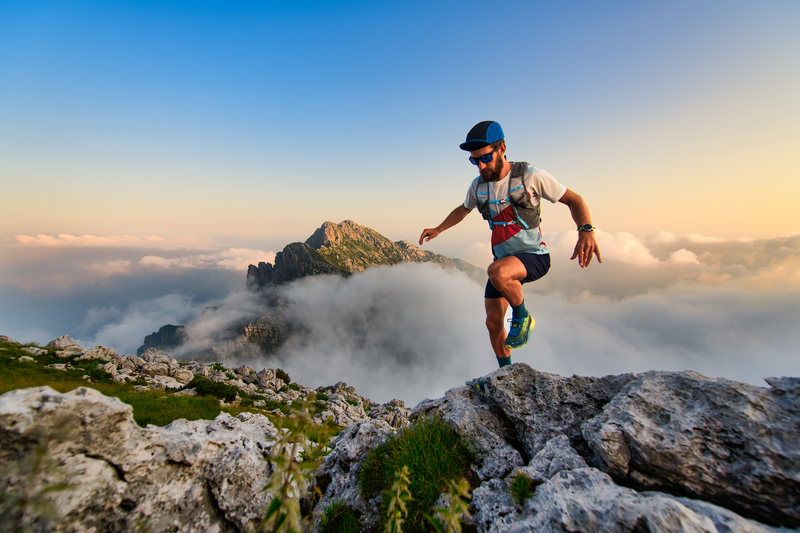
95% of researchers rate our articles as excellent or good
Learn more about the work of our research integrity team to safeguard the quality of each article we publish.
Find out more
ORIGINAL RESEARCH article
Front. Genet. , 20 May 2021
Sec. Genetics of Aging
Volume 12 - 2021 | https://doi.org/10.3389/fgene.2021.657636
This article is part of the Research Topic Genomics, Epigenomics and Aging View all 5 articles
Background: Neurodegenerative Diseases (NDs) are age-dependent and include Alzheimer’s disease (AD), Parkinson’s disease (PD), progressive supranuclear palsy (PSP), frontotemporal dementia (FTD), and so on. There have been numerous studies showing that accelerated aging is closely related (even the driver of) ND, thus promoting imbalances in cellular homeostasis. However, the mechanisms of how different ND types are related/triggered by advanced aging are still unclear. Therefore, there is an urgent need to explore the potential markers/mechanisms of different ND types based on aging acceleration at a system level.
Methods: AD, PD, PSP, FTD, and aging markers were identified by supervised machine learning methods. The aging acceleration differential networks were constructed based on the aging score. Both the enrichment analysis and sensitivity analysis were carried out to investigate both common and specific mechanisms among different ND types in the context of aging acceleration.
Results: The extracellular fluid, cellular metabolisms, and inflammatory response were identified as the common driving factors of cellular homeostasis imbalances during the accelerated aging process. In addition, Ca ion imbalance, abnormal protein depositions, DNA damage, and cytoplasmic DNA in macrophages were also revealed to be special mechanisms that further promote AD, PD, PSP, and FTD, respectively.
Conclusion: The accelerated epigenetic aging mechanisms of different ND types were integrated and compared through our computational pipeline.
With the further extension of human life, the number of elderly people is increasing and the incidence rate of senile neurodegenerative diseases (ND) is also rising (Kovacs, 2017). Although human life expectancy has been improved in recent years, ND have become the most common diseases affecting elderly populations (Heemels, 2016), with a large number of people being affected by Alzheimer’s disease (AD), Parkinson’s disease (PD), frontotemporal dementia (FTD), and progressive Supranuclear Palsy (PSP). Life is disrupted by the development of NDs in aging patients, with concomitant implications in terms of social resources and economic costs. It is estimated that the prevalence of NDs will increase to 131 million in the next few decades (Santiago et al., 2020), meaning that NDs have become an important topic of global concern.
A large amount of epidemiological evidence has shown that NDs are closely related to advanced brain aging, which is often considered to be one of the driving factors of ND (Mayne et al., 2020). There were a series of risk factors that interact with each other in the aging brain coordinately, where the immune system is dysregulated in abnormal aging (Liang et al., 2017). For example, chronic inflammation was considered to be one of the pathogenic factors of NDs in elderly people (Liang et al., 2017). In addition, the functions in the mitochondrial OXPHOS system are diminished in ND patients (Grimm and Eckert, 2017). Moreover, the accumulation of mitochondrial dysfunction (such as mtDNA mutation, increased oxidative stress, and mitochondrial transport/distribution defects) are more serious in ND (Grimm and Eckert, 2017).
There are a series of common characteristics of the advanced aging brain shared by different ND types, such as imbalances in cellular homeostasis and advanced cell death (Xu et al., 2020). If the cells cannot return to homeostasis for a long time, it may lead to irreversible abnormal cell death, along with continuous dysfunctions or the pathological state (Xu et al., 2020). Cellular homeostasis is thought to be related to cell cycle arrest, cell senescence, apoptosis, and autophagy (Dodig et al., 2019). The DNA damage repair response, imbalance of cellular energy metabolisms, and immune homeostasis can lead to cellular homeostasis disorder. For instance, the protein stabilization network is particularly important in neurons, and its abnormality was considered to be closely related to ND. In addition, the nervous system diseases shared common pathogenic factors, such as oxidative stress, environmental stress, and protein dysfunction, disrupting the protein stability in cells. Studies have proven that the mechanisms related to protein stability might be the basis of the etiology of ND (Höhn et al., 2020). Furthermore, there are other pathogenic factors shared by different ND types in the context of aging brains, such as oxidative stress, extracellular fluid, and protein dysfunction, which are also related to imbalances in cellular homeostasis. For example, research has proved that the mechanisms relating to protein stability might be the basis of the etiology of NDs (Höhn et al., 2020). Various studies have also reported that metal ion homeostasis (e.g., copper, iron, and zinc) is dysregulated during advanced brain aging, then NDs are induced. The excessive accumulation of metal ions has been found in a large number of ND patients (Ashraf et al., 2018). In short, the mechanism of ion imbalance in neurons is also thought to be related to NDs (Jomova et al., 2010). Thus, both ion homeostasis disorder and protein homeostasis disorder led to irreversible cellular homeostasis disorders. Based on previous reports, we can hypothesize that cellular homeostasis imbalance is one of the most important risk factors of ND during advanced brain aging (Figure 1A).
Although a series of studies have shown that the occurrence of ND was mainly due to aging acceleration as well as the imbalance of cellular homeostasis, resulting in cell dysfunction and pathological state (Bohlen et al., 2019), the relative mechanisms still need to be explored more thoroughly. Machine learning technology can be leveraged to classify healthy and diseased populations (Camacho et al., 2018). Moreover, using a prediction model based on omic profiles to identify potential biomarkers is informative to the experimental design, evaluation, diagnosis, and treatment of ND (Camacho et al., 2018). There are also some studies using network methods to analyze the common and specific molecular characteristics of different ND types, but the mechanisms of how ND was related/triggered by accelerated aging as well as cellular homeostasis imbalances, still need to be investigated more systematically (Stopa et al., 2018; Santiago et al., 2020). We therefore urgently require explorations of these mechanisms based on proper datasets.
In this paper, the relationship between aging, cellular homeostasis, and ND (including AD, PD, PSP, and FTD), as well as the relative mechanisms involved, were analyzed by our computational pipeline based on a series of methylation profiles in the GEO dataset. As shown in Figure 1B, this workflow involved five stages. (1) The AD, PD, PSP, FTD, and normal aging markers were identified by machine learning methods, respectively. (2) The aging score and disease score were given accordingly, thus the accelerated aging pattern in ND was validated. (3) According to the correlation between each pair of genes and the aging score, the aging acceleration differential network was thereby constructed. (4) Both network and enrichment analysis were be used to explore the mechanisms that relate accelerated aging to ND, respectively. (5) Sensitivity analysis was then performed to further investigate cellular homeostasis imbalances in different ND types using the Markov Chain Monte Carlo (MCMC) method.
