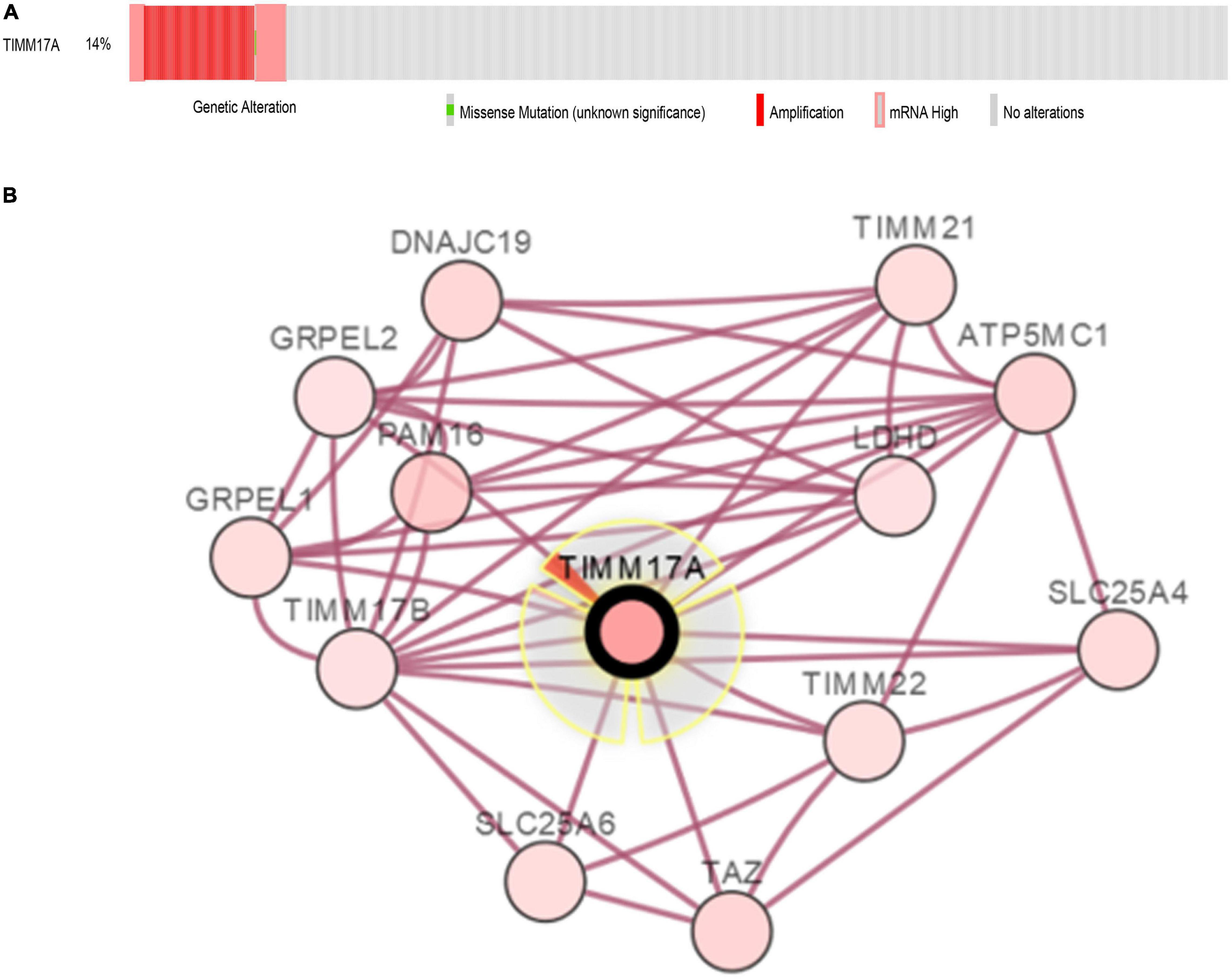
Figure 4. Visual summary of TIMM17A alterations and biological interaction networks in breast carcinoma (cBioPortal). (A) OncoPrint of TIMM17A alterations in BRCA. The OncoPrint provides an overview of genomic alterations in TIMM17A affecting individual samples (columns) in BRCA from the TCGA dataset. The different types of genetic alterations are indicated by unique colors. (B) Network view of the TIMM17A neighborhood in BRCA. TIMM17A is the seed gene (indicated with thick border), and all other genes altered in BRCA are automatically identified Darker red indicates increased alteration frequency in BRCA. The interaction types are derived from the Biological Pathway Ex-change (BioPAX). The red connection indicates proteins are members of the same complex.
Biological Interaction Networks Associated With TIMM17A Alterations in BRCA
To construct a biological interaction network for TIMM17A in BRCA, we obtained neighbor genes with expression alterations at frequencies >5% using the Network function of cBioPortal (Figure 4B and Table 1), which retrieves networks of queried genes from public pathway databases, such as the Human Reference Protein Database, Reactome, and Memorial Sloan-Kettering Cancer Center Cancer Cell Map (Tung et al., 2016). Neighbor genes with the highest alteration frequencies were PAM16 (8.78%), ATP5MC1 (7.5%), and TAZ (7.04%). The 50 most frequently altered neighbor genes were then subjected to GO analysis, which indicated that this group was enriched in genes related to “mitochondrial transport” and “mitochondrial protein import” (Figures 5A–D).
