- 1Hunan Key Laboratory of Viral Hepatitis, Xiangya Hospital, Central South University, Changsha, China
- 2Department of Infectious Disease, Xiangya Hospital, Central South University, Changsha, China
- 3Department of Dermatology, Xiangya Hospital, Central South University, Changsha, China
- 4National Clinical Research Center for Geriatric Disorders, Xiangya Hospital, Central South University, Changsha, China
Background: Enhancer RNAs (eRNAs) are intergenic long non-coding RNAs (lncRNAs) that participate in the progression of malignancies by targeting tumor-related genes and immune checkpoints. However, the potential role of eRNAs in hepatocellular carcinoma (HCC) is unclear. In this study, we aimed to construct an immune-related eRNA prognostic model that could be used to prospectively assess the prognosis of patients with HCC.
Methods: Gene expression profiles of patients with HCC were downloaded from The Cancer Genome Atlas (TCGA). The eRNAs co-expressed from immune genes were identified as immune-related eRNAs. Cox regression analyses were applied in a training cohort to construct an immune-related eRNA signature (IReRS), that was subsequently used to analyze a testing cohort and combination of the two cohorts. Kaplan-Meier and receiver operating characteristic (ROC) curves were used to validate the predictive effect in the three cohorts. Gene Set Enrishment Analysis (GSEA) computation was used to identify an IReRS-related signaling pathway. A web-based cell type identification by estimating relative subsets of RNA transcripts (CIBERSORT) computation was used to evaluate the relationship between the IReRS and infiltrating immune cells.
Results: A total of sixty-four immune-related eRNAs (IReRNAs) was identified in HCC, and 14 IReRNAs were associated with overall survival (OS). Five IReRNAs were used for constructing an immune-related eRNA signature (IReRS), which was shown to correlate with poor survival and to be an independent prognostic biomarker for HCC. The GSEA results showed that the IReRS was correlated to cancer-related and immune-related pathways. Moreover, we found that IReRS was correlated to infiltrating immune cells, including CD8+ T cells and M0 macrophages. Finally, differential expressions of the five risk IReRNAs in tumor tissues vs. adjacent normal tissues and their prognostic values were verified, in which the AL445524.1 may function as an oncogene that affects prognosis partly by regulating CD4-CLTA4 related genes.
Conclusion: Our results suggest that the IReRS could serve as a biomarker for predicting prognosis in patients with HCC. Additionally, it may be correlated to the tumor immune microenvironment and could also be used as a biomarker in immunotherapy for HCC.
Introduction
Hepatocellular carcinoma (HCC) is the main type of primary liver cancer, which is the fifth most common cancer and the second most frequent cause of cancer-associated deaths (European Association for the Study of the Liver, 2018). A poor prognosis in patients with HCC was in part a result of incomplete understanding of epigenetic and heterogeneous gene alterations to reliably obtain predictable biomarkers (El-Serag and Rudolph, 2007). A liable assessment model is needed to improve survival prognosis and appropriate treatment decision in patients with HCC.
HCC is closely related to chronic liver inflammation and is considered to be a typical immunogenic cancer (Pardee and Butterfield, 2012). Tumor associated macrophages (TAM) and myeloid-derived suppressive cells (MDSC) in the HCC microenvironment release cytokines, such as IL-6 and tumor necrosis factor (TNF), thereby inducing cancer cell proliferation and inhibiting apoptosis through activation of NF-κB and Stat3 (Iliopoulos et al., 2009; Yu et al., 2009). Immunosuppressive cells in the HCC tumor microenvironment can interfere with immune monitoring, leading to tumor’s immune evasion or immune escape (Zamarron and Chen, 2011). A scoring of a gene ontology and pathway analysis has shown that HCC-related genes are also closely related to genes involving in immune microenvironment (Ge et al., 2020). Immunotherapies targeting co-inhibitory receptors (i.e., CTLA4 and PD1) have been used to treat some cancers, such as pulmonary cancers and metastatic melanoma (Wolchok, 2015; Chikuma, 2017). Therefore, an immune-related prognostic model for HCC is worth exploring.
Long non-coding RNAs (lncRNAs) act as important regulators in the tumor progression of various cancers, including HCC (Shi et al., 2016; Li et al., 2017; Yu et al., 2020). Enhancer RNA (eRNA) is a type of lncRNA transcribed from intergenic enhancers, and exists widely in most human cells and tissues (Chen et al., 2017). Emerging evidence suggests that eRNAs are integral components of enhancer function. Recent studies have revealed the involvement of eRNAs in the progression of cancers (Liu et al., 2018; Zhang Z. et al., 2019). For example, eRNA KLK3e is able to activate KLK3 and to alter androgen receptor dependent gene expression, and subsequently to promote cancer cell proliferation in a prostate cancer line (Hsieh et al., 2014); NET1e is highly expressed in breast invasive carcinoma, and is associated with poor prognosis (Li et al., 2015); TAOK1e is related to the overall survival of patients with clear cell renal cell carcinoma; EN1e is highly expressed in breast carcinoma; CELF2e is highly expressed in stage III stomach adenocarcinoma; and APH1A is highly expressed in grade 3 HCC (Zhang Z. et al., 2019). Studies have shown that lncRNAs are also closely related to the immune microenvironment of HCC (Hong et al., 2020; Kong et al., 2020). However, the prognostic role of immune-related eRNAs in HCC remains unclear.
In this study, we explored the prognostic significance of immune-related eRNAs (IReRNAs) in HCC from The Cancer Genome Atlas (TCGA) database. We screened IReRNAs to construct a novel eRNA prognostic model, termed immune-related eRNA signature (IReRS), which was shown here to correlate with poor survival and to be an independent prognostic factor. Moreover, the IReRS was correlated to immune-cell infiltration. Finally, a risk eRNA, AL445524.1, was identified as a key HCC-related eRNA and closely related to tumor immunity by regulating downstream genes.
Materials and Methods
Identification of Immune-Related eRNAs
A total of 567 immune-related genes (IRGs) in HCC from the Molecular Signature Database v4. 0 were identified by GSEA. The expression data and clinical data of HCC were downloaded from TCGA. This dataset contains 371 HCC tumor samples and 54 adjacent normal samples. Samples without complete survival information were removed. Ultimately, 366 HCC samples were included for subsequent analysis. eRNAs were predicted using PreSTIGE as previously described (Murakami et al., 2015; Gu et al., 2019). Pearson correlation analysis was carried out between the expression level of eRNAs and the expression level of IRGs to identify immune-related eRNAs (Pearson correlation coefficient > 0.4, p < 0. 001).
Random Grouping of Data
The expression level of immune-related eRNAs (HtSeq-FPKM) and clinical data of 366 HCC samples were downloaded from TCGA on June 16, 2020. A total of 1,580 eRNAs were identified in HCC tissues from TCGA dataset. Using Pearson correlation analysis, 64 Immune-related eRNAs were identified in HCC (R > 0.4, p < 0.001). The 366 samples were randomly divided into two groups, a training cohort (60%) and a testing cohort (40%), using the R package “caret.” The clinical characteristics of the patients with HCC are shown in Table 1.
Construction of a Prognostic IReRS
We performed Cox analysis and lasso regression to establish the IReRS in the training cohort, and then verified the IReRS in both the testing cohort and the entire cohort. The risk score was calculated using eRNA expression and coefficient values (Gu et al., 2019). Fourteen eRNAs were identified as being associated with overall survival (OS) by the univariate Cox regression models (Figure 1A). In addition, the least absolute shrinkage and selection operator (LASSO) regression analysis was performed (Figures 1B,C), and finally, 5 immune-related risk eRNAs (FAM120AOS, AL445524.1, AC073257.2, LINC00513, STK3) were identified to construct the risk model by the multivariate Cox regression analysis (Figure 1D). The formula to calculate the risk score for the IReRS is: 0.4705 × expression of FAM120AOS + 0.2308 × expression of AL445524.1 + 0.6886 × expression of AC073257.2 + 0.3875 × expression of LINC00513 + 0.4941 × expression of STK3.