DNA methylation profiles were used in this work, more specifically, 22905 cpg sites from 6 GSE profiles, the details of which are shown in Supplementary Text 1, 2. The aging predictor and disease predictor were modeled by the classification ensemble algorithm of the classification tree (shown in detail in the section “Materials and Methods” below), and the best predictor was selected based on 10-fold cross-validation (Table 1 and Supplementary Text 1). The learning curves of the training data set are shown in Figure 2.
Figure 2. The learning curve and ROC curve in the aging predictor and improved PD predictor. (A) The learning curve of the aging predictor. (B) The ROC curve of the aging predictor. (C) The learning curve of the AD predictor. (D) The ROC curve of the AD predictor. (E) The learning curve of the PD predictor. (F) The ROC curve of the PD predictor. (G) The learning curve of the PSP predictor. (H) The ROC curve of the PSP predictor. (I) The learning curve of the FTD predictor. (J) The ROC curve of the FTD predictor.
According to the cross-validation results, the top 46, 35, 14, 23, and 34 dimensions of cpg sites were identified as risk biomarkers related to aging, AD, PD, PSP, and FTD, respectively. Next, these five prediction results were verified in the independent test data set. This ensured that the classification results in the test data had adequate accuracy (Table 1). The receiver operating characteristic (ROC) curves of the predictors are also shown in Figure 2. The area under the ROC curve (AUC) 0.818, 0.693, 0.642, 0.903, and 0.857 in the predictor of aging for AD, PD, PSP, and FTD, respectively. Overall these results showed that the predictor had high accuracy and efficiency.
It has been reported that the identified biomarkers are closely related to aging and ND (Table 2; Salmerón et al., 2001; Hu et al., 2004; Katoh, 2008; Qiu and Ghosh, 2008; Fujimoto et al., 2009; Su et al., 2009; Kozarova et al., 2011; Euskirchen et al., 2012; Berwick and Harvey, 2014; Lopez-Pelaez et al., 2014; Alquézar et al., 2016; Chailangkarn et al., 2016; Densham et al., 2016; Husain et al., 2016; Pîrşcoveanu et al., 2017; Kurihara et al., 2019; Monteiro and Forti, 2019). Interestingly, these related functions (i.e., DNA damage repair response, mitochondrial dysfunction, or Ca ion homeostasis disorder), further indicated the imbalance of cellular homeostasis, and abnormal cell death was further induced across various ND types. Therefore, cellular homeostasis disorder may not only disrupt normal brain functioning but also lead to pathological changes related to ND.
To study the accelerated aging pattern in ND, the aging score was calculated based on 46 aging risk biomarkers (shown in the section “Materials and Methods” below). Both the median and median scores for different age groups are shown in Table 3. With the increase in age, the aging scores in both disease and normal aged individuals showed an upward trend. The results also showed that the accelerated aging pattern in ND compared with the normal aged people, which was consistent with previous studies (Liu et al., 2020). Compared with the chronological age, the aging score was more informative. The Kolmogorov–Smirnov (K–S) test was used to test the aging scores of each ND type as well as the healthy aged sample coming from the normal distribution. The results showed that the p-value was close to 0 and rejects the original hypothesis (shown in Supplementary Table 1). Consequently, the Kruskal–Wallis test was then executed to verify whether the aging score reflected a significant difference between ND and normal individuals, where different age groups, as well as different ND types, were compared. The results are shown in Figure 3, Table 3, Supplementary Figure 1, and Supplementary Table 2. These results indicate that the aging scores of ND sample individuals exhibit a significantly accelerated aging pattern, compared with those of normal aged individuals (p < 0.05).
Figure 3. The results of the Kruskal–Wallis test for aging scores of ND individuals and normal individuals in different age groups. (A) Age ≥ 50; (B) Age ≥ 55; (C) Age ≥ 60; (D) Age ≥ 65; (E) Age ≥ 70; (F) Age ≥ 75; and (G) Age ≥ 80.
To better study the potential mechanisms between aging and ND, the aging acceleration differential network was constructed, where FDR < 0.1 was used to ensure reliable correlation. The (partial) coefficients were also summarized and used to compare the relationship of each pair of cpg cites in the context of aging. Aimed at verifying the scale-free characteristics, the probability corresponding to logarithmic transformation and its degree were used to test the power-law distribution (shown in the section “Materials and Methods”). The node degree distribution curve and Pearson correlation coefficient of different ND types are shown in Figure 4.
Figure 4. The degree distribution of the acceleration aging network. (A) AD; (B) PD; (C) PSP; (D) FTD; and (E) All NDs.
The results revealed that the aging acceleration network was with the scale-free pattern. The degree and frequency were inversely correlated, and only a small proportion of genes had a high degree. Furthermore, the Fisher’s exact test was carried out to calculate the similarity between the different networks based on the training data and the test data, respectively, and the result showed a p-value very close to 0 (1e-23238, 1e-131120, 1e-3103, 1e-44540, and 1e-139070 for AD, PD, PSP, FTD, and all NDs, respectively). In addition, the node with the highest degree in the network was also significantly correlated with maintaining cellular homeostasis (Table 4; Crowe et al., 1994; Dong et al., 1997; Keats et al., 2007; Tang et al., 2011; Kaneko et al., 2016; Kula et al., 2019; Anerillas et al., 2020).
To further investigate the potential mechanisms between aging and ND, each shortest path of aging-AD, aging-PD, aging-PSP, and aging-FTD were identified based on the aging acceleration differential network using the Dijkstra algorithm. Enrichment analysis was then performed based on each of the shortest paths, the special functions (i.e., the Kyoto Encyclopedia of Genes and Genomes (KEGG) pathway, and the Biological Process (BP) term in Gene Ontology (GO), shown in Figure 5 and Table 5). These indicated multiple dysfunctions of different ND types (McGeer et al., 1989; Behl, 2000; Nagatsu et al., 2000; Everse et al., 2011; Obulesu and Lakshmi, 2014; Caputi and Giron, 2018; Cui and Xu, 2018; Yan et al., 2018; Liu et al., 2019; Porro et al., 2019; Starr, 2019; Ashrafizadeh et al., 2020; Burgaletto et al., 2020; Luo et al., 2020; Paul et al., 2021), respectively.
Figure 5. The shortest paths for enrichment analysis of KEGG and BP. (A,B) AD enrichment results; (C,D) PD enrichment results; (E,F) PSP enrichment results; (G,H) FTD enrichment results; (A,C,E,G) enriched KEGG pathway; (B,D,F,H) enriched BP terms; The yellow nodes represent the aging biomarkers, the blue nodes represent the genes connecting aging biomarkers and ND biomarkers, the green nodes represent the ND biomarkers, and the genes in the red square frames coincide with those genes in the enriched functions.
Table 5. Enrichment analysis results (minimum p-value and FDR) of the four disease enrichment pathways are observed, respectively.
In addition, common enriched functions were investigated to study the crucial mechanisms across different ND types, where the top ten KEGG pathways are shown in Figure 6. For example, the Toll-like receiver (TLR) signaling pathway was the top KEGG pathway related to all four ND types. The TLRs activated their downstream pathways and then induced NF-κB and Pro-Il-1β, both of which are related to neuroinflammation and the pathogenesis of a variety of neurological diseases (Azam et al., 2019). Furthermore, the activation of TLRs can also induce the inflammatory response of macrophages by activating the transcription cascade (Lauterbach et al., 2019). The inflammatory response induced by activation of TLRs is considered to be closely related to AD, PD, PSP, and FTD (López González et al., 2016). Therefore, the importance of the Toll-like receiver signaling pathway is reflected in a variety of ND types. Furthermore, cytokine receptor interaction is also critical in ND progression. For example, microglia can release cytokines to trigger a cellular inflammatory response and participate in the occurrence and development of NDs (Colonna and Butovsky, 2017). Cellular apoptosis is also a fundamental process in the progression of nervous system diseases (Radi et al., 2014).