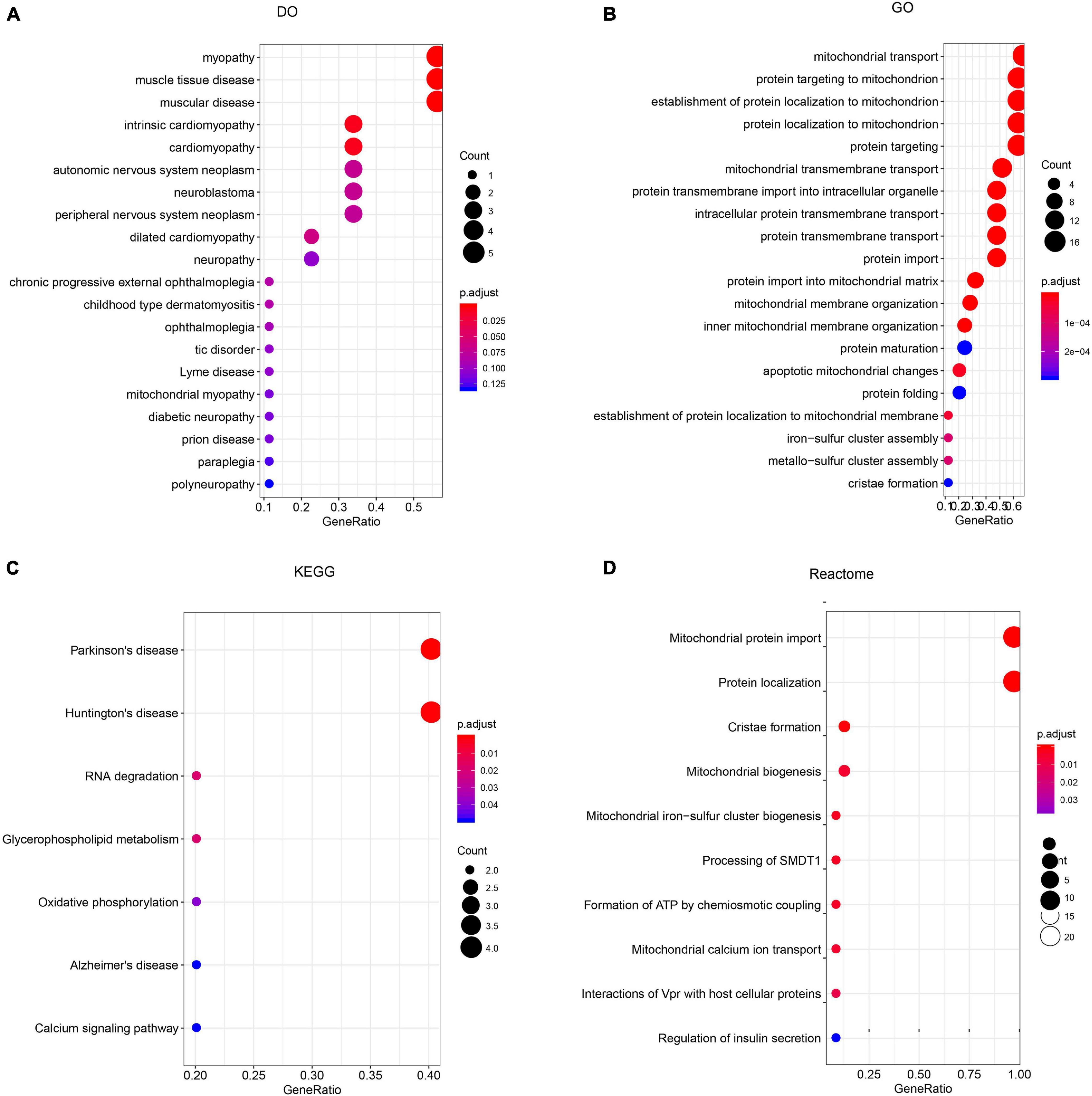
Figure 5. Enrichment analysis of TIMM17A neighbor genes altered in breast carcinoma. The bubble diagrams display the enrichment results of the top 50 TIMM17A neighbor genes altered in BRCA. (A) Disease Ontology (DO). (B) Gene ontology (GO). (C) Kyoto Encyclopedia of Genes and Genomes (KEGG). (D) Reactome analysis.
Enrichment Analysis of TIMM17A Functional Networks in BRCA
GO and KEGG Pathway Analysis of mRNAs Co-regulated With Mutant TIMM17A in BRCA
We then identified mRNAs demonstrating correlated expression with mutant TIMM17A in BRCA from TCGA using the Function module of LinkedOmics (Vasaikar et al., 2018). The volcano plot (Figure 6A) indicated that 7,811 genes (dark red dots) demonstrated mRNA expression levels that were positively correlated with mutant TIMM17A expression whereas 12,344 genes (dark green dots) demonstrated mRNA expression levels that were negatively correlated with mutant TIMM17A expression [false discovery rate (FDR) < 0.01]. The 50 significant gene sets exhibited both positive and negative correlations with TIMM17A, as shown by a heat map (Figures 6B,C). Thus, TIMM17A alterations in BRCA have widespread effects on the transcriptome. The statistical scatter plots for individual genes are shown in Supplementary Figures 1A–E. TIMM17A mutation was strongly and positively correlated with expression of UBE2T [positive rank #1, Pearson’s correlation (r) = 0.650, p = 5.20779257291395e−132], TMEM183A (r = 0.648, p = 2.22E−131), CACYBP (r = 0.628, p = 5.14E−121), SNRPE (r = 0.644, p = 6.90E−129), and RABIF (r = 0.623, p = 9.86E−120). GO analysis indicated that these networks are significantly enriched in genes with functions related to “mitochondrial inner membrane,” “mitochondrial protein complex,” and “chromosomal region.” Furthermore, these genes contribute to “non-coding (nc)RNA processing,” “chromosome segregation,” and “rRNA metabolic process,” and act on “RNA and structural constituents of ribosome” (Figures 7A–C and Supplementary Tables 1–3). KEGG pathway analysis indicated that these genes are related to “oxidative phosphorylation,” “spliceosome,” and “ribosome” pathways (Figures 7D,E and Supplementary Table 4). Thus, genes coregulated with mutant TIMM17A in BRCA participate extensively in “oxidative phosphorylation” and “cell cycle regulation.” To further validate this network-level relationship between TIMM17A and cell cycle regulator, we examined the association of TIMM17A expression with cell cycle phase distribution in BRCA cell lines by flow cytometry. The proportions of cells in S-phases were reduced by TIMM17A knockdown (Figures 8A–E), suggesting that TIMM17A serves to enhance cancer cell proliferation.
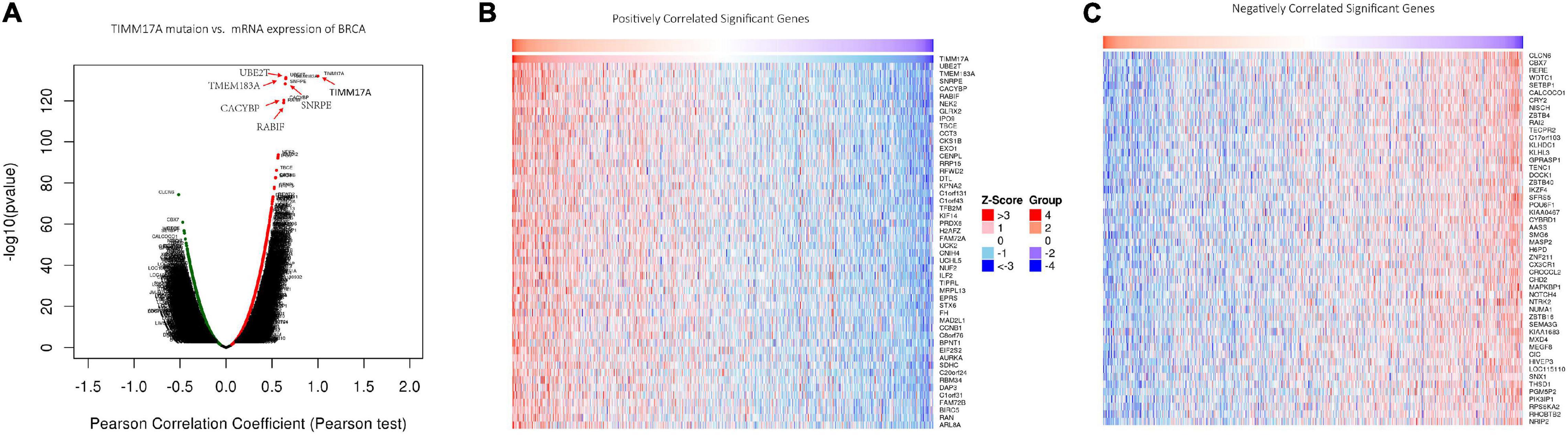
Figure 6. Genes differentially expressed in correlation with TIMM17A expression among breast carcinoma patients (LinkedOmics). (A) Pearson’s correlation analyses to assess the association between TIMM17A expression and that other genes differentially expressed in BRCA. (B,C) Heat maps showing genes positively and negatively correlated with TIMM17A expression in BRCA (TOP 50). Red indicates positively correlated genes and green indicates negatively correlated genes.