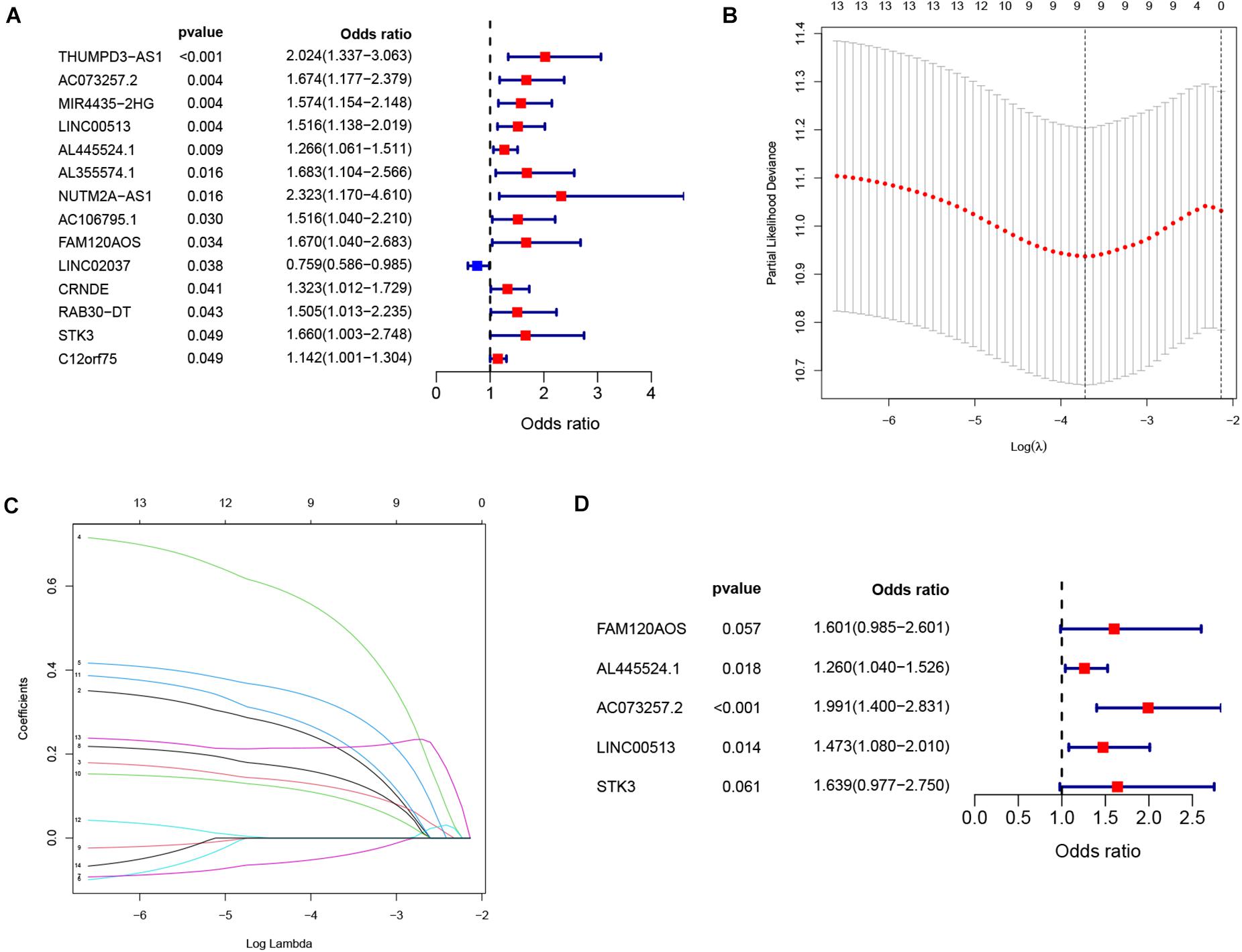
Figure 1. Identification of immune-related eRNAs from hepatocellular carcinoma. (A) Univariate Cox regression models identified 14 immune-related eRNAs that are associated with OS. Two analyses (B,C) by a lasso regression further fine-tuned the selection of immune-related eRNAs. (D) Multivariate Cox regression analysis identified five immune-related eRNAs for the construction of a prognostic model.
The median risk score in the training cohort served as the cutoff value, which was applied to divide the entire cohort into a low-risk group and a high-risk group. For survival analysis, Kaplan–Meier survival curves were constructed for both the low and high-risk groups in all three cohorts using R package “survival,” and two-sided log-rank test of p < 0.05 was considered statistically significantly.
The prognostic value of the IReRS was checked using the R package “survival.” Furthermore, a survival receiver operating characteristic (ROC) curve was constructed using the R package “survival ROC” to verify the prognostic performance.
A nomogram was constructed from the risk score and other clinical parameters in each cohort. ROC curves were used to compare the prognostic value of risk scores with other clinical features using R software “ROC package.”
Gene Set Enrichment Analysis
We analyzed the enrichment terms in the entire TCGA cohort using GSEA software version 4.1.1 (Cambridge, MA, United States) to reveal pathways related to IReRS (Mootha et al., 2003; Subramanian et al., 2005). The gene sets of “ h. all. v7.1. symbols. gmt (cancer hallmarks) and c7. all. v7.1. symbols. gmt (Immunologic signatures) ” were selected for GSEA analysis, and a p < 0.05 along with a false discovery rate < 0.05 was considered statistically significant.
Infiltrating Immune Cells in HCC
The data on infiltration of immune cells in HCC were obtained using CIBERSORT (Newman et al., 2015). Differences of infiltrating immune cells in high- and low-risk HCC samples were examined by Wilcoxon. Using the R ‘‘ESTIMATE’’ software package, we calculated tumor microenvironment score. DNA methylation Stemness score (DNASS) and RNA Stemness score (RNASS) were downloaded from the UCSC database1. The correlation between risk score and tumor microenvironment and tumor stem cell score in HCC was calculated using the R software package (“CorrPlot”).
Immune Checkpoint Blockade (ICB) Analysis in HCC
Six genes were previously reported as key targets of immune checkpoint inhibitors: TIM-3, IDO1, CTLA-4, PD-1, PD-L1, and PD-L2 (Goodman et al., 2017; Kim et al., 2017; Nishino et al., 2017). The difference of immune checkpoint inhibitor treatment in malignant tumor is related to the difference of immune checkpoint gene expression (Gu et al., 2019). The correlation between the six immune checkpoint inhibitors and our immune-related signature was analyzed to explore the possible role of the IReRNA and IReRS in HCC ICB therapy by Spearman’s correlation coefficient.
The Expression of the Immune-Related Risk eRNAs
The expression level of risk eRNAs were identified from TCGA datasets. The R package ‘‘survival’’ was then used to study the prognostic values of risk eRNAs in HCC. The tSNE analysis of risk eRNA-associated genes was carried out using web tools2.
Statistical Analysis
The Wilcoxon signed-rank test was used for the correlation analysis between IReRS and the clinical characteristics of patients with HCC. The relationships among IReRS and immune cells, immune cell markers, and ICB were analyzed by Spearman’s correlation coefficient. Kaplan-Meier curves were used for survival analysis. Among 567 IRGs, the genes associated with AL445524.1 were screened by Pearson correlation analysis.
Results
Validation of the IReRS in TCGA
Patients in high-risk group had a significantly worse OS in the training cohort, testing cohort and entire cohort (Figures 2A,D,G, p = 4.238e-3, p = 3.944e-2, and p = 5.504e-4, respectively). The survival time decreased as the risk score increased (Figures 2B,E,H). The computed area under the curve (AUC) values of the IReRS were 0.704, 0.692, and 0.699 for survival time of 1, 2, and 3 years, respectively, in the training cohort (Figure 2C), and the corresponding values of 0.669, 0.602, and 0.571 in the testing cohort (Figure 2F) and of 0.688, 0.653, and 0.642 in the entire cohort (Figure 2I) were also comparable. The results indicate that the model has good predictive ability, specificity, and sensitivity.