In order to further investigate the complex cellular homeostasis imbalances across different ND types, a global sensitivity analysis was performed using the MCMC method (shown in section “Materials and Methods”). To study the relationship between aging and ND markers, the differential MCMC K–S statistics were calculated based on each “aging-ND” pair; then the top ranked “aging-ND” pairs were identified for each disease (as described in the section “Materials and Methods”). The aging markers shared by different ND types as well as the disease markers within each specific ND type were then explored, based on both aging and ND MCMC differences.
TSC2 was the risk marker with the highest absolute MCMC difference across the four types of ND. It has been reported that the phosphorylation of TSC2 could restore the expression of NF-κB protein, which is vital to reduce the production of inflammatory mediators and ND markers (Kumar et al., 2017). In addition, the aging markers identified in all four diseases were identified according to the obtained “aging-ND” pairs. Among them, there were two aging markers with high frequencies. CHGN-1 (CSGALNACT1, frequency = 40) is a key enzyme for the production of CSPGs, participating in the demyelination, remyelination, axonal degeneration, and regeneration of the central nervous system (Saigoh et al., 2016). SLC15A2 (PEPT2, frequency = 24) can affect the production of pro-inflammatory cytokines by macrophages (Gupta et al., 2013). The excessive release of pro-inflammatory cytokines causes neuronal damage (Shen et al., 2004).
In AD, CDCA7L was with the highest absolute MCMC difference. It was associated with the intelligence quotient (Pan et al., 2011), which could even inhibit the Monoamine oxidase A(MAOA) promoter as well as neuronal cell death (Ou et al., 2006).
EFNB2 had the highest absolute MCMC difference in PD. EFNB2 was involved in adjusting the development of the nervous system and the neuronal migration (Lévy et al., 2018), and could also activate the Eph/efn forward signaling pathway as well as cell apoptosis (Zhong et al., 2019).
In PSP, the marker with the highest absolute MCMC difference was BCL2, which could affect the activity of the mitochondrial complex (Chong et al., 2020). BCL2 could also enhance the anti-apoptotic effect of nerve growth factor (NGF) and promote the survival and differentiation of nerve cells (Troullinaki et al., 2019).
In FTD, MICAL1 had the highest absolute MCMC difference. Through interacting with STK38 and STK38L, it acted as a negative regulator of cell apoptosis (Zhou et al., 2011). MICAL1 is also involved in the regulation of lamina specific connections in the nervous system (Schmidt et al., 2008).
The potential network markers were also found out based on each “aging-ND” pair from the results of sensitivity analysis by summarizing the betweenness in the aging acceleration differential network, as shown in Table 6, Figure 7, and Supplementary Figure 2. As a result, DUSP12 had the largest betweenness across the four ND types. DUSP12 could regulate the c-Jun N-terminal kinase (JNK) signaling pathway by dephosphorylating its substrate, which was critical to cell differentiation, apoptosis, and other neural functions in ND progression (Ha et al., 2019). It also has been reported that the overexpression of DUSP12 inhibited the production of pro-inflammatory cytokines and chemokines (Cho et al., 2017).
Furthermore, the genes (cpg site) within each shortest “aging-ND” path were also identified. For example, the top network (betweenness) marker in AD was RALGPS2. It has hitherto been revealed that RALGPS2 silencing induces cell apoptosis, by improving cell cycle inhibitors p27 and p21 (Santos et al., 2016). The top network marker in PD was MEOX2 (GAX). The deletion of MEOX2 can lead to decreased capillary density as well as resting cerebral blood flow, and then promote the loss of hypoxic angiogenesis in the brain. It causes hypoxia reaction and cell death (Wu et al., 2005). QRSL1, whose mutation may lead to dysfunction of mitochondrial energy production and mitochondrial disorder (Albers and Beal, 2002), was the top network marker in PSP. The top network marker in FTD was C3. C3 uptake by cells reduces stress-related cell death (e.g., oxidative stress or starvation). In the process of inflammation, storing C3 in cells can prevent some substances that induce cell death (Kulkarni et al., 2019). In brief, these network markers were also informative and indicate the relationship between cellular homeostasis and NDs.
In this article, a series of computational methods were integrated to explore the potential mechanisms between aging and four types of ND, as well as the commonalities and specificities of these four types of ND based on aging acceleration. Firstly, aging markers and ND markers were identified by the aging predictor and disease predictor, respectively. Secondly, according to the selected aging markers, the aging score of dementia patients showed an accelerated aging pattern compared with normal aged people. Furthermore, the aging acceleration differential network was constructed based on the aging score, then crucial shortest “aging-ND” paths were discovered.
The aging markers (using MCMC) indicated that an imbalance in cellular homeostasis is the key bridge, linking accelerated aging and ND. For example, the top aging marker was TSC2, as a risk marker of ND by affecting the inflammatory response (Kumar et al., 2017). Furthermore, other aging markers revealed the critical roles of cellular homeostasis in ND progression. CHGN (CSGALNACT1) and SLC15A2. CHGN-1 are the critical enzymes for CSPG production (Saigoh et al., 2016), which is the main pericellular and extracellular component of the regulatory environment (Hu et al., 2018). SLC15A2 (PEPT2) plays a key role in regulating the concentration of neuropeptides in extracellular fluid (Dheen et al., 2007).
The top risk marker in the AD predictor was SMARCA4, which participated in cellular biological processes by altering the contact of DNA histone in the nucleosome (Euskirchen et al., 2012). RALGPS2 was with the largest betweenness in AD and as a potential pathogenic index of AD (Liu et al., 2007). The marker with the highest absolute MCMC difference in AD was CDCA7L, as the regulator of caspase-3, which played a significant role in cell death progression (Yosefzon et al., 2018). The enrichment result of AD indicated apoptosis. Our results emphasize that cellular homeostasis disorder in patients with AD may be due to the imbalance of intracellular calcium homeostasis and abnormal DNA repair, resulting in excessive cellular apoptosis and then leading to AD.
DUSP12 was the top risk marker in the PD predictor, as a regulator of the cell cycle (Kozarova et al., 2011). MEOX2 was the top network marker in PD, leading to the loss of neurons and a significant reduction in the microvessels associated with plaque, which further indicated the synergistic effect of vascular compromise and amyloid deposition on the dysfunction of neurons (Soto et al., 2016). EFNB2 (with the maximum differential MCMC value) is closely related to the cellular autophagy pathway (Zhong et al., 2019); it could also activate the quiescent static stem cells and promote the depletion of cells (Ottone et al., 2014). Enrichment results identified the cytokine receptor interaction involved in many cellular processes, including cell growth, cell differentiation, cell apoptosis, cellular homeostasis, and so on. Our results suggest that the disorder of cytokines, oxidative stress, and protein deposition may lead to disorder of homeostasis and cell death, and then trigger the occurrence of PD.
The results indicate that BRCA1 was the top risk marker in PSP. The high expression of BRCA1 can affect the DNA self repair pathway and cause DNA damage (Densham et al., 2016). In addition, the top network marker (with the highest betweenness) was QRSL1. The mitochondrial dysfunctions induced by QRSL1 play an important role in advanced PSP (Albers and Beal, 2002). BCL2 was with the highest absolute MCMC difference in PSP, which could prevent oxidative stress-induced DNA damage as well as cell death (Chong et al., 2020). It is also the key regulator of cell apoptosis (Roberts, 2020). In summary, DNA damage, advanced neuroinflammation, and cell death induce the occurrence of PSP.