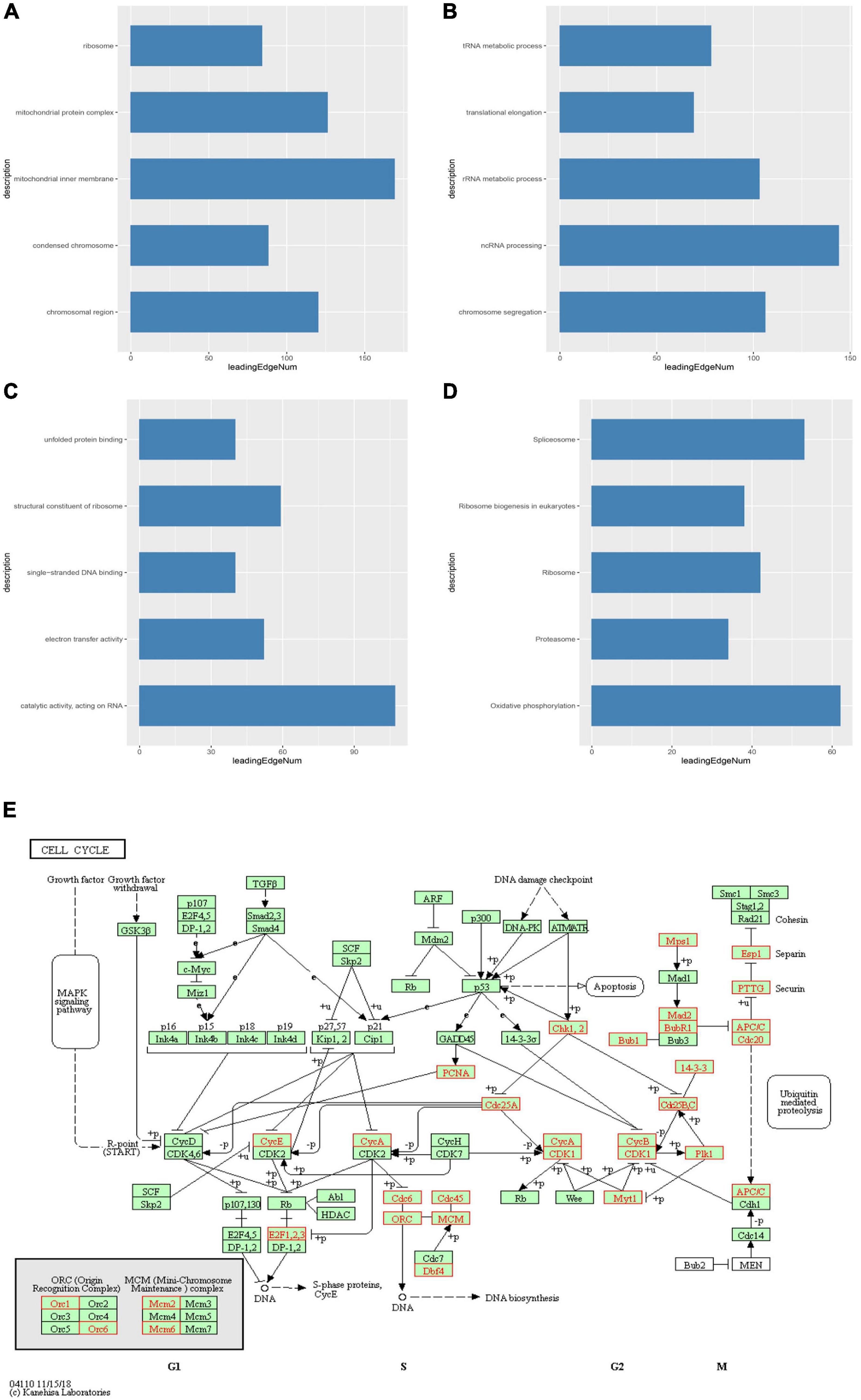
Figure 7. Significantly enriched GO annotations and KEGG pathways of TIMM17A network genes in breast carcinoma. GO annotations and KEGG pathways of genes with expression levels associated with TIMM17A expression in BRCA analyzed using GSEA. (A) Cellular components. (B) Biological processes. (C) Molecular functions. (D) KEGG pathway analysis. The blue column represents –log10(p). (E) KEGG pathway annotations of the cell cycle pathway. Red marked nodes are associated with the LeadingEdgeGene.
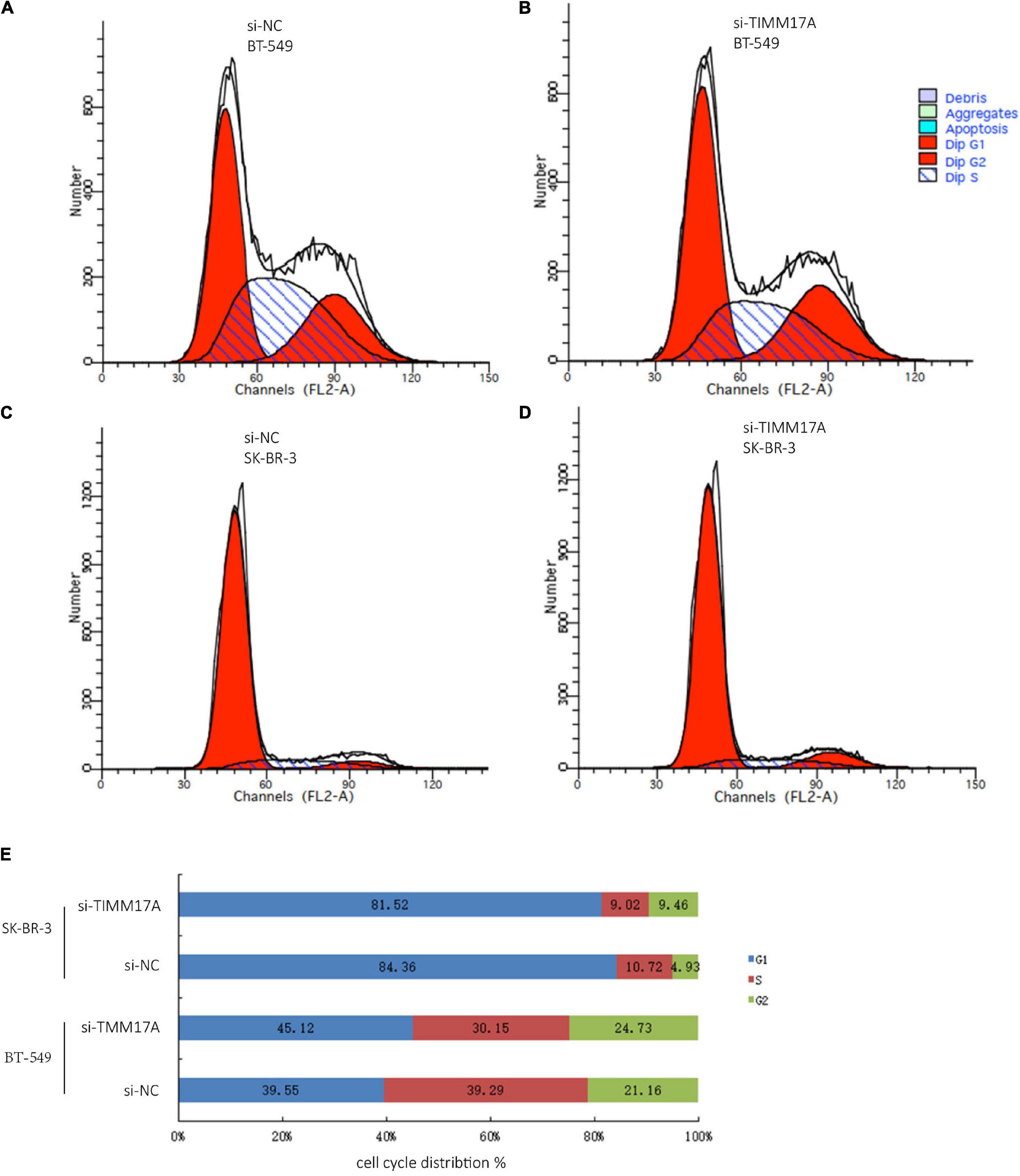
Figure 8. Cell cycle analysis of BRCA cells. (A) Cell cycle analysis of BT-549 cells by flow cytometry. (B) Cell cycle analysis of TIMM17A knockdown BT-549 cells by flow cytometry. (C) Cell cycle analysis of SK-BR-3 cells by flow cytometry. (D) Cell cycle analysis of TIMM17A knockdown SK-BR-3 cells by flow cytometry. (E) Cell cycle distribution of siRNA-SK-BR-3, NC-SK-BR-3, siRNA-BT-549 and NC-BT-549.