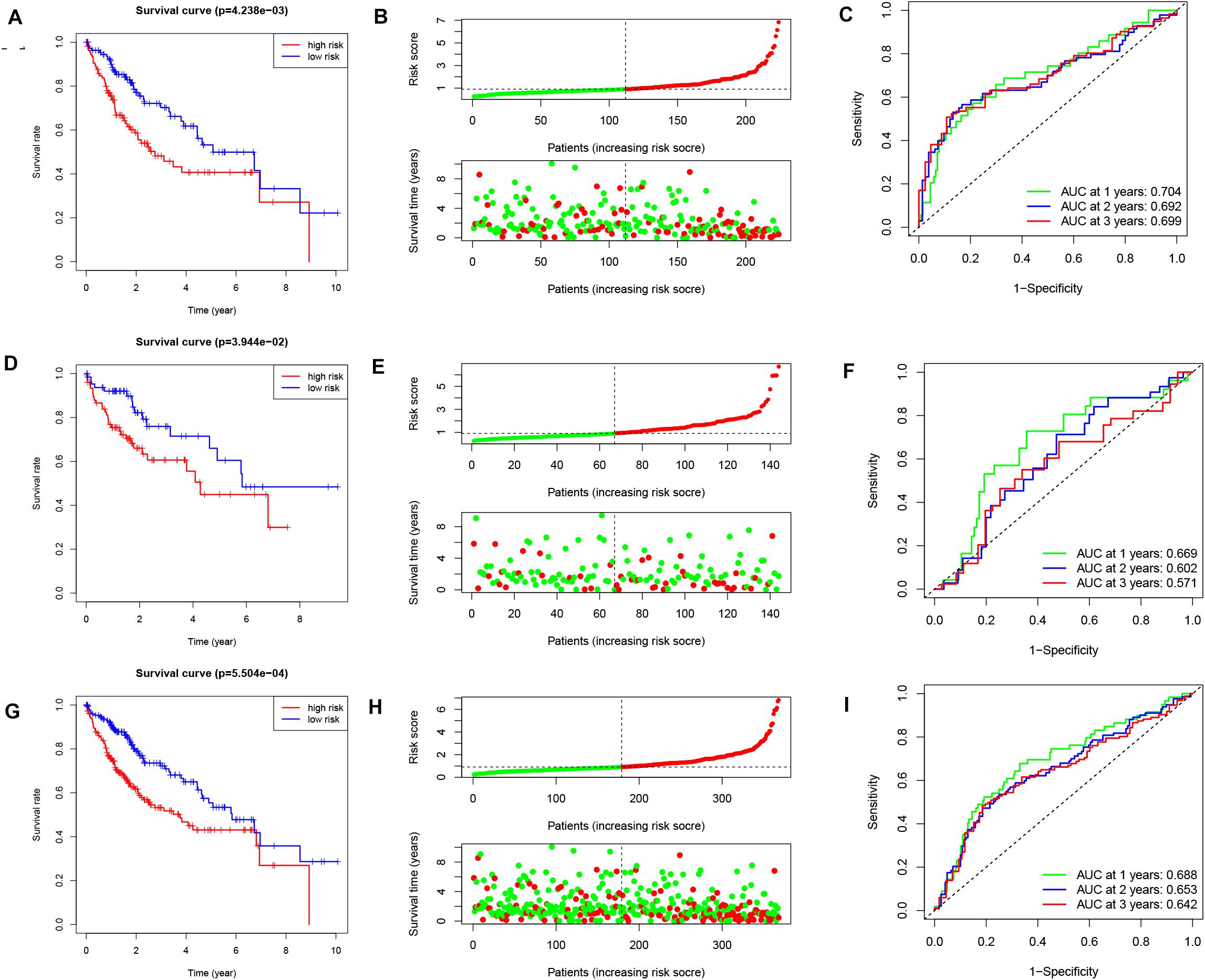
Figure 2. Kaplan–Meier survival analysis, risk score analysis and ROC curve analysis. Kaplan-Meier survival curve, risk score, and ROC curve analysis of the immune-related eRNA signature were illustrated within the training cohort [(A–C), respectively], within the testing cohort [(D–F), respectively], and within the entire cohort [(G–I), respectively].
Correlations Between the Clinicopathologic Characteristics and the IReRS in HCC
We next analyzed the relationships between IReRS and clinical characteristics, including age (≤55, >55 years old), race, gender, survival status, pathological tumor stage, recurrence, and histological grade by Pearson correlation analysis. The IReRS risk score, however, did not significantly correlate with clinical characteristics in training or in testing cohort. In the entire cohort, the risk score was found to be significantly correlated with histological grade and survival status (Figure 3).
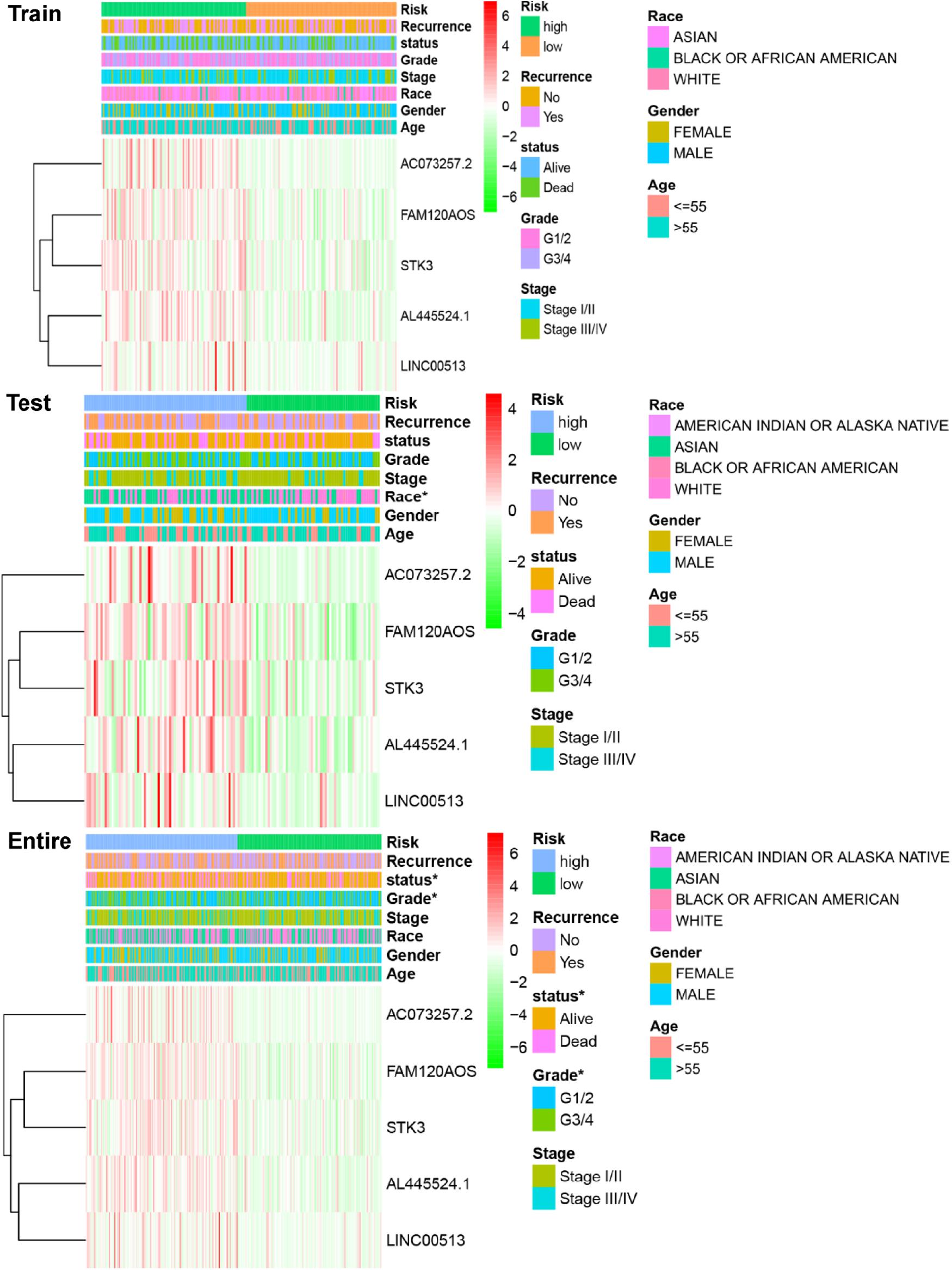
Figure 3. Relationships between the risk score and clinical characteristics of HCC in three cohorts. Significant associations of risk scores with patients’ survival status and histological grade in the entire cohort (bottom panel) were observed. *p < 0.05.