The top FTD risk marker IKBKB plays an important role in the NF-κB signaling pathway (Salmerón et al., 2001), which is the main cause of FTD (Alquézar et al., 2016). Furthermore, MICAL was with the highest absolute MCMC difference in FTD, playing an important role in cellular redox regulation, survival, development, and death (Ortegón Salas et al., 2020). C3 was the top network marker in FTD. The content of C3 in FTD was significantly increased (Katzeff et al., 2020). The enrichment results showed that the cytosolic DNA sensing pathway can damage the nervous system. Therefore, based on the above studies, FTD is considered the imbalance of intracellular inflammatory factors and excessive oxidative stress, leading to an imbalance of cellular homeostasis.
The extracellular fluid, cellular metabolisms, and inflammatory response were considered the common characteristics of the cellular homeostasis imbalance across different types of ND in the context of aging acceleration. Another study found that the extracellular fluid of microglia can affect cellular homeostasis and cell survival (Erny et al., 2015). Cell metabolites are also crucial for cellular homeostasis (Koo and Guan, 2018). Cellular inflammation can trigger a cellular stress response. When neuroinflammation occurs, extracellular fluid is considered the key index of cellular homeostasis imbalance. There is various evidence to suggest that many types of ND are related to the inflammatory response (Stephenson et al., 2018). Moreover, these risk factors interact with each other and promote the development of NDs.
In cell death and neurodegenerative theory, the dynamic balance of neurons is induced by advanced brain aging, and accompanied by pathological changes (Andreone et al., 2020), such as mitochondrial dysfunction, and oxidative stress, which are causes that drive advanced cell apoptosis. The abnormal apoptosis of nerve cells then leads to a decline in normal function and eventually the occurrence of ND. Our results also found critical mechanisms of cellular homeostasis imbalance in different ND progressions, thus both the common and specific cellular homeostasis imbalances are summarized. Moreover, these results indicate the critical mechanisms of cellular homeostasis imbalance in different ND types, thus both the common and specific cellular homeostasis imbalances were summarized (Figure 8). Interestingly, the common characteristics were identified as extracellular fluid, cell metabolism, and the inflammatory response, based on aging acceleration. In terms of specific characteristics, abnormal calcium homeostasis and the DNA repair pathway damage lead to advanced cell death and then promote the occurrence of AD. This may be due to abnormal protein deposition, oxide accumulation, and cytokine disorder (related to advanced oxidative stress), leading to the progression of PD. DNA damage and mitochondrial dysfunction induce PSP. Abnormal cellular differentiation or the overloading of cytoplasmic DNA in macrophages triggers extra inflammatory factors in FTD.
Figure 8. The mechanism of ND induced by acceleration aging. The brown gene is a biomarker of ND. The gray gene is the top MCMC marker of aging. The red gene is the top MCMC marker of each ND type. The pink gene is the network node with highest betweenness. The yellow arrow indicates the connection of Calcium homeostasis. The purple arrow represents a link to DNA damage repair response. The green arrow represents the link with the mitochondrial function. The brown arrow represents a link to oxidative stress. The black arrow indicates the connection with ubiquitin. The red arrow indicates the connection of the intracellular signal.
The present study used machine learning methods to identify the risk markers of different types of ND and the normal aging process for each disease, respectively. The aging score was thereby summarized. The results showed that ND sample individuals exhibited significantly accelerated aging patterns. By comparing the correlation of each pair of cpg sites between ND and the normal aged group, aging acceleration differential networks were constructed. In addition, the mechanisms of cellular homeostasis imbalances across different ND types were found based on enrichment analysis and sensitivity analysis. The results showed that in the background of accelerated aging, extracellular fluid, cell metabolism, and the inflammatory response induce imbalances in cellular homeostasis, which trigger ND progression. The specific mechanisms of AD, PD, PSP, and FTD were also identified, including Ca ion disorder, protein deposition, DNA damage, and dysfunctions in macrophages, respectively.
The DNA methylation profiles were obtained from the Gene Expression Omnibus (GEO) database (Supplementary Table 1), including GSE15745, GSE51923, GSE53740, GSE57361, GSE66351, and GSE138597, along with the age index. These datasets were from seven different platforms: GPL6104, GPL8178, GPL8490, GPL5175, GPL13534, GPL 11154, and GPL 21145.
The steps of obtaining DNA methylation profiles were as follows:
(1) cpg sites with missing values ≥ 30% were deleted.
(2) According to different brain regions, the k-nearest neighbor algorithm (k = 10, with the Euclidean distance) was used to supplement the missing values.
(3) Individuals without the age index were deleted.
(4) Patients with early onset ND (age ≤ 50) were removed.
(5) z-score normalization was performed based on the healthy aged individuals.
(6) The Singular Value Decomposition (SVD) method was used to eliminate inter-sample variation based on the top three principal components in healthy aged individuals.
(7) The z-score was then utilized to normalize all individuals based on the mean and standard deviation of healthy aged individuals.
The final sample included 366 healthy youth samples (age ≤ 50, 250, in the training data and 116 in the test data), 442 normal old individuals (age > 50, 300+142), 128 AD individuals (85+43), 36 PD individuals (25+11), 123 FTD individuals (85+38), and 42 PSP individuals (30+12). The DNA methylation data set included 22905 cpg sites (Supplementary Text 2).
After randomization as well as a random disorder, the healthy population samples were divided into training data set and test data set. The ratio of training data set samples to test data set samples were close to 2:1. The ReliefF algorithm was used to select key features, then the first 50 models were studied to train predictors. The optimal model was selected by 10-cross validation. To verify the accuracy of the aging predictor, the selected model was verified in the test data set.
(1) The normal aged group (age > 50) were labeled as 1 and the young healthy group (age ≤ 50) were labeled as 0.
(2) The 22,905 cpg sites were sorted by the ReliefF algorithm.
(3) The predictor was generated using the ensemble learning algorithm.
The ensemble learning algorithm established 100 decision tree models, and then put the data in the 100 classifiers for decision-making. The classification result was optimized to get the most answers from the 100 classifiers. For 100 classifiers, weak weight was given to the classifiers with more wrong classification results using the formula of the weight coefficient. The calculation method was as follows:
and the classifier weight was:
where m was the number of predicted variables; Dn was the weight of each sample;
εn was the classifier error; xn was the member of predicted variables; yn represented the corresponding attribute value of xn and hn was each attribute.
One hundred weak classifiers were superimposed to generate the strongest classifier. The optimal model was selected by 10-fold cross validation. Ultimately, the model with the highest accuracy rate was chosen. The identified features were considered as aging and each ND marker, respectively.
To construct disease predictors, 442 healthy aged individuals, 128 AD individuals, 36 PD individuals, 123 FTD individuals, and 42 PSP individuals were randomly chosen. In the classification process of each disease predictor, the ND sample was labeled as 1 and the normal aged sample was labeled as 0. To avoid the unbalance of samples in machine learning, the normal aged group (training data) were divided into 3, 9, 7, and 3 subgroups to compare with AD, PD, PSP, and FTD (Supplementary Table 2), respectively.
For each sample, the aging score was calculated as follows:
(1) The regression result (from young as 0 to old as 1) was used as the aging score using the ensemble learning algorithm from 100 regression tree models based on aging markers.
(2) The K–S test was used to test whether the aging score came from the normal distribution. The p-value was the least significant level to reject the original hypothesis. The smaller the p-value, the easier it was to reject the original hypothesis. Both the original aging score and the score adjusted by the transformation of the chronological age were tested, where the chronological age was transformed using the sigmoid function:
The transformed age was predicted based on the aging scores using linear regression:
where b is the regression coefficient for aging score.
(3) The Kruskal–Wallis test was used to compare the accelerated aging pattern between ND and normal aged individuals for different age groups.
(4) In addition, the risk score of each ND predictor was also calculated for further network analysis.
To further reveal the relationship between aging and ND, the aging acceleration network was constructed based on the training data set and test data set.
(1) To compare the relationship of each pair of cpg sites in the context of the aging process, both the Pearson correlation coefficient for each pair of cpg sites and the partial correlation coefficient based on the aging score was calculated based on the normal aged group and each ND group, respectively.