Kinases, miRNAs, and Transcription Factors Targeting TIMM17A-Associated Genes in BRCA
Finally, we analyzed the kinases, microRNAs (miRNAs), and transcription factors positively correlated with TIMM17A expression in BRCA by LinkedOmics. The top five most strongly associated kinases were cyclin-dependent kinase 1 (CDK1), polo-like kinase 1 (PLK1), aurora kinase B (AURKB), cyclin-dependent kinase 2 (CDK2), and checkpoint kinase 1 (CHEK1) (Table 2 and Supplementary Table 5). The strongly associated miRNAs included CCAGGGG (MIR-331), GAGCCTG (MIR-484), GACAATC (MIR-219), CCCAGAG (MIR-326), and AGCTCCT (MIR-28) (Table 2 and Supplementary Table 6). The associated transcription factors were mainly of the E2F transcription factor (E2F) family, including E2F_Q6, E2F1_Q6, E2F1DP2_01, E2F_02, and GGAANCGGAANY (Table 2 and Supplementary Tables 5–7). The protein–protein interaction network constructed by GeneMANIA revealed many correlations with CDK1, miRNA-331, and TF E2F_Q6.
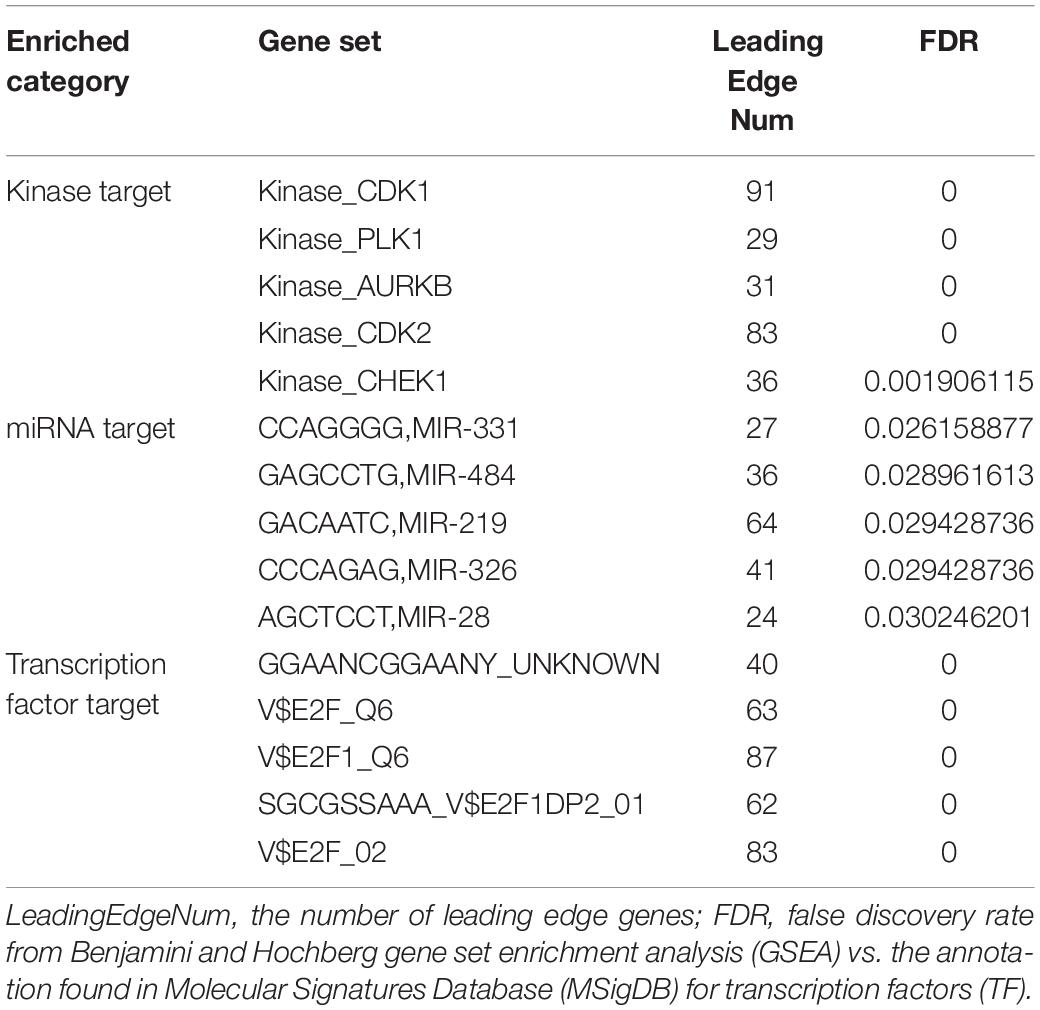
Table 2. Kinases, miRNAs, and transcription factors targeting TIMM17A-associated gene networks in breast carcinoma (LinkedOmics).
The gene set enriched in CDK1 targets is responsible mainly for regulating cell cycle G2/M-phase transition, cell division, organelle fission, and the cell cycle checkpoint (Figure 9). To validate the association between CDK1 and TIMM17A, we analyzed the correlation between TIMM17A expression and CDK1 expression both in silico and in BRCA cell lines. Expression of CDK1 was positively correlated with TIMM17A expression in theUALCAN database (Pearson’s r = 0.32) (Figure 10A). Furthermore, western blotting revealed that TIMM17A knockdown reduced CDK1expression in BRCA cell lines (Figure 10B). The gene set enriched in miRNA-331 targets is involved mainly in regulation of cell-cycle adhesion (Table 2 and Supplementary Figure 2), so we also examined the correlation between TIMM17A and miRNA expression in BRCA cell lines. Indeed, TIMM17A knockdown altered the expression level of miRNA331 as well as expression of miRNA219 and miRNA 326 in BRCA cell lines (Figures 10C,D). Similarly, the gene set enriched in transcription factor E2F_Q6 targets is involved mainly in regulation of DNA replication, cell cycle checkpoint, and mitotic cell cycle (Supplementary Figure 3).