Identification of Independent Prognostic Factors
We next used univariate and multivariate Cox regression analyses to examine the independent prognostic role of IReRS with clinical characteristics, including age, gender, histological grade, pathological tumor stage, recurrence, and riskscore. As shown in Figure 4, univariate and multivariate Cox regression analyses showed that the pathological tumor stage and risk score were independent prognostic factors for OS. Multivariate Cox regression analysis further identified the IReRS to be an independent prognostic factor for OS in the training cohort and the entire cohort with an HR of 1. 625 (1.352–1.953) and 1.256 (1.083–1.458), respectively. The results suggest that IReRS, in addition to the tumor stage and risk score, could be used as an independent prognostic factor for OS in patients with HCC.
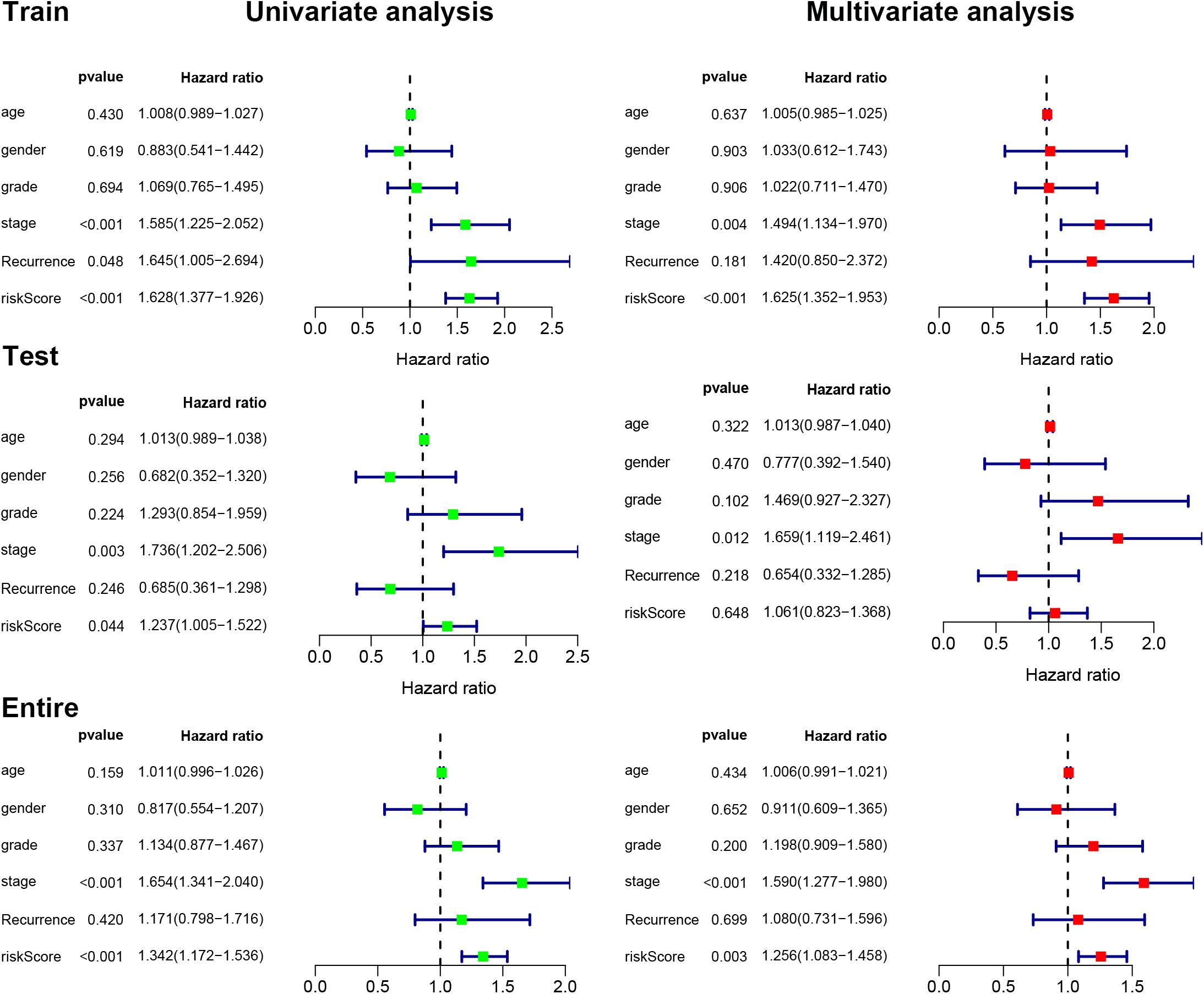
Figure 4. Univariate and multivariate Cox regression analyses. Univariate and multivariate Cox regression analyses illustrated the clinical features within the training, testing, and entire cohorts.
Construction of the Prognostic Nomogram
We constructed a nomogram to predict OS in patients (311 HCC patients with known clinical characters) with HCC based on the risk score, age, gender, histological grade, pathological tumor stage, and recurrence (Figure 5). Subsequently, we used the ROC curve to assess the accuracy of the risk model (Figure 5). The computed AUC values of risk score and tumor stage were 0.738 and 0.690, respectively, in the training cohort, which are comparable to 0.642 and 0.586, respectively, in the testing cohort, and 0.702 and 0.646, respectively, in the entire cohort. The results suggest that the prognostic models constructed with the 5 immune-related eRNAs may be reliable predictors of OS in patients with HCC.
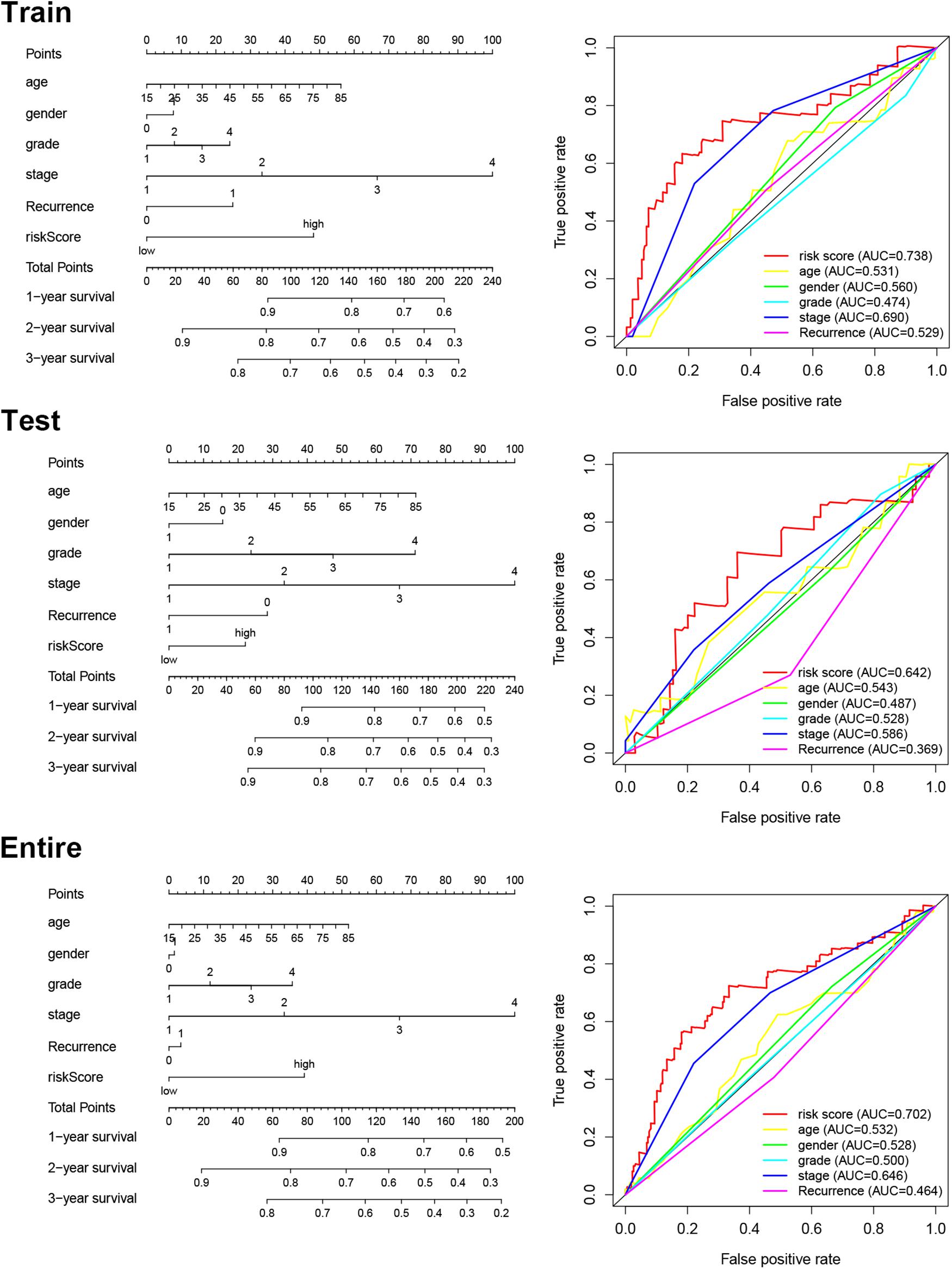
Figure 5. Nomogram for predicting OS and ROC curves of the risk scores and clinical features in each cohort.