(2) The Benjamin-Hochberg False Discovery Rates (FDR) method was used to adjust the p-values of the correlation coefficient as well as the partial correlation coefficient.
(3) The differences of correlation and partial correlation were summarized for each ND group as well as the normal aged group.
(4) The edge between the two cpg sites was retained if the sign of the difference value in the ND group and the normal aged group was opposite, as well as FDR < 0.1 in step (2).
(5) The scale-free characteristics of aging acceleration differential networks were verified by the power-law distribution.
(6) The shortest path between each pair of aging and ND markers was picked out based on each aging acceleration differential network using the Dijkstra algorithm, respectively.
(7) The network was constructed based on the training data and used for further analysis (i.e., identifying the shortest path, exploring potential functions between aging and ND, etc.), and the network constructed based on the test data was used to validate the training network.
As a result, five types of aging differential networks were constructed: AD, PD, PSP, FTD, and all four NDs together.
Global sensitivity analysis was used to investigate different cellular homeostasis imbalances in the background of aging acceleration, based on the MCMC method. Both the common and specific characteristics across different ND types were analyzed. To explore the common characteristics among different ND, we made a presumption that if the marker indicated the high risk scores in more than one ND type, then it might reveal the common mechanisms across multiple ND types.
The MCMC method was used for sampling from certain posterior distributions following a given probabilistic background in a high-dimensional space. The key step in MCMC was to construct a Markov chain whose equilibrium distribution equals the target probability distribution. It proceeded as follows:
(1) Construct a transition kernel of an ergodic Markov chain. In this study, the prior distribution for each of the parameters was the normal distribution based on both aging and disease markers for each ND types, respectively.
(1) Simulate the chain until it reaches an equilibrium. The Metropolis-Hastings sampling method was used to determine whether the new sample (θ∗) is acceptable based on the α value:
where P(θn| X) and P(θ∗| X) are the posterior probabilities of the n-th accepted sample and the new sample, q(θn→θ∗) represents the transition probability from the n th accepted sample to the new sample, and q(θ∗→θn) is the transition probability from the new sample to the n th accepted sample.
In this article, the mean value of the aging score as well as four types of ND scores were used to evaluate the common characteristics across multiple types of ND. The mean value of the aging score and each ND risk score was used to evaluate the special characteristics of each type of ND.
1. Perform global sensitivity analysis. In this study, the K–S statistic was used to calculate the sensitivity of each parameter.
where F1 was the cumulative distribution of samples with a minus value after normalization, whereas F2 was the cumulative distribution of samples with the plus value. The interval for the K–S statistic was set to two (based on the sign of normalized DNA methylation profiles). The MCMC pseudocode is provided in Supplementary Text 4, 5.
The biological functions of the existing genes were found by enrichment analysis. Gene Ontology (GO) terminology and KEGG pathway were taken from the gene set enrichment analysis (GSEA) platform (version 7.2)1. A hypergeometric test was used to estimate the enrichment degree of the KEGG pathway or go BP term. The hypergeometric test formula was:
where N was the gene set of the whole gene, M was the known genes (e.g., KEGG pathway, or BP terms), N was the number of identified genes in each shortest pathway, and k was the number of common genes between the known genes and the identified candidate genes (in each aging-ND shortest path). The p-values of each pathway were controlled by the Benjamin-Hochberg (BH) method. To ensure the reliability of the results, FDR < 0.05 was selected.
The original contributions presented in the study are included in the article/Supplementary Material, further inquiries can be directed to the corresponding author/s.
FS, YH, and YW performed the algorithm and analyzed the data. FS, YH, YC, XY, and YW wrote the manuscript. XS and YW designed and sponsored the study. All authors read and approved the manuscript.
This work was supported by the National Natural Science Foundation of China (32000478 to YW) and National Key R&D Program of China (2016YFC0901704, 2017YFA0505500, 2017YFC0907505, and 2017YFC0908405). The funders had no role in study design, data collection, and analysis, the decision to publish, or the preparation of the manuscript.
The authors declare that the research was conducted in the absence of any commercial or financial relationships that could be construed as a potential conflict of interest.
We thank Charlesworth Author Services (http://www.charlesworthauthorservices.com/∼Frontiers) for its linguistic assistance during the preparation of this manuscript.
The Supplementary Material for this article can be found online at: https://www.frontiersin.org/articles/10.3389/fgene.2021.657636/full#supplementary-material
Supplementary Figure 1 | The results of the Kruskal–Wallis test for age-matched different ND samples and normal samples. (A) AD; (B) PD; (C) PSP; (D) FTD.
Supplementary Figure 2 | The network marker with the highest betweenness of different ND. (A) AD; (B) PD; (C) PSP; (D) FTD.
Supplementary Table 1 | The p-value of K–S test of the aging score.
Supplementary Table 2 | The chronological age and aging scores of each ND group and the normal aged group (after age-matched).
Supplementary Text 1 | The detailed datasets used in this work.
Supplementary Text 2 | The cpg sites used in this work.
Supplementary Text 3 | The selected risk markers of the aging predictor and each ND predictor, along with their ReliefF weights.
Supplementary Text 4 | The pseudocode of MCMC for the common characteristics across multiple ND types.
Supplementary Text 5 | The pseudocode of MCMC for the special characteristics of each ND type.
AD, Alzheimer’s disease; AUC, area Under the ROC Curve; BH, Benjamin-Hochberg; BP, biological process; FDR, false discovery rates; FTD, frontotemporal dementia; GO, Gene Ontology; KEGG, Kyoto Encyclopedia of Genes and Genomes; K–S, Kolmogorov–Smirnov; MCMC: Markov Chain Monte Carlo; ND, neurodegenerative disease; PD, Parkinson’s disease; PSP, progressive supranuclear palsy; ROC, receiver operating characteristic.
Albers, D. S., and Beal, M. F. (2002). Mitochondrial dysfunction in progressive supranuclear palsy. Neurochem. Int. 40, 559–564. doi: 10.1016/s0197-0186(01)00126-7
Alquézar, C., de la Encarnación, A., Moreno, F., López de Munain, A., and Martín-Requero, Á (2016). Progranulin deficiency induces overactivation of WNT5A expression via TNF-α/NF-κB pathway in peripheral cells from frontotemporal dementia-linked granulin mutation carriers. J. Psychiatry Neurosci. 41, 225–239. doi: 10.1503/jpn.150131
Andreone, B. J., Larhammar, M., and Lewcock, J. W. (2020). Cell death and neurodegeneration. Cold Spring Harb. Perspect. Biol. 12:a036434. doi: 10.1101/cshperspect.a036434
Anerillas, C., Abdelmohsen, K., and Gorospe, M. (2020). Regulation of senescence traits by MAPKs. Geroscience 42, 397–408. doi: 10.1007/s11357-020-00183-3
Ashraf, A., Clark, M., and So, P. W. (2018). The aging of iron man. Front. Aging Neurosci. 10:65. doi: 10.3389/fnagi.2018.00065
Ashrafizadeh, M., Rafiei, H., Mohammadinejad, R., Afshar, E. G., Farkhondeh, T., and Samarghandian, S. (2020). Potential therapeutic effects of curcumin mediated by JAK/STAT signaling pathway: a review. Phytother. Res. 34, 1745–1760. doi: 10.1002/ptr.6642
Azam, S., Jakaria, M., Kim, I. S., Kim, J., Haque, M. E., and Choi, D. K. (2019). Regulation of toll-like receptor (TLR) signaling pathway by polyphenols in the treatment of age-linked neurodegenerative diseases: focus on TLR4 signaling. Front. Immunol. 10:1000. doi: 10.3389/fimmu.2019.01000
Behl, C. (2000). Apoptosis and Alzheimer’s disease. J. Neural Transm. 107, 1325–1344. doi: 10.1007/s007020070021
Berwick, D. C., and Harvey, K. (2014). The regulation and deregulation of Wnt signaling by PARK genes in health and disease. J. Mol. Cell Biol. 6, 3–12. doi: 10.1093/jmcb/mjt037
Bohlen, C. J., Friedman, B. A., Dejanovic, B., and Sheng, M. (2019). Microglia in brain development, homeostasis, and neurodegeneration. Annu. Rev. Genet. 53, 263–288.