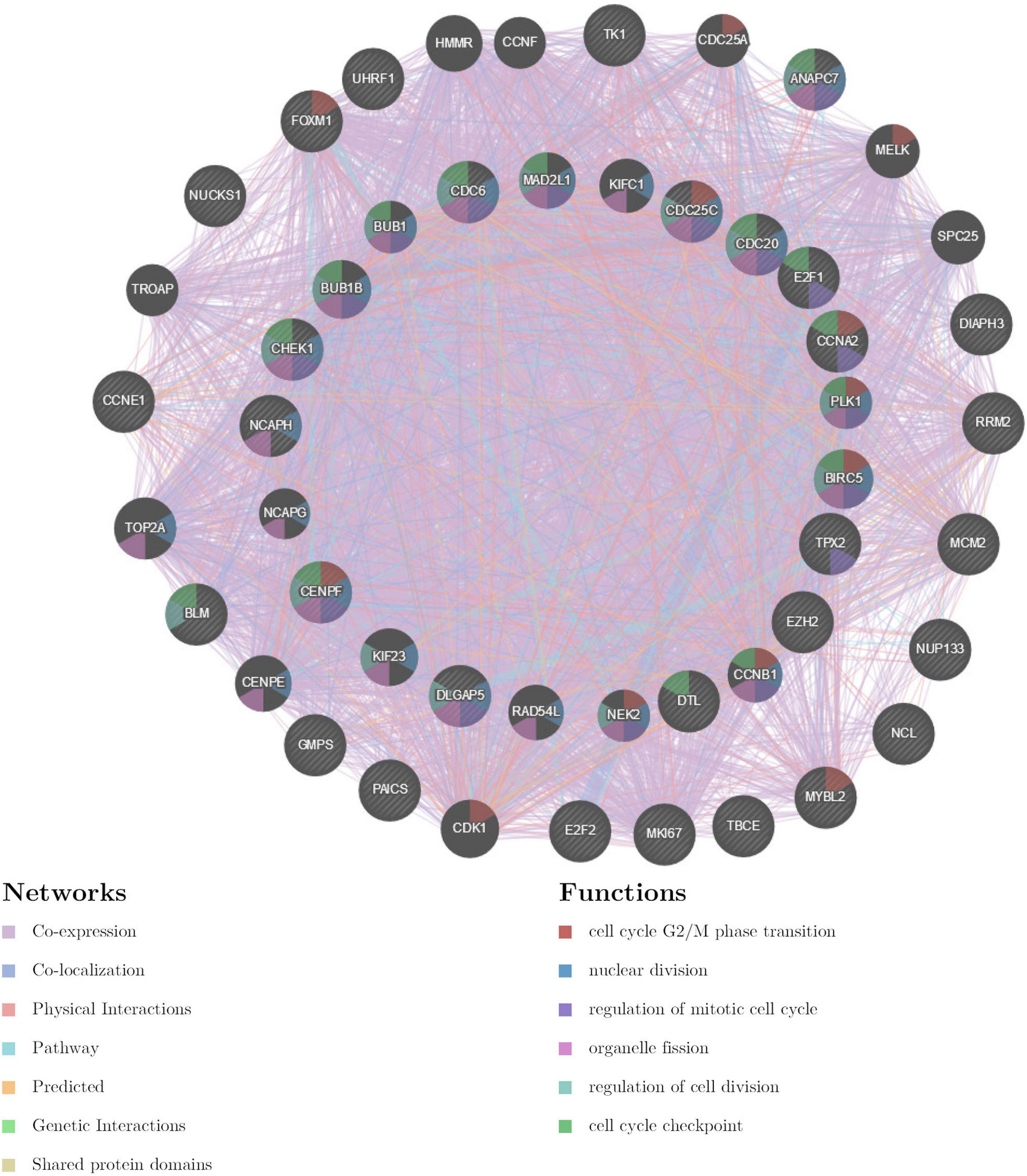
Figure 9. Protein–protein interaction network of ATR kinase targets (GeneMANIA). Protein–protein interaction (PPI) network and functional analysis of the gene set enriched in targets of CDK1. Different colors of the network edge indicate the bioinformatics methods applied: co-expression, co-localization, physical interactions, pathway, predicted, genetic interactions, and shared protein domains. The different colors for the network nodes indicate the biological functions of the set of enrichment genes.
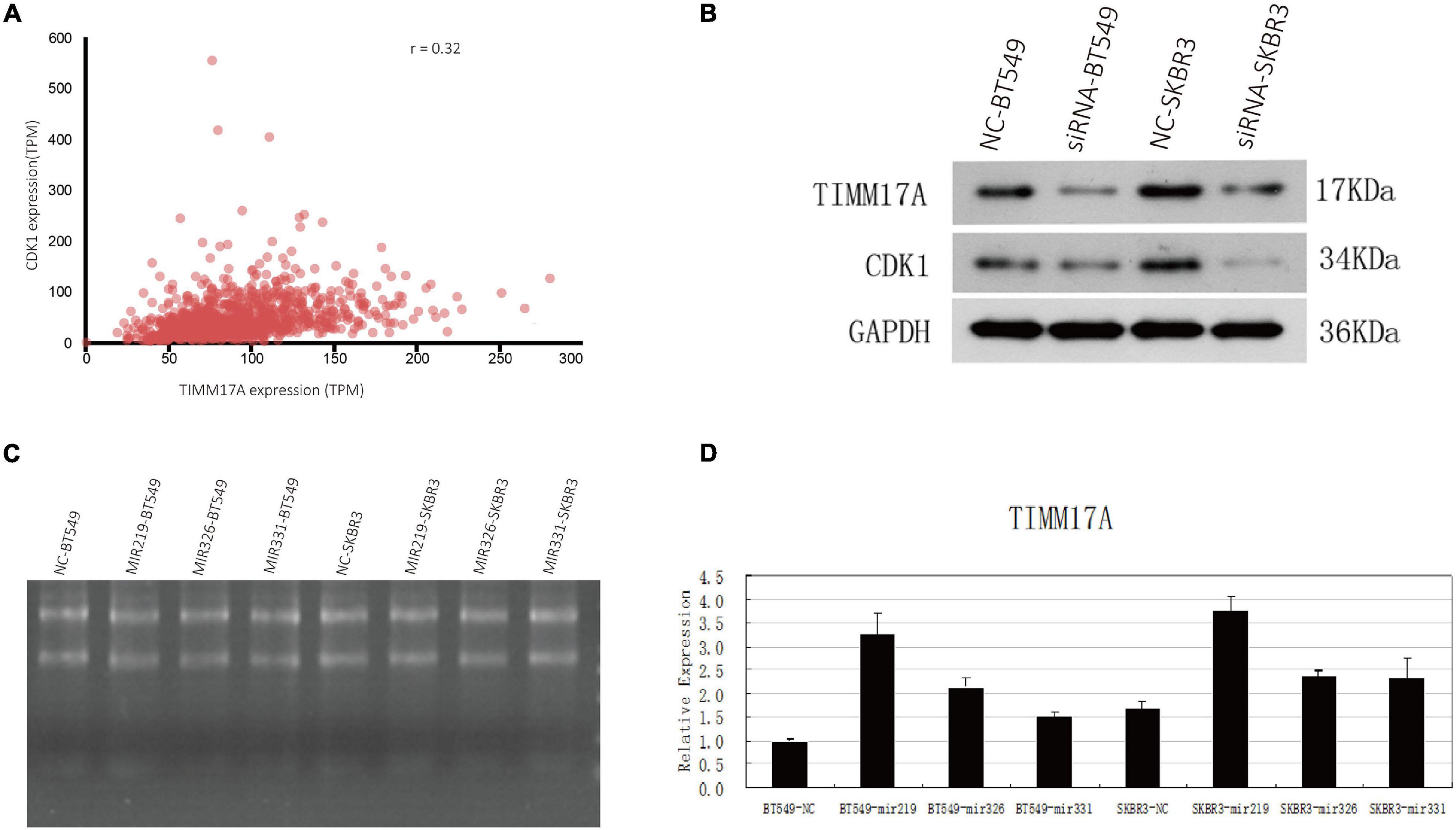
Figure 10. Associations of TIMM17A expression with CDK1 expression and microRNAs expression levels. (A) Relationship between TIMM17A and CDK1 expression levels. (B) Western blot analysis showing the effects of TIMM17A knock down on the expression levels of CDK1 and GAPDH. (C) RNA electrophoretogram of miRNA 219, miRNA 326, and miRNA 331 in BRCA cells. (D) Expression levels of miRNA 219, miRNA 326 and miRNA 331 in TIMM17A knockdown BRCA cells.