The IReRS-Mediated Signaling Pathway in HCC
We next used GSEA to reveal the IReRS-mediated signaling pathway. As shown in Figure 6A, six cancer hallmarks were significantly enriched in high-risk HCC, including basal transcription factors, bladder cancer, cell cycle, notch signaling pathway, pancreatic cancer and pathways in cancer. Moreover, the GSEA results showed that high score of IReRS was also associated with numerous immune processes (Figure 6B), including GSE10239_NAIVE_VS_KLRG1NT_EFF_CD8_TCELL_DN, GS E14308_TH1_VS_NAIVE_CD4_TCELL_UP, GSE14908_ATO PIC_VS_NONATOPIC_PATIENT_RESTING_CD4_TCELL_DN, GSE15930_NAIVE_VS_24H_IN_VITRO _STIM_INFAB_CD8_ TCELL_DN, GSE21238_TFH_ VS_GERMINAL_CENTER_ TFH_CD4_TCELL_DN, GSE2770_IL12_ACT_VS_ACT_CD4_ TCELL_6H_UP, and GSE39820_TGFBETA3_IL6_VS_TGFBET A3_IL6_IL23A_TREATED_CD4_TCELL_DN These results indicated that IReRS may be involved in the progression of HCC by regulating the tumor immune microenvironment.
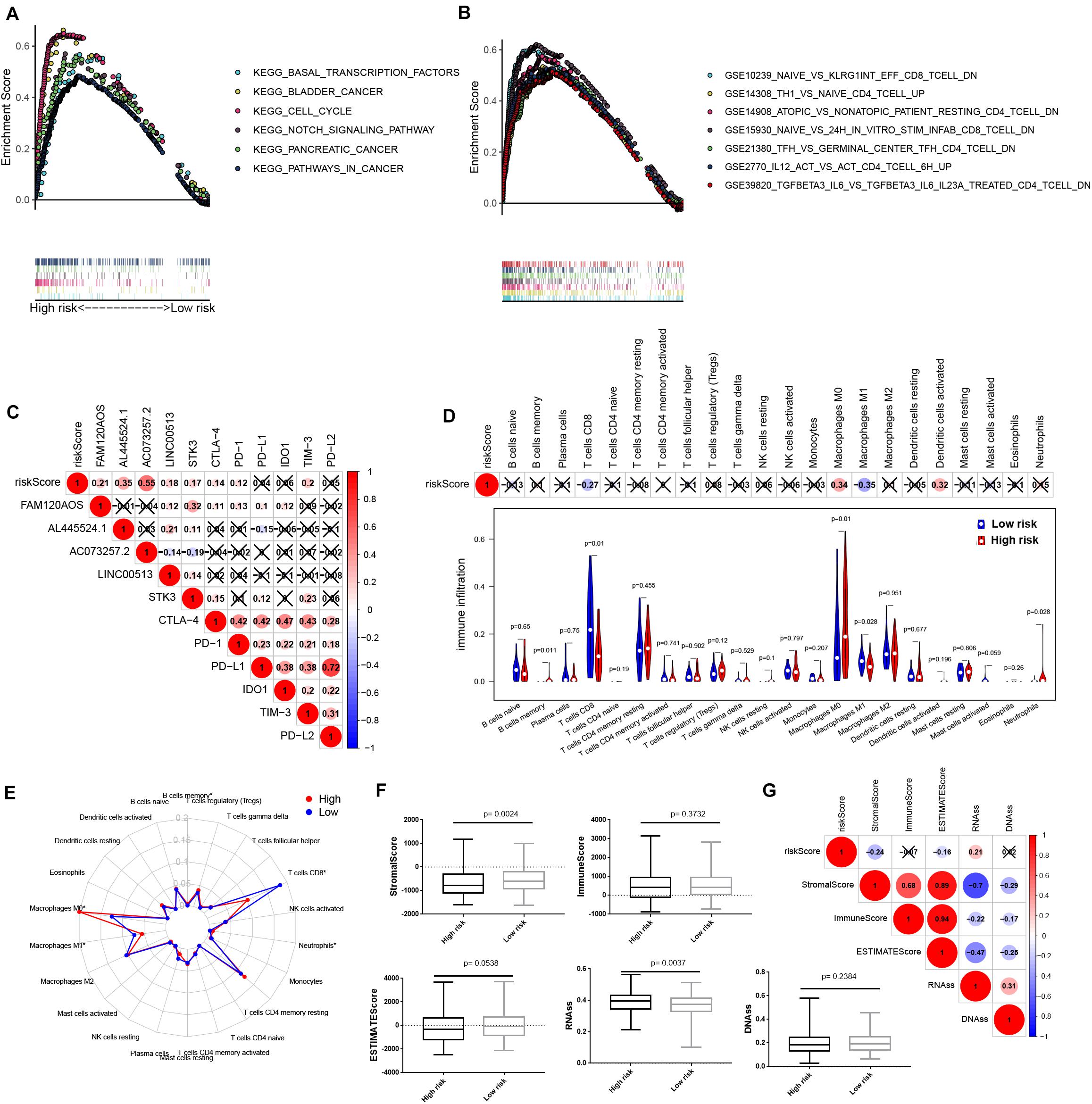
Figure 6. IReRS GSEA and relationship with immune checkpoint and immune cell infiltration. (A) Six cancer hallmarks were significantly enriched in the high-risk patients. (B) Seven immune signatures were significantly enriched in the high-risk patients. (C) Associations between immune-related eRNA signature and immune checkpoint genes were detected. Violin plot (D), Radar map (E) were used to display the differences of the infiltrating immune cells in HCC patients between high- and low-risk groups. Boxplot (F) and correlation heat map (G) illustrated the correlations of risk score to immunoscore and tumor stem cell in HCC.
The Relationship Between IReRS and Immune Cell Infiltration
The application of ICB for immunotherapy has become a promising aid to the treatment of various cancers, including HCC (Goodman et al., 2017; Kim et al., 2017; Nishino et al., 2017). Therefore, we investigated the possible role of our IReRS in the ICB therapy of HCC (Figure 6C) by evaluating the relationships of the six well known targets of immune checkpoint inhibitors (including TIM-3, IDO1, CTLA-4, PD-1, PD-L1, and PD-L2) to the IReRS and immune-related eRNAs. We found that the IReRS was minimally correlated to the expression of the six genes.
Next, we assessed the relationship between the IReRS and tumor immune microenvironment in HCC. Immune cell infiltration was obtained using CIBERSORT and the correlation between the IReRS risk score and immune cell infiltration was analyzed. We found that infiltration of CD8 T cells was negatively correlated with risk score, while the presence of macrophages M0 was positively correlated with risk score (Figures 6D,E). We next analyzed the correlation of the risk score to tumor microenvironment and tumor stem cell score in HCC, and found negative correlation with stromal score but positive correlation with RNAss (Figures 6F,G).
Association of AL445524.1 With Poor Prognosis
We next analyzed the expression and prognosis of the five modeled risk eRNAs. As shown in Figure 7A, all 5 risk eRNAs were significantly upregulated in HCC tissues compared to normal tissues. Moreover, both AL445524.1 (p = 0.015) and LINC00513 (P = 0.04) were associated with poor OS (Figure 7B). Further analyses of eRNA-related genes using Pearson correlation analysis with a significant level of R > 0.3 and p < 0.05 identified 35 genes to be positively associated with AL445524.1 (Figure 7C), in which RABIF, CYTOR, CCT3, NME1, and KRTCAP2 were highly correlated with a significant level of R > 0.5 and p < 0.05. Then, we analyzed expression of the 5 eRNA-related genes in immune cells of HCC using tSNE cluster web tool (see text footnote 2). As shown in Figure 7D, the five genes were expressed more abundantly in the C8_CD4-CTLA4 bundle of HCC tissues than in normal tissues.