Burgaletto, C., Munafò, A., Di Benedetto, G., De Francisci, C., Caraci, F., Di Mauro, R., et al. (2020). The immune system on the TRAIL of Alzheimer’s disease. J. Neuroinflammation. 17:298. doi: 10.1186/s12974-020-01968-1
Camacho, D. M., Collins, K. M., Powers, R. K., Costello, J. C., and Collins, J. J. (2018). Next-generation machine learning for biological networks. Cell 173, 1581–1592. doi: 10.1016/j.cell.2018.05.015
Caputi, V., and Giron, M. C. (2018). Microbiome-gut-brain axis and toll-like receptors in Parkinson’s disease. Int. J. Mol. Sci. 19:1689. doi: 10.3390/ijms19061689
Chailangkarn, T., Trujillo, C. A., Freitas, B. C., Hrvoj-Mihic, B., Herai, R. H., Yu, D. X., et al. (2016). A human neurodevelopmental model for Williams syndrome. Nature 536, 338–343. doi: 10.1038/nature19067
Cho, S. S. L., Han, J., James, S. J., Png, C. W., Weerasooriya, M., Alonso, S., et al. (2017). Dual-specificity phosphatase 12 targets p38 MAP kinase to regulate macrophage response to intracellular bacterial infection. Front. Immunol. 8:1259. doi: 10.3389/fimmu.2017.01259
Chong, S. J. F., Iskandar, K., Lai, J. X. H., Qu, J., Raman, D., Valentin, R., et al. (2020). Serine-70 phosphorylated Bcl-2 prevents oxidative stress-induced DNA damage by modulating the mitochondrial redox metabolism. Nucleic Acids Res. 48, 12727–12745. doi: 10.1093/nar/gkaa1110
Colonna, M., and Butovsky, O. (2017). Microglia function in the central nervous system during health and neurodegeneration. Annu. Rev. Immunol. 35, 441–468.
Crowe, P. D., VanArsdale, T. L., Walter, B. N., Ware, C. F., Hession, C., Ehrenfels, B., et al. (1994). A lymphotoxin-beta-specific receptor. Science 264, 707–710. doi: 10.1126/science.8171323
Cui, D., and Xu, X. (2018). DNA methyltransferases, DNA methylation, and age-associated cognitive function. Int. J. Mol. Sci. 19:1315. doi: 10.3390/ijms19051315
Densham, R. M., Garvin, A. J., Stone, H. R., Strachan, J., Baldock, R. A., Daza-Martin, M., et al. (2016). Human BRCA1-BARD1 ubiquitin ligase activity counteracts chromatin barriers to DNA resection. Nat. Struct. Mol. Biol. 23, 647–655. doi: 10.1038/nsmb.3236
Dheen, S. T., Kaur, C., and Ling, E. A. (2007). Microglial activation and its implications in the brain diseases. Curr. Med. Chem. 14, 1189–1197. doi: 10.2174/092986707780597961
Dodig, S., Čepelak, I., and Pavić, I. (2019). Hallmarks of senescence and aging. Biochem. Med. 29:030501. doi: 10.11613/BM.2019.030501
Dong, H., O’Brien, R. J., Fung, E. T., Lanahan, A. A., Worley, P. F., and Huganir, R. L. (1997). GRIP: a synaptic PDZ domain-containing protein that interacts with AMPA receptors. Nature 386, 279–284. doi: 10.1038/386279a0
Erny, D., Hrabě de Angelis, A. L., Jaitin, D., Wieghofer, P., Staszewski, O., David, E., et al. (2015). Host microbiota constantly control maturation and function of microglia in the CNS. Nat. Neurosci. 18, 965–977. doi: 10.1038/nn.4030
Euskirchen, G., Auerbach, R. K., and Snyder, M. S. W. I. (2012). /SNF chromatin-remodeling factors: multiscale analyses and diverse functions. J. Biol. Chem. 287, 30897–30905. doi: 10.1074/jbc.R111.309302
Everse, J., Liu, C. J., and Coates, P. W. (2011). Physical and catalytic properties of a peroxidase derived from cytochrome c. Biochim. Biophys. Acta 1812, 1138–1145. doi: 10.1016/j.bbadis.2011.05.003
Fujimoto, T., Tomizawa, M., and Yokosuka, O. (2009). SiRNA of frizzled-9 suppresses proliferation and motility of hepatoma cells. Int. J. Oncol. 35, 861–866. doi: 10.3892/ijo_00000400
Grimm, A., and Eckert, A. (2017). Brain aging and neurodegeneration: from a mitochondrial point of view. J. Neurochem. 143, 418–431. doi: 10.1111/jnc.14037
Gupta, S. K., Haigh, B. J., Griffin, F. J., and Wheeler, T. T. (2013). The mammalian secreted RNases: mechanisms of action in host defence. Innate. Immun. 19, 86–97. doi: 10.1177/1753425912446955
Ha, J., Kang, E., Seo, J., and Cho, S. (2019). Phosphorylation dynamics of JNK signaling: effects of dual-specificity phosphatases (DUSPs) on the JNK pathway. Int. J. Mol. Sci. 20:6157. doi: 10.3390/ijms20246157
Höhn, A., Tramutola, A., and Cascella, R. (2020). Proteostasis failure in neurodegenerative diseases: focus on oxidative stress. Oxid. Med. Cell Longev. 2020:5497046. doi: 10.1155/2020/5497046
Hu, M. C., Lee, D. F., Xia, W., Golfman, L. S., Ou-Yang, F., Yang, J. Y., et al. (2004). IkappaB kinase promotes tumorigenesis through inhibition of forkhead FOXO3a. Cell. 117, 225–237. doi: 10.1016/s0092-8674(04)00302-2
Hu, Y., Song, F., Jiang, H., Nuñez, G., and Smith, D. E. (2018). SLC15A2 and SLC15A4 mediate the transport of bacterially derived Di/Tripeptides to enhance the nucleotide-binding oligomerization domain-dependent immune response in mouse bone marrow-derived macrophages. J. Immunol. 201, 652–662. doi: 10.4049/jimmunol.1800210
Husain, A., Begum, N. A., Taniguchi, T., Taniguchi, H., Kobayashi, M., and Honjo, T. (2016). Chromatin remodeller SMARCA4 recruits topoisomerase 1 and suppresses transcription-associated genomic instability. Nat. Commun. 7:10549. doi: 10.1038/ncomms10549
Jomova, K., Vondrakova, D., Lawson, M., and Valko, M. (2010). Metals, oxidative stress and neurodegenerative disorders. Mol. Cell Biochem. 345, 91–104. doi: 10.1007/s11010-010-0563-x
Kaneko, M., Iwase, I., Yamasaki, Y., Takai, T., Wu, Y., Kanemoto, S., et al. (2016). Genome-wide identification and gene expression profiling of ubiquitin ligases for endoplasmic reticulum protein degradation. Sci. Rep. 6:30955. doi: 10.1038/srep30955
Katoh, M. (2008). WNT signaling in stem cell biology and regenerative medicine. Curr. Drug Targets 9, 565–570. doi: 10.2174/138945008784911750
Katzeff, J. S., Bright, F., Lo, K., Kril, J. J., Connolly, A., Crossett, B., et al. (2020). Altered serum protein levels in frontotemporal dementia and amyotrophic lateral sclerosis indicate calcium and immunity dysregulation. Sci. Rep. 10:13741. doi: 10.1038/s41598-020-70687-7
Keats, J. J., Fonseca, R., Chesi, M., Schop, R., Baker, A., Chng, W. J., et al. (2007). Promiscuous mutations activate the noncanonical NF-kappaB pathway in multiple myeloma. Cancer Cell 12, 131–144. doi: 10.1016/j.ccr.2007.07.003