Discussion
Most BRCA cases are caused by a germline mutation in a single gene or multiple genes, of which the most frequent are in BRCA1, BRCA2, and p53 (Tung et al., 2016). Our analysis revealed an inverse association between TIMM17A expression level and both OS and DFMS. About 90% of all tumor recurrences and metastases occur within 10 years of first diagnosis, so we chose 10 years as the limit for survival analyses. Surprisingly, survival analyses of multiple public datasets for this period revealed that patients with high expression of TIMM17A have worse clinical outcome. Further subgroup analysis of multiple clinicopathological features revealed that TIMM17A mRNA expression was higher in BRCA patients than healthy individuals but did not differ according to sex, age, ethnicity, tumor stage, tumor grade, or BRCA subtype. The TIMM17A copy number was also significantly higher in BRCA tissues than normal tissues, and amplification was the most common genomic alteration with a change in copy number >2.
Expression of mutant TIMM17A was strongly correlated with expression levels of UBE2T, TMEM183A, CACYBP, SNRPE, and RABIF (top 5). The functional network of TIMM17A in BRCA participates extensively in chromosome segregation, oxidative phosphorylation, and cell cycle regulation. Indeed, flow cytometry demonstrated that TIMM17A expression was strongly associated with cell cycle phase distribution in BRCA cell lines. Specifically, TIMM17A knockdown reduced the proportions of cells in S-phases, suggesting that TIMM17A enhances the rate of proliferation. Collectively, these results demonstrate that high TIMM17A expression enhances BRCA aggression, potentially by promoting fasting cell cycle progression.
LinkedOmics analysis identified numerous additional network components and other gene sets with high weight coexpression and protein interactions with TIMM17A. Genes coregulated with TIMM17A were enriched in cell cycle modulators and targets of CDK1, MIR-331, and E2F family transcription factors. Activation of CDK1 induces phosphorylation of lamin, disintegration of the nuclear fiber layer and nuclear membrane, phosphorylation of histone H1, and chromosome condensation (Haneke et al., 2020), processes required for cell division. The evolution of kinase signaling is promoted by gain or loss of phosphorylation sites in rapidly evolving regions (Holt et al., 2009). Ravindran Menon et al. (2018) found that tumor initiation is associated with regulation of transcription factor Sox2 activity by CDK1, suggesting the CDK1–Sox2 pathway as a potential therapeutic target. Mitotic chromosome segregation also depends on H2B serine 6 phosphorylation by CDK1 (Seibert et al., 2019). Unregulated CDK1-mediated phosphorylation allows the cell cycle to proceed unchecked, leading to tumor formation (Cheng et al., 2020). The current analysis identifies multiple additional CDK1-targeted pathways for potential therapeutic intervention. Moreover, we confirmed a strong correlation between TIMM17A and CDK1 in BRCA cells at both transcriptional and protein expression levels as evidenced by the marked CDK1 downregulation following TIMM17A knockdown.
Xuefang et al. (2020) reported that miR-331-3p promoted apoptosis of nasopharyngeal carcinoma cells by targeting the elf4B-PI3K-AKT pathway, and Chen et al. (2018) recently reported that miR-331 inhibits the proliferation and invasion of melanoma cells. At the beginning of S-phase, CDK2 combines with cyclin A to inactivate E2F transcription factors which is a precondition for the completion of S-phase, while persistent E2F activity leads to apoptosis (Krek et al., 1995; Fueyo et al., 1998; Lukas et al., 1999). Therefore, selective inhibition of CDK2/cyclin A may increase E2F expression, which in turn could lead to S-phase arrest or apoptosis. Transcription factor E2F1 (Schuldt, 2011) plays an important role in cell cycle control and tumor suppressor gene function, and it is also the target of transforming protein of small DNA oncoviruses (Krek et al., 1995; Chen et al., 2009). These E2F family members contain multiple highly conserved domains (Osorio, 2015; Kent and Leone, 2019), including a DNA-binding domain, dimerization domain interacting with transcription factor protein (DP) regulated by differentiation, an acid amino acid-rich transactivation domain, and a tumor suppressor protein-related domain within the transactivation domain. In addition, E2F proteins E2F2 and E2F3 have cyclin-binding domains. These proteins preferentially bind to retinoblastoma protein pRb in a cell cycle-dependent manner, allowing both cell proliferation and p53-dependent or p53-independent apoptosis (Osorio, 2015). These results provide potential directions for future research on the molecular mechanisms of BRCA.
Data Availability Statement
The original contributions presented in the study are included in the article/Supplementary Material, further inquiries can be directed to the corresponding author/s.
Author Contributions
JJC and CXW contributed to data analysis. JJC, JYC, and LH drafted the manuscript. LH and JJC performed the experiments. ZHS and QZ designed the experiments and revised the manuscript. All of the authors critically revised the manuscript, gave final approval of the version to be published, and agreed to be accountable for all aspects of the work.
Funding
This work was financially supported by a scholarship from the National Natural Science Foundation of China (31901035) and the Guangdong Province Foundation for Basic and Applied Basic Project (2020A1515010951).
Conflict of Interest
The authors declare that the research was conducted in the absence of any commercial or financial relationships that could be construed as a potential conflict of interest.
Publisher’s Note
All claims expressed in this article are solely those of the authors and do not necessarily represent those of their affiliated organizations, or those of the publisher, the editors and the reviewers. Any product that may be evaluated in this article, or claim that may be made by its manufacturer, is not guaranteed or endorsed by the publisher.
Acknowledgments
We would like to acknowledge the help from the staff at the General Hospital of Southern Theatre Command of PLA.