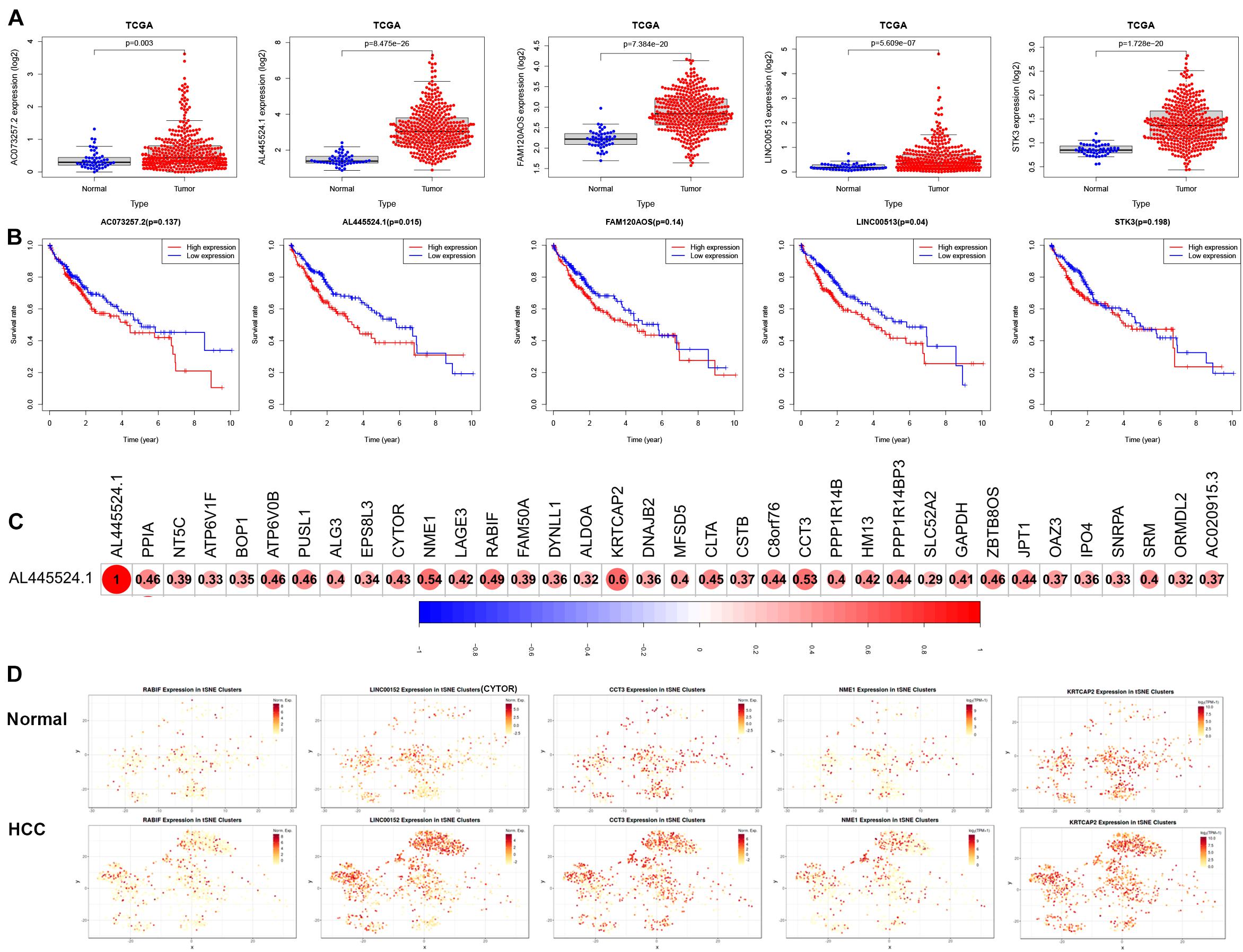
Figure 7. The expression of 5 modeled risk eRNAs and associated genes in HCC prognosis. (A) Risk eRNAs are differentially expressed between TCGA normal and HCC tissues. (B) Survival curves analyzed the role between high and low expression of risk eRNA. (C) AL445524.1 is positively associated with 35 genes. (D) The tSNE analysis of AL445524.1 associated five genes.
Discussion and Conclusion
HCC is an immunologically-related malignancy, often arising from the context of persistent chronic inflammatory liver diseases, such as non-alcoholic fatty liver disease, chronic viral hepatitis B, and chronic viral hepatitis C (Champiat et al., 2016). The shift in the immune response from anti-tumor status to tumor tolerance contributes to the development and progression of HCC (Zamarron and Chen, 2011). Immune cells and immune-related genes have been reported as novel prognosis biomarkers and therapeutic targets for HCC (Jayant et al., 2020; Zhang et al., 2020). In this study, we aimed to construct a novel immune-related eRNA prognostic signature of HCC, and to characterize the eRNA signature as a useful prognostic tool and to identify therapeutic targets for HCC.
eRNAs have been found to be an important regulators of the immune response, and are involved in a mutiple tumorigenic signaling pathways, such as p53, PPARr, and immune checkpoints (Melo et al., 2013; Jayant et al., 2020), which are closely related to malignancy formation and progression. In this study, we constructed a five-gene IReRS as a new prognostic model and validated its predictive utility. On three datasets (the entire TCGA cohort and two subgroups), the predictive utility of the prognostic model was verified by Kaplan-Meier survival analyses and ROC curves. Both the clinical parameters and IReRS risk scores, in Cox regression analyses or constructed in a prognostic nomogram, predict the prognosis of HCC. More importantly, the predictive value of risk scores is superior to that of clinical parameters.
Next, the GSEA analysis showed that the IReRS was associated with multiple cancer related pathways and immune related pathways. Moreover, risk score was negatively correlated with CD8+ T cell and positively correlated with M0 macrophage infiltration in HCC. It was reported that an increase in CD8+ tumor infiltrating lymphocyte (TIL) was associated with improved overall survival in patients with HCC (Garnelo et al., 2017). Depletion of CD8+ TIL was found to be associated with the development of HCC (Wang et al., 2019).CD8 + T cells are important effector of TIL subsets in HCC, and play an important role in tumor immune surveillance and tumor eradication (Flecken et al., 2014). In contrast, immunosuppressive tumors associated macrophages (TAMs) are relevant to poor prognosis in HCC (Zhang Q. et al., 2019). Recently, accumulation of a large number of non-polarized M0 macrophages has been found in glioblastoma (Gabrusiewicz et al., 2016), and has been linked to tumor progression (Huang et al., 2020). In conclusion, risk score based on IReRS is an important predictor for the dysfunction of tumor immune microenvironment.
Finally, we analyzed the role and potential mechanism of 5 risk eRNAs. There have been limited PubMed reports on these five eRNAs in cancers, in which the FAM120AOS is highly expressed in colorectal cancer (Akbari et al., 2020) and glioma (Nikas, 2016); the AC073257.2 targeting GLI2, affects the growth and proliferation of cellular keloids (Huang et al., 2018); and the LINC00513 is identified in systemic lupus erythematosus (SLE) and associated with the type I interferon pathway by promoting phosphorylation of STAT1 and STAT2 proteins (Xue et al., 2018). In our study, all risk eRNAs were significantly upregulated in HCC tissues compared to adjacent normal tissues. Moreover, the risk gene AL445524.1 was demonstrated to be associated significantly with poor prognosis of OS and immune-related genes including RABIF, CYTOR, CCT3, NME1, and KRTCAP2. The RABIF has been identified to be a biomarker candidate of breast cancer development (Switnicki et al., 2016) and associated with HCC (Li et al., 2021). The CYTOR has been reported being upregulated in many cancers, including HCC, lung adenocarcinoma, and renal cell carcinoma (Liang et al., 2018). The CCT3 acts upstream of YAP and TFCP2 as a potential target and tumour biomarker in liver cancer (Liu et al., 2019). The NME1 promotes dynamin 2 oligomerization and regulated tumor cell endocytosis, motility, and metastasis (Khan et al., 2019). KRTCAP2, MUC1, and TRIM46 are found to be highly expressed as chimeras in ovarian cancer (Kannan et al., 2015). Our study further shows that these 5 genes are highly expressed in the CD4-CLTA4 cluster of HCC. In a single-arm phase II study of 21 patients with advanced liver cancer, Child-Pugh type A or B, and/or hepatitis C, the anti-CTLA4 antibody tremelimumab is notably able to control the liver diseases (Sangro et al., 2013). Therefore, the AL445524.1 may be used as an indicator for therapeutic choice of anti-CLTA4.