Koo, J. H., and Guan, K. L. (2018). Interplay between YAP/TAZ and Metabolism. Cell Metab. 28, 196–206. doi: 10.1016/j.cmet.2018.07.010
Kovacs, G. G. (2017). Concepts and classification of neurodegenerative diseases. Handb. Clin. Neurol. 145, 301–307. doi: 10.1016/B978-0-12-802395-2.00021-3
Kozarova, A., Hudson, J. W., and Vacratsis, P. O. (2011). The dual-specificity phosphatase hYVH1 (DUSP12) is a novel modulator of cellular DNA content. Cell Cycle 10, 1669–1678. doi: 10.4161/cc.10.10.15641
Kula, B., Chen, T. J., and Kukley, M. (2019). Glutamatergic signaling between neurons and oligodendrocyte lineage cells: is it synaptic or non-synaptic? Glia 67, 2071–2091. doi: 10.1002/glia.23617
Kulkarni, H. S., Elvington, M. L., Perng, Y. C., Liszewski, M. K., Byers, D. E., Farkouh, C., et al. (2019). Intracellular C3 protects human airway epithelial cells from stress-associated cell death. Am. J. Respir. Cell. Mol. Biol. 60, 144–157. doi: 10.1165/rcmb.2017-0405OC
Kumar, P., Raman, T., Swain, M. M., Mishra, R., and Pal, A. (2017). Hyperglycemia-induced oxidative-nitrosative stress induces inflammation and neurodegeneration via augmented tuberous sclerosis complex-2 (TSC-2) activation in neuronal cells. Mol. Neurobiol. 54, 238–254. doi: 10.1007/s12035-015-9667-3
Kurihara, M., Mano, T., Saito, Y., Murayama, S., Toda, T., and Iwata, A. (2019). Colocalization of BRCA1 with Tau aggregates in human tauopathies. Brain Sci. 10:7. doi: 10.3390/brainsci10010007
Lauterbach, M. A., Hanke, J. E., Serefidou, M., Mangan, M. S. J., Kolbe, C. C., Hess, T., et al. (2019). Toll-like receptor signaling rewires macrophage metabolism and promotes histone acetylation via ATP-citrate lyase. Immunity 51, 997–1011.e7. doi: 10.1016/j.immuni.2019.11.009
Lévy, J., Haye, D., Marziliano, N., Casu, G., Guimiot, F., Dupont, C., et al. (2018). EFNB2 haploinsufficiency causes a syndromic neurodevelopmental disorder. Clin. Genet. 93, 1141–1147. doi: 10.1111/cge.13234
Liang, Z., Zhao, Y., Ruan, L., Zhu, L., Jin, K., Zhuge, Q., et al. (2017). Impact of aging immune system on neurodegeneration and potential immunotherapies. Prog. Neurobiol. 157, 2–28. doi: 10.1016/j.pneurobio.2017.07.006
Liu, C., Arnold, R., Henriques, G., and Djabali, K. (2019). Inhibition of JAK-STAT signaling with baricitinib reduces inflammation and improves cellular homeostasis in progeria cells. Cells 8:1276. doi: 10.3390/cells8101276
Liu, C. C., Yamazaki, Y., Heckman, M. G., Martens, Y. A., Jia, L., Yamazaki, A., et al. (2020). Tau and apolipoprotein E modulate cerebrovascular tight junction integrity independent of cerebral amyloid angiopathy in Alzheimer’s disease. Alzheimers Dement. 16, 1372–1383. doi: 10.1002/alz.12104
Liu, F., Arias-Vásquez, A., Sleegers, K., Aulchenko, Y. S., Kayser, M., Sanchez-Juan, P., et al. (2007). A genomewide screen for late-onset Alzheimer disease in a genetically isolated Dutch population. Am. J. Hum. Genet. 81, 17–31. doi: 10.1086/518720
López González, I., Garcia-Esparcia, P., Llorens, F., and Ferrer, I. (2016). Genetic and transcriptomic profiles of inflammation in neurodegenerative diseases: Alzheimer, Parkinson, Creutzfeldt-Jakob and Tauopathies. Int. J. Mol. Sci. 17:206. doi: 10.3390/ijms17020206
Lopez-Pelaez, M., Lamont, D. J., Peggie, M., Shpiro, N., Gray, N. S., and Cohen, P. (2014). Protein kinase IKKβ-catalyzed phosphorylation of IRF5 at Ser462 induces its dimerization and nuclear translocation in myeloid cells. Proc. Natl. Acad. Sci. U.S.A. 111, 17432–17437. doi: 10.1073/pnas.1418399111
Luo, W., Wang, Y., Zhang, L., Ren, P., Zhang, C., Li, Y., et al. (2020). Critical role of cytosolic DNA and its sensing adaptor STING in aortic degeneration, dissection, and rupture. Circulation 141, 42–66. doi: 10.1161/CIRCULATIONAHA.119.041460
Mayne, K., White, J. A., McMurran, C. E., Rivera, F. J., and de la Fuente, A. G. (2020). Aging and neurodegenerative disease: is the adaptive immune system a friend or foe? Front. Aging Neurosci. 12:572090. doi: 10.3389/fnagi.2020.572090
McGeer, P. L., Akiyama, H., Itagaki, S., and McGeer, E. G. (1989). Immune system response in Alzheimer’s disease. Can. J. Neurol. Sci. 16(Suppl. 4), 516–527. doi: 10.1017/s0317167100029863
Monteiro, L. F., and Forti, F. L. (2019). Network analysis of DUSP12 partners in the nucleus under genotoxic stress. J. Proteomics 197, 42–52. doi: 10.1016/j.jprot.2019.02.008
Nagatsu, T., Mogi, M., Ichinose, H., and Togari, A. (2000). Changes in cytokines and neurotrophins in Parkinson’s disease. J. Neural Transm. Suppl. 60, 277–290. doi: 10.1007/978-3-7091-6301-6_19
Obulesu, M., and Lakshmi, M. J. (2014). Apoptosis in Alzheimer’s disease: an understanding of the physiology, pathology and therapeutic avenues. Neurochem. Res. 39, 2301–2312. doi: 10.1007/s11064-014-1454-4
Ortegón Salas, C., Schneider, K., Lillig, C. H., and Gellert, M. (2020). Signal-regulated oxidation of proteins via MICAL. Biochem. Soc. Trans. 48, 613–620. doi: 10.1042/BST20190866
Ottone, C., Krusche, B., Whitby, A., Clements, M., Quadrato, G., Pitulescu, M. E., et al. (2014). Direct cell-cell contact with the vascular niche maintains quiescent neural stem cells. Nat. Cell Biol. 16, 1045–1056. doi: 10.1038/ncb3045
Ou, X. M., Chen, K., and Shih, J. C. (2006). Monoamine oxidase A and repressor R1 are involved in apoptotic signaling pathway. Proc. Natl. Acad. Sci. U.S.A. 103, 10923–10928. doi: 10.1073/pnas.0601515103
Pan, Y., Wang, K. S., and Aragam, N. (2011). NTM and NR3C2 polymorphisms influencing intelligence: family-based association studies. Prog. Neuropsychopharmacol. Biol. Psychiatry 35, 154–160. doi: 10.1016/j.pnpbp.2010.10.016
Paul, B. D., Snyder, S. H., and Bohr, V. A. (2021). Signaling by cGAS-STING in neurodegeneration, neuroinflammation, and aging. Trends Neurosci. 44, 83–96. doi: 10.1016/j.tins.2020.10.008
Pîrşcoveanu, D. F. V., Pirici, I., Tudorică, V., Bălşeanu, T. A., Albu, V. C., Bondari, S., et al. (2017). Tau protein in neurodegenerative diseases – a review. Rom. J. Morphol. Embryol. 58, 1141–1150.