Supplementary Material
The Supplementary Material for this article can be found online at: https://www.frontiersin.org/articles/10.3389/fgene.2021.658154/full#supplementary-material
Supplementary Figure 1 | Correlations between TIMM17A expression and UBE2T, TMEM183A, SNRPE, CACYBP, and RABIF expression (LinkedOmics). The scatter plots show Pearson’s correlations between TIMM17A expression and the expression levels of UBE2T (A), TMEM183A (B), SNRPE (C), CACYBP (D), and RABIF (E).
Supplementary Figure 2 | Protein–protein interaction network of miRNA 331 targets (GeneMANIA). Protein–protein interaction (PPI) network and functional analysis of the gene set enriched in miRNA 331 targets. Different colors of the network edge indicate the bioinformatics method applied: co-localization, co-expression, shared protein domains, pathway, and genetic interactions. The different colors for the network nodes indicate the biological functions of the set of enrichment genes.
Supplementary Figure 3 | Protein–protein interaction network of transcription factor E2F_Q6 targets (GeneMANIA). Protein–protein interaction (PPI) network and functional analysis indicating the gene set enriched in transcription factor E2F_Q6 targets. Different colors of the network edge indicate the bioinformatics method applied: co-expression, physical interaction, pathway, co-localization, and predicted. The different colors for the network nodes indicate the biological functions of the set of enrichment genes.
Supplementary Table 1 | Significantly enriched GO annotations (cellular components) of TIMM17A in breast carcinoma (LinkedOmics).
Supplementary Table 2 | Significantly enriched GO annotations (biological processes) of TIMM17A in breast carcinoma (LinkedOmics).
Supplementary Table 3 | Significantly enriched GO annotations (molecular functions) of TIMM17A in breast carcinoma (LinkedOmics).
Supplementary Table 4 | Significantly enriched KEGG pathway annotations of TIMM17A in breast carcinoma (LinkedOmics).
Supplementary Table 5 | Significantly enriched kinase-target networks of TIMM17A in breast carcinoma (LinkedOmics).
Supplementary Table 6 | Significantly enriched miRNA-target networks of TIMM17A in breast carcinoma (LinkedOmics).
Supplementary Table 7 | Significantly enriched transcription factor-target networks of TIMM17A in breast carcinoma (LinkedOmics).
Supplementary Table 8 | The sequences of primers used for qPCR.
Footnotes
- ^ www.oncomine.org
- ^ http://ualcan.path.uab.edu/
- ^ http://cbioportal.org
- ^ http://www.linkedomics.org/
- ^ https://www.kegg.jp/
- ^ http://genemania.org/
References
Ahn, S., Kim, H. J., Kang, E., Kim, E. K., Kim, S. H., and Kim, J. H. (2020). Genomic profiling of multiple breast cancer reveals inter-lesional heterogeneity. Br. J. Cancer 122, 697–704. doi: 10.1038/s41416-019-0713-1
Bomer, U., Rassow, J., Zufall, N., Pfanner, N., Meijer, M., and Maarse, A. C. (1996). The preprotein translocase of the inner mitochondrial membrane: evolutionary conservation of targeting and assembly of Tim17. J. Mol. Biol. 262, 389–395. doi: 10.1006/jmbi.1996.0522
Bray, F., Ferlay, J., Soerjomataram, I., Siegel, R. L., Torre, L. A., and Jemal, A. (2018). Global cancer statistics 2018: GLOBOCAN estimates of incidence and mortality worldwide for 36 cancers in 185 countries. CA Cancer J. Clin. 68, 394–424. doi: 10.3322/caac.21492
Chandrashekar, D. S., Bashel, B., Balasubramanya, S. A. H., Creighton, C. J., Ponce-Rodriguez, I., Chakravarthi, B. V. S. K., et al. (2017). UALCAN: a portal for facilitating tumor subgroup gene expression and survival analyses. Neoplasia 19, 649–658. doi: 10.1016/j.neo.2017.05.002
Chen, H. Z., Tsai, S. Y., and Leone, G. (2009). Emerging roles of E2Fs in cancer: an exit from cell cycle control. Nat. Rev. Cancer 9, 785–797. doi: 10.1038/nrc2696
Chen, L., Ma, G., Cao, X., An, X., and Liu, X. (2018). MicroRNA-331 Inhibits Proliferation and Invasion of Melanoma Cells by Targeting Astrocyte-Elevated Gene-1. Oncol. Res. 26, 1429–1437. doi: 10.3727/096504018x15186047251584
Cheng, C., Yun, F., Ullah, S., and Yuan, Q. (2020). Discovery of novel cyclin-dependent kinase (CDK) and histone deacetylase (HDAC) dual inhibitors with potent in vitro and in vivo anticancer activity. Eur. J. Med. Chem. 189:112073. doi: 10.1016/j.ejmech.2020.112073
Fueyo, J., Gomez-Manzano, C., Yung, W. K., Liu, T. J., Alemany, R., McDonnell, T. J., et al. (1998). Overexpression of E2F-1 in glioma triggers apoptosis and suppresses tumor growth in vitro and in vivo. Nat. Med. 4, 685–690. doi: 10.1038/nm0698-685
Gao, J., Aksoy, B. A., Dogrusoz, U., Dresdner, G., Gross, B., Sumer, S. O., et al. (2013). Integrative analysis of complex cancer genomics and clinical profiles using the cBioPortal. Sci. Signal. 6:pl1. doi: 10.1126/scisignal.2004088
Garrido-Castro, A. C., and Winer, E. P. (2018). Predicting breast cancer therapeutic response. Nat. Med. 24, 535–537. doi: 10.1038/s41591-018-0033-7
Haneke, K., Schott, J., Lindner, D., Hollensen, A. K., Damgaard, C. K., Mongis, C., et al. (2020). CDK1 couples proliferation with protein synthesis. J. Cell Biol. 219:e201906147. doi: 10.1083/jcb.201906147
Harbeck, N., and Gnant, M. (2017). Breast cancer. Lancet 389, 1134–1150. doi: 10.1016/s0140-6736(16)31891-8
Hayes, M. D. W. D. N. (2010). ConsensusClusterPlus: a class discovery tool with confidence assessments and item tracking. Bioinformatics 26, 1572–1573. doi: 10.1093/bioinformatics/btq170
Holt, L. J., Tuch, B. B., Villén, J., Johnson, A. D., Gygi, S. P., and Morgan, D. O. (2009). Global analysis of Cdk1 substrate phosphorylation sites provides insights into evolution. Science 325, 1682–1686. doi: 10.1126/science.1172867
Kanehisa, M., Furumichi, M., Tanabe, M., Sato, Y., and Morishima, K. (2017). KEGG: new perspectives on genomes, pathways, diseases and drugs. Nucleic Acids Res. 45, D353–D361. doi: 10.1093/nar/gkw1092
Kannangai, R., Vivekanandan, P., Martinez-Murillo, F., Choti, M., and Torbenson, M. (2007). Fibrolamellar carcinomas show overexpression of genes in the RAS, MAPK, PIK3, and xenobiotic degradation pathways. Hum. Pathol. 38, 639–644. doi: 10.1016/j.humpath.2006.07.019