In summary, the immune-related eRNAs and modeled eRNA signature are independent prognostic biomarkers and are predictors of the immune microenvironment in HCC. Thus this study provides insights into a potentially novel predictive biomarker for the prognosis and survival of patients with HCC, and is expected to provide some possible options of improved immunotherapies.
Data Availability Statement
The original contributions presented in the study are included in the article/supplementary material, further inquiries can be directed to the corresponding author/s.
Author Contributions
SC and YZ: methodology and writing—original draft. XH and RC: investigation. XH, RC, SC, and YZ: writing—review and editing. All authors contributed to the article and approved the submitted version.
Funding
This work was supported by the National Natural Science Foundation of China (81703149, 82070613, and 81970523), the National Natural Sciences Foundation of Hunan province (2019JJ30041, 2020JJ5950, and 2020JJ4877), and the Innovation-Driven Project of Central South University (2020CX044).
Conflict of Interest
The authors declare that the research was conducted in the absence of any commercial or financial relationships that could be construed as a potential conflict of interest.
Acknowledgments
The results (published or shown) here are in whole or part based upon data generated by the TCGA Research Network: https://www.cancer.gov/tcga. We thank AiMi Academic Services (www.aimieditor.com) for english language editing and review services.
Abbreviations
AUC, the area under receiver operating characteristic curve; CTLA-4, cytotoxic T-lymphocyte antigen 4; GSEA, Gene Set Enrichment Analysis; HCC, hepatocellular carcinoma; ICB, immune checkpoint blockade; IReRS, immune-related eRNA signature; IRGs, immune-related genes; MDSC, myeloid-derived suppressive cells; OS, overall survival; PD-1, programmed death 1; PD-L1, programmed death ligand 1; PD-L2, programmed death ligand 2; PTGES2, prostaglandin E synthase 2; ROC, receiver operating characteristic; TAM, tumor associated macrophages; TCGA, The Cancer Genome Atlas; TIM-3, T-cell immunoglobulin domain and mucin domain-containing molecule-3.
Footnotes
References
Akbari, F., Peymani, M., Salehzadeh, A., and Ghaedi, K. (2020). Integrative in silico and in vitro transcriptomics analysis revealed new lncRNAs related to intrinsic apoptotic genes in colorectal cancer. Cancer Cell Int. 20:546. doi: 10.1186/s12935-020-01633-w
Champiat, S., Lambotte, O., Barreau, E., Belkhir, R., Berdelou, A., Carbonnel, F., et al. (2016). Management of immune checkpoint blockade dysimmune toxicities: a collaborative position paper. Ann. Oncol. 27, 559–574. doi: 10.1093/annonc/mdv623
Chen, H., Du, G., Song, X., and Li, L. (2017). Non-coding transcripts from enhancers: new insights into enhancer activity and gene expression regulation. Genom. Proteom. Bioinform. 15, 201–207. doi: 10.1016/j.gpb.2017.02.003
Chikuma, S. (2017). CTLA-4, an essential immune-checkpoint for T-Cell Activation. Curr. Top. Microbiol. Immunol. 410, 99–126. doi: 10.1007/82_2017_61
El-Serag, H. B., and Rudolph, K. L. (2007). Hepatocellular carcinoma: epidemiology and molecular carcinogenesis. Gastroenterology 132, 2557–2576. doi: 10.1053/j.gastro.2007.04.061
European Association for the Study of the Liver (2018). EASL clinical practice guidelines: management of hepatocellular carcinoma. J. Hepatol. 69, 182–236. doi: 10.1016/j.jhep.2018.03.019
Flecken, T., Schmidt, N., Hild, S., Gostick, E., Drognitz, O., Zeiser, R., et al. (2014). Immunodominance and functional alterations of tumor-associated antigen-specific CD8+ T-cell responses in hepatocellular carcinoma. Hepatology 59, 1415–1426. doi: 10.1002/hep.26731
Gabrusiewicz, K., Rodriguez, B., Wei, J., Hashimoto, Y., Healy, L. M., Maiti, S. N., et al. (2016). Glioblastoma-infiltrated innate immune cells resemble M0 macrophage phenotype. JCI Insight 1:85841. doi: 10.1172/jci.insight.85841
Garnelo, M., Tan, A., Her, Z., Yeong, J., Lim, C. J., Chen, J., et al. (2017). Interaction between tumour-infiltrating B cells and T cells controls the progression of hepatocellular carcinoma. Gut 66, 342–351. doi: 10.1136/gutjnl-2015-310814
Ge, P. L., Li, S. F., Wang, W. W., Li, C. B., Fu, Y. B., Feng, Z. K., et al. (2020). Prognostic values of immune scores and immune microenvironment-related genes for hepatocellular carcinoma. Aging 12, 5479–5499. doi: 10.18632/aging.102971
Goodman, A., Patel, S. P., and Kurzrock, R. (2017). PD-1-PD-L1 immune-checkpoint blockade in B-cell lymphomas. Nat. Rev. Clin. Oncol. 14, 203–220. doi: 10.1038/nrclinonc.2016.168
Gu, X., Wang, L., Boldrup, L., Coates, P. J., Fahraeus, R., Sgaramella, N., et al. (2019). AP001056.1, A prognosis-related enhancer RNA in squamous cell carcinoma of the head and neck. Cancers (Basel) 11:347. doi: 10.3390/cancers11030347
Hong, W., Liang, L., Gu, Y., Qi, Z., Qiu, H., Yang, X., et al. (2020). Immune-related lncRNA to construct novel signature and predict the immune landscape of human hepatocellular carcinoma. Mol. Ther. Nucleic Acids 22, 937–947. doi: 10.1016/j.omtn.2020.10.002
Hsieh, C. L., Fei, T., Chen, Y., Li, T., Gao, Y., Wang, X., et al. (2014). Enhancer RNAs participate in androgen receptor-driven looping that selectively enhances gene activation. Proc. Natl. Acad. Sci U S A 111, 7319–7324. doi: 10.1073/pnas.1324151111
Huang, H., Fu, S., and Liu, D. (2018). Detection and analysis of the hedgehog signaling pathway-related long non-coding RNA (lncRNA) expression profiles in keloid. Med. Sci. Monit. 24, 9032–9044. doi: 10.12659/MSM.911159
Huang, L., Wang, Z., Chang, Y., Wang, K., Kang, X., Huang, R., et al. (2020). EFEMP2 indicates assembly of M0 macrophage and more malignant phenotypes of glioma. Aging 12, 8397–8412. doi: 10.18632/aging.103147
Iliopoulos, D., Hirsch, H. A., and Struhl, K. (2009). An epigenetic switch involving NF-kappaB, Lin28, Let-7 MicroRNA, and IL6 links inflammation to cell transformation. Cell 139, 693–706. doi: 10.1016/j.cell.2009.10.014
Jayant, K., Habib, N., Huang, K. W., Podda, M., Warwick, J., and Arasaradnam, R. (2020). Immunological basis of genesis of hepatocellular carcinoma: Unique challenges and potential opportunities through immunomodulation. Vaccines (Basel) 8:247. doi: 10.3390/vaccines8020247
Kannan, K., Kordestani, G. K., Galagoda, A., Coarfa, C., and Yen, L. (2015). Aberrant MUC1-TRIM46-KRTCAP2 Chimeric RNAs in high-grade serous ovarian carcinoma. Cancers (Basel) 7, 2083–2093. doi: 10.3390/cancers7040878
Khan, I., Gril, B., and Steeg, P. S. (2019). Metastasis suppressors NME1 and NME2 Promote Dynamin 2 oligomerization and regulate tumor cell endocytosis, motility, and metastasis. Cancer Res. 79, 4689–4702. doi: 10.1158/0008-5472.CAN-19-0492