Porro, C., Cianciulli, A., Trotta, T., Lofrumento, D. D., and Panaro, M. A. (2019). Curcumin regulates anti-inflammatory responses by JAK/STAT/SOCS signaling pathway in BV-2 microglial cells. Biology (Basel) 8:51. doi: 10.3390/biology8030051
Qiu, Z., and Ghosh, A. (2008). A calcium-dependent switch in a CREST-BRG1 complex regulates activity-dependent gene expression. Neuron 60, 775–787. doi: 10.1016/j.neuron.2008.09.040
Radi, E., Formichi, P., Battisti, C., and Federico, A. (2014). Apoptosis and oxidative stress in neurodegenerative diseases. J. Alzheimers Dis. 42(Suppl. 3), S125–S152. doi: 10.3233/JAD-132738
Roberts, A. W. (2020). Therapeutic development and current uses of BCL-2 inhibition. Hematology Am. Soc. Hematol. Educ. Program. 2020, 1–9. doi: 10.1182/hematology.2020000154
Saigoh, K., Yoshimura, S., Izumikawa, T., Miyata, S., Tabara, Y., Matsushita, T., et al. (2016). Chondroitin sulfate β-1,4-N-acetylgalactosaminyltransferase-1 (ChGn-1) polymorphism: association with progression of multiple sclerosis. Neurosci. Res. 108, 55–59. doi: 10.1016/j.neures.2016.01.002
Salmerón, A., Janzen, J., Soneji, Y., Bump, N., Kamens, J., Allen, H., et al. (2001). Direct phosphorylation of NF-kappaB1 p105 by the IkappaB kinase complex on serine 927 is essential for signal-induced p105 proteolysis. J. Biol. Chem. 276, 22215–22222. doi: 10.1074/jbc.M101754200
Santiago, J. A., Bottero, V., and Potashkin, J. A. (2020). Transcriptomic and network analysis identifies shared and unique pathways across dementia spectrum disorders. Int. J. Mol. Sci. 21:2050. doi: 10.3390/ijms21062050
Santos, A., Parrini, M. C., and Camonis, J. (2016). RalGPS2 is essential for survival and cell cycle progression of lung cancer cells independently of its established substrates ral GTPases. PLoS One 11:e0154840. doi: 10.1371/journal.pone.0154840
Schmidt, E. F., Shim, S. O., and Strittmatter, S. M. (2008). Release of MICAL autoinhibition by semaphorin-plexin signaling promotes interaction with collapsin response mediator protein. J. Neurosci. 28, 2287–2297. doi: 10.1523/JNEUROSCI.5646-07.2008
Shen, H., Smith, D. E., Keep, R. F., and Brosius, F. C. III (2004). Immunolocalization of the proton-coupled oligopeptide transporter PEPT2 in developing rat brain. Mol. Pharm. 1, 248–256. doi: 10.1021/mp049944b
Soto, I., Grabowska, W. A., Onos, K. D., Graham, L. C., Jackson, H. M., Simeone, S. N., et al. (2016). Meox2 haploinsufficiency increases neuronal cell loss in a mouse model of Alzheimer’s disease. Neurobiol. Aging 42, 50–60. doi: 10.1016/j.neurobiolaging.2016.02.025
Starr, J. M. (2019). Ageing and epigenetics: linking neurodevelopmental and neurodegenerative disorders. Dev. Med. Child Neurol. 61, 1134–1138. doi: 10.1111/dmcn.14210
Stephenson, J., Nutma, E., van der Valk, P., and Amor, S. (2018). Inflammation in CNS neurodegenerative diseases. Immunology 154, 204–219. doi: 10.1111/imm.12922
Stopa, E. G., Tanis, K. Q., Miller, M. C., Nikonova, E. V., Podtelezhnikov, A. A., Finney, E. M., et al. (2018). Comparative transcriptomics of choroid plexus in Alzheimer’s disease, frontotemporal dementia and Huntington’s disease: implications for CSF homeostasis. Fluids Barriers CNS 15, 18. doi: 10.1186/s12987-018-0102-9
Su, L., Lv, X., Xu, J., Yin, D., Zhang, H., Li, Y., et al. (2009). Neural stem cell differentiation is mediated by integrin beta4 in vitro. Int. J. Biochem. Cell Biol. 41, 916–924. doi: 10.1016/j.biocel.2008.09.001
Tang, F., Wang, B., Li, N., Wu, Y., Jia, J., Suo, T., et al. (2011). RNF185, a novel mitochondrial ubiquitin E3 ligase, regulates autophagy through interaction with BNIP1. PLoS One 6:e24367. doi: 10.1371/journal.pone.0024367
Troullinaki, M., Alexaki, V. I., Mitroulis, I., Witt, A., Klotzsche-von Ameln, A., Chung, K. J., et al. (2019). Nerve growth factor regulates endothelial cell survival and pathological retinal angiogenesis. J. Cell Mol. Med. 23, 2362–2371. doi: 10.1111/jcmm.14002
Wu, Z., Guo, H., Chow, N., Sallstrom, J., Bell, R. D., Deane, R., et al. (2005). Role of the MEOX2 homeobox gene in neurovascular dysfunction in Alzheimer disease. Nat. Med. 11, 959–965. doi: 10.1038/nm1287
Xu, D., Zhao, H., Jin, M., Zhu, H., Shan, B., Geng, J., et al. (2020). Modulating TRADD to restore cellular homeostasis and inhibit apoptosis. Nature 587, 133–138. doi: 10.1038/s41586-020-2757-z
Yan, Z., Gibson, S. A., Buckley, J. A., Qin, H., and Benveniste, E. N. (2018). Role of the JAK/STAT signaling pathway in regulation of innate immunity in neuroinflammatory diseases. Clin. Immunol. 189, 4–13. doi: 10.1016/j.clim.2016.09.014
Yosefzon, Y., Soteriou, D., Feldman, A., Kostic, L., Koren, E., Brown, S., et al. (2018). Caspase-3 regulates YAP-dependent cell proliferation and organ size. Mol. Cell 70, 573–587.e4. doi: 10.1016/j.molcel.2018.04.019
Zhong, S., Pei, D., Shi, L., Cui, Y., and Hong, Z. (2019). Ephrin-B2 inhibits Aβ25-35-induced apoptosis by alleviating endoplasmic reticulum stress and promoting autophagy in HT22 cells. Neurosci. Lett. 704, 50–56. doi: 10.1016/j.neulet.2019.03.028
Keywords: aging, neurodegenerative disease, cellular homeostasis, network analysis, supervised machine learning
Citation: Shi F, He Y, Chen Y, Yin X, Sha X and Wang Y (2021) Comparative Analysis of Multiple Neurodegenerative Diseases Based on Advanced Epigenetic Aging Brain. Front. Genet. 12:657636. doi: 10.3389/fgene.2021.657636
Received: 23 January 2021; Accepted: 16 April 2021;
Published: 20 May 2021.
Edited by:
Xiao Dong, University of Minnesota, United StatesReviewed by:
Zhipeng Liu, Purdue University, United StatesCopyright © 2021 Shi, He, Chen, Yin, Sha and Wang. This is an open-access article distributed under the terms of the Creative Commons Attribution License (CC BY). The use, distribution or reproduction in other forums is permitted, provided the original author(s) and the copyright owner(s) are credited and that the original publication in this journal is cited, in accordance with accepted academic practice. No use, distribution or reproduction is permitted which does not comply with these terms.
*Correspondence: Yin Wang, Y2hpbmF3YW5neWluQGZveG1haWwuY29t
Disclaimer: All claims expressed in this article are solely those of the authors and do not necessarily represent those of their affiliated organizations, or those of the publisher, the editors and the reviewers. Any product that may be evaluated in this article or claim that may be made by its manufacturer is not guaranteed or endorsed by the publisher.
Research integrity at Frontiers
Learn more about the work of our research integrity team to safeguard the quality of each article we publish.