Kent, L. N., and Leone, G. (2019). The broken cycle: E2F dysfunction in cancer. Nat. Rev. Cancer 19, 326–338. doi: 10.1038/s41568-019-0143-7
Krek, W., Xu, G., and Livingston, D. M. (1995). Cyclin A-kinase regulation of E2F-1 DNA binding function underlies suppression of an S phase checkpoint. Cell 83, 1149–1158. doi: 10.1016/0092-8674(95)90141-8
Krug, K., Jaehnig, E. J., Satpathy, S., Blumenberg, L., Karpova, A., Anurag, M., et al. (2020). Proteogenomic Landscape of Breast Cancer Tumorigenesis and Targeted Therapy. Cell 183, 1436–1456.e31. doi: 10.1016/j.cell.2020.10.036
Liang, Y., Zhang, H., Song, X., and Yang, Q. (2020). Metastatic heterogeneity of breast cancer: molecular mechanism and potential therapeutic targets. Semin. Cancer Biol. 60, 14–27. doi: 10.1016/j.semcancer.2019.08.012
Lukas, C., Sørensen, C. S., Kramer, E., Santoni-Rugiu, E., Lindeneg, C., Peters, J. M., et al. (1999). Accumulation of cyclin B1 requires E2F and cyclin-A-dependent rearrangement of the anaphase-promoting complex. Nature 401, 815–818. doi: 10.1038/44611
Mishra, N. K., Southekal, S., and Guda, C. (2019). Survival Analysis of Multi-Omics Data Identifies Potential Prognostic Markers of Pancreatic Ductal Adenocarcinoma. Front. Genet. 10:624. doi: 10.3389/fgene.2019.00624
Okano, M., Oshi, M., Butash, A. L., Katsuta, E., Tachibana, K., Saito, K., et al. (2019). Triple-Negative breast cancer with high levels of annexin a1 expression is associated with mast cell infiltration, inflammation, and angiogenesis. Int. J. Mol. Sci. 20:4197. doi: 10.3390/ijms20174197
Osorio, J. (2015). Cell cycle: repurposing MYC and E2F in the absence of RB. Nat. Rev. Mol. Cell Biol. 16, 516–517. doi: 10.1038/nrm4044
Radvanyi, L. G. (2018). Targeting the cancer mutanome of breast cancer. Nat. Med. 24, 703–704. doi: 10.1038/s41591-018-0065-z
Ravindran Menon, D., Luo, Y., Arcaroli, J. J., Liu, S., KrishnanKutty, L. N., Osborne, D. G., et al. (2018). CDK1 Interacts with Sox2 and Promotes Tumor Initiation in Human Melanoma. Cancer Res. 78, 6561–6574. doi: 10.1158/0008-5472.can-18-0330
Rhodes, D. R., Yu, J., Shanker, K., Deshpande, N., Varambally, R., Ghosh, D., et al. (2004). ONCOMINE: a cancer microarray database and integrated data-mining platform. Neoplasia 6, 1–6. doi: 10.1016/s1476-5586(04)80047-2
Salhab, M., Patani, N., Jiang, W., and Mokbel, K. (2012). High TIMM17A expression is associated with adverse pathological and clinical outcomes in human breast cancer. Breast Cancer 19, 153–160. doi: 10.1007/s12282-010-0228-3
Schuldt, A. (2011). Cell cycle: E2F1 ensures the endocycle. Nat. Rev. Mol. Cell Biol. 12:768. doi: 10.1038/nrm3232
Seibert, M., Krüger, M., Watson, N. A., Sen, O., Daum, J. R., Slotman, J. A., et al. (2019). CDK1-mediated phosphorylation at H2B serine 6 is required for mitotic chromosome segregation. J. Cell Biol. 218, 1164–1181. doi: 10.1083/jcb.201806057
Tung, N., Lin, N. U., Kidd, J., Allen, B. A., Singh, N., Wenstrup, R. J., et al. (2016). Frequency of Germline Mutations in 25 Cancer Susceptibility Genes in a Sequential Series of Patients With Breast Cancer. J. Clin. Oncol. 34, 1460–1468. doi: 10.1200/jco.2015.65.0747
Vasaikar, S. V., Straub, P., Wang, J., and Zhang, B. (2018). LinkedOmics: analyzing multi-omics data within and across 32 cancer types. Nucleic Acids Res. 46, D956–D963. doi: 10.1093/nar/gkx1090
Warde-Farley, D., Donaldson, S. L., Comes, O., Zuberi, K., Badrawi, R., Chao, P., et al. (2010). The GeneMANIA prediction server: biological network integration for gene prioritization and predicting gene function. Nucleic Acids Res. 38, W214–W220. doi: 10.1093/nar/gkq537
Xu, X., Qiao, M., Zhang, Y., Jiang, Y., Wei, P., Yao, J., et al. (2010). Quantitative proteomics study of breast cancer cell lines isolated from a single patient: discovery of TIMM17A as a marker for breast cancer. Proteomics 10, 1374–1390. doi: 10.1002/pmic.200900380
Xuefang, Z., Ruinian, Z., Liji, J., Chun, Z., Qiaolan, Z., Jun, J., et al. (2020). miR-331-3p Inhibits Proliferation and Promotes Apoptosis of Nasopharyngeal Carcinoma Cells by Targeting elf4B-PI3K-AKT Pathway. Technol. Cancer Res. Treat. 19:1533033819892251. doi: 10.1177/1533033819892251
Yang, X., Si, Y., Tao, T., Martin, T. A., Cheng, S., Yu, H., et al. (2016). The Impact of TIMM17A on Aggressiveness of Human Breast Cancer Cells. Anticancer Res. 36, 1237–1241.
Yeo, S. K., and Guan, J. L. (2017). Breast Cancer: multiple subtypes within a Tumor? Trends Cancer 3, 753–760. doi: 10.1016/j.trecan.2017.09.001
Zacharakis, N., Chinnasamy, H., Black, M., Xu, H., Lu, Y. C., Zheng, Z., et al. (2018). Immune recognition of somatic mutations leading to complete durable regression in metastatic breast cancer. Nat. Med. 24, 724–730. doi: 10.1038/s41591-018-0040-8
Keywords: TIMM17A, breast cancer, data mining, regulatory network, cell cycle, CDK1
Citation: Cai JJ, Chen JY, Huang L, Wang CX, Zhang WY, Zhou Q and Sun ZH (2021) A TIMM17A Regulatory Network Contributing to Breast Cancer. Front. Genet. 12:658154. doi: 10.3389/fgene.2021.658154
Received: 25 January 2021; Accepted: 15 June 2021;
Published: 05 August 2021.
Edited by:
Salvatore Piscuoglio, University of Basel, SwitzerlandReviewed by:
Pranjal Sarma, University of Cincinnati, United StatesUdo Jeschke, Ludwig-Maximilians-Universität München, Germany
Copyright © 2021 Cai, Chen, Huang, Wang, Zhang, Zhou and Sun. This is an open-access article distributed under the terms of the Creative Commons Attribution License (CC BY). The use, distribution or reproduction in other forums is permitted, provided the original author(s) and the copyright owner(s) are credited and that the original publication in this journal is cited, in accordance with accepted academic practice. No use, distribution or reproduction is permitted which does not comply with these terms.
*Correspondence: Quan Zhou, springzhou@yeah.net; Zhaohui Sun, zhaohui3@126.com
†These authors have contributed equally to this work and share first authorship