Kim, J. Y., Lee, E., Park, K., Park, W. Y., Jung, H. H., Ahn, J. S., et al. (2017). Immune signature of metastatic breast cancer: Identifying predictive markers of immunotherapy response. Oncotarget 8, 47400–47411. doi: 10.18632/oncotarget.17653
Kong, W., Wang, X., Zuo, X., Mao, Z., Cheng, Y., and Chen, W. (2020). Development and validation of an immune-related lncRNA signature for predicting the prognosis of hepatocellular carcinoma. Front. Genet. 11:1037. doi: 10.3389/fgene.2020.01037
Li, S., Huang, Y., Huang, Y., Fu, Y., Tang, D., Kang, R., et al. (2017). The long non-coding RNA TP73-AS1 modulates HCC cell proliferation through miR-200a-dependent HMGB1/RAGE regulation. J. Exp. Clin. Cancer Res. 36:51. doi: 10.1186/s13046-017-0519-z
Li, W., Hu, Y., Oh, S., Ma, Q., Merkurjev, D., Song, X., et al. (2015). Condensin I and II complexes license full estrogen receptor alpha-dependent enhancer activation. Mol. Cell 59, 188–202. doi: 10.1016/j.molcel.2015.06.002
Li, Y., Qi, D., Zhu, B., and Ye, X. (2021). Analysis of m6A RNA methylation-related genes in liver hepatocellular carcinoma and their correlation with survival. Int. J. Mol. Sci. 22:1474. doi: 10.3390/ijms22031474
Liang, J., Wei, X., Liu, Z., Cao, D., Tang, Y., Zou, Z., et al. (2018). Long noncoding RNA CYTOR in cancer: A TCGA data review. Clin. Chim. Acta 483, 227–233. doi: 10.1016/j.cca.2018.05.010
Liu, Y., Ding, M., Gao, Q., He, A., Liu, Y., and Mei, H. (2018). Current advances on the important roles of enhancer RNAs in gene regulation and cancer. Biomed. Res. Int. 2018:2405351. doi: 10.1155/2018/2405351
Liu, Y., Zhang, X., Lin, J., Chen, Y., Qiao, Y., Guo, S., et al. (2019). CCT3 acts upstream of YAP and TFCP2 as a potential target and tumour biomarker in liver cancer. Cell Death Dis. 10:644. doi: 10.1038/s41419-019-1894-5
Melo, C. A., Drost, J., Wijchers, P. J., van de Werken, H., de Wit, E., Oude Vrielink, J. A., et al. (2013). eRNAs are required for p53-dependent enhancer activity and gene transcription. Mol. Cell 49, 524–535. doi: 10.1016/j.molcel.2012.11.021
Mootha, V. K., Lindgren, C. M., Eriksson, K. F., Subramanian, A., Sihag, S., Lehar, J., et al. (2003). PGC-1alpha-responsive genes involved in oxidative phosphorylation are coordinately downregulated in human diabetes. Nat. Genet. 34, 267–273. doi: 10.1038/ng1180
Murakami, S., Gadad, S. S., and Kraus, W. L. (2015). A PreSTIGEous use of LncRNAs to predict enhancers. Cell Cycle 14, 1619–1620. doi: 10.1080/15384101.2015.1032650
Newman, A. M., Liu, C. L., Green, M. R., Gentles, A. J., Feng, W., Xu, Y., et al. (2015). Robust enumeration of cell subsets from tissue expression profiles. Nat. Methods 12, 453–457. doi: 10.1038/nmeth.3337
Nikas, J. B. (2016). Independent validation of a mathematical genomic model for survival of glioma patients. Am. J. Cancer Res. 6, 1408–1419.
Nishino, M., Ramaiya, N. H., Hatabu, H., and Hodi, F. S. (2017). Monitoring immune-checkpoint blockade: response evaluation and biomarker development. Nat. Rev. Clin. Oncol. 14, 655–668. doi: 10.1038/nrclinonc.2017.88
Pardee, A. D., and Butterfield, L. H. (2012). Immunotherapy of hepatocellular carcinoma: Unique challenges and clinical opportunities. Oncoimmunology 1, 48–55. doi: 10.4161/onci.1.1.18344
Sangro, B., Gomez-Martin, C., de la Mata, M., Inarrairaegui, M., Garralda, E., Barrera, P., et al. (2013). A clinical trial of CTLA-4 blockade with tremelimumab in patients with hepatocellular carcinoma and chronic hepatitis C. J. Hepatol. 59, 81–88. doi: 10.1016/j.jhep.2013.02.022
Shi, L., Peng, F., Tao, Y., Fan, X., and Li, N. (2016). Roles of long noncoding RNAs in hepatocellular carcinoma. Virus Res. 223, 131–139. doi: 10.1016/j.virusres.2016.06.008
Subramanian, A., Tamayo, P., Mootha, V. K., Mukherjee, S., Ebert, B. L., Gillette, M. A., et al. (2005). Gene set enrichment analysis: a knowledge-based approach for interpreting genome-wide expression profiles. Proc. Natl. Acad. Sci. U S A 102, 15545–15550. doi: 10.1073/pnas.0506580102
Switnicki, M. P., Juul, M., Madsen, T., Sorensen, K. D., and Pedersen, J. S. (2016). PINCAGE: probabilistic integration of cancer genomics data for perturbed gene identification and sample classification. Bioinformatics 32, 1353–1365. doi: 10.1093/bioinformatics/btv758
Wang, X., He, Q., Shen, H., Xia, A., Tian, W., Yu, W., et al. (2019). TOX promotes the exhaustion of antitumor CD8(+) T cells by preventing PD1 degradation in hepatocellular carcinoma. J. Hepatol. 71, 731–741. doi: 10.1016/j.jhep.2019.05.015
Xue, Z., Cui, C., Liao, Z., Xia, S., Zhang, P., Qin, J., et al. (2018). Identification of LncRNA Linc00513 containing lupus-associated genetic variants as a novel regulator of interferon signaling pathway. Front. Immunol. 9:2967. doi: 10.3389/fimmu.2018.02967
Yu, H., Pardoll, D., and Jove, R. (2009). STATs in cancer inflammation and immunity: a leading role for STAT3. Nat. Rev. Cancer 9, 798–809. doi: 10.1038/nrc2734
Yu, S., Li, N., Wang, J., Fu, Y., Huang, Y., Yi, P., et al. (2020). Correlation of long noncoding RNA SEMA6A-AS1 expression with clinical outcome in HBV-Related Hepatocellular Carcinoma. Clin. Ther. 42, 439–447. doi: 10.1016/j.clinthera.2020.01.012
Zamarron, B. F., and Chen, W. (2011). Dual roles of immune cells and their factors in cancer development and progression. Int. J. Biol. Sci. 7, 651–658. doi: 10.7150/ijbs.7.651
Zhang, Q., He, Y., Luo, N., Patel, S. J., Han, Y., Gao, R., et al. (2019). Landscape and dynamics of single immune cells in hepatocellular carcinoma. Cell 179, 829–845. doi: 10.1016/j.cell.2019.10.003
Zhang, Y., Zhang, L., Xu, Y., Wu, X., Zhou, Y., and Mo, J. (2020). Immune-related long noncoding RNA signature for predicting survival and immune checkpoint blockade in hepatocellular carcinoma. J. Cell Physiol. 235, 9304–9316. doi: 10.1002/jcp.29730
Keywords: non-coding RNA, immune signature, overall survival, tumor microenvironment, hepatocellular carcinoma
Citation: Cai S, Hu X, Chen R and Zhang Y (2021) Identification and Validation of an Immune-Related eRNA Prognostic Signature for Hepatocellular Carcinoma. Front. Genet. 12:657051. doi: 10.3389/fgene.2021.657051
Received: 22 January 2021; Accepted: 17 May 2021;
Published: 11 June 2021.
Edited by:
Yih-Horng Shiao, United States Patent and Trademark Office, United StatesReviewed by:
Qiyao Zhang, First Affiliated Hospital of Zhengzhou University, ChinaQingjia Chi, Wuhan University of Technology, China
Copyright © 2021 Cai, Hu, Chen and Zhang. This is an open-access article distributed under the terms of the Creative Commons Attribution License (CC BY). The use, distribution or reproduction in other forums is permitted, provided the original author(s) and the copyright owner(s) are credited and that the original publication in this journal is cited, in accordance with accepted academic practice. No use, distribution or reproduction is permitted which does not comply with these terms.
*Correspondence: Yiya Zhang, yiya0108@csu.edu.